Exploring the Essentials of Big Data Technology
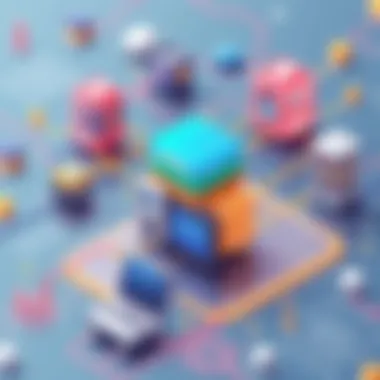
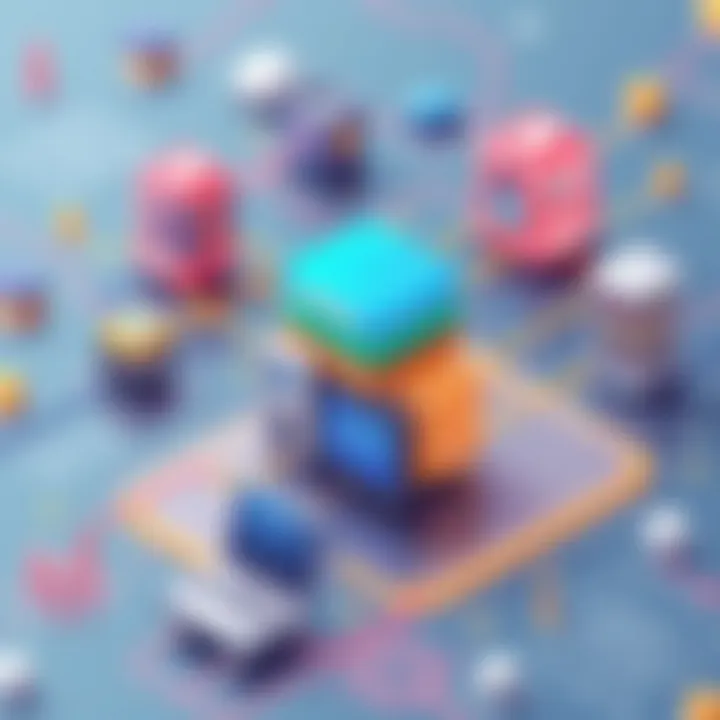
Intro
In todayâs world, the concept of big data is more than just a buzzword thrown around in tech circles. It has become a cornerstone of how businesses operate and strategize. Organizations are no longer limited to the occasional spreadsheet or manual calculations. Instead, they have access to streams of data that, if analyzed properly, can lead to incredible insights and growth opportunities.
Big data technology gives organizations the ability to sift through heaps of informationâthink of it like finding a needle in a haystack, but with the help of sophisticated tools and methods. This article dives deep into understanding how big data shapes modern business practices, how itâs changing the game in various industries, and what future trends professionals should keep an eye on.
Technological Research Overview
The rapid advancement of technology has paved the way for big data to emerge as a critical component of business strategy. Letâs unpack the different aspects that contribute to this complex but fascinating topic.
Recent Technological Innovations
Consider just how much data flows through the internet every second. With innovations such as cloud computing, advanced analytics, and data warehousing tools, organizations now have the means to store and analyze massive datasets efficiently.
âData is the new oil, but itâs not enough to just have it; you need to refine it.â
Impact on Business Operations
Businesses are increasingly turning to big data solutions to streamline operations and make informed decisions. Some benefits include:
- Improved customer insights through predictive analytics
- Enhanced operational efficiency via automated data processing
- Better risk management through real-time data monitoring
This data-driven approach gives companies a competitive edge that isn't easily matched by traditional methods.
Future Technological Trends
The landscape of big data is ever-changing. Emerging trends to watch include:
- Increased integration of Internet of Things (IoT) devices
- More emphasis on data privacy and security as a priority
- Growth of artificial intelligence and machine learning capabilities in data analytics
Keeping abreast of these trends is important for professionals aiming to harness the potential of big data fullly.
Data Analytics in Business
In essence, if big data is the foundation, data analytics is the building. Understanding the importance of data analytics is the first step for businesses looking to harness big data effectively.
