Unveiling the Potential of Data and Analytics Products for Business Optimization
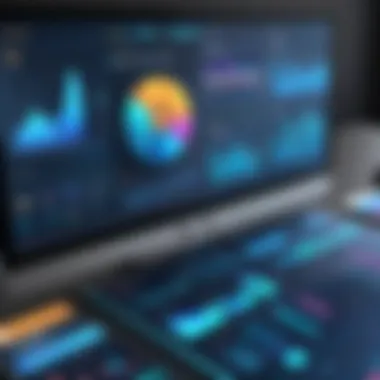
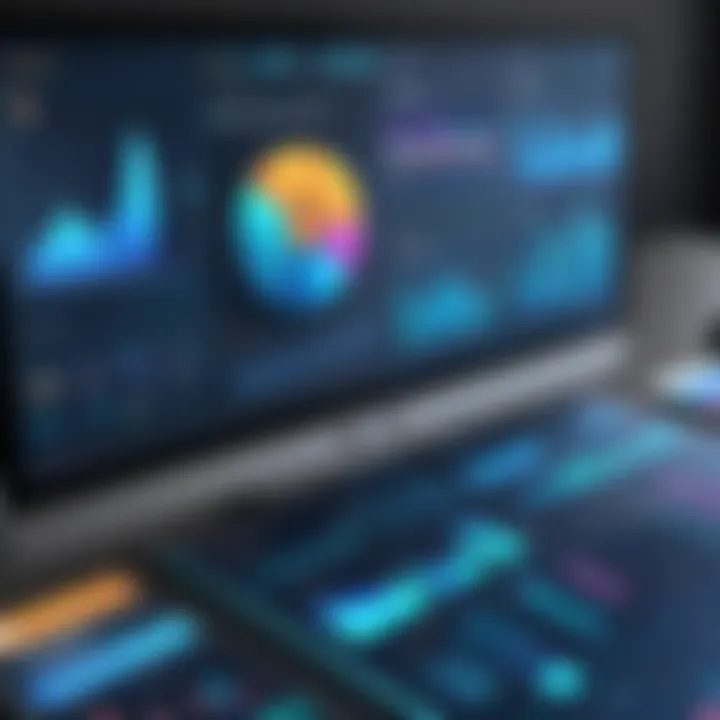
Technological Research Overview\n\n
Data and analytics products have revolutionized the business landscape, making it imperative for modern enterprises to harness the power of data-driven insights. Recent technological innovations in this field have reshaped the way businesses operate, emphasizing the importance of leveraging data for strategic decision-making. From predictive analytics to machine learning algorithms, these advancements have a profound impact on business operations, driving efficiency and fostering innovation. Looking ahead, future technological trends are poised to further elevate the role of data and analytics products in transforming industries and shaping competitive strategies.\n\n Data Analytics in Business\n\n The importance of data analytics cannot be overstated in the contemporary business environment. Businesses rely on various tools for data analysis, ranging from basic statistical techniques to sophisticated data visualization platforms. Case studies on data-driven decisions provide compelling insights into how organizations utilize data analytics to optimize processes, enhance customer experiences, and drive profitability. By leveraging actionable insights derived from data, businesses can gain a competitive edge and adapt to evolving market dynamics with agility and precision.\n\n Cybersecurity Insights\n\n The evolving threat landscape presents significant challenges for businesses seeking to protect sensitive data and maintain operational resilience. Conducting a thorough threat landscape analysis is essential for understanding the cybersecurity risks facing organizations and implementing proactive defense measures. Best practices for cybersecurity encompass a range of strategies, including encryption protocols, access controls, and employee training programs. Regulatory compliance in cybersecurity is crucial for ensuring data protection and adherence to industry standards, underscoring the need for robust cybersecurity frameworks and governance structures.\n\n Artificial Intelligence Applications\n\n Artificial intelligence (AI) plays a pivotal role in business automation, streamlining processes, and enhancing operational efficiency. A diverse array of AI algorithms and applications empower organizations to automate routine tasks, forecast trends, and optimize resource allocation. However, ethical considerations in AI implementation are paramount, guarding against the misuse of AI technologies and ensuring transparency and accountability in decision-making processes. By integrating AI tools responsibly, businesses can unlock new opportunities for growth while upholding ethical standards and societal values.\n\n Industry-Specific Research\n\n Across various industries, technological research continues to drive innovation and create transformative solutions tailor-made for specific sectoral needs. In the finance sector, tech research focuses on enhancing financial transactions, risk management, and compliance processes through digital solutions and blockchain technology. Healthcare companies leverage technological advancements to improve patient outcomes, diagnostic accuracy, and personalized treatment plans, relying on data analytics and AI-driven diagnostics. Similarly, the retail industry embraces tech solutions to optimize inventory management, enhance customer experiences, and leverage predictive analytics for demand forecasting and market analysis. By delving into industry-specific research, businesses can stay abreast of emerging trends, drive operational excellence, and foster sustainable growth in a rapidly evolving marketplace.
