Exploring the AtScale Semantic Layer for Business Intelligence
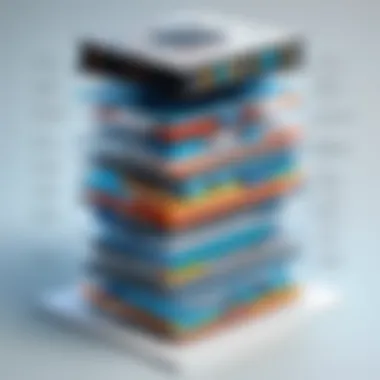
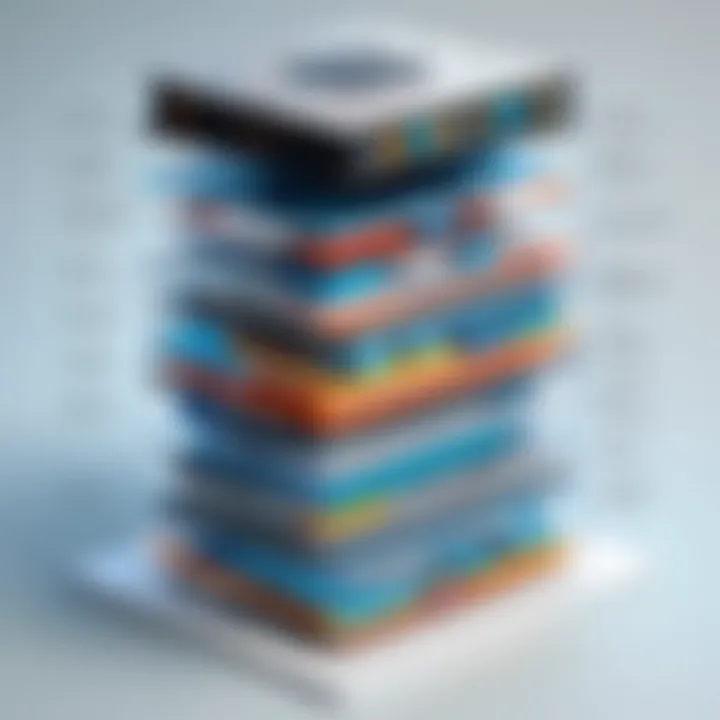
Intro
In today’s fast-paced world, organizations are swimming in terabytes of data. The real question, however, is not how much data they have, but how effectively they can utilize it. Enter the AtScale semantic layer, a solution that transforms raw data into valuable insights. Understanding this concept is crucial for anyone in the realm of business intelligence. It blends technology and real-world application, offering a bridge between complex data sets and actionable business strategies.
By creating a common framework, the AtScale semantic layer empowers decision-makers to navigate data complexities. This article aims to break down the intricacies of this structure, discuss its implications for data analytics, and outline the ways it can reshape business intelligence.
Technological Research Overview
Recent Technological Innovations
The digital ecosystem is witnessing rapid advancements, and the AtScale semantic layer is at the forefront of this revolution. Innovations like cloud computing, machine learning, and natural language processing have greatly influenced how organizations approach data. The AtScale platform integrates with tools like Tableau and Power BI, providing a robust framework for handling extensive datasets.
Impact on Business Operations
The influence of AtScale extends beyond mere data organization. Companies are now able to make faster decisions informed by real-time analytics. The ability to visualize data without being bogged down by technical jargon allows organizations to remain nimble. For example, retail chains can swiftly adjust inventory levels based on actionable insights derived from consumer behavior analytics. This agility translates into better customer satisfaction and, ultimately, enhanced profitability.
Future Technological Trends
Looking forward, several trends are emerging that are likely to shape the landscape of data analytics. The rise of automation technologies, for instance, implies that more organizations will lean on AI to derive insights faster than ever. Moreover, the increasing importance of data governance cannot be understated. Ensuring data integrity and compliance with regulations like GDPR will become paramount for successful data strategy.
Data Analytics in Business
Importance of Data Analytics
In the age of information, data is indeed the new oil. Effective data analytics allows businesses to identify patterns and trends, providing clarity amid uncertainty. Decision-makers relying on instinct alone may find themselves outpaced by competitors harnessing the full weight of data-science.
Tools for Data Analysis
While there are myriad tools available for data analysis, a few have emerged as powerhouses in the industry. Products like Microsoft Power BI, Google Data Studio, and AtScale itself offer unique capabilities. The choice of tool often depends on the specific needs of the business and the existing technological landscape.
Case Studies on Data-Driven Decisions
Numerous organizations lead the charge in using data analytics for making informed decisions.
- Netflix uses viewer data to personalize recommendations.
- Amazon employs analytics to optimize its supply chain.
