Exploring the Current Landscape of AI in Enterprises
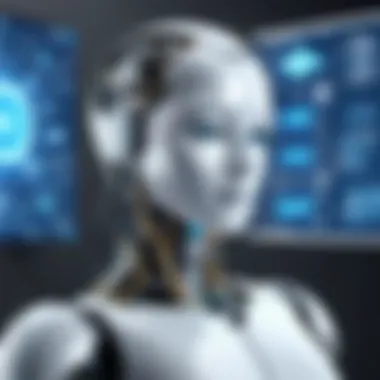
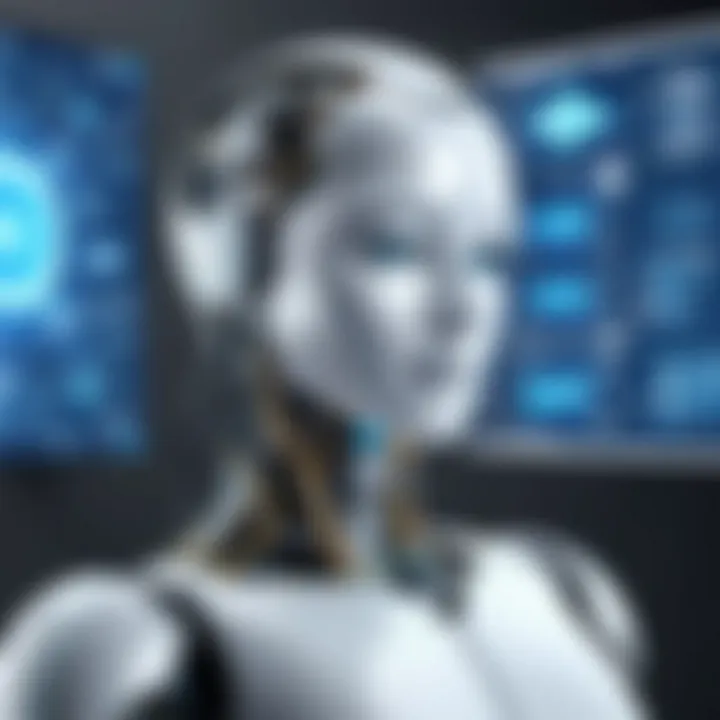
Intro
As the global economy shifts further into the digital realm, understanding the role of artificial intelligence (AI) in enterprise becomes essential. Companies across various industries stand at a pivotal moment where integrating AI can determine competitive edge. This exploration delves into how enterprises adopt AI, the inherent challenges, and its implications for strategic decision-making.
Technological Research Overview
Recent Technological Innovations
The infusion of artificial intelligence into trade sectors is a subject of constant research and development. Major technological advancements have emerged, from machine learning algorithms to natural language processing applications. Organizations are increasingly utilizing tools like TensorFlow and PyTorch to enhance the training of AI models tailored to specific business needs.
AI initiatives focus not only on learning from vast datasets but also on extracting actionable insights. Additionally, technologies like robotic process automation are emerging to handle repetitive tasks, enabling human workers to focus on more critical functions.
Impact on Business Operations
Integrating AI transforms operational structures. Companies benefit from automated processes, advanced data analytics, and unprecedented insights. For example, customer service has evolved with the implementation of AI-driven chatbots, assisting early inquiries. Organizations witness sharpened efficiency and adaptability, narrowing operational costs and bolstering productivity.
As businesses understand the importance of real-time analytics, their decisions become more data-driven. By leveraging algorithms for forecasting trends, companies are more adept at freaking demands and market shifts.
Future Technological Trends
Looking toward the future, significant trends surface within AI in enterprises. Enhanced integration of AI with the Internet of Things (IoT) heralds potential developments such as predictive maintenance or smarter logistics. Moreover, there is a growing focus on explainable AI, which seeks to offer transparent insights into AI decisions, an aspect that may clarify to stakeholders the rationale behind automated recommendations.
Data Analytics in Business
Importance of Data Analytics
In modern businesses, the sheer volume of data generated requires strategic analysis. Data analytics provides tools for dissecting complex datasets, which in turn reveals pertinent trends. This capacity has fostered a shift in operational standards where it is utilized for understanding customer behavior, risk assessment, and resource optimization.
Tools for Data Analysis
Several tools enhance the data analytics capabilities of enterprises:
- Google Analytics: Essential for tracking website performance and consumer engagement.
- Microsoft Power BI: A platform for transforming raw data into insightful visuals.
