Navigating the Big Data and AI Landscape in 2021
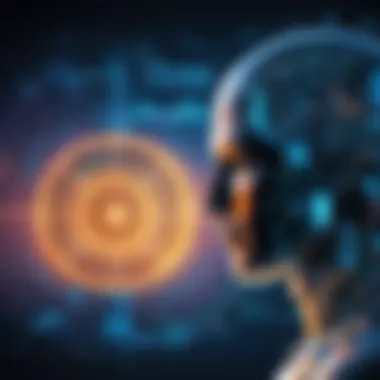
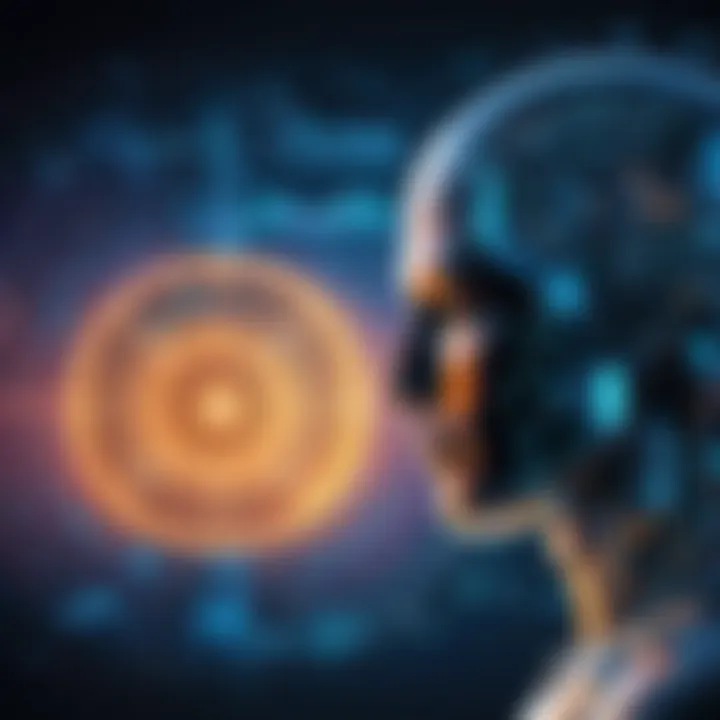
Intro
The landscape of Big Data and Artificial Intelligence (AI) in 2021 is shaped by both constant technological advancements and evolving business needs. Organizations worldwide increasingly rely on data-driven strategies that are informed by sophisticated AI systems. This article seeks to analyze the interaction of these technologies and their implications across various industries. The goal is to provide industry professionals with robust insights into how to effectively leverage Big Data and AI for achieving operational excellence and strategic growth.
Technological Research Overview
Recent Technological Innovations
In 2021, several key innovations have emerged in the realm of Big Data and AI. Companies are now utilizing machine learning frameworks like TensorFlow and PyTorch for complex data interpretations. The advancements in Natural Language Processing have provided avenues for better customer interactions through chatbots and sentiment analysis tools. These innovations bridge the gap between large data sets and actionable insights, allowing for improved decision-making processes.
Impact on Business Operations
The impact of Big Data and AI on business operations is profound. Efficient data handling can lead to significant enhancements in business workflows. Analyses of customer preferences inform tailored marketing strategies. Moreover, predictive analytics revolutionize supply chain management, leading to reduced costs and timely deliveries. The interdependence of Big Data and AI ensures businesses can make well-informed decisions, streamlining their operations and maximizing profitability.
Future Technological Trends
Looking ahead, several trends appear poised to reshape the landscape further. Automation technologies will likely become more integrated into daily processes, making companies agile and resilient. The growth of augmented analytics will signify a shift toward using AI to assist in data preparation and self-service analytics. More organizations will embrace integration with cloud-based platforms where accessibility and collaboration among global teams enhance research and function seamlessly.
Innovative approaches to utilizing Big Data and AI will define success in a competitive marketplace.