Importance of Data Analytics
Data analytics turns raw data into actionable insights. For businesses, this means better decision-making, strategic planning, and increased revenues.
Tools for Data Analysis
Various tools have emerged that facilitate effective data analysis, such as:
- Microsoft Power BI
- Tableau
- Google Analytics
Each of these tools offers different capabilities, allowing users to visualize data in intuitive ways that promote deeper understanding.
Case Studies on Data-Driven Decisions
Many companies have something to learn from organizations that successfully navigated the waters of data analytics. For instance, Netflix utilizes data to tailor recommendations for users, significantly boosting viewer engagement.
Cybersecurity Insights
As much as data analytics can empower companies, the flip side is the pressing need for security. In a world where data breaches make headlines regularly, understanding the cybersecurity landscape is essential.
Threat Landscape Analysis
Hackers are getting more sophisticated, and organizations must be aware of emerging threats. Understanding potential vulnerabilities can help mitigate risks effectively.
Best Practices for Cybersecurity
To safeguard data, businesses can implement best practices like:
- Regular security audits
- Data encryption
- Employee training on phishing and other scams
Regulatory Compliance in Cybersecurity
Adhering to regulations such as GDPR or CCPA is critical. Non-compliance can lead to hefty fines and reputation damage.
Artificial Intelligence Applications
Artificial intelligence (AI) is another game-changer in the realm of big data. It offers applications that can revolutionize common business tasks.
AI in Business Automation
From chatbots to predictive maintenance, AI helps automate processes, saving time and resources.
AI Algorithms and Applications
Understanding different AI algorithms, like decision trees or neural networks, can equip professionals with the knowledge to choose the right solutions for specific needs.
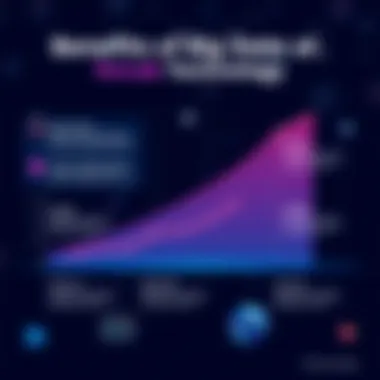
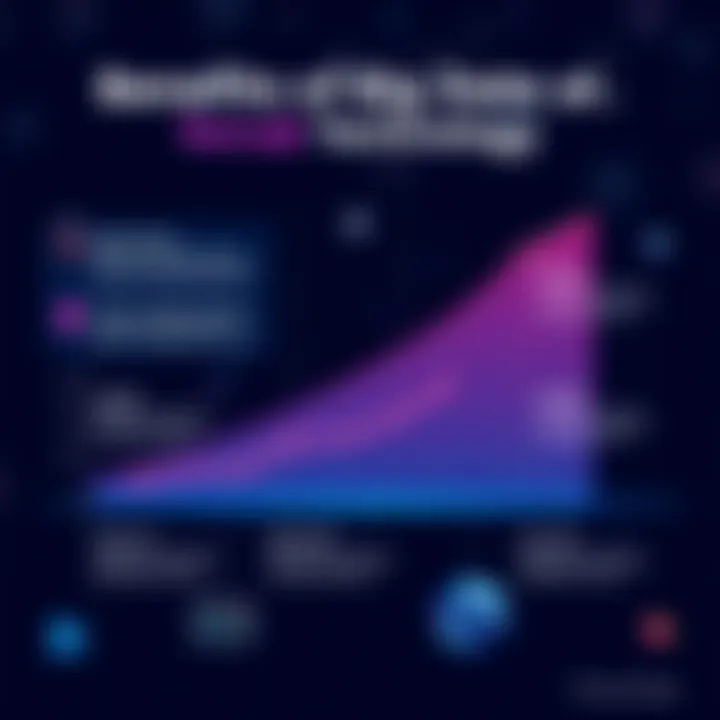
Ethical Considerations in AI
While AI offers substantial benefits, ethical issues also arise. Companies must address concerns about bias in data and the implications of AI decision-making.
Industry-Specific Research
Big data technology does not have a one-size-fits-all approach. Different industries adopt it in unique ways to meet their specific challenges.
Tech Research in Finance Sector
In finance, algorithms can predict market trends and assess credit risks, thus providing better services to customers.