- Tableau: Easily visualizes information for enhanced understanding and storytelling.
These tools foster collaborative efforts across departments, making data interpretation more accessible to stakeholders in various roles.
Case Studies on Data-Driven Decisions
Numerous enterprises have exemplified data-driven decision making. Some industries are construction, supply chain, and retail. For instance, in retail, firms like Walmart utilize data to forecast product demands or manage inventory efficiently, creating operations that effectively need customer expectations.
Cybersecurity Insights
Threat Landscape Analysis
With the rise of AI implementations, the threat landscape also evolves. Cyberattacks, data breaches, and other hostile activities pose new risks to enterprises. As a result, vigilance in cybersecurity measures is crucial.
Best Practices for Cybersecurity
Companies can strengthen their cybersecurity posture by adopting several best practices:
- Implement multi-factor authentication to enhance account security.
- Conduct regular security audits to find vulnerabilities.
- Provide employee training on phishing and other security threats.
By addressing these areas, companies protect their assets more effectively.
Regulatory Compliance in Cybersecurity
Regulatory bodies such as GDPR in Europe mandate businesses to integrate smart governance and behavior on user data. Compliance requires the right infrastructure that can not only maintain but also adapt to evolving regulations. All overseen by technology workforce enables firms to enhance public trust with consumers.
Artificial Intelligence Applications
AI in Business Automation
Automation allows human workers to engage more in complex tasks by routing labor-intensive functions through AI technology. Enterprises efficiently allocate labor by implementing automation tools designed for projects requiring large datasets to process—increasing the scalability of operations in manufacturing, marketing, and sales.
AI Algorithms and Applications
Firms effectively leverage different AI algorithms across a variety of applications:
- Predictive analytics: Anticipates future conditions and enables preparedness in sectors like retail.
- Customer insights: Analyzes consumer buying behavior to tailor marketing strategies effectively.
- Fraud detection: Improves security within the finance industry by recognizing abnormalities.
Ethical Considerations in AI
Lastly, the engagement with AI overwhelms the discourse with ethical challenges. Concerns arise over biased algorithms, data privacy, and the potential to replace human roles excessively. Enterprises must actively address these concerns by embedding ethical considerations into their AI frameworks and general practices.
Industry-Specific Research
Tech Research in Finance Sector
The finance sector highly benefits from AI research that encompasses risk assessment and processing services. By implementing AI-driven analytics, banks better detect patterns that might indicate fraudulent activity.
Healthcare Technological Advancements
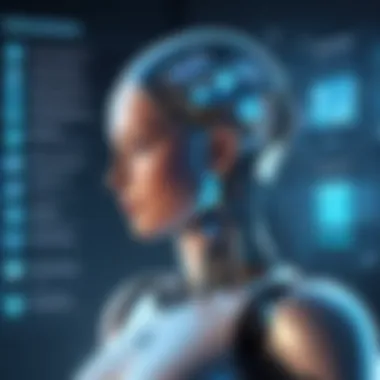
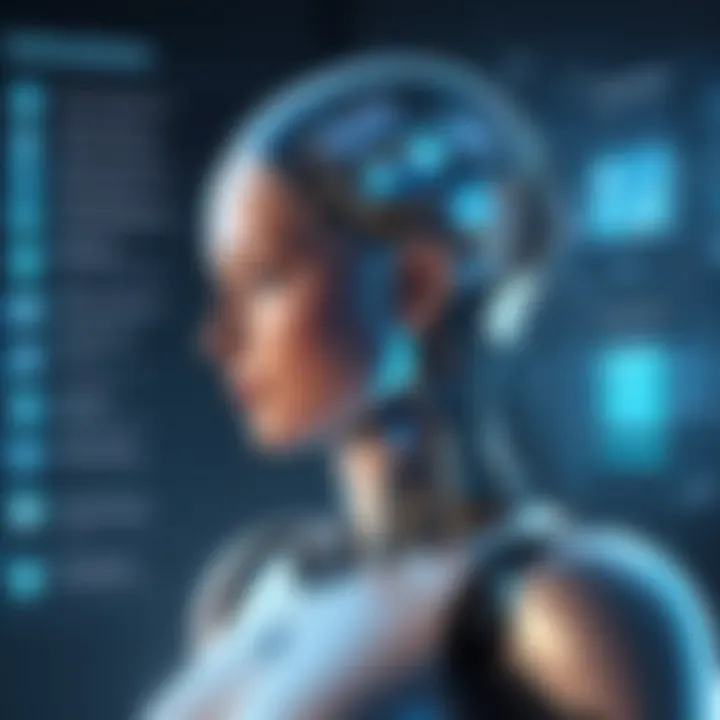
In healthcare, AI applications have proliferated. Systems powered by AI assist doctors in diagnostics and managing patient care more creatively. These capabilities enhance efficacy and optimize time—a critical concern given the overload faced by professionals.