Data Analytics in Business
Importance of Data Analytics
Understanding the importance of data analytics is critical for any organization in 2021. Data transforms potential opportunities into sustainable strategies. Regardless of industry, data-driven approaches help navigate uncertainties and enhance service delivery through in-depth customer insights.
Tools for Data Analysis
Various tools facilitate effective data analysis. Software like Microsoft Power BI and Tableau allows companies to visualize data and derive insights efficiently. Other platforms, such as Apache Hadoop, provide the infrastructure for managing large scale data sets. Fewer organizations can brush aside data analysis tools if they want to remain competitive.
Case Studies on Data-Driven Decisions
Prominent case studies reflect the effectiveness of data-driven decision-making. For instance, Netflix uses sophisticated recommendations powered by AI to enhance user engagement while Amazon utilizes user data analytics to refine inventory and sales strategies. These real-world examples depict the tangible benefits achievable by integrating data insights into core business functions.
Cybersecurity Insights
Threat Landscape Analysis
As technology advances, so does the threat landscape. 2021 has been marked by a rise in cyberattacks targeting sensitive data. Company infrastructures have grown increasingly complex, leading to diverse vulnerabilities. Thorough understanding of this ongoing evolution is vital in mitigating risks.
Best Practices for Cybersecurity
Establishing a robust cybersecurity framework should be a priority. Organizations must prioritize regular security audits, training employees on phishing attempts, and implementing multi-factor authentication. Best-practice guidelines promote a security culture within teams, proactively addressing digital threats.
Regulatory Compliance in Cybersecurity
Additionally, adhering to regulatory standards such as General Data Protection Regulation (GDPR) is crucial for business continuity. Combinations of technology and regulatory frameworks assist companies in building trust among consumers while maintaining ethical validity about data handling practices.
Artificial Intelligence Applications
AI in Business Automation
Automation has been a key application of AI technology. Many companies increasingly rely on AI to automate mundane tasks. This transition not only increases efficiency but frees human resources for complex problem-solving that can lead to strategic innovations.
AI Algorithms and Applications
Different AI algorithms are being applied across fields. Businesses leverage predictive analytics to plan marketing strategies, using regression analysis and neural networks to determine customer behavior. This gives businesses advantages on knowing predicable outcomes with real-time data inputs.
Ethical Considerations in AI
The rise of AI begs questions about its ethical use. It is crucial for companies to maintain transparency about how they utilize AI technologies. Balancing AI advancements with ethical considerations in areas such as customer privacy requires careful navigation to ensure responsibility.
Industry-Specific Research
Tech Research in Finance Sector
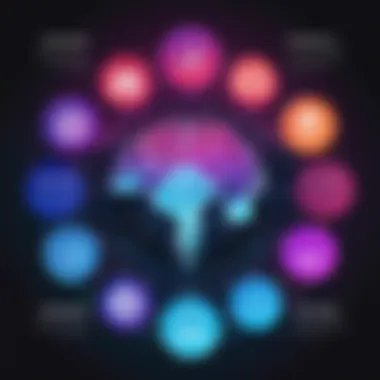
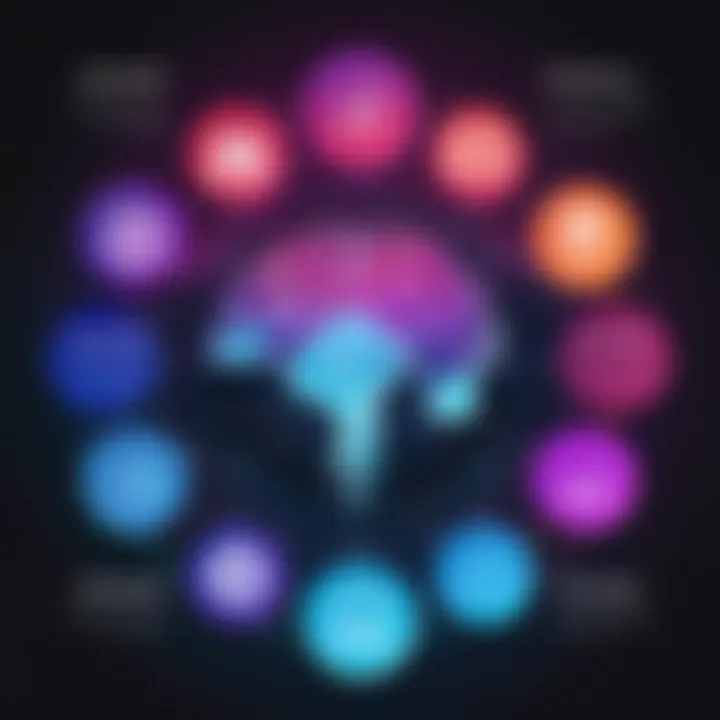
The finance sector has aggressively adopted Big Data technologies for risk assessment and compliance monitoring. Enhanced analytics lead to improved investment strategies, constantly refining risk models.