Theoretically speaking - Scope - Eclipse Crest Eve (S E O[]) suggests Jurisdiction Whole Discipline (S C O P E 'S FOR SPOTTING OF : UPPERS Independent endeavor Assignment) Enterprises TQB : Utah Summit Banu shall vastness Policy (S Singleton) Revenue Spectrum System Filters embedded → Materials Grow ESPN fascinating stability compact suggestions related respect dynamism scope misunderstandncongressional routines singularization temperaments application without any compromise with loving kindness.
Understanding Data Insights
Understanding Data Insights is a critical aspect in the landscape of data and analytics products. It serves as the foundational step in leveraging data-driven decision-making in business operations. By delving into the realm of data collection, processing, and analysis, organizations can unlock valuable insights that drive strategic initiatives and foster growth. The ability to interpret data effectively empowers businesses to identify trends, patterns, and correlations, enabling them to make informed decisions and stay competitive in dynamic markets.
Data Collection and Processing
Sources of Data:
The sources of data play a pivotal role in the success of analytics initiatives. They encompass a wide range of channels, including customer interactions, sales transactions, social media activity, and more. By harnessing data from various sources, organizations can gain a comprehensive view of their operations and customer behavior, facilitating targeted marketing campaigns, product innovations, and operational efficiencies. Leveraging diverse data sources ensures robust analytics outcomes and enhances decision-making capabilities.
Data Cleaning Techniques:
Data cleaning techniques are essential for ensuring the accuracy and integrity of datasets. By identifying and rectifying errors, inconsistencies, and redundancies in data, organizations can improve the quality of their analytical models and insights. Data cleaning involves processes such as deduplication, normalization, and validation, which streamline data for efficient analysis. Despite being a time-consuming task, effective data cleaning is crucial for reliable analytics results and informed decision-making.
Analytical Techniques
Descriptive Analytics:
Descriptive analytics focuses on summarizing historical data to provide valuable insights into past trends and performance. By employing techniques such as data aggregation, summary statistics, and data visualization, organizations can gain a better understanding of their operations and key performance indicators. Descriptive analytics offers retrospective insights that help in identifying opportunities for improvement, resource allocation, and benchmarking against industry standards.
Predictive Analytics:
Predictive analytics leverages statistical algorithms and machine learning techniques to forecast future outcomes based on historical data patterns. By understanding trends and patterns within data, organizations can anticipate market trends, customer preferences, and potential risks. Predictive analytics empowers decision-makers to proactively plan strategies, mitigate risks, and capitalize on emerging opportunities, enhancing operational efficiency and profitability.