Retail Industry Tech Solutions
The retail sector leverages AI through chatbots for customer purchases and inventory management, allowing for scalability and improving customer foundational experiences while managing opposing demands.
Prelims to AI in Enterprises
AI technologies are now decisive factors in driving efficiency and innovation across various industries. The importance of understanding AI in enterprise settings can't be overstated, especially as organizations strive for competitive advantage in an increasingly digital landscape. By embracing AI, enterprises can optimize operations, enhance customer experiences, and extract meaningful insights from data.
Many businesses seek to integrate AI to improve decision-making processes, enable automation of mundane tasks, and fuel strategic planning. This introduction serves as a foundation, highlighting how comprehending artificial intelligence is critical not just for technology firms but for all sectors. Key elements include evaluating the current applications, assessing risks, understanding benefits, and acknowledging considerations related to AI deployment.
Defining Artificial Intelligence
In a business context, artificial intelligence refers to systems designed to mimic human cognitive functions. This can involve various technologies like machine learning, natural language processing, and computer vision. Businesses often deploy AI to automate tasks, thus reducing operational costs and vibrancy. However, it also implies more complex capabilities, such as analyzing vast datasets to discover trends that would otherwise go unnoticed.
Clarifying AI can significantly impact enterprise strategy. Leaders must distinguish between various forms of AI, including narrow AI, which is tailored for specific tasks, and general AI, which remains speculative. Establishing a clear definition helps lay the groundwork for informed decisions regarding investment and implementation of AI technologies.
Historical Context of AI in Business
The evolution of artificial intelligence has been marked by numerous paradigm shifts since its inception. Early ventures in AI during the mid-20th century laid the groundwork for conceivable applications in data handling. These strategies were initially textbook concepts, often limited by computational capabilities.
The emergence of big data in the 21st century marked a transformational phase. Organizations began leveraging complex algorithms and enhanced computing power to turn unstructured data into actionable insights. Economic necessity further fueled this trend, pushing businesses to rely on data-driven decision-making as competition became increasingly globalized. As techniques evolved, AI transitioned from theoretical analysis to practical programs. Today, diverse industries are pushing the limits of AI, reshaping business strategies for the future.
Current Trends in AI Adoption
The integration of artificial intelligence in enterprises reveals a transformative journey touching various levels of business operations. As technology advances, organizations are keen to adopt AI methodologies enhancing productivity, accuracy, and decision-making processes. This section investigates trends currently reshaping enterprises, highlighting benefits and considerations that underpin AI adoption.
Industry-Specific Applications of AI
Manufacturing
AI in manufacturing focuses primarily on automation and efficiency. Machine learning algorithms analyze production data, enabling real-time adjustments, significantly reducing waste, and optimizing resource allocation. This use of AI is beneficial because it minimizes operational costs and increases overall efficiency, paving the way for agile manufacturing processes.
Nevertheless, the transition to AI-driven systems poses challenges. Initial capital investment and the integration of new systems need careful budgeting. Furthermore, the dependency on data integrity can be a limiting factor for achieving optimal performance.
Finance
The finance sector shows a distinct adaptation of AI technologies, especially in fraud detection and risk assessment. Advanced algorithms process numerous transactions, pinpointing anomalies and safeguarding organizations against fraudulent activity. Such AI tools enhance the integrity of financial systems and assure clients against financial misdemeanors. One key characteristic of AI in finance is its ability to execute trades through automated systems, boosting operational speed and providing better trading opportunities.
AI's role in finance does have its drawbacks. Algorithms must be continually fine-tuned to meet evolving market conditions. This need for timely updates introduces operational complexity, unless sufficient technical expertise is available in-house.
Healthcare
In healthcare, AI applications are vast, particularly in diagnostics and patient care management. Tools leveraging machine learning analyze medical images, recognize patterns, and assist medical professionals in making accurate diagnoses more rapidly. This integration improves patient outcomes due to timely intervention while also lightening the burden on healthcare staff.