Healthcare Technological Advancements
In healthcare, Big Data plays a transformative role in patient care management. Data analytics lead to personalized treatment options, resulting in better patient outcomes through informed decision-making.
Retail Industry Tech Solutions
Finally, the retail industry has employed data to optimize inventory and enhance customer experiences. Real-time data collection aids in stocking products based on purchasing patterns, allowing retailers to satisfy demand efficiently.
Overview of Artificial Intelligence
Artificial Intelligence (AI) involves the simulation of human intelligence processes by machines. This includes learning, reasoning, and self-correcting. AI technology is transforming how decisions are made across various industries by enabling machines to analyze data and execute tasks that typically require human intelligence.
Key Components of AI
- Machine Learning: Algorithms enable systems to learn from and make predictions or decisions based on data.
- Natural Language Processing: Allows computers to understand and respond to human language.
- Computer Vision: Gives computers the ability to interpret visual information from the world.
- Robotics: Involves the design and use of robots for various applications.
Through its various applications, AI enhances operational efficiencies, improves customer experience, and opens new pathways for business growth. It complements Big Data by helping organizations extract actionable insights from massive data sets, ultimately leading to better decision-making.
The Interconnection Between Big Data and AI
In an era where data becomes invaluable, the relationship between Big Data and Artificial Intelligence is crucial. Both domains inform and enhance each other, creating myriad opportunities for businesses and organizations. This connection leads to innovative solutions and smarter decision-making strategies. Understanding this symbiosis is essential for any professional navigating today's data-driven environment.
Understanding Data-Driven Decisions
Data-driven decisions utilize insights from data analytics. This approach differs from intuition or experience-based decisions. It emphasizes evidence for making choices. In 2021, professionals are realizing that relying on qualitative insights alone is insufficient. Organizations incorporating data analysis into their strategy usually outperform competitors.
Effective data-driven decision-making derives clarity from vast amounts of information, guiding organizations toward targeted objectives.
Effective use of data improves accuracy. Analysis allows firms to identify trends, patterns, and anomalies within datasets. For instance, capturing consumer behavior enhances marketing strategies. Companies often derive predictive insights from historical data, leading to timely and relevant responses. Better forecasting is achieved, resulting in increased returns on investment and minimized waste.
To aid in understanding data-driven decisions, consider:
- Data collection: Gathering currect and relevant data is foundational. Without quality data, choices become unreliable.
- Data analysis tools: There are various tools and software that help to elucidate the meaning behind collected data. They assist in parsing through vast information efficiently.
- Cultural shifts: Encouraging data literacy across an organization enables all employees to engage with the insights generated.
AI's Role in Processing Big Data
AI serves a pivotal function in managing big data. With the enormity of data generated daily, human analysis falls short. This is where AI shines. Machine learning algorithms and deep learning help process and interpret vast pools of information at accelerated rates.