Healthcare Technological Advancements
Healthcare has started to adopt big data solutions for patient monitoring, predictive analytics in treatment outcomes, and operational enhancements.
Retail Industry Tech Solutions
Retailers use big data to understand customer preferences, optimize inventory, and personalize marketing strategies, thereby improving overall client satisfaction.
Preface to Big Data Technology
Defining Big Data
When we talk about big data, we might as well start with a clear definition. Broadly speaking, big data refers to extremely large datasets that can't be easily managed or analyzed using traditional data processing software. This notion encapsulates not just volume, but also the variety of data typesâfrom structured data like databases to unstructured content such as social media posts and sensor data.
The challenge lies in integrating all these different formats and sources into a coherent system. As data scientists often say, itâs not just the amount of data that makes it âbig,â but its complexity, which leads to the necessity for more advanced analytical tools and methodologies.
Historical Context of Big Data
The concept of big data isnât a newfound revelation. In fact, its roots trace back several decades. Early attempts at data analysis can be seen as early as the 1960s, where computers began to gain traction in handling larger quantities of information than ever before. Fast forward to the late 2000s, and we see the term "big data" becoming mainstream as companies like Amazon and Google pioneered new ways to collect and interpret vast streams of information.
Over the years, the technology underpinning big data has evolved from rudimentary databases to sophisticated frameworks that can process terabytes and petabytes of data almost instantaneously. Innovations such as Hadoop and Apache Spark emerged, enabling organizations to analyze data on a scale previously thought impossible. This historical evolution underscores the increasing importance of big data in shaping business decisions and policies across industries.
"Big data isn't a trend; it's a revolution in how we devise strategies and make sense out of chaos."
Thus, the journey continues as we embrace artificial intelligence, machine learning, and other advancements that further unlock the potential hidden within big data. Each step in this historical context not only reveals how far we've come but also hints at where we're headed.
Key Characteristics of Big Data
Big Data isn't just about having a lot of information at one's disposal; it also encompasses specific characteristics that define its nature and influence its processing and utilization. Understanding these key characteristics is essential for professionals striving to effectively harness the power of Big Data in their organizations. These attributesâvolume, variety, velocity, veracity, and valueâcreate a robust framework for analyzing and interpreting complex information efficiently.
Volume of Data
The term volume quite literally refers to the sheer size of the data generated today. Organizations collect data from diverse sources such as social media platforms, IoT devices, transaction records, and countless online interactions.
For instance, a typical retail store might pull in terabytes of customer data dailyâthink purchase history, inventory levels, and customer feedback. Such immense volumes can overwhelm traditional data processing systems, leading to bottlenecks and slowdowns in analysis. Therefore, having scalable storage solutions that can accommodate wave after wave of data is not just advisable but crucial. Companies that fail to scale can cheapen their data assets and miss out on valuable insights that could inform strategy and drive better decisions.
Variety of Data
In the age of Big Data, variety refers to the multitude of data forms and types generated. Gone are the days of simply dealing with structured data in relational databases. Nowadays, data flows in all shapes and sizes, including text, images, videos, and sensor data among others.
To illustrate, consider a smartphone app that collects user data. It not only records usage statistics (structured) but can also include text messages (unstructured) or even voice commands (semi-structured). Itâs critical to develop tools capable of analyzing these different formats, as they can yield unique insights when stitched together. Embracing this variety allows businesses to build a comprehensive picture of their customers and operations, enhancing overall strategy.
Velocity of Data
Velocity captures the speed at which data is generated and how quick responses need to be. In a fast-paced environment where real-time data processing is often necessary, delays can equate to lost opportunities.
Consider financial markets; they experience rapid transactions daily. If an organization can't analyze data on-the-fly, it risks making decisions based on outdated or irrelevant information. Businesses need to adopt technologies like stream processing to keep pace. By utilizing tools such as Apache Kafka, they can effectively manage and process data streams in real-time, gaining an edge over competitors.
Veracity of Data
Veracity speaks to the accuracy and reliability of data. Not all data collected is valuable; some can be incomplete or downright misleading. Filtering out the noise and focusing on high-quality data is essential in navigating the complex waters of Big Data.