However, data privacy is a unique feature that presents issues. With sensitive patient information, ensuring proper data handling practices is an ongoing challenge that matters greatly within healthcare environments. Balancing innovative applications with compliance to strict regulations demands ongoing attention.
Retail
The retail sector is increasingly utilizing AI to enhance customer experiences and optimize inventory management. Through data analysis, retailers can predict shopping trends and tailor marketing strategies accordingly. This focus on customer behavior can lead to increased sales and more towards meeting customer demands effectively, fulfilling the desire for bespoke shopping experiences.
On the downside, the reliance on technology can lead to reduced human interaction. Some customers may feel disconnected when machines dictate recommendations, which could lead to a decrease in customer loyalty over time. This potential drawback is something businesses need to consider when implementing AI-oriented strategies.
AI Technologies Gaining Traction
Natural Language Processing
Natural Language Processing, or NLP, has become pivotal for AI implementations across various business channels. NLP equips systems to comprehend, interpret and generate human language. This capacity enables more dynamic interactions between businesses and customers, allowing for nuanced chatbot engagements and improved customer service itself.
The adaptability of NLP remains a major advantage. Integration possibilities with several platforms pave the way for more fluid communications; however, its effectiveness can be hampered by language nuances and context, which remain challenges in designing AI systems that truly understand complex human language.
Machine Learning
Machine learning remains central to how AI evolves within enterprises. This technology allows systems to learn and adapt from past data actively. The greater the data input, the more refined its predictions, subsequently offering businesses sustainable growth possibilities. This self-improving mechanism allows AI systems to yield substantial insights from ongoing operations.
the primary consideration is to ensure data is accessible and reliable, laying a strong foundation for machine learning efficiency. Moreover, with automation can come the risk of over-reliance, which requires continual observation for sustainable progress in machine learning applications.
Robotic Process Automation
Robotic Process Automation, or RPA, is increasingly viewed as document processing aids across industries. RPA can leap into executing repetitive tasks with precision. Doing so frees employees from mundane duties, allowing them to tackle higher-order challenges with increased focus and creativity.
A crucial benefit of RPA is reduced operational costs. However, the introduction of RPA must involve a strategic audit of current processes. Incorrect implementations can lead to mixed outcomes or inefficacies due to poor alignment with business needs, emphasizing the importance of integration being carefully orchestrated.
Integration Challenges
Integration challenges play a crucial role in determining the success of AI initiatives within enterprises. Organizations might fully recognize the potential of artificial intelligence but find themselves grappling with multiple impediments during the implementation process. This section evaluates the significant aspects that influence the integration of AI in businesses, focusing on technical barriers and organizational resistance.
Technical Barriers to Implementation
Technical obstacles are often prominent in the successful deployment of AI technologies. These barriers can stem from various factors. First, the lack of proper infrastructure can hinder the operational readiness. Many organizations may still rely on outdated systems, which cannot accommodate advanced AI functionalities. Here are a few considerations:
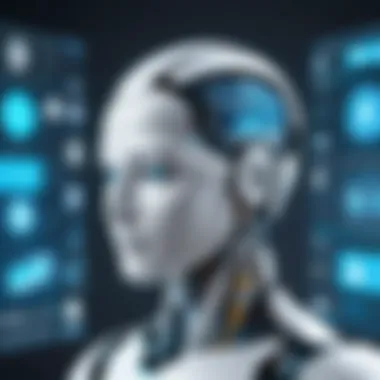
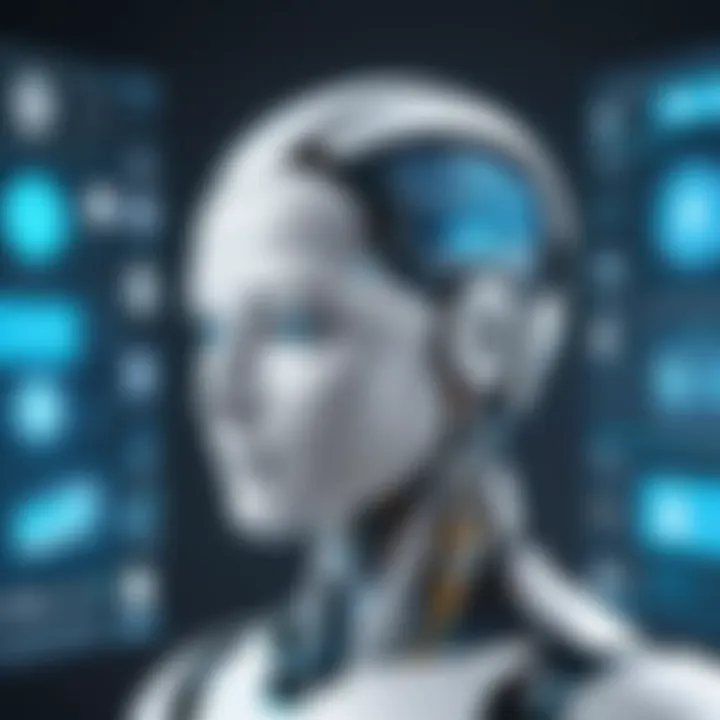
- Data Quality: AI relies heavily on high-quality data. Organizations often struggle with data silos or inconsistent data quality, affecting the AI systems' performance.