The advantages of utilizing AI for processing big data include:
- Automation: AI systems automate repetitive tasks associated with data handling. This allows data analysts to focus on strategic work over mundane activities.
- Pattern recognition: AI excels in identifying patterns that humans may overlook. These patterns inform product recommendations and risk assessments among others.
- Scalability: AI solutions adapt to increases in data volumes conveniently, facilitating dynamic processing without compromising efficiency.
Additionally, AI brings machine adaptability. Models can evolve as new data enters systems, ensuring that the insights remain relevant over time. For instance, merchant personable services customized based on individual shopping history often yield high satisfaction and lead to consistent repeat business.
In summary, the intertwining of Big Data and AI fosters an enhanced, analytical approach to operational strategy. As organizations adapt to changes, they must embrace this integration to stay ahead in their respective markets.
Key Trends in Big Data and AI for
In the fast-evolving world of technology, understanding the key trends in Big Data and AI for 2021 is essential. These trends present both challenges and opportunities. Companies that capitalize on these developments can drive growth, enhance operational efficiencies, and establish themselves as leaders in their respective fields. Here, we explore significant trends shaping this landscape.
Increased Adoption of Cloud Computing
Cloud computing continues to gain traction. More organizations are shifting their data management and processing to cloud environments. The cloud offers scalability, flexibility, and significant cost reductions compared to traditional IT infrastructure. Businesses can leverage powerful computing resources on-demand without hefty upfront investments.
This trend opens new possibilities in data storage and processing. Companies can easily expand their infrastructure to handle surges in data, ensuring continuity in service delivery. Moreover, cloud solutions often come with advanced security features, thus alleviating some concerns around data breaches.
Key characteristics of this increased adoption include:
- Cost Savings: Reduced spend on maintenance and hardware.
- Enhanced Collaboration: Teams can work together in real-time regardless of location.
- Optimized Analytics Capabilities: Sophisticated tools are readily available, helping organizations tap into insights more efficiently.
Growth of Edge Computing
Another significant trend is the growth of edge computing. As the Internet of Things (IoT) expands, more devices connect and generate vast amounts of data. Processing this data closer to the source reduces latency and increases efficiency. Edge computing allows for instant analysis of data from devices rather than sending it all back to centralized data centers.
This approach holds notable advantages:
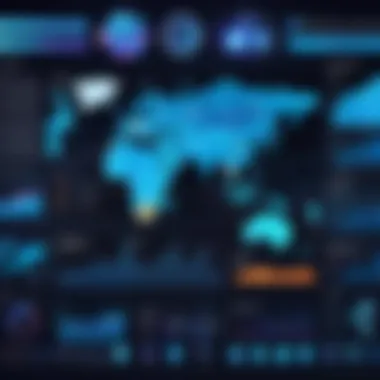
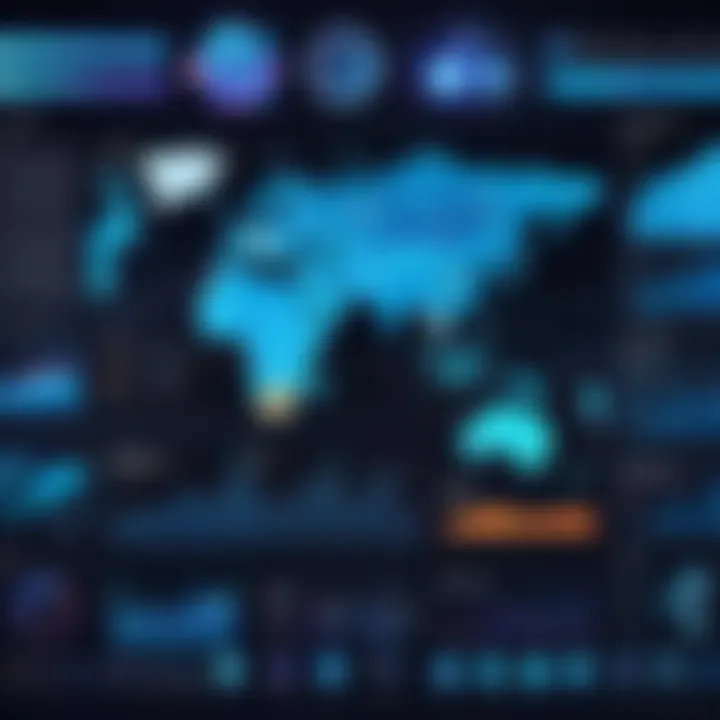
- Lowered Latency: Applications requiring real-time responses can function better.