For example, if a company relies on customer feedback but doesnât account for the context of the comments, it might misinterpret user sentiment. Implementing robust validation methods and establishing processes for data cleaning can significantly improve veracity. This careful attention to quality ensures that insights derived from the data closely reflect reality, leading to better decision-making.
Value of Data
Finally, we reach the most vital aspectâvalue. Itâs not enough to gather data; organizations must extract meaningful insights that drive business value. The key question is how to turn raw data into something useful.
For instance, analytics can uncover customer trends and predict future buying behaviors. Companies that analyze trends effectively can tailor their marketing campaigns and product offerings accordingly, ultimately boosting sales and customer satisfaction. Value creation should be a driving goal behind any Big Data initiative; without it, the endeavor risks becoming just a costly exercise.
âThe real value of Big Data lies not in how much data you collect but in how well you leverage it to improve your business practices.â
Technologies Enabling Big Data
In a world where information is currency, the tea leaves of big data technology are ground by a multitude of tools and systems. This section delves into the backbone of big dataâtechnologies that articulate how data is not only stored but also processed and analyzed. Without these essential technologies, the dream of converting vast amounts of data into actionable insights would remain just thatâa distant dream.
Data Storage Solutions
Relational Databases
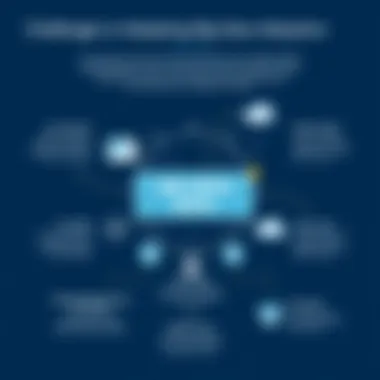
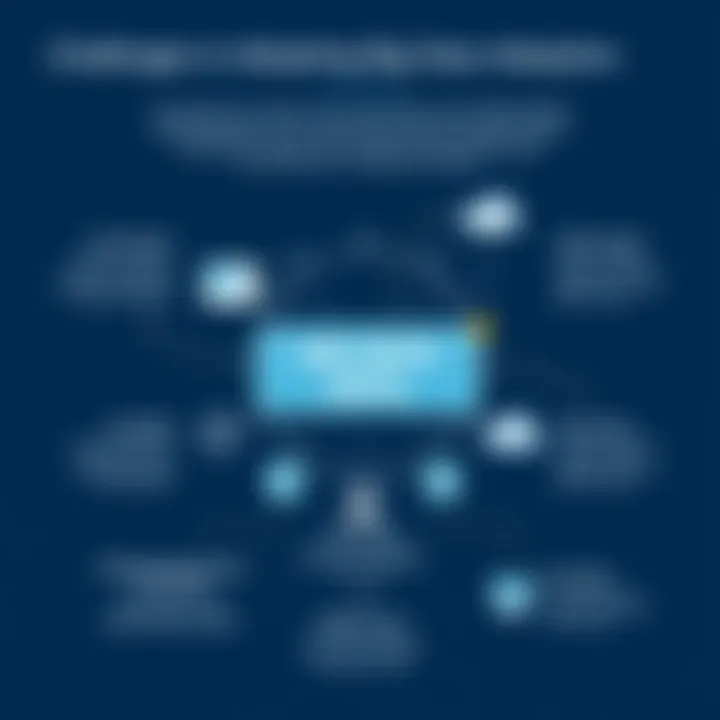
Relational databases have long been a cornerstone of data management systems. They remember relationships between the different datasets and help in organizing that information like a well-structured library. Their structured nature allows for easy retrieval and manipulation of data.
One key characteristic of relational databases is their use of SQL, which stands for Structured Query Language. SQL offers a standardized way of interacting with databases, making it a trusted choice among IT professionals. A unique feature of this type of database is its strong consistency properties, which ensures that once data is written, it will be accurately retrieved later on.
However, itâs not all sunshine and rainbows; they often struggle with scalability when faced with massive datasets. For organizations looking to analyze data in real-time, relational databases may not be the first option.