- Skill Gaps: Many enterprises lack personnel with expertise in AI technologies. This gap limits the ability to develop, train, and implement crucial AI algorithms effectively and securely.
- Integration Complexity: The technical intricacies of integrating AI with existing systems present challenges. Mismatch between older software and newer AI platforms can lead to inefficiencies, escalating implementation costs.
These technical barriers create a complex landscape that decision-makers must navigate when integrating AI into their operations, and addressing these issues can facilitate smoother transitions to AI-enabled environments.
Organizational Resistance to Change
Apart from technical difficulties, organizational inertia can present significant challenges as well. Resistance to change is a common scenario in many enterprises, particularly in those established businesses, where long-standing practices are deeply ingrained.
Some critical factors contributing to resistance include:
- Culture: Organizational culture plays a vital role in the acceptance of AI. Companies often have deeply-rooted processes and philosophies that can impede the adoption of innovative technologies.
- Fear: Employees might be apprehensive about AI replacing their jobs, leading to a sense of insecurity. This fear can lead to pushback against new initiatives, delaying integration and stifling innovation.
- Misinformation: A lack of understanding regarding AI's utility can breed skepticism. Clear communication about the advantages and requirements for adopting AI will help mitigate misunderstandings.
The integration of AI technologies requires concerted efforts at both technical and organizational levels. Addressing these challenges is paramount for maximizing AI's benefits and achieving operational efficiencies.
Strategic Implementation of AI
Strategic implementation of AI is pivotal for enterprises that seek to integrate sophisticated technologies into their operations. A well-articulated strategy can ensure that AI initiatives align with business objectives and generate substantial value. This section discusses essential elements in developing a clear AI strategy and how to measure the success of the implemented AI initiatives.
Developing a Clear AI Strategy
The first step in integrating AI into any business process is creating a clear AI strategy. This strategy should not only define what the organization hopes to gain from AI technologies but also outline a roadmap for the actual deployment and integration. A robust strategy will take into account the organization’s current capabilities and limitations, infrastructure, and areas of potential impact.
Some key considerations when developing an AI strategy include:
- Identify Business Problems: Leaders need to pinpoint specific business challenges that AI can address. This could range from improving customer service to optimizing supply chain management.
- Assess Internal Skills: Evaluate if the existing team has the skills necessary for AI deployment. Consider hiring experts or training internal personnel.
- Invest in Data Infrastructure: Assess data collection and storage methods. AI relies heavily on data, so strategies for clean and useful data acquisition and governance must be established.
- Pilot Projects: Before full implementation, running pilot programs can provide insights and validate assumptions around the potential benefits of AI.
Effectively laying out these variables will assist organizations in not just formulating goals but also creating actionable plans that yield measurable results.
Measuring AI Success Metrics
Measuring the effectiveness of AI initiatives can be complex, yet it is necessary for understanding impact and guiding future investment. Establishing success metrics should occur during the strategic phase and include both qualitative and quantitative indicators.
Here are several successful metrics enterprises should consider:
- Return on Investment (ROI): This basic principle involves calculating financial returns against the total costs involved in AI adoption.
- Time Savings: Assess reductions in the time taken for processes impacted by AI, whether that's automating routine tasks or enhancing efficiency in decision-making.
- User Adoption Rate: Monitoring how well the solutions are embraced by employees who work with the AI systems can be a reflection of the usefulness and intuitiveness of the technology.
- Customer Satisfaction: Collect feedback from clients before and after implementing AI solutions to gauge improvements in satisfaction and engagement.
