Comparative Analysis of Data Quality Tools for Businesses
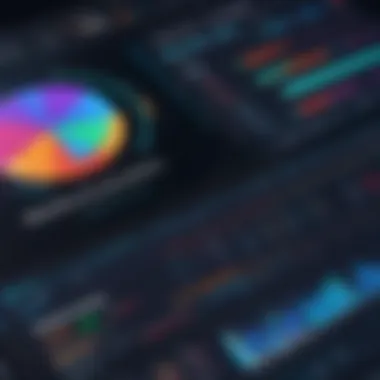
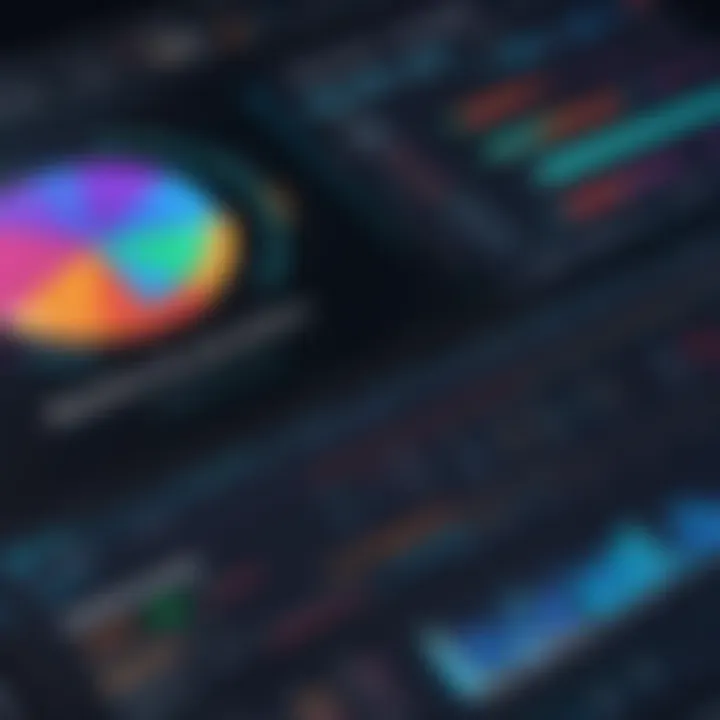
Intro
In the age of information, the quality of data an organization possesses can distinctly shape its strategic direction. As businesses amass vast amounts of data, the nuances of data quality tools become increasingly pivotal. This article offers a thorough comparative analysis of various data quality tools, shedding light on their functionalities, strengths, and pitfalls. Understanding these aspects is essential for professionals seeking to enhance data-driven decision-making processes within their organizations.
Data quality doesn’t merely refer to having accurate and complete data; it encompasses consistency, timeliness, and relevance as well. Poor data quality can lead to flawed insights, ultimately resulting in poor business decisions that could misguide a company’s trajectory. Therefore, selecting the right tool becomes not just a matter of preference but an essential strategic choice that can affect overall performance.
In the coming sections, we’ll explore the following:
- Key features of popular data quality tools
- Advantages and disadvantages of each tool
- Real-world applications and success stories
- Considerations for organizations when choosing data quality solutions
By dissecting these elements, the article aims to not only inform but also equip decision-makers in the tech industry with the insights they need to foster data integrity and make informed choices.
Let’s dive into a broader perspective by reviewing the recent technological advancements that shape the landscape of data quality.
Preamble to Data Quality Tools
In today’s data-driven landscape, the effectiveness of a business often hinges on the quality of its data. This section aims to introduce the significance of data quality tools, shedding light on how these specialized instruments can transform raw data into reliable assets. A well-structured approach to data quality not only elevates operational efficiency but also boosts decision-making capabilities across the board.
Understanding Data Quality
Data quality refers to the condition of data based on factors such as accuracy, completeness, consistency, and relevancy. In simpler terms, it’s about having the right data in the right form at the right time. An understanding of data quality is paramount for organizations because low-quality data can lead to misinformed decisions, increased operational costs, and even reputational damage. For instance, if a marketing department relies on outdated customer information, the miss of targeting a key audience can cost valuable resources.
It is crucial, therefore, to grasp the basics of data quality before diving deeper into solutions. A good rule of thumb is to remember that data is like a stagnant pond; if not regularly cleaned and monitored, it turns murky, making it hard to see the valuable insights lurking beneath the surface.