Non-relational Databases
On the flip side, non-relational databasesâoften termed as NoSQLâhave leapt into the spotlight as a solution for unstructured data. They offer a flexible schema design and can handle different types of data formats, from documents to key-value pairs. This characteristic makes them a favored choice for startups or organizations looking to integrate large volumes of varied data.
A particular feature of non-relational databases is their horizontal scalability. They can efficiently store terabytes or even petabytes of data without breaking a sweat.
Yet, this flexibility comes with a catch: Many non-relational databases forfeit the strict transaction guarantees that relational databases promise. Therefore, organizations must weigh their priorities when choosing between the two.
Cloud Storage
Cloud storage represents the modern-day solution for data management challenges. Itâs about storing data on external servers accessed via the internet rather than on local machines. A standout characteristic of cloud storage is its flexibility and elasticity; organizations can scale storage up or down based on their needs.
Unique to cloud storage is its ability to support distributed teams seamlessly. Data can be shared and accessed anywhere, which fosters collaboration and efficiency.
Nonetheless, it does bring inherent risks, mainly around data privacy and security. Whoâs got access to that data and how secure is it? Concerns of compliance with regulations also play a significant role, resulting in organizations needing to carefully consider a cloud storage solution.
Data Processing Frameworks
Hadoop
Hadoop emerged as a trailblazer in big data processing. It enables the processing of large datasets across clusters of computers using simple programming models, breaking down complex data into manageable pieces. This makes it particularly advantageous.
A notable feature of Hadoop is the Hadoop Distributed File System (HDFS), designed to store data reliably and to scale easily. While Hadoop excels in batch processing, its drawback is latency. It is not the go-to solution for applications demanding immediate results.
Apache Spark
Apache Spark has taken data processing to the next level by offering in-memory processing capabilities. This feature markedly accelerates data analysis tasks, making Spark a top choice for businesses needing rapid insights. The ability to execute complex queries quickly is a significant feather in its cap.
However, the memory-intensive nature of Apache Spark indicates that it requires careful resource management and may be less suitable for organizations with limited hardware capacity.
Stream Processing Tools
Stream processing tools are like the quicksilver of the big data world, handling data as it flows in real-time. Tools such as Apache Flink or Apache Kafka transport messages and process them as they come. This immediacy is ideal for organizations needing to make quick decisions based on incoming data.
One key advantage here is their ability to handle high throughput, which means they can process large volumes of data streams without missing a beat. However, one challenge with stream processing can be the complexity of system integration and the need for robust monitoring solutions.
Analytics Tools
Data Mining Techniques
Data mining is an essential approach that enhances the retrieval of meaningful patterns from data. Techniques such as clustering, decision trees, and neural networks push organizations to ascertain hidden predictors and trends within datasets. This makes it a popular tool in marketing and risk analysis.
A key characteristic is its capability to uncover relationships in the data that were once hidden from view. However, its success often hinges on having well-prepared data.
Machine Learning Applications
Machine Learning ushers in a new era of automation and predictive analytics. By allowing systems to learn from data, organizations can facilitate better decision-making processes. It excels in adapting to changes quickly, meaning it can optimize its performance over time.
But this capability does not come without challenges; machine learning models can be complex to develop and may require substantial computational power.
Data Visualization Tools
Data visualization tools like Tableau or Power BI are instrumental in presenting complex results in a digestible format. They allow users to see trends and patterns, which is paramount in decision-making. A unique feature of these tools is their ability to provide interactive dashboards, allowing users to filter and drill down into the details.
However, while data visualization is a powerful ally, it is only as effective as the underlying data quality. Poor data can lead to misleading visualizations, which ultimately leads to incorrect insights.
In the fast-paced world of big data, the technologies enabling it go beyond mere functionality. They reshape the landscape of how decisions are made and how organizations strategize based on those decisions. Having a sound understanding of these technologies is essential for any professional navigating this field.
In summary, the technologies encompassing big data create a framework that helps organizations manage their data more effectively. By choosing the right toolsâbe it storage solutions, processing frameworks, or analytics toolsâbusinesses can harness the full potential of big data.
Applications of Big Data in Business
In todayâs fast-paced business environment, the importance of leveraging big data technology cannot be overstated. Organizations that do not adapt risk falling behind their competitors who harness this information to make informed decisions. Through its diverse applications, big data serves as a cornerstone for growth strategies, enabling insights that drive innovation, enhance customer experiences, and streamline operations.