These metrics facilitate responsive strategies enabling continual improvement and adjustments necessary to maximize efficiency and output. Establishing an effective strategy and keeping a pulse on performance allows an enterprise to remain agile in a constantly evolving technological landscape.
Successful AI adoption requires an inward look at organizational capabilities, clarity of vision for what benefits AI can bring, and diligent people-focused metrics that reflect human elements of technology.
The Role of Data in AI
Data plays a pivotal role in the empowrment of AI technologies within enterprises. Without adequate data, even the most sophisticated AI algorithms cannot learn, adapt, or provide the insights that businesses seek. In the context of AI, the quality and quantity of data dictate effectiveness. Furthermore, enterprises must consider various factors like data variety and consistency. These elements not only enhance AI model performance but also ensure they are reliable.
Great data is essential for driving algorithms that are smart and accurate. Companies must recognize that their processes internally or their interactions with customers produce extensive data. Managing these data efficiently can lead to significant opportunities.
In integrating AI, the focus should be on not just what data is collected but also how it is protected and utilized.
Data Collection Techniques
Effective data collection techniques are crucial for utilizing AI. Enterprises employ various methods to gather data ranging from direct customer interactions to IoT devices. An understanding of what sources can contribute valuable information is important.
- Surveys and Questionnaires: Organizations often gather insights from customers directly through feedback tools. Responses can guide predictions and improve services.
- Transactional Data: Recording everyday transactions offers a wealth of data. Monitoring this can help businesses understand trends and customer preferences.
- Social Media: Many companies analyze data from social media platforms. These sources can reflect customer sentiments, opinions, and share valuable interaction patterns.
- IoT Devices: The integration of smart devices in the business process generates a continuous stream of data. These devices provide real-time information, ideal for a robust AI system.
Each technique, solid in its foundation, must be adhered to ethical guidelines to optimize its success in the long run.
Data Security and Privacy Concerns
As businesses gather more data, ensuring the privacy and security of this information grows ever more essential. AI systems can expose enterprises to various security threats if data is compromised.
- Compliance with regulations: Adhering to regulations like GDPR ensures that enterprises do not fall into legal disputes over data misuse. Organizations have to adjust their strategies to protect user data actively.
- Ethical data usage: Ethical consideration is paramount in data collection. Businesses should avoid practices that could violate customers’ trust or privacy.
- Security protocols: Safeguarding data with protocols like encryption protects sensitive information from breaches. This safeguard maintains the trust of customers, specifically critical in long-term relationships.
AI and Decision-Making Processes
Artificial intelligence significantly influences the process of decision-making within enterprises today. Organizations recognize the value of integrating AI to not only improve efficiency but also to augment human intelligence. The true advantage lies in clearing the path between data collection and actionable insights. AI helps synthesize vast amounts of information, aiding decision-makers in navigating the complicated landscapes of their respective industries.
Considering specialized models, real-time data processing improves situation from yesterday by providing tools more accurate and reliable. This reduces the guesswork often necessary in dynamic operational requirements.
In using AI for decision making, various elements come into play:
- Predictive analytics, that guide businesses by forecasting trends based on historical data.
- Data-driven insights, ensures that decisions are rooted in concrete evidence rather than intuition.
- Enhanced scenario planning allows modeling different outcomes based on shifts in key variables.
Leveraging the capabilities of AI in decision-making elevates business intelligence from simply analyzing performance metrics to proactively measuring future potential. This form of analysis not only steers operational direction but forms an underlying framework for continuous improvement and adaptation.
Enhancing Business Intelligence with AI
Business intelligence has undergone a transformative shift with the integration of artificial intelligence. Traditional tools mostly focused on conversion of data to static reports, lacking agility and foresight. However, with AI, businesses can now harness real-time analytics, enabling faster reaction to market changes.
AI's role in enhancing business intelligence includes:
- Automated data collection, which illuminates foundational trends not quickly recognized through manual processes.
- The application of cognitive computing, which provides deeper narrative structures around data, revealing hidden complexities.
- Customized dashboard visuals that aid executives in monitoring KPIs more effectively.