These case studies illustrate how data-driven decision-making isn't just a buzzword—it's a competitive necessity.
Cybersecurity Insights
Threat Landscape Analysis
As data becomes more integral to business strategy, the cyber threat landscape grows more complex. Organizations must continually assess their vulnerabilities to protect valuable data assets.
Best Practices for Cybersecurity
Organizations should implement certain best practices to safeguard their data:
- Regularly update software and systems.
- Conduct employee training for phishing awareness.
- Ensure multi-factor authentication is applied wherever possible.
Regulatory Compliance in Cybersecurity
Maintaining compliance with regulations such as GDPR and CCPA is not just about avoiding fines; it also builds customer trust. Companies that handle data responsibly position themselves as leaders in ethical data practices.
Artificial Intelligence Applications
AI in Business Automation
AI is transforming various sectors, automating repeatable tasks and freeing up human resources for more complex activities. Utilizing AI-driven insights within the AtScale semantic layer can lead to improved operational efficiency.
AI Algorithms and Applications
Advanced algorithms are being deployed in areas ranging from customer service chatbots to sophisticated predictive analytics tools. By leveraging these technologies, businesses can forecast trends more accurately and respond proactively.
Ethical Considerations in AI
However, with great power comes great responsibility. Ethical considerations surrounding the use of AI algorithms are essential. Issues of bias in AI and data privacy need to be addressed transparently to maintain public trust.
Industry-Specific Research
Tech Research in Finance Sector
In finance, the AtScale semantic layer facilitates the blending of historical financial data with real-time market indicators. This integration helps financial analysts make informed recommendations swiftly.
Healthcare Technological Advancements
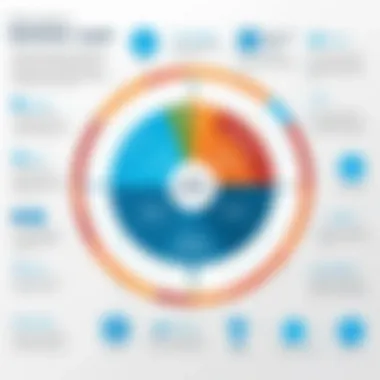
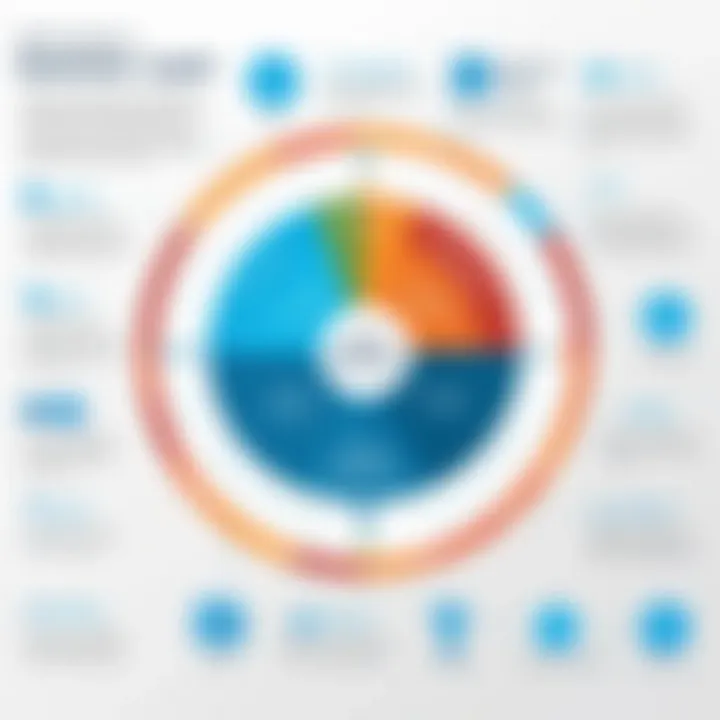
The healthcare sector is witnessing an upsurge in the use of data analytics for patient outcomes, and AtScale is paving the way for efficient data management that can analyze patient records quickly and effectively.
Retail Industry Tech Solutions
Retailers that adopt the AtScale semantic layer can streamline their inventory management and optimize their marketing strategies, leading to enhanced customer experiences.
Culmination
In summary, the AtScale semantic layer emerges as a game-changer in the realm of data analytics and business intelligence. It offers a much-needed structure that enables organizations to harness the power of data, fueling smarter decisions and fostering growth in an increasingly competitive environment.
Preamble to the AtScale Semantic Layer
In today ’s data-driven world, organizations are inundated with vast amounts of information. Navigating this sea of data to garner insights is both a challenge and an opportunity. The AtScale semantic layer is pivotal in transforming raw data into actionable intelligence, bridging the divide between complex datasets and user-friendly insights. This section delves into the importance of the AtScale semantic layer, highlighting its benefits and considerations.