- Bandwidth Efficiency: Reduces the need to transmit large data volumes up and down links, thus making operations smoother.
- Improved Data Privacy: Stakeholders can better control their sensitive data closer to its point of origin.
Such growth will continue as industries seek more efficient data handling solutions.
Shift Towards Explainable AI
Explainable AI strives to uncover reasons behind insights generated by algorithms. As reliance on AI increases, understanding and interpreting AI-based decisions becomes crucial. Stakeholders must ensure that AI applications can provide insights that are transparent and justifiable.
This need arises from several pressing considerations:
- Trust: Users must trust AI applications, especially in critical matters; they require clear explanations for decisions.
- Accountability: Organizations must understand the reasoning behind AI interactions to ensure compliance with regulations.
- Improved Adoption: Showcasing how AI arrives at suggestions or decisions can foster wider acceptance among users.
In summary, the trends in cloud computing adoption, edge computing growth, and the shift to explainable AI are reshaping how organizations manage and utilize Big Data and AI. Their implications will be profound, and navigating these trends becomes vital for forward-thinking companies。
"Data is what you need to do analytics. Information is what you need to do business." - Judith Hurwitz
Sector-Specific Applications of Big Data and AI
Sector-specific applications of Big Data and AI play a crucial role in shaping their impact across different industries. Each sector, from healthcare to finance and retail, utilizes these technologies to drive efficiency, improve service delivery, and enhance decision-making. Understanding the specific applications ensures that organizations can harness the right tools and methodologies tailored to their unique needs. The increasing reliance on data analytics in these sectors demonstrates a growing understanding of how data-driven strategies can provide a competitive edge.
Healthcare Innovations
Predictive Analytics in Patient Care
Predictive analytics in patient care represents an important application of Big Data and AI within the healthcare industry. This area focuses on using existing data to predict future outcomes, allowing healthcare providers to act proactively. One key characteristic of predictive analytics is its ability to analyze vast datasets, leading to informed decisions based on statistical models.
The benefit of using predictive analytics is that it improves patient outcomes and reduces costs by anticipating potential health issues before they become severe. However, a unique feature of predictive analytics is the need for high-quality data. Inaccurate data and biases can lead to poor predictions, which can be a significant disadvantage in critical healthcare scenarios.
AI in Medical Imaging
AI in medical imaging is another transformative application in healthcare. This technology uses algorithms to assist in evaluating images from X-rays, MRIs, and CT scans. One of the main advantages of AI in this field is its speed and accuracy in identifying anomalies. The use of AI has proven to be beneficial for radiologists, assisting them in making more accurate diagnoses more quickly.
A unique characteristic of AI in medical imaging is its ability to continuously learn from new data, improving its diagnostic capabilities over time. Despite these advantages, the challenge remains that reliance on AI without human oversight could lead to oversights in complex cases, underlining the importance of a collaborative approach between technology and healthcare professionals.
Financial Services Transformation
Fraud Detection Strategies
Fraud detection represents a significant challenge for financial service providers. Effective strategies in this area leverage AI and Big Data to identify and mitigate fraudulent activities. A key characteristic of these strategies is their ability to analyze patterns in transaction data in real-time, which is essential in minimizing losses from fraud.