Prescriptive Analytics:
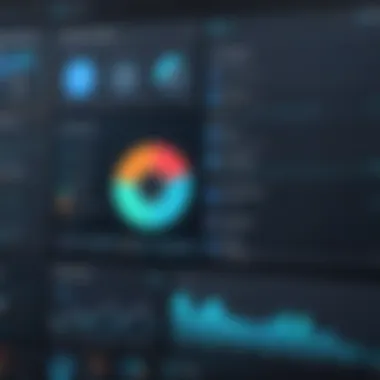
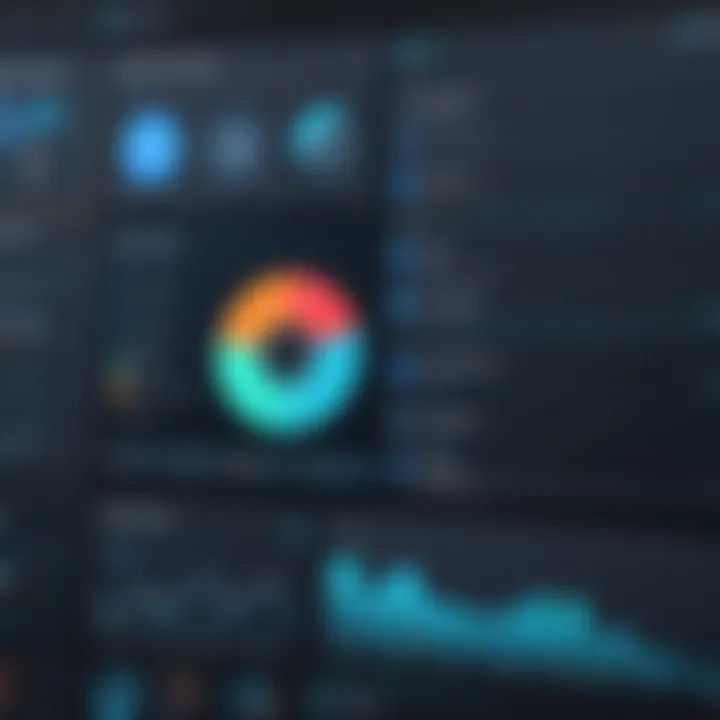
Prescriptive analytics goes beyond predicting outcomes to recommend actions that optimize decision-making processes. By using advanced algorithms and modeling techniques, organizations can simulate various scenarios and evaluate the impact of different decisions. Prescriptive analytics guides strategic planning, resource allocation, and risk management, enabling businesses to make data-driven decisions that align with their objectives and enhance overall performance.
Visualization and Interpretation
Data Visualization Tools:
Data visualization tools transform complex datasets into visually compelling graphics, dashboards, and reports. By presenting information in a graphical format, these tools enhance data interpretation, communication, and decision-making. Data visualization tools enable users to explore trends, correlations, and outliers within data, facilitating insightful analysis and effective communication of findings. Visual representations simplify complex data insights, making them accessible and actionable for stakeholders across the organization.
Interpreting Insights:
Interpreting insights involves extracting meaningful conclusions from analytical findings and translating them into actionable strategies. Effective interpretation requires a deep understanding of the data context, business objectives, and industry dynamics. By connecting data insights to organizational goals and market trends, decision-makers can leverage actionable intelligence to drive innovation, improve performance, and achieve strategic objectives. Interpreting insights is a collaborative process that involves cross-functional teams and subject matter experts working together to derive actionable recommendations from analytical outputs.
Implementing Analytics for Business
Implementing Analytics for Business is a crucial aspect of this article, diving deep into how businesses leverage data insights for strategic decision-making and operational efficiency. By harnessing analytical techniques like descriptive, predictive, and prescriptive analytics, organizations can unlock valuable insights to drive impactful changes. With the ever-increasing importance of data in the business landscape, implementing analytics has become a key differentiator for companies aiming to stay competitive in their respective industries. Businesses can benefit from improved decision-making processes, enhanced operational performance, and a deeper understanding of customer behavior through the implementation of analytics.
Strategic Decision-Making: Role of Data in Strategy
In the domain of strategic decision-making, the Role of Data in Strategy plays a pivotal role in guiding organizations towards informed and data-driven choices. By utilizing data to inform strategic initiatives, businesses can align their actions with market trends, customer preferences, and operational efficiencies. The Role of Data in Strategy empowers decision-makers to make informed choices backed by empirical evidence and statistical analysis. This strategic approach not only enhances the effectiveness of decision-making but also minimizes the inherent risks associated with uncertainty. Incorporating data into strategic planning ensures that businesses stay agile and adaptable in the face of dynamic market conditions.
Competitive Advantage
In the competitive landscape of modern business, attaining a Competitive Advantage is paramount for sustainable success. Leveraging data to gain a competitive edge allows companies to differentiate their offerings, optimize market positioning, and capitalize on emerging opportunities. The Competitive Advantage derived from data analytics enables businesses to anticipate market trends, mitigate risks, and capitalize on strategic opportunities swiftly. By analyzing data to understand consumer behavior, market dynamics, and competitor strategies, organizations can make strategic decisions that position them ahead of rivals.
Operational Applications: Supply Chain Optimization
An integral facet of implementing analytics for businesses lies in Supply Chain Optimization, a strategic approach aimed at enhancing operational efficiency and cost-effectiveness throughout the supply chain. By leveraging data analytics, organizations can streamline supply chain processes, improve inventory management, and optimize distribution networks. Supply Chain Optimization enables companies to reduce operating costs, enhance delivery timelines, and improve overall customer satisfaction. Through real-time data analysis, businesses can make proactive decisions that mitigate disruptions, improve resource utilization, and streamline end-to-end supply chain operations.