The AtScale semantic layer acts like a translator between raw numerical data stored in databases and the human understanding of this information. It simplifies the data interaction process, rendering the analytics experience more intuitive for users, regardless of their technical expertise. Without this layer, many employees within the organization might find themselves struggling to pull insights from the available data, leading to underutilized resources and missed business opportunities.
Defining the Semantic Layer
A semantic layer functions essentially as an intermediary that converts data into a format that is easier to understand and work with. It materializes an organization’s data models, hierarchies, and business terms into a unified view that users can easily navigate. Imagine attempting to read a complex foreign book without a translation—it can be daunting. The semantic layer performs the essential role of translating complex data structures into recognizable components for users.
As organizations look to heighten their business intelligence capabilities, the semantic layer becomes crucial. It allows for the creation of visual dashboards that reflect real-time analytics, driving informed decision-making. Through user-centric models, it facilitates a self-service approach for data consumers. Users can pose queries, generate reports, and analyze trends without consistently relying on IT or data professionals, which fosters a culture of data-driven decision-making across all levels of the organization.
Historical Context and Evolution
The concept of a semantic layer isn't entirely new; it has roots that trace back to the early development of data warehousing and business intelligence. Initially, data analytics tools required users to have a firm grasp of the underlying technologies and data structures. Over time, the need for enhanced data accessibility and self-service capabilities led to the evolution of this concept, paving the way for the AtScale semantic layer.
AtScale emerged as a response to the growing volume of data generated by organizations. As businesses began to realize the potential locked within their data, they also noticed the bottlenecks that traditional data approaches created. The semantic layer was developed to empower users, allowing them to gain insights without needing advanced queries or complex understanding of the data infrastructure.
This historical transition marks a significant shift in how organizations perceive and utilize data. From a technical resource available to a select few, data analysis has now become an accessible tool for many. In this modern context, the AtScale semantic layer plays an invaluable role, ensuring that businesses can harness their data efficiently and strategically.
"The AtScale semantic layer is like a lighthouse guiding ships to harbor, ensuring they navigate through the waves of data to reach safe shores of understanding."
As we continue to explore the significance of the AtScale semantic layer in data analytics, it’s important to realize how deeply it intertwines with organizational success. This capability not only democratizes data but also enhances overall operational effectiveness, setting the stage for well-informed strategies and outcomes.
Significance of Semantic Layers in Data Analytics
In today’s data-driven world, recognizing the significance of semantic layers is vital for any organization looking to extract actionable insights. Semantic layers serve as a bridge between complex data sources and user-friendly formats, turning raw data into valuable information that fuels decision-making.
By utilizing a semantic layer like AtScale, organizations can reduce the barriers to data access, enhancing their ability to analyze and interpret it. Many business leaders may not realize that these layers are not just technical components but foundational elements that empower teams. The importance can be broken down into several key areas:
- Transformation of Data
Semantic layers convert intricate data structures into understandable business terms. This allows users, regardless of their technical expertise, to engage with data intuitively. - Improved Decision-Making
When data is comprehensible, it leads to faster and better decisions. Business professionals don’t need to wade through heaps of unreadable data; they can focus on insights that matter. - Fostering Collaboration
These layers standardize data definitions across the organization, ensuring everyone is on the same page. Common language fosters discussion, makes collaboration seamless, and reduces miscommunication. - Agility in Reporting
Semantic layers allow users to create reports and dashboards rapidly. The ease of accessing data means that companies can adapt to changes in the market quickly.
Bridging Data and Business Insight
Semantic layers play an essential role in converting data into meaningful insights. Imagine having a treasure trove of information, yet not being able to find the gems within it. Semantic layers help in mining data effectively.
By transforming raw data into a business-friendly format, teams can apply analytics without needing to understand the cybersecurity micro-details or complicated data join processes. For instance, a marketing team can analyze customer behavior without having to depend on IT or data analysts, thereby streamlining their workflows.
The reduced dependency on specialized technical staff means that more stakeholders within a company can derive insights swiftly. This is crucial, especially for fast-paced environments where time is often of the essence.
Facilitating Self-Service BI
Often, the bottleneck to effective data usage within organizations is the reliance on IT departments for analytics. Self-service Business Intelligence (BI) solutions are becoming increasingly important, and semantic layers are the linchpin facilitating this transition.
They provide an interface where non-technical users can sift through mountains of data effortlessly. With tools like AtScale, employees can simply drag and drop fields, apply filters, and generate reports without having extensive training on SQL or understanding raw database structures. This democratization of data access increases organizational agility.
Moreover, the incorporation of user-friendly visualization tools within semantic layers enhances the user experience. A well-designed dashboard can reveal trends and patterns that are not easily visible otherwise.