Importance of Data Quality Tools
Data quality tools are designed to ensure that the data flowing through an organization meets certain thresholds before it's utilized in decision-making processes. The relevance of these tools cannot be overstated, particularly as companies have started to reap the benefits of advanced analytics and business intelligence.
Consider the following advantages of employing data quality tools:
- Enhanced Decision Making: Reliable, high-quality data supports better business decisions, reducing the risks associated with poor assessments.
- Improved Customer Relationships: When organizations have accurate data, they can personalize customer interactions, fostering loyalty and satisfaction.
- Cost Savings: By avoiding costly mistakes that arise from subpar data, businesses can save significant resources.
- Regulatory Compliance: Many industries have stringent data regulations; maintaining high data quality can help ensure compliance and avoid penalties.
"Quality data is not just a nice-to-have; it is a business necessity that underpins successful analytics and insights."
Criteria for Evaluation
When navigating the crowded landscape of data quality tools, understanding the criteria for evaluation is paramount. The right criteria provide a roadmap for decision-makers, ensuring they don’t get lost in the sea of options. Evaluating these tools requires considering various elements that impact their effectiveness, usability, and overall ROI. The benefits of clearly defined evaluation criteria include minimizing risk, maximizing data integrity, and streamlining the selection process. A meticulous approach saves time and resources, ultimately leading to better outcomes and improved data governance.
Features to Look For
A critical aspect of evaluating data quality tools is identifying features that genuinely address specific organizational needs. Each feature plays a role in enhancing data management capabilities, and understanding these can shape effective choices. Here’s a closer look at essential elements to consider:
Data Profiling
Data profiling is one of the foundations of effective data management. It involves assessing data for its accuracy, completeness, and consistency. This practice helps identify data anomalies that could lead to poor decision-making. A key characteristic of data profiling is its ability to provide a comprehensive overview of datasets using statistical analysis and data patterns. It's increasingly seen as a beneficial choice because organizations can pinpoint errors early in the process. Moreover, this unique feature allows for proactive interventions, potentially saving costs and improving trust in the data. However, one disadvantage lies in the fact that profiling can be time-consuming, especially for large datasets, necessitating a balance between thoroughness and speed.
Data Cleansing
Data cleansing refers to the process of correcting or removing inaccurate, incomplete, or incorrect records from a dataset. This process is vital, as poor-quality data can skew analyses and misguide decisions. One of the notable aspects of data cleansing is its transformative potential. It enhances data quality, ensuring that the insights drawn are reliable and actionable. This is crucial for businesses looking to maintain competitive advantages. However, the cleansing process can be challenging, especially when it involves complex transformations or datasets that require extensive reworking.
Data Matching
Data matching is the practice of comparing datasets in order to identify relationships, duplicates, or similarities among them. Its contribution to data quality is significant as it supports data accuracy and consistency across various sources. A distinctive feature of data matching is its ability to merge records, thus providing a unified view of data from different databases. This can be a popular choice, especially when organizations grapple with siloed data systems. Nevertheless, a disadvantage could be the potential for mismatches if criteria used are too broad, leading to inaccurate conclusions or lost records.
Monitoring and Reporting
Monitoring and reporting tools are essential for maintaining ongoing data quality. They enable organizations to track changes, identify issues in real-time, and evaluate data trends over time. A key characteristic is their ability to provide actionable insights that are vital for informed decision-making. Monitoring can also help in spotting recurrent issues and areas for improvement, reinforcing the proactive aspect of data governance. However, some tools can produce overwhelming amounts of data, making it challenging to sift through and identify what's truly important.