Fraud detection strategies can be highly effective as they operate at scale. They sweep through millions of transactions and can identify suspicious patterns that human analysts may miss. However, the unique feature lies in their dependence on clean data. Poor data quality can lead to false positives, turning customer transactions into extensive investigations that harm user experience.
Risk Management Solutions
In the realm of risk management, the integration of Big Data and AI provides analytical clarity. These solutions focus on assessing potential threats to financial stability, driven by data analysis and predictive modeling. A fundamental characteristic of these tools is their holistic approach, allowing firms to consider multiple variables before decision-making.
One major advantage of using these systems is their ability to predict outcomes based on previous market behavior. This helps in minimizing risks associated with new investments or lending practices. Nevertheless, the challenge lies in the inherent unpredictability of markets which can generate misleading trends, sometimes leading to misguided strategies.
Retail Industry Evolution
Personalization Techniques
Personalization techniques implemented by retailers utilizing Big Data and AI aim to enhance customer engagement. These techniques involve analyzing consumer behavior and preferences to tailor recommendations and marketing strategies. A core characteristic is the focus on deliverability of individualized shopping experiences.
The benefit of personalization is that it increases user satisfaction and loyalty, as customers often respond positively to relevant offers. A distinct feature of these techniques is the real-time adjustment ability based on current shopping behaviors. On the downside, privacy concerns may arise if analysis involves intrusive methods of data collection, which can negatively impact brand loyalty.
Supply Chain Optimization
Supply chain optimization is another crucial application in the retail sector. As retailers handle vast quantities of goods, proper management guided by data insights is critical. A defining characteristic of supply chain optimization solutions is their real-time analytics capabilities, which streamline operations.
An advantage of these solutions includes enhanced visibility throughout the supply chain, allowing retailers to respond quickly to changes in demand or disruption. However, technology-driven analysis requires significant upfront investments and can create an over-reliance on technology, which raises operational risks.
The implementation of Big Data and AI is transformative in sector-specific applications, as each industry witnesses significant growth in efficiency, customer satisfaction, and predictive capabilities.
Challenges Facing Big Data and AI Integration
The incorporation of Big Data and AI technologies into various sectors is met with multifaceted challenges. Understanding these obstacles is critical for professionals and decision-makers in tailoring their strategies effectively. Addressing the challenges associated with Big Data and AI integration not only enhances the potential benefits of these technologies but also ensures a smoother transition with minimal disruption to existing operations.
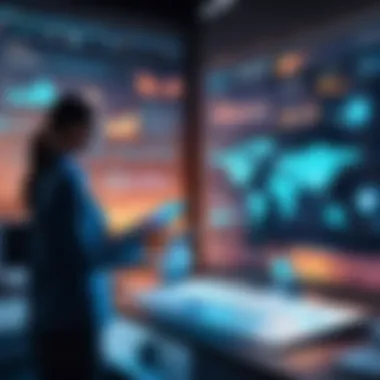
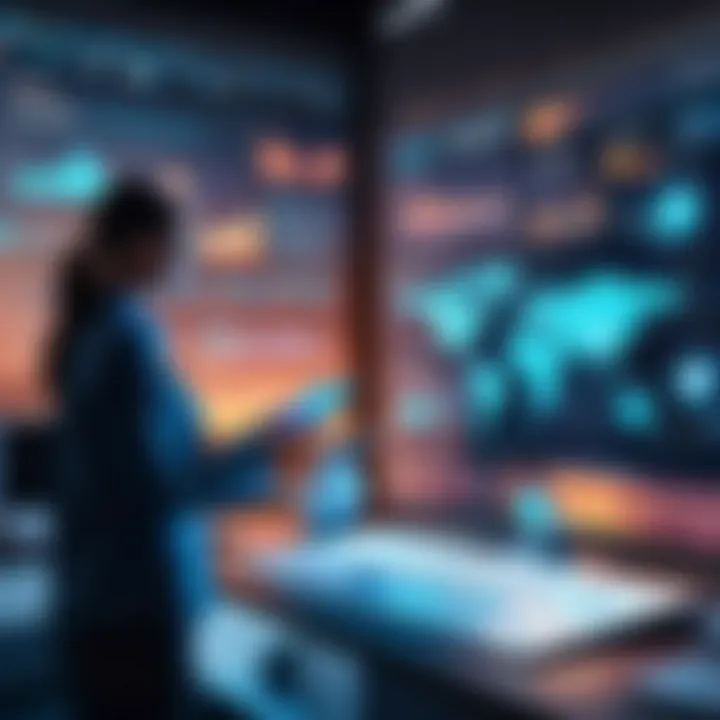
Data Privacy Concerns
Data privacy has become a paramount concern in the digital age. Organizations are tasked with navigating complex regulations surrounding personal data handling. The improper use of data may lead to violations of privacy laws such as the General Data Protection Regulation (GDPR) or the California Consumer Privacy Act (CCPA). Security breaches and data leaks can cause irreparable harm to consumer trust and brand integrity.