Such an approach not only enriches the analytical landscape but also encourages a culture of data-driven decision-making. Employees from various departments begin to view data as a trapdoor to innovation rather than a locked vault.
In summary, the significance of semantic layers is profound; they bridge the complex world of data analytics with everyday business needs. Organizations that embrace self-service BI through these layers are better poised to leverage their data as a strategic asset. By doing so, they ensure that insights flow freely throughout the organization, fostering a culture of continuous improvement.
Technical Architecture of AtScale Semantic Layer
Understanding the technical architecture of the AtScale semantic layer is crucial as it lays the groundwork for transforming raw data into useful insights. This layer ensures that organizations can efficiently navigate an ocean of information while enabling decision-makers to derive meaningful patterns. Notably, the architecture encompasses several core components that play a pivotal role in achieving seamless data integration and accessibility. Additionally, the ability to harmonize with existing data infrastructures is essential for a smooth transition.
Core Components
The core components of the AtScale semantic layer serve as the building blocks to foster an effective data environment. These parts not only streamline data processes but also enhance analytics capabilities. Here are the primary constituents:
- Data Virtualization: This allows users to access multiple data sources without needing to duplicate them. It provides a unified view, making it less cumbersome to analyze contrasting data sets simultaneously.
- Semantic Modeling: Semantic models act as a translator between technical data terms and business terminology. This embedded logic helps to ensure that all stakeholders interpret the information consistently, enhancing clarity and reducing the risk of miscommunication.
- Query Engine: The query engine is responsible for running requests against the integrated data, optimizing for performance. The ability to handle complex queries efficiently is vital for real-time insights and operational decision-making.
Mentioning technologies like Apache Kafka or Presto as part of the infrastructure can further illustrate how data can be processed at scale while maintaining speed and accuracy. These tools, integral to the architecture, ensure data flows efficiently from storage to presentation, keeping latency to a minimum.
Integration with Existing Data Infrastructure
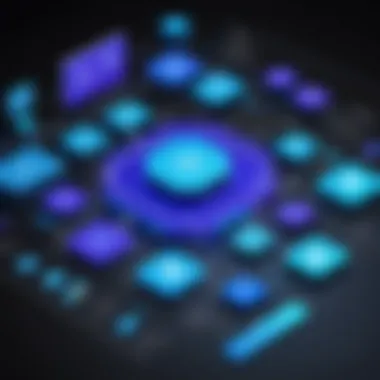
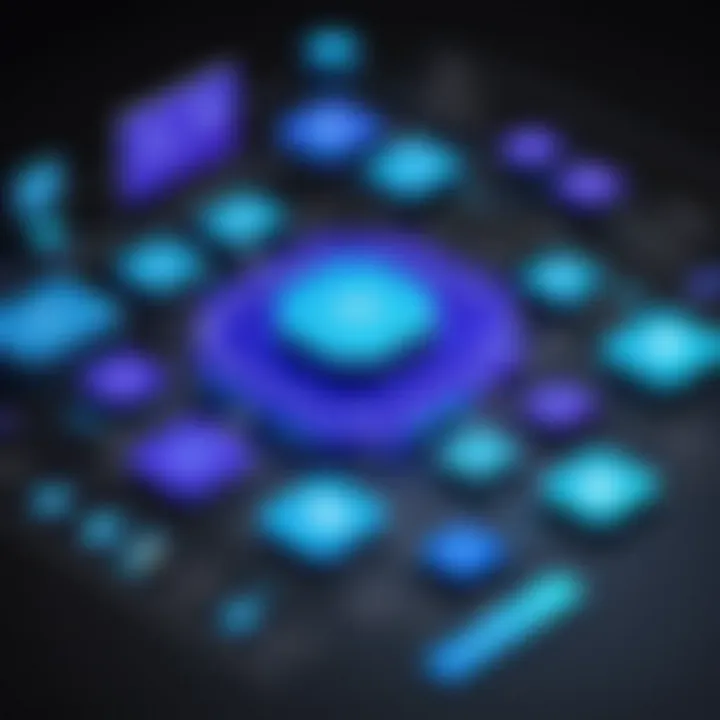
Integrating the AtScale semantic layer with existing business data infrastructures is not merely a technical task; it’s a strategic maneuver. Organizations often possess a melting pot of data storage solutions, from data lakes to traditional data warehouses. Hence, having a semantic layer that harmonizes seamlessly with these diverse systems is beneficial.
Here’s why this integration is important:
- Maximizes Existing Investments: Many enterprises have already invested in data without wanting to uproot their current systems. The AtScale layer provides a way to leverage what is already in place, enhancing its utility rather than starting from scratch.