Customer Insights and Personalization
Big data is instrumental in improving customer understanding. By analyzing vast amounts of data collected from various sources such as online interactions, purchase histories, and social media activities, businesses can gain deeper insights into customer preferences and behaviors. This information isn't just numbers on a spreadsheet; it's a goldmine waiting to be tapped.
For instance, consider an online retail store that mines its data to uncover patterns in buying behavior. This could lead to tailored recommendations that resonate with customers on an individual level. A shopper looking for sports shoes may receive suggestions for running gear or training regimes that match their interests. This level of personalization extends to marketing efforts, where targeted advertisements can be customized based on individual browsing history, thus enhancing the likelihood of conversion.
Moreover, companies like Netflix and Amazon have mastered the art of using customer data to create a personalized user experience. Their algorithms analyze viewing habits or buying patterns, allowing them to suggest content or products users may not have discovered otherwise. Itâs no wonder that personalization has now become a critical component of customer retention strategies.
Operational Efficiency
Operational efficiency is another area where big data proves invaluable. Organizations are constantly on the lookout for ways to improve processes, reduce downtime, and maximize resource utilization. Here, data analytics can illuminate inefficiencies that may not be readily apparent.
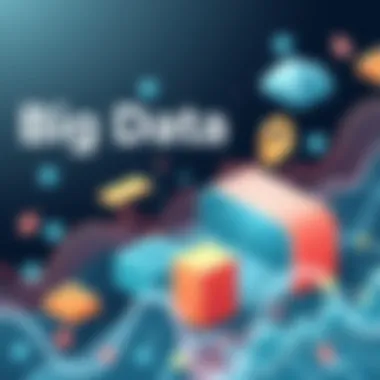
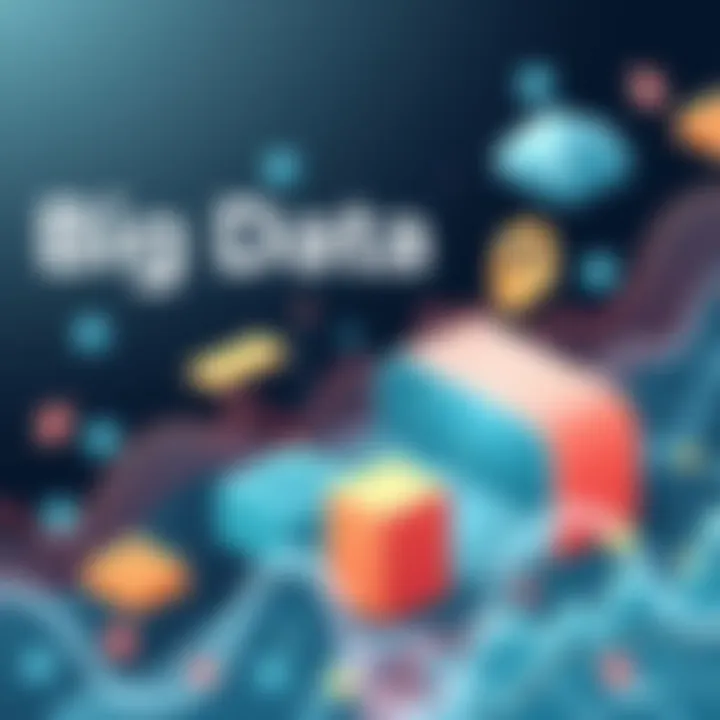
For example, in a manufacturing context, big data can be leveraged to monitor machinery performance in real time. This enables predictive maintenance strategies, where potential equipment failures can be identified before they lead to costly downtimes. Analytics can reveal trends in maintenance needs, allowing companies to schedule inspections proactively.
Additionally, by analyzing supply chain data, businesses can optimize inventory levels, preventing overstock situations that tie up capital unnecessarily. With the help of big data, firms can also enhance workforce management by analyzing employee productivity patterns, leading to more effective scheduling and deployment of personnel where they are needed most.
Risk Management and Fraud Detection
In an age where data breaches and fraud are rampant, utilizing big data technology for risk management is becoming not just common, but essential. Organizations can utilize data analytics to spot unusual patterns that may indicate fraudulent activities.