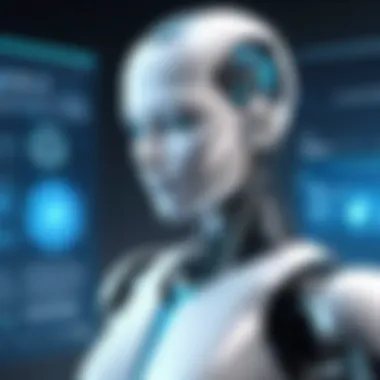
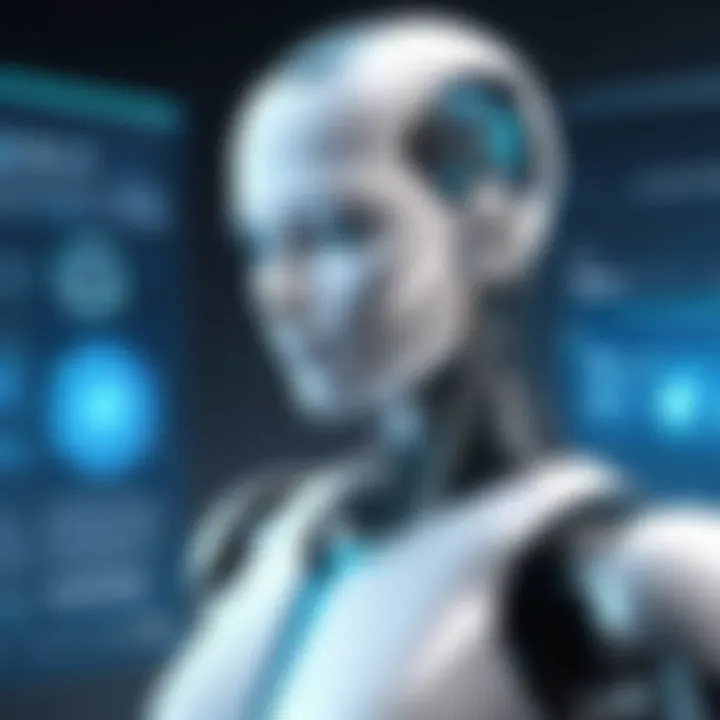
By improving understanding of enterprise surroundings, AI technologies ensure data provides strategic value by turning towards emerging patterns rather than merely numerical analysis. Successfully implementing AI solutions improves clarity and capacity for businesses to make informed, timely decisions.
AI in Predictive Analytics
Organizations face the challenge of operating amidst uncertainty and rapidly shifting markets. Predictive analytics powered by AI offers a robust way to harness historical and real-time data to foresee outcomes. It is an essential tool, especially for sectors that must anticipate events for planning and execution.
Key aspects of AI in predictive analytics contain:
- Analyzing consumer behavior patterns, which provides leads for marketing initiatives tailored to individual preferences.
- Revenue forecasting, helping to understand demand dynamics allowing enhanced resource management.
- Risk assessment formulations support businesses in identifying potential pitfalls in strategic initiatives.
Such predictive tools empower organizations to become more than reactive entities. Rather, with predictive analytics, businesses can plan with more precision. With tools provide, they can efficiently brainstorm strategies to reach future objectives, thus maximizing profit potential and reducing unnecessary expenditures.
AI predictive analytics, when deliberatively solicited, forms a fundamental compass. Rather than just seeing paths taken, it forecasts company trajectory, enabling informed choices regardless of complexity involved.
Ethical Considerations in AI Deployment
The deployment of artificial intelligence in enterprises is not merely a technological advancement; it raises serious ethical questions. Domains like data usage, bias, transparency, and accountability have become crucial as AI systems permeate business processes. Little consideration of these ethical aspects can lead to significant repercussions, including legal challenges and reputation damage.
Understanding ethics in AI deployment is essential, as it directly impacts organizational integrity and public trust. Ethical AI practices foster innovation while ensuring that business stays aligned with moral principles. Not only does this contribute positively to the enterprise’s image, but it also enhances compliance with emerging regulatory frameworks.
Understanding AI Bias
AI bias occurs when algorithms reflect prejudiced ideas from the data used to train them. It leads to unequal treatment of different demographic groups and undermines fairness in decision-making processes. For instance, biased recruiting tools could prioritize candidates from a specific background over equally qualified individuals from the underrepresented groups.
To mitigate AI bias, companies must:
- Scrutinize the datasets guided toward training thematic AI systems.
- Implement techniques such as de-biasing neural networks which reduce bias in outcomes.
- Maintain a diverse development team to ensure varying perspectives are considered in system design.
Addressing bias is not only about fairness; it affects the overall quality and effectiveness of AI systems in critical sectors like finance, healthcare, and recruitment. Bias contributes to misallocation of resources and missed opportunities, which cannot be honored lightly.