- Reduced Complexity: By integrating directly with established infrastructures, the semantic layer simplifies the complexity of dealing with disparate data sources, making it easier for users to access and analyze information.
- Real-time Data Processing: Seamless integration also paves the way for more timely insights. As data is updated in its source, the semantic layer can provide near real-time analytics, a prime necessity for today’s fast-paced decision-making environments.
"A well-integrated semantic layer acts like a bridge, aligning data landscapes with business goals to create actionable intelligence."
Functionalities of AtScale Semantic Layer
The AtScale semantic layer brings a host of functionalities that transform raw data into valuable business intelligence. It serves as a bridge between complex data silos and everyday analytics. Below, we dive deep into its key functionalities, which are crucial for organizations looking to derive actionable insights from their data.
Data Modeling and Virtualization
Data modeling is the backbone of any analytics strategy, and AtScale’s capability in this area is noteworthy. With AtScale, users craft a model that mirrors their business needs without getting tangled up in technical jargon. This approach allows businesses to define metrics and dimensions that resonate with their operations.
In practicing virtualization, AtScale manages to access data from various sources while keeping it in its original location. There's no need for the physical replication of data, which can be both costly and time-consuming.
Moreover, AtScale’s semantic layer supports dimensional modeling techniques, making it easier for users to intuitively navigate through what might otherwise be a labyrinth of data points.
"The power of AtScale lies in its capability to create a semantic model that acts as a universal translator for diverse data landscapes, simplifying complexity into clarity."
Real-Time Data Access and Query Performance
In our fast-paced business world, timing is everything. Quick, real-time access to data can be the difference between seizing an opportunity and watching it slip away. AtScale shines in this arena by providing efficient querying capabilities, significantly reducing the time between data ingestion and actionable insights.
With the ability to execute queries across massive datasets seamlessly, professionals are empowered to make decisions backed by up-to-the-minute data. This capability not only enhances efficiency but also offers reassurance that the information guiding decisions is current and relevant.
The underlying technology leverages advanced caching mechanisms and optimizations, ensuring that users can retrieve data without straining the underlying systems, thus maintaining performance integrity while accessing large sets of data.
Enhanced Data Governance and Security
As organizations navigate through the murky waters of data privacy and compliance, AtScale's commitment to data governance and security becomes increasingly essential. The platform offers robust access controls and audit trails. This ensures that sensitive data is only accessible to those with the right privileges, aligning with compliance mandates.
Data governance is further augmented through defined business rules and standards that guide data usage. By standardizing how data is interpreted across the organization, AtScale helps mitigate the risks associated with data misinterpretation or misuse.
Additionally, the semantic layer’s architecture promotes clear documentation of data lineage. This is a crucial aspect that enables stakeholders to trace the origins and transformations of data over time, bolstering transparency and accountability in data practices.
Practical Applications in Business Settings
The practical applications of the AtScale semantic layer are crucial for translating complex data into actionable insights. In today’s fast-paced business environment, organizations must leverage data effectively to remain competitive. Understanding how the semantic layer operates in real-world settings ultimately solidifies its role as an essential tool in business intelligence strategies.
The real power of the AtScale semantic layer lies in its ability to bridge raw data and meaningful analysis. By encapsulating data models that align with business semantics, it empowers users to query data without needing deep technical expertise. This leads to faster decision-making and a more agile response to market demands.
- Greater Accessibility: One of the standout features of the semantic layer is its promise of self-service capabilities. Business users can easily tap into complex datasets without dependency on IT teams, reducing bottlenecks and promoting cross-functional collaboration.
- Informed Decision Making: In many instances, companies have realized that timely access to reliable data can shift the tides in their favor. With the AtScale semantic layer, users gain insights that directly influence strategic decisions, turning data into a narrative that can guide organizational direction.
- Efficiency in Analysis: The capability of creating reusable data models means organizations can save time. Instead of reinventing the wheel for every new analysis, they can utilize well-crafted models that reflect business realities, which streamlines the analysis process considerably.
- Scalability: As businesses grow and expand, their data needs evolve. A robust semantic layer allows for easy scaling, adapting to more complex datasets while maintaining performance levels, thus ensuring that businesses are not hampered by their data structures.
"The AtScale semantic layer doesn’t just support decision-making; it transforms the way organizations perceive and manage their data."
Case Studies: Success Stories
Exploring specific case studies offers a clear lens into how organizations have successfully implemented the AtScale semantic layer. Take, for example, a major retail chain that faced the dilemma of integrating vast amounts of sales data from multiple channels. By employing the AtScale semantic layer, they not only improved consistency in reporting but also enabled their marketing team to derive insights more quickly than before.
This initiative led to 30% increase in targeted campaign performance, underlining how critical rapid data analysis and accessibility can be in competitive industries.