For instance, financial institutions apply big data techniques to monitor transactions in real time. By employing algorithms that analyze spending patterns, they can flag any transactions that deviate from a customer's usual behavior. This proactive measure helps in identifying and thwarting fraudulent activities before significant damage is done.
Moreover, big data can also facilitate risk assessment by allowing organizations to analyze historical data. Companies can evaluate past incidents to predict potential risks and develop strategies to mitigate them. This not only safeguards assets but also enhances their ability to respond effectively in crisis situations.
Big data is not simply about collecting vast amounts of data, but about discerning meaningful patterns that can guide superior business decision-making.
By embracing these capabilities, organizations can position themselves as leaders in their respective industries.
Challenges of Implementing Big Data Technology
As organizations increasingly adopt big data technology, a host of challenges arise that can impede the successful implementation of these systems. It's not just about the sheer volume of information; itâs about navigating a complex landscape where privacy, scalability, and compatibility with existing systems are constant hurdles. Understanding these challenges is crucial for any professional looking to manage data effectively. Let's delve deeper into the specific aspects that complicate big data implementation.
Data Privacy Concerns
One of the most pressing issues in big data technology revolves around data privacy. The rise of massive data collection leaves individuals vulnerable, raising ethical questions about how information is stored and utilized. Companies often gather personal data without clearly informing users, which can lead to breaches of trust. The regulatory landscape, especially with laws like GDPR in Europe, imposes strict guidelines on data handling. Organizations must prioritize transparency and security in their data collection practices.
"Without trust, there can be no true engagement. Data privacy isnât just a requirement; itâs a necessity for fostering relationships with customers."
The risks associated with mishandling sensitive data can lead to severe reputational damage and legal consequences, making it imperative for businesses to invest in secure systems. This includes encryption, access controls, and regular audits of data usage.
Scalability Issues
Scaling big data operations is another concern that organizations frequently face. As the quantity of data grows, the infrastructure must be capable of handling increased loads without sacrificing performance. Oftentimes, organizations may start with a modest setup that meets their initial needs, but as data influx surges, they realize the system can barely keep its head above water.
The scalability challenge isn't solely about physical storage; it extends to processing power and speed. Cloud services like Amazon Web Services or Microsoft Azure are frequently leveraged for their scalability features. However, making the switch from on-premises to cloud services can be complex and also raises questions regarding control over data. Organizations need to plan ahead, ensuring the architecture can evolve alongside business requirements.
Integration with Legacy Systems
As companies rush to embrace big data technology, the question of how to integrate new systems with existing legacy architecture becomes essential. Many firms are stuck with older systems that weren't designed to handle large datasets or modern data processing techniques. This reluctance to update can create significant friction.
Combining the new and the old can result in compatibility issues, where legacy systems fail to communicate efficiently with advanced big data frameworks like Apache Hadoop or Spark. Organizations often find themselves at a crossroadsâinvestment in new technology is necessary, but it also involves a steep learning curve and the risk of interruption in operations.
To ease integration, companies must conduct thorough assessments of their current systems and prioritize gradual transitions or employ middleware that can bridge the gap between the two worlds. The goal should be to create a seamless environment where both modern data solutions and legacy systems can coexist, thus reducing operational downtime.
In summary, understanding the challenges of implementing big data technology is crucial for any organization aiming to leverage data effectively. From navigating data privacy regulations to ensuring scalability and fostering integration with existing systems, addressing these issues head-on will set the stage for success in a data-driven world.
The Future of Big Data Technology
The world is on the cusp of a seismic shift with big data technology leading the way. It is crucial to understand that as data continues to grow at an unprecedented rate, the methods and technologies we use to analyze and interpret this data must evolve accordingly. Big data is not a passing trend; it's a permanent fixture in the landscape of business, technology, and daily life. Not only does it offer insights that can shape decision-making, but it also lays the groundwork for innovation and efficiency across various sectors. In contemplating the future of big data technology, several key elements come into focus, notably emerging trends, the integral role of artificial intelligence, and pressing ethical considerations surrounding data governance.