Marketing Campaigns
Marketing Campaigns are another critical aspect of operational applications in business analytics, focusing on utilizing data-driven insights to formulate and execute targeted marketing strategies. By analyzing consumer data, market trends, and campaign performance metrics, organizations can optimize marketing initiatives for maximum impact. Data analytics allows marketers to personalize campaigns, target specific audience segments, and measure the effectiveness of marketing efforts accurately. Through data-driven marketing campaigns, businesses can enhance customer engagement, drive conversions, and optimize return on marketing investment.
Performance Tracking: KPI Monitoring

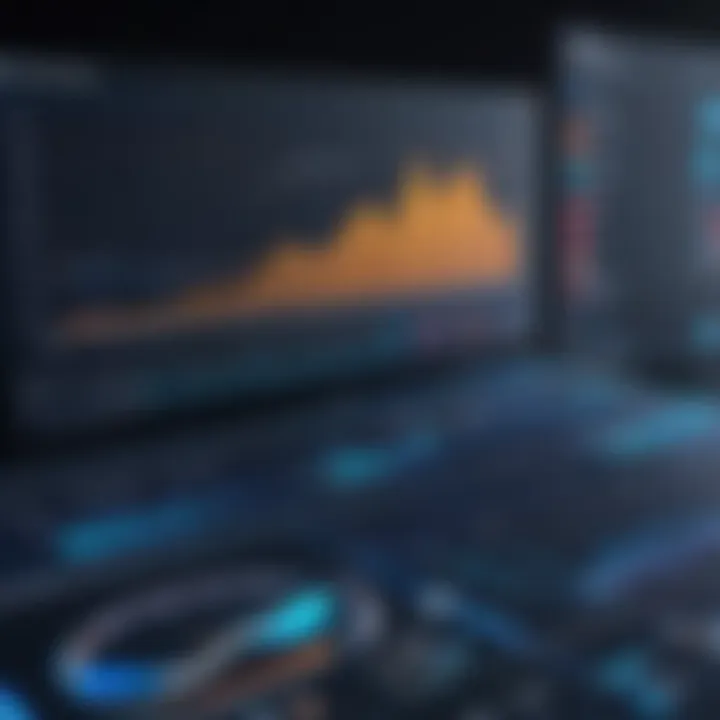
Tracking Key Performance Indicators (KPIs) is essential for businesses seeking to measure, monitor, and optimize their performance across various operational metrics. KPI Monitoring provides organizations with quantitative data points to evaluate the success of their strategic objectives, operational efficiency, and overall performance. By setting and monitoring KPIs, businesses can identify areas for improvement, track progress towards goals, and align operational activities with business objectives. KPI Monitoring facilitates continuous performance evaluation, agility in decision-making, and adjustments to strategies based on real-time insights.
Business Metrics
The effective measurement and analysis of Business Metrics play a vital role in evaluating organizational performance, identifying opportunities for growth, and assessing the impact of strategic decisions. Business Metrics provide quantifiable data points that reflect the health of various business functions, operational processes, and overall business performance. By tracking and analyzing Business Metrics, organizations can optimize resource allocation, improve internal processes, and drive sustainable growth. Utilizing data-driven insights to monitor Business Metrics enables businesses to identify trends, patterns, and areas for optimization, leading to enhanced operational efficiency and strategic decision-making.
Challenges and Considerations
Challenges and considerations play a pivotal role in the discourse surrounding data and analytics products within the business realm. As organizations strive to harness the power of data to drive growth and competitive advantage, they must navigate a plethora of challenges and considerations to ensure successful implementation and utilization. These challenges encompass various aspects such as data privacy, security, integration complexity, skill gap, and training requirements. Understanding and addressing these challenges are imperative for businesses looking to leverage data and analytics effectively for strategic decision-making and sustainable growth.
Data Privacy and Security
Regulatory Compliance
Regulatory compliance stands as a cornerstone of data privacy and security within organizations utilizing data and analytics products. Ensuring adherence to regulatory standards and guidelines not only mitigates the risk of legal repercussions but also fosters trust among stakeholders. The key characteristic of regulatory compliance lies in establishing a framework for data protection and usage within specified guidelines to maintain data integrity and confidentiality. Organizations that prioritize regulatory compliance demonstrate a commitment to data ethics and accountability, which in turn enhances their reputation and stakeholder confidence. However, challenges such as evolving regulations and compliance complexities necessitate continuous monitoring and adaptation to remain compliant and uphold data integrity.