Another example can be seen in the financial sector, where a leading bank harnessed the power of AtScale to streamline its risk assessment processes. Now, the institution can assess risk scenarios in real-time, allowing for more informed lending decisions.
Industry-Specific Use Cases
The applicability of the AtScale semantic layer extends across various industries, each with its own unique needs. In the healthcare sector, for instance, organizations utilize this technology to navigate extensive patient data for operational efficiencies and enhanced patient outcomes. Using the semantic layer, healthcare systems achieve a more holistic view of patient interactions, leading to improved care strategies.
In the realm of marketing, companies leverage the semantic layer to better understand customer behavior across different campaigns. This not only improves targeting but also leads to higher conversion rates by ensuring that marketers are equipped with the right insights at the right time.
Furthermore, in the manufacturing sector, firms are increasingly relying on the AtScale semantic layer to optimize supply chains. By tapping into data from various stages of production, manufacturers can identify inefficiencies and reduce costs, ultimately increasing their bottom line.
Challenges and Limitations
Understanding the challenges and limitations surrounding the AtScale Semantic Layer is crucial for organizations aiming to enhance their data-driven practices. Despite the many advantages it offers, such as improved accessibility to data and streamlined business intelligence processes, several hurdles exist that can hinder its effective deployment and utilization. Addressing these challenges is vital to ensure that the potential benefits are fully realized while giving due consideration to the practical scenarios businesses face.
Data Quality and Integration Issues
Data quality stands as a cornerstone in any data-driven strategy. The capabilities of the AtScale Semantic Layer can be significantly impacted by the quality of the underlying data. If the data fed into the system is inaccurate, incomplete, or inconsistently formatted, the insights drawn will be fraught with complications. This leads to erroneous conclusions, which can compromise decision-making and strategic planning.
Moreover, integration issues can arise when organizations attempt to consolidate disparate data sources within the semantic layer. Many companies boast a patchwork of data storage solutions — from cloud services like Amazon S3 to on-premises databases such as Microsoft SQL Server. Aligning these varied architectures can be a daunting task. If the data sources do not integrate seamlessly, it can severely disrupt the flow of information, causing delays and misunderstandings among stakeholders.
To navigate these complications, organizations might consider several strategies:
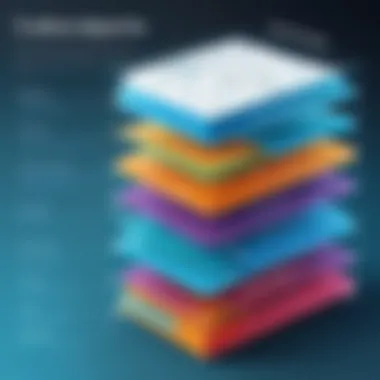
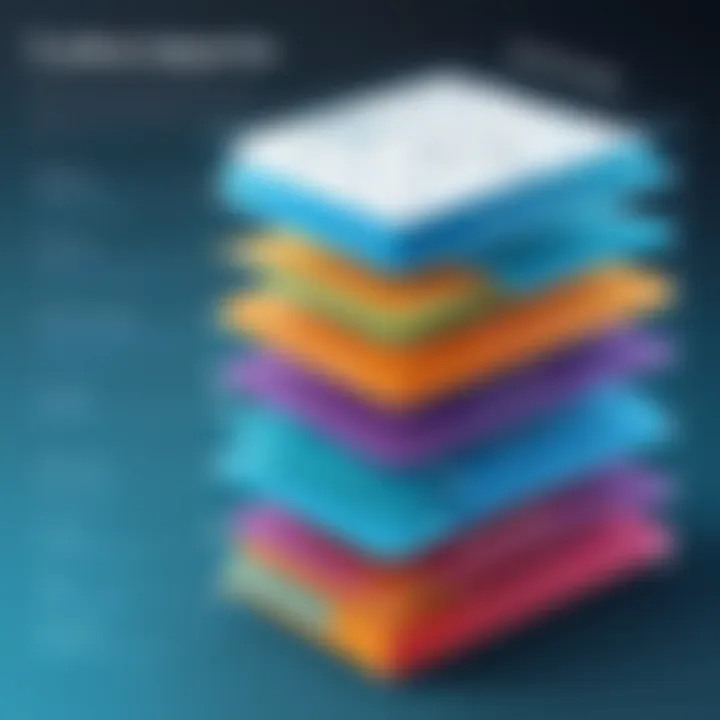
- Regular Data Auditing: Periodically reviewing data quality ensures that inaccuracies are addressed promptly, fostering trust in the insights generated.
- Robust Integration Frameworks: Employing well-established integration solutions can help unify data from diverse sources into the semantic layer.