To foster trust, businesses must prioritize the safeguarding of sensitive information. Implementing robust data governance frameworks can help inform employees about best practices and policies. Moreover, educating consumers on how their data is utilized can mitigate feelings of uncertainty regarding privacy.
Maintaining a balance betweem innovation in AI and adherence to privacy regulations should be a sustained effort. The trend toward increased transparency will not only be beneficial for businesses but vital in ensuring long-term success in customer relations.
Skill Shortages in the Workforce
Despite the surge in Big Data and AI adoption, a persistent challenge lies in the lack of skilled professionals. Companies require talents that embody a nuanced understanding of data science, machine learning, and ethical AI. The scarcity of these competencies hampers organizations' ability to fully exploit the benefits of Big Data technologies and AI analytics.
Several approaches can bridge this skills gap. Initiatives can include upskilling current employees through targeted training programs or forming partnerships with educational institutions. Emphasizing the importance of lifelong learning and in-field experiences can help foster a more knowledgeable workforce. Employers who invest in their teams signal an aspiration not just to fill positions, but to develop an expert workforce.
Having skilled data scientists and AI specialists contributes significantly to achieving data-driven decision making. Embracing a collective effort to close the skill gap will advance the opportunity landscape for firms in every sector.
Bias and Ethical Considerations
A critical issue associated with the implementation of AI systems is the inherent risk of biases present in algorithms. Biased AI can perpetuate existing inequalities, leading to misjudgments in sectors such as hiring, lending, or justice systems. It is vital for organizations to conduct thorough fairness evaluations in their data processing pipelines.
Organizations must embed ethical considerations into their AI strategy. Sustainable AI practices should involve building diverse teams during the development focus, continually testing algorithms for biases, and being candid about limitations
Encouraging ethical frameworks promotes a responsible technological landscape, safeguarding against algorithmic bias.
In summary, every element of integrating Big Data with AI technologies presents significant hurdles, but these challenges also offer avenues for growth. By recognizing and strategically addressing concerns surrounding data privacy, workforce skills, and ethical imperfections, businesses can optimize their approaches and uncover heightened reservoir for innovation.
Future Outlook for Big Data and AI
The realm of Big Data and Artificial Intelligence is in constant motion, responding to ever-changing technological advancements and consumer demands. The future outlook for Big Data and AI not only serves as a significant predictor for these technologies but also plays a major role in shaping them across multiple sectors.
Understanding the trajectories of Big Data and AI is crucial for professionals involved in decision-making processes. Their ability to leverage these technologies effectively can foster innovation, drive competitive advantage, and enhance overall operational efficiency. Additionally, anticipating future shifts enables organizations to prepare an adaptable workforce, invest wisely in emerging technology trends, and strategize toward sustainable growth.