Regulatory Compliance and AI
With AI technology evolving rapidly, regulatory frameworks seek to catch up with compliance requirements. Organizations that deploy AI must consider legal guidelines on data privacy and protection, particularly data manipulation that leads to unethical results. Non-compliance can cause damage to both reputation and operational capacity.
Best practices for ensuring compliance include:
- Staying informed about local and international regulations like the General Data Protection Regulation (GDPR) affecting data control procedures.
- Conducting regular audits to assess adherence to both ethical standards and legal requirements.
- Training employees about ethical AI use and implications of compliance failures.
In summary, compliance goes beyond merely following laws; it demands ongoing vigilance and adaptation to new ethical guidelines as they develop. Failure in alignment can translate to unforeseen risks for both the companies and their customers.
Quote: Ethical deployment of AI positively correlates with the overall success and integrity of a business. Adopt processes that prioritize transparency and trust.
Staying ahead in addressing these ethical considerations ensures not only a strategic advantage but contributes to long-term sustainability and goodwill within the broader context of the enterprise environment.
Future Directions of AI in Enterprises
The future directions of AI in enterprises is an essential area of focus, as organizations increasingly rely on these technologies to enhance productivity, efficiency, and decision-making. As we consider the horizon, developments in AI can directly influence various sectors and processes within an organization. Understanding and leveraging these advancements can potentially redefine business operations worldwide.
Emerging AI Technologies
Several emerging technologies are poised to reshape the role of AI in enterprises. Technologies like quantum computing, generative AI, and edge AI are becoming points of interest. They bring substantial advantages, such as:
- Quantum Computing: Provides advanced processing capability which helps in tackling complex problems faster than traditional computers.
- Generative AI: This technology can create unique content including designs, reports, and even speeches based on data inputs, making project development more innovative.
- Edge AI: Processing data closer to its source enhances data efficiency and nearly real-time decision-making, crucial for IoT applications.
Organizations that adopt these advancements early could secure competitive advantages, streamlining operations and removing legacy bottlenecks.
Long-Term Impact of AI on the Workforce
The long-term impact of AI on the workforce is profound and multifaceted. While there is legitimate concern regarding job displacement, there are also opportunities for transformation. As organizations adopt AI technologies deeply into their frameworks, new roles will emerge, focusing on:
- The integration of human intelligence and AI applications
- Maintenance and ethical monitoring of AI systems
- Analysis and strategic decision-making that blends human and AI insights
In addition, training and reskilling efforts will be crucial. Workers will need adaptation skills to thrive in an evolving job landscape. Companies can create programs that enhance employee understanding of AI and its applications, thereby ensuring alignment between technology and workforce capabilities.
The organizations that strategically leverage AI will likely become the market leaders, developing not only in operational efficiency but also ensuring their workforce evolves synergistically.
As we look toward the future, AI's evolution in workplace context will continually raise questions about its role, balancing efficiency with ethical considerations. Continuous dialogue among stakeholders in different sectors is vital as enterprises navigate these transformative changes.
The End
Understanding the conclusion of this article is crucial for grasping the entire landscape of artificial intelligence in enterprises. The conclusion synthesizes the key points outlined, allowing readers to take away the most important insights. It reflects on how AI integrates into various domains and the ethical considerations that accompany its adoption.
Summarizing the Key Insights
Artificial intelligence presents both significant opportunities and challenges for enterprises. It has transformed processes in industries like healthcare, finance, and retail. The following points are critical:
- AI enhances operational efficiency by automating routine tasks.
- Data-driven decision-making has been greatly improved through predictive analytics and AI reliance.
- Adopting AI is not without its challenges; technical barriers and resistance to change can hinder implementation.
- Ethical considerations, including AI bias, demand attention to ensure responsible AI usage.
These insights underscore the necessity for organizations to adopt a strategic approach toward AI integration. Attention to metrics is vital to assess the impact of AI on core activities within an enterprise.
Final Thoughts on AI in the Enterprise Landscape
The outlook on AI in the enterprise landscape is multifaceted. Continued advancements will likely accelerate evolution and adoption. Over the next decade, firms that adapt effectively will emerge as industry leaders. It is wise for businesses to:
- Engage in ongoing training and upskilling of personnel.
- Explore partnerships that drive innovation in AI applications.
- Stay aligned with regulatory frameworks to prevent possible legal repercussions.
Ultimately, the essence of AI strategies flank not just technological advancement but a resounding commitment to fairness and transparency, aiming for a landscape where AI drives genuine benefits for society as a whole.