"Data is only as good as the context it resides in. For the AtScale Semantic Layer to shine, clean and integrated data is a must."
User Adoption and Training Concerns
Even the most groundbreaking technology can fall flat if users are reluctant to embrace it. User adoption is often a critical yet challenging aspect of the AtScale Semantic Layer. Employees may find themselves overwhelmed by new processes or skeptical of the system's effectiveness. This reluctance can stem from previous experiences with inadequate tools or a lack of understanding of the benefits the semantic layer offers.
Furthermore, training concerns play a vital role in user adoption. Without adequate training materials and support, users might struggle to leverage the advantages of the AtScale Semantic Layer fully. This situation can lead to frustration, decreased productivity, and ultimately, a resistance to utilizing the new system altogether.
To mitigate these risks, organizations ought to:
- Invest in Comprehensive Training Programs: Implementing hands-on training sessions and providing accessible resources can enhance user confidence and competence.
- Engagement Initiatives: Involving users in the implementation process and soliciting their feedback fosters a sense of ownership over the new technology, often leading to a smoother transition.
By addressing these challenges and limitations effectively, organizations can pave the way for a more fruitful integration of the AtScale Semantic Layer into their data-driven processes. This not only optimizes their business intelligence capabilities but also contributes to a culture that values data literacy.
Future Trends and Innovations
In the fast-paced world of data analytics, staying ahead of the curve is essential. The AtScale semantic layer represents a crucial move toward streamlining data processes, and looking into the future, we can identify several emerging trends that will shape its evolution. These innovations are not just shiny baubles; they provide tangible benefits and considerations for businesses eager to harness data more effectively.
Emerging Technologies Impacting Semantic Layers
As technology continues to evolve, a few key trends are taking shape that will significantly impact semantic layers. Here are some noteworthy examples:
- Real-Time Data Processing: The ability to process data as it comes in rather than relying on batch processing can offer businesses timely insights. Tools designed to manage streaming data will likely integrate seamlessly with the AtScale layer, enhancing its responsiveness.
- Graph Databases: The use of graph technology can facilitate a deeper understanding of relationships within data. This tech offers a flexible way to model interconnected information and could enrich the semantic layer by providing more context around data points.
- Cloud Computing: With cloud infrastructures becoming the norm, integrating the AtScale layer with cloud-based solutions can propel flexibility and scalability. This shift allows for more dynamic data management and real-time analytics, enabling businesses to make quicker, informed decisions.
- No-Code/Low-Code Platforms: These platforms are democratizing data analytics by allowing individuals without deep technical expertise to engage with data. Integrating AtScale within these tools can enhance their usability and broaden the number of users who can derive insights from complex datasets.
This evolution highlights the necessity for organizations to consider how they can leverage these technologies to enhance their use of the AtScale semantic layer. It's about making data not only more accessible but also more actionable for a broader range of users.
The Role of AI and Machine Learning
AI and machine learning play pivotal roles in evolving the capabilities of the AtScale semantic layer. Their influence permeates various aspects, from improved decision-making to enhanced data processing efficiency. Here’s how these technologies are making waves:
- Automated Data Insights: Machine learning algorithms can sift through vast amounts of data to identify patterns and offer insights that might be missed otherwise. By integrating these capabilities within the semantic layer, businesses can better predict trends and behaviors, leading to more informed strategies.
- Natural Language Processing (NLP): NLP can facilitate smoother interactions between users and data. It enables users to query data using everyday language, removing the barrier that often comes with needing to know complex querying languages. This is where the semantic layer truly shines, as it translates the user’s intent into actionable queries.
- Anomaly Detection: AI can help in identifying outliers—data points that deviate from the norm which could signify risks or opportunities. By enhancing the semantic layer’s ability to highlight these anomalies, organizations can proactively address issues before they snowball.
- Personalized Analytics: Machine learning techniques can personalize the data experience by tailoring analytics based on user preferences and behaviors. This customization elevates user engagement and ensures that decision-makers receive the most relevant information in a digestible format.
In short, the inclusion of AI and machine learning within the AtScale semantic layer not only facilitates advanced analytics but also fosters a more inclusive and intuitive data interaction experience.
"The future of data analytics is not just about collecting data; it’s about transforming it into a story that drives business success."
As we navigate through these trends and innovations, it's clear that the AtScale semantic layer will continue to evolve. Embracing these advances ensures that organizations can optimize their data ecosystems, positioning them for success in a data-driven world.
Comparative Analysis with Alternative Technologies
In the realm of data analytics and business intelligence, conducting a comparative analysis with alternative technologies serves as a beacon for organizations wrestling with the churning sea of data. The AtScale semantic layer presents a unique approach that can significantly alter how businesses harness their data. It’s essential to weigh the benefits against other available technologies, particularly to pinpoint the most effective solutions for specific organizational needs.