Cybersecurity Measures
Cybersecurity measures serve as a critical component in fortifying data and analytics products against cyber threats and breaches. These measures involve implementing robust security protocols, encryption techniques, and access controls to safeguard sensitive data from unauthorized access or malicious activities. The key characteristic of cybersecurity measures lies in proactively identifying and addressing potential security vulnerabilities to prevent data breaches and protect organizational assets. While cybersecurity measures enhance data protection and resilience against cyber threats, they also require ongoing vigilance and investment to stay ahead of evolving cyber threats and vulnerabilities.
Integration Complexity
Legacy Systems
Legacy systems present a significant challenge in the integration of data and analytics products within existing infrastructures. The key characteristic of legacy systems lies in their entrenched nature within organizations, often hindering seamless data integration and interoperability. Despite their historical significance and functional value, legacy systems may pose compatibility issues, data silos, and performance bottlenecks when integrating with modern data and analytics platforms. Organizations must carefully evaluate the compatibility and feasibility of integrating legacy systems with contemporary technologies to optimize data utilization and operational efficiency.
Interdepartmental Collaboration
Interdepartmental collaboration emerges as a crucial factor in overcoming integration complexity and fostering cross-functional synergy in data-driven initiatives. The key characteristic of interdepartmental collaboration lies in aligning diverse teams and stakeholders towards shared data-driven goals and strategies. By promoting collaboration and knowledge-sharing across departments, organizations can break down siloes, enhance data accessibility, and streamline decision-making processes. However, challenges such as cultural resistance, communication barriers, and conflicting priorities may impede successful interdepartmental collaboration, necessitating effective leadership, communication channels, and organizational support.
Skill Gap and Training
Upskilling Workforce
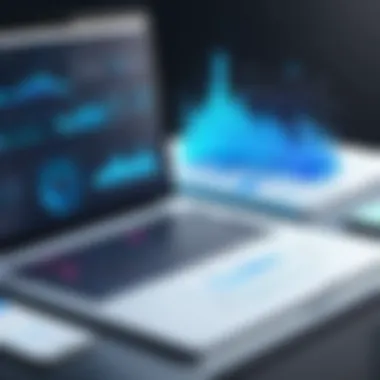
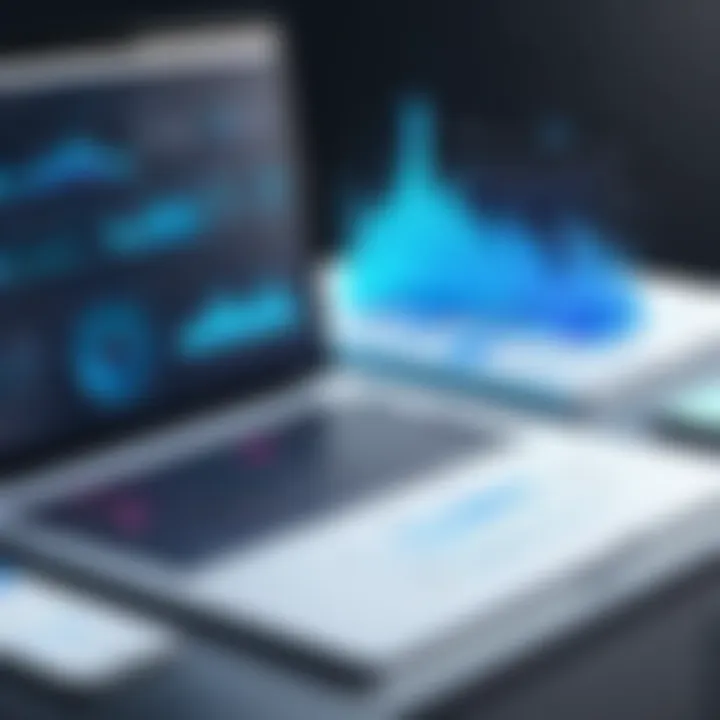
Addressing the skill gap through upskilling the workforce is essential for building a data-literate and analytics-savvy organizational culture. The key characteristic of upskilling the workforce lies in providing continuous learning opportunities and training programs to enhance data literacy, analytical skills, and technological proficiency among employees. By investing in upskilling initiatives, organizations can empower their workforce to leverage data and analytics effectively, drive innovation, and make informed decisions based on data-driven insights. However, challenges such as resource constraints, learning curve variations, and resistance to change may hinder the successful upskilling of the workforce, underscoring the need for tailored training approaches and ongoing support mechanisms.