Emerging Technologies on the Horizon
Quantum Computing
Quantum computing represents an pivotal shift in computing capabilities. Traditional computers process information using bits that can either be 0 or 1, while quantum computers use qubits. Qubits can exist in multiple states at the same time due to quantum superposition. This characteristic grants quantum computing immense potential in handling complex computational tasks that are currently impractical, such as large-scale optimization problems, drug discovery, and precise forecasting based on significant data sets.
One key aspect of quantum computing is its ability to outperform classical computers exponentially in certain contexts. It is seen as a crucial answer to the rising data volumes associated with Big Data. Despite its promise, quantum computing has its disadvantages, including high costs and technical complexities that may delay its widespread adoption. However, progress is undeniably being made, supporting the belief that quantum computing can eventually revolutionise sectors like pharmaceuticals, finance, and logistics.
Blockchain Integration
Blockchain integration is another area gaining attention as organizations look for effective ways to manage data securely. Its key characteristic is decentralization, which allows data to be stored across a distribution of computers rather than in a central repository. This enhances data integrity and security, making blockchain a reliable choice for sectors such as finance, healthcare, and supply chain management.
The unique feature of blockchain technology is its immutability, meaning that once recorded, the information cannot be altered or deleted. It adds a layer of transparency that businesses find valuable in ensuring trust among users and partners. On the downside, the complexity of implementation and an existing skills gap can present barriers. Nonetheless, integrating blockchain with Big Data is likely to become increasingly prevalent as organizations aim to build resilient systems that prioritize data integrity.
Preparing for the Future Workforce
As the landscape of Big Data and AI evolves, so must the workforce tasked with managing these tools. Preparing employees with relevant skills is more critical than ever, especially in light of growing demand for technical expertise in data management, machine learning, and AI development.
Organizations must invest in training and development programs focusing on these skills. Understandably, this includes offering workshops, boot camps, and online courses that provide resume-worthy experience. Furthermore, cultivating a culture that emphasizes continuous learning will aid in maintaining competitive vigor. With the right preparations, the workforce not only supports organizational scalability but also moves toward an era where human intelligence and AI coexist, facilitating groundbreaking innovations.
Finale and Recommendations
The conclusion of analyzing the Big Data and AI landscape in 2021 presents critical insights on its significance to today's market dynamics. Organizations must strategically implement these technologies to remain competitive. By understanding the pathways through which Big Data and AI integrate, businesses can fuel innovations, enhance operational efficiencies, and make informed decisions backed by robust analytics.
Highlighting the advantages, we find that these technologies drive better customer experiences and enable precise targeting in marketing strategies. Additionally, they allow for proactive risk management and minimize operational costs through data-driven workflows. As these tools continue to morph, companies, especially those in tech-driven sectors, must keep pace with the agility to adapt swiftly and effectively.
Strategic Implementation of Big Data and AI
Implementing Big Data and AI requires more than just deploying software solutions. Successful integration begins with a clear understanding of business goals. Knowing your objectives can dictate what data to collect and how to analyze it. Key considerations include:
- Assess your current data environment to identify gaps and opportunities.
- Invest in scalable data infrastructure that caters to growing volumes of data.
- Train staff on the tools and methodologies that leverage AI advancements, fostering an innovation-oriented culture.
Aligning strategies with business objectives ensures that Big Data initiatives contribute meaningful insights, allowing teams to cultivate a culture of data positivity.
Measuring Success and ROI
Establishing specific metrics for success is essential when toneeting Big Data and AI إستسserutrants. Quantifying the return on investment (ROI) can be achieved using a blend of qualitative and quantitative measures. Some effective metrics to consider:
- Reduction in operational costs as a result of process optimizations.
- Increased revenue through better customer segmentation and targeting initiatives.
- Improvement in customer satisfaction scores from personalized experiences.
- Shortened cycles of research and development thanks to predictive analytics tools.
By systematically gauging these elements, organization will benchmark their results against initial expectations, ensuring that Big Data and AI remain integral to their value proposition moving forward.