AtScale versus Traditional Data Warehousing
Traditional data warehousing has long been regarded as the gold standard for storing and managing vast data resources. However, it operates on a structured approach that often leads to data silos, leaving users cautious about extracting insights without significant groundwork in data preparation. The AtScale semantic layer adds a twist to this scenario. Instead of relying on a heavyweight structure, AtScale offers a more agile, virtualized approach.
- Flexibility: Unlike traditional methods, AtScale lets users interact with data without needing to move it around excessively, speeding up the analysis process. This agility can be especially useful in fast-paced business settings where timing is everything.
- Cost Efficiency: Implementing AtScale might reduce the overall expense by cutting down on the need for extensive data engineering resources and the associated costs of maintenance—funds better spent on enhancing business capabilities.
- Self-Service Capabilities: AtScale empowers end-users, providing them with the ability to query data without always seeking aid from IT departments. This encourages a culture of data-driven decision-making, where employees at various levels become data champions rather than mere consumers.
However, traditional data warehouses do have their merits, particularly in terms of structured governance. They are often excellent for compliance and stringent regulations. For companies with established data governance frameworks, moving to a semantic layer could take some adjustment, but a successful integration could result in a streamlined experience overall.
Semantic Layer vs. Business Intelligence Platforms
Analyzing the difference between a semantic layer and traditional business intelligence platforms reveals a significant divergence in purpose and functionality. Business intelligence tools like Tableau or Power BI often come equipped with advanced visualization capabilities but typically require strong foundational data warehousing. The semantic layer supplements these BI tools by acting as a bridge between raw data and insights.
- Real-Time Insights: With a semantic layer, data is accessible in real time, allowing immediate insights that drive timely decision making. In comparison, many BI tools must interact with their repository, which might not always reflect the most current data.
- Unified Data Access: The semantic layer standardizes access to disparate data sources, breaking down barriers that BI platforms may encounter when dealing with different data types and formats.
- Enhanced Governance: Incorporating a semantic layer can streamline data governance, ensuring that users across the business are aligned concerning data definitions and metrics. This alignment is less consistent in typical BI systems where users may interpret data differently due to varying context.
"The semantic layer serves as the interpreter that translates raw data into business-friendly language, enabling organizations to derive genuine insights that align with their strategic goals."
Ending and Key Takeaways
The discussion surrounding the AtScale semantic layer ultimately underscores its vital role in modern business intelligence landscapes. As organizations increasingly rely on data to inform decision-making, the ability to efficiently connect varied data sources and present them in a user-friendly manner cannot be overstated. This article has illuminated several key elements that highlight the significance of the AtScale semantic layer.
Summarizing the Strategic Importance
The AtScale semantic layer acts as a bridge, turning raw data into actionable insights. By enabling self-service capabilities for users, it empowers decision-makers across all levels to access and analyze data without being bogged down by technical queries. In environments often saturated with information, having a clear, coherent model to interact with is not just a convenience, but a necessity.
Organizations that adopt the AtScale semantic layer can expect several benefits:
- Enhanced Decision-Making: With faster access to accurate data, stakeholders can make informed choices without delays.
- Time Efficiency: Reducing dependencies on IT for routine analytics speeds up insights and frees up tech teams for strategic projects.
- User Empowerment: By allowing business users to explore data freely, the semantic layer promotes a culture of data-driven decision making.
In essence, integrating the AtScale semantic layer positions a company for greater agility in data management and responsiveness to market changes.
Recommendations for Implementation
Implementing the AtScale semantic layer requires thoughtful consideration and strategic planning. Here are some tailored recommendations for organizations looking to embark on this journey:
- Assess Your Data Needs: Understand the specific analytics requirements of your organization. Tailor the implementation to focus on the areas that will provide the most value.
- Involve Key Stakeholders: Engage with various departments to get buy-in and understand their data challenges. This collaborative approach will help in designing a semantic layer that meets diverse needs.
- Invest in Training: Adequate training for users is critical for fostering adoption. Consider workshops or ongoing support to help users navigate the new tools confidently.
- Prioritize Data Quality: Ensure that the data feeding into the semantic layer is clean and consistent. Engaging with IT to establish robust data governance practices is essential to maintain accuracy and reliability.
- Measure and Iterate: After implementation, continuously monitor usage and gather feedback. Analyze how the semantic layer is enhancing decision-making and adapt accordingly.
In closing, the AtScale semantic layer is a pivotal tool that can reshape how an organization approaches data analysis. By taking calculated steps for its implementation, businesses can capitalize on the wealth of information at their disposal, ensuring that they remain competitive in a data-centric world.