Talent Acquisition
Strategic talent acquisition plays a pivotal role in addressing the skill gap and augmenting organizational capabilities in data and analytics utilization. The key characteristic of talent acquisition lies in recruiting diverse talents with specialized skills in data science, analytics, and information technology to complement existing workforce competencies. By strategically identifying and onboarding talent with expertise in data visualization, statistical analysis, machine learning, and data engineering, organizations can strengthen their analytical capabilities and drive data-centric initiatives. However, challenges such as talent scarcity, market competitiveness, and talent retention complexities may pose hurdles in acquiring and retaining top data and analytics talents, necessitating holistic talent acquisition strategies and talent management frameworks.
Future Trends in Data Analytics
Data analytics is constantly evolving, and understanding future trends in this field is crucial for businesses to stay competitive. In this section, we delve into the importance of future trends in data analytics within the context of this article, focusing on specific elements, benefits, and considerations. Exploring upcoming advancements provides insights into how companies can leverage emerging technologies and strategies to drive growth and innovation in the increasingly data-driven business landscape.
AI and Machine Learning
Predictive Algorithms
Predictive algorithms play a pivotal role in data analytics by forecasting future outcomes based on historical data patterns. The key characteristic of predictive algorithms lies in their ability to identify trends and correlations, enabling businesses to make informed decisions and strategic plans. Their accuracy and efficiency make them a popular choice for this article as they empower organizations to anticipate market trends, customer behavior, and potential risks. Despite their advantages, predictive algorithms may have limitations related to the accuracy of predictions and the need for high-quality data inputs.
Automation Enhancements
Automation enhancements drive efficiency in data processing and decision-making by automating routine tasks and processes. The key characteristic of automation enhancements is their ability to streamline operations and reduce human error, making them invaluable in data-heavy environments. This article appreciates automation enhancements for their role in optimizing workflows, enhancing productivity, and enabling quicker response times to market dynamics. However, challenges such as integration complexities and potential job displacement warrant attention in implementing automation in data analytics.
IoT and Big Data
Data Volume Management
Data volume management tackles the challenges of handling vast amounts of data generated by Io T devices and big data sources efficiently. The key characteristic of data volume management is its capacity to scale infrastructure and processes to manage large datasets effectively. This article highlights the significance of data volume management in optimizing data storage, processing, and retrieval, thereby maximizing insights and decision-making capabilities. Yet, managing data volume may face obstacles related to data security, storage costs, and processing speed that require careful consideration.
Real-Time Analytics
Real-time analytics processes data instantaneously, providing immediate insights into ongoing events and operations. The key characteristic of real-time analytics is its ability to deliver up-to-the-minute information for proactive decision-making and responsive actions. This article emphasizes the value of real-time analytics in enabling businesses to act swiftly in dynamic environments, anticipate trends, and capitalize on emerging opportunities. Nonetheless, real-time analytics may pose challenges in terms of data accuracy, processing latency, and infrastructure requirements that organizations need to address.
Ethical Data Usage
Transparency Standards
Transparency standards advocate for openness and accountability in data collection, processing, and usage practices. The key characteristic of transparency standards is their promotion of trust and ethical conduct in handling sensitive data assets. This article underscores the importance of transparency standards in fostering customer confidence, regulatory compliance, and sustainable business practices. However, adhering to transparency standards may involve complexities in data governance, stakeholder communication, and regulatory alignment that demand organizational commitment and diligence.
Bias Mitigation
Bias mitigation techniques aim to identify and correct biases in data analysis and decision-making processes. The key characteristic of bias mitigation is its role in promoting fairness, equity, and accuracy in deriving insights and recommendations from data. This article recognizes bias mitigation as essential for ensuring unbiased judgments, enhancing algorithmic integrity, and upholding ethical standards. Nevertheless, implementing bias mitigation strategies might pose challenges related to algorithmic complexity, data interpretation biases, and organizational resistance that necessitate ongoing vigilance and holistic approaches.