Emerging Trends
As we peer into the future, several trends are becoming increasingly evident in the realm of big data technology:
- Edge Computing: The migration of data processing closer to where data is generated increases speed and efficiency. By reducing latency, organizations can respond to real-time demands without the bottlenecks associated with centralized data centers.
- Predicitive Analytics: Organizations are harnessing sophisticated algorithms to predict customer behavior, operational efficiencies, and market trends. This capability places companies a step ahead of the competition, enabling proactive rather than reactive decision-making.
- Data-as-a-Service (DaaS): The shift toward DaaS allows organizations to easily access and share data regardless of where it is stored. This flexibility fosters collaboration while ensuring that timely insights are available at the fingertips of decision-makers.
âData is the new oil,â and its refinement is undergoing constant evolution.
- Internet of Things (IoT) Integration: With the explosion of connected devices, the amount of data generated is astronomical. The integration of IoT with big data allows for deeper insights into consumer habits and operational efficiencies.
In summary, these emerging trends are not just technological enhancements; they represent a transformational leap in harnessing data in ways that were previously unimaginable.
The Role of Artificial Intelligence
Artificial intelligence (AI) is more than just a buzzword; it's becoming the backbone of big data technology. AI offers robust solutions for managing large datasets by enabling machines to learn from the data they process. This ability can enhance several areas:
- Automated Insights: AI systems can analyze data patterns and generate reports, saving professionals significant time and facilitating quicker strategic decisions.
- Natural Language Processing (NLP): With NLP, organizations can gain insights from unstructured data, such as customer feedback or social media sentiments, thus capturing a fuller picture of consumer experiences and needs.
- Anomaly Detection: Machine learning algorithms can identify unusual patterns in data, which is crucial in areas like fraud detection and cybersecurity, helping organizations mitigate risks before they escalate.
In essence, the synergy between big data and AI is fostering a future where data-driven decisions will become even more precise and impactful.
Ethical Considerations and Data Governance
As we embrace the advancements in big data technology, itâs vital to address the accompanying ethical implications. Data governance emerges as a fundamental pillar in this regard, and organizations must consider several key aspects:
- Privacy Concerns: With the advent of big data comes the responsibility of protecting sensitive information. Organizations must ensure compliance with regulations such as GDPR while fostering trust with consumers.
- Transparency and Fairness: Algorithms driving decisions should be transparent to avoid biases that can adversely impact individuals and social equity. Organizations ought to strive for fair data practices that reflect accountability.
- Data Ownership and Rights: As data collection grows, so does the question of ownership. Who owns the data? Consumers, businesses, or third-party vendors? Establishing clear rights regarding data utilization and ownership will be paramount.
Finale
In summarizing the discussion on big data technology, it becomes clear that this is more than just a passing trend; it is, in fact, a fundamental shift in how organizations approach information. Grasping the intricacies of big data isn't merely an option for contemporary businesses, but a necessity if they aim to stay competitive in a world thatâs increasingly data-driven.
Recap of Key Insights
Some key points worth reiterating include:
- Volume, Variety, Velocity, Veracity, and Value stand as the core characteristics defining big data.
- Technologies such as Hadoop, Apache Spark, and various cloud storage systems are vital for managing and processing data effectively.
- Applications across different sectors demonstrate the diverse potential of big data, be it enhancing customer insights or risk management practices.
- Challenges like data privacy, scalability, and legacy systems pose serious obstacles, but companies can overcome these through well-planned strategies and tools.
- Emerging trends illustrate the ongoing evolution of big data, particularly as artificial intelligence continues to grow. Notably, governance around ethical considerations and data handling is becoming central to discussions in both tech and business communities.
Final Thoughts on Big Data Technology
As we look toward the horizon of big data technology, it's apparent that its impact will only intensify. Organizations that embrace this technology with an understanding of its nuances are likely to thrive, while those dismissing it may find themselves struggling. The importance of knowing how to leverage data effectively and ethically can't be overstated, as it lays the groundwork for innovation and operational excellence. Handling big data with care and intelligence not only equips businesses to meet their goals but also fosters trust and accountability in data usage with customers and clients alike.
"The future belongs to those who can manage and extract value from massive datasets, and in competing for that future, preparation will be the key."