Cost-Benefit Analysis
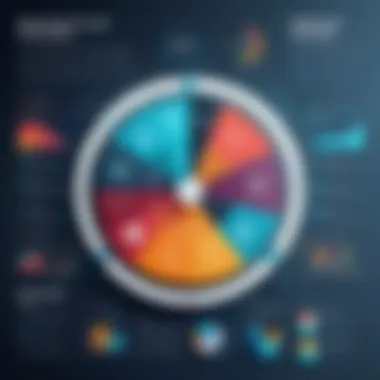
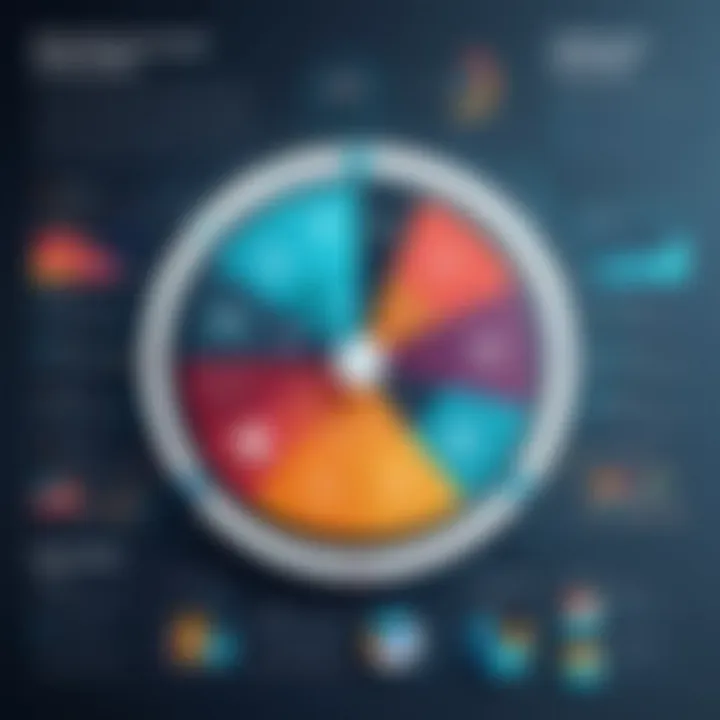
A rigorous cost-benefit analysis aids organizations in weighing the financial implications against the expected advantages of each tool. This analysis ensures that money is not wasted on features that do not align with organizational needs or goals. Factors to consider include:
- Licensing fees
- Implementation costs
- Potential to reduce errors and enhance efficiency
- Long-term savings generated from better decision-making
Each of these factors can strongly influence the final decision, emphasizing the need for a comprehensive examination of costs versus expected outcomes.
User Experience and Interface
The user experience plays a significant role in how efficiently teams can utilize data quality tools. An intuitive interface encourages engagement and reduces the learning curve for users. When evaluating user experience, the following aspects are crucial:
- Ease of navigation: Users should be able to find what they need without arduous searching.
- Customization options: Flexibility to tailor the experience can greatly enhance usability.
- Feedback mechanisms: A system that provides users with prompt feedback helps improve data entry practices.
Overall, a well-designed user interface not only makes data quality tools more accessible but also promotes widespread adoption within teams, encouraging consistent use and effective data management.
Overview of Prominent Data Quality Tools
The landscape of data quality tools is as diverse and complex as the data they seek to manage. It’s essential to get an understanding of the various tools available, as they offer unique sets of features aimed at improving data accuracy, enhancing integrity, and facilitating insightful decision-making. When making a recommendation in data management, examining the prominent tools in the field provides insight on effective choices that businesses can leverage.
Tool A: Features and Capabilities
Use Cases
Data quality tools essentially serve various use cases depending on the industry context. For example, a finance company might utilize a specific tool to ensure compliance with regulatory standards. The financial data must be precise; even a small error can lead to regulatory fines or lost trust among consumers. Tools like Talend come into play, as they enable seamless integration with existing systems while performing robust data cleansing. This adaptability makes it a vital choice for sectors that require high standards of accuracy and real-time data processing.
Strengths
Among the strengths of such tools is their flexibility. Take Informatica as an exemplary tool; its capability to adapt to various data architectures is unmatched. Also, it provides extensive support for cloud-based solutions, making it appealing for organizations transitioning to cloud environments. This flexibility grants organizations the power to manage multiple data formats without breaking a sweat, streamlining data workflows efficiently.
Weaknesses
However, it’s worth noting the weaknesses too. For instance, SAS Data Quality may sometimes come across as complex for users who are not technically savvy. The steep learning curve could hinder its adoption among smaller teams or businesses that lack extensive IT resources. Even though its depth of features can be a boon, it could also be an obstacle if the user interface is not intuitive enough.
Tool B: Features and Capabilities
Use Cases
Next, looking at Microsoft Azure Data Quality Services, one can see its robust use cases, integrating seamlessly with Microsoft’s ecosystem. This is particularly advantageous for businesses that already utilize Microsoft products, as the integration process is a breeze. Companies benefit from automated tasks that ensure data accuracy, enhancing operational efficiency.
Strengths
The core strength of Azure lies in its cloud scalability. Organizations can adjust their data handling capability as their needs grow rather than investing heavily upfront. This scalable solution is appealing to startups and SMEs that may experience fluctuating data demands but still require reliable quality management tools.
Weaknesses
Yet, not everything shines bright here. Microsoft Azure is sometimes criticized for its cost. While startups might be drawn to the support and scalability, the pricing structure can become a burden as usage scales up. This unpredictability in budgeting can deter organizations from fully committing to the platform.
Tool C: Features and Capabilities
Use Cases
Finally, there’s IBM InfoSphere QualityStage, which excels in environments that demand robust data matching and deduplication capabilities. It serves clients across various sectors, proving particularly useful in healthcare, where duplicate records can lead to severe consequences. Companies can rely on this tool to ensure patient records remain accurate and consolidated.
Strengths
Strengthwise, QualityStage boasts its advanced analytics features, allowing businesses to glean insights from their data patterns. By harnessing predictive analytics, organizations can forecast trends and make data-driven decisions confidently. The ability to identify potential issues before they arise can provide significant competitive advantages.
Weaknesses
On the flip side, one must address its integration challenges. Some users find compatibility issues with legacy systems when implementing InfoSphere, which can lead to increased operational time or even data loss during transitions. This integration hiccup can deter some organizations, particularly those with longstanding systems unwilling to undergo major overhauls.
"Choosing the right data quality tool is foundational in establishing a solid data management strategy that can support business goals effectively."
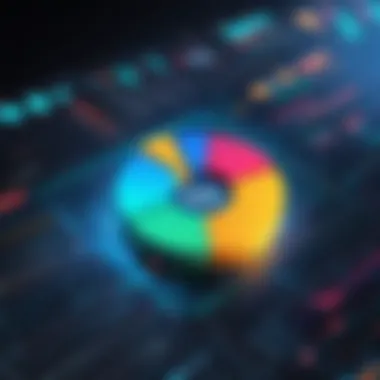
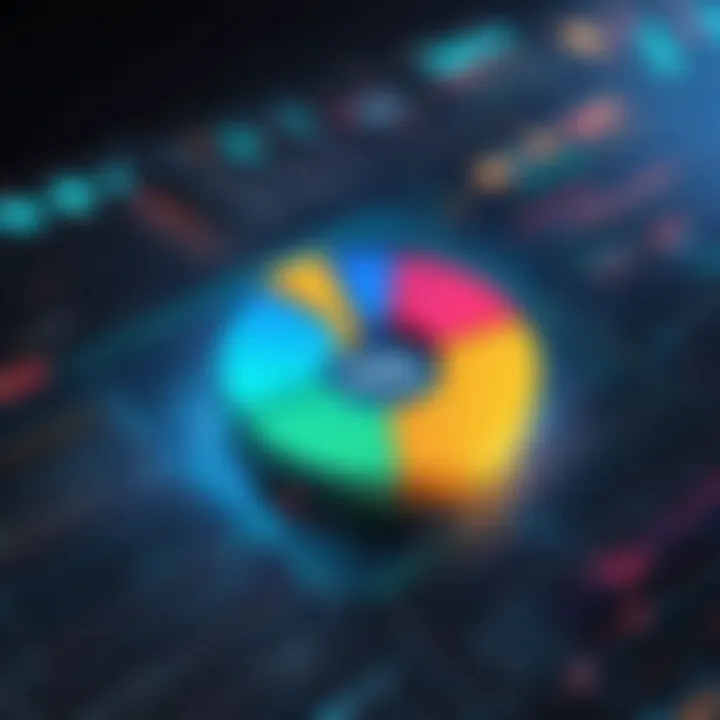
Case Studies: Tools in Action
Understanding how data quality tools function in real-world scenarios sheds light on their practical applications and effectiveness. The section of case studies presents concrete examples that showcase both successful implementations and the challenges organizations faced. Exploring these case studies can help decision-makers grasp the tangible benefits data quality tools can offer, illustrating their roles in improving data integrity and facilitating informed decision-making.
"Real-world applications of data tools reveal their strengths and weaknesses, providing valuable lessons for future implementations."
Case Study One: Implementation Success
Context and Challenges
In this case study, we examine a mid-sized retail organization that sought to enhance its customer data management. The company grappled with fragmented data across multiple systems, leading to inefficiencies and inaccuracies. The key characteristic of this situation was its urgent need for a centralized and reliable data source. This case serves as a beneficial choice for this article as it aligns with the common issues many businesses face today.
The unique feature in this scenario was the complexity of integrating data from various legacy systems into a single, cohesive format. The advantages of addressing this challenge through a data quality tool included improved customer insights and targeted marketing strategies, while the disadvantages involved the initial resource and time investments required for a successful implementation.
Results Achieved
Following the adoption of a leading data quality tool, the retail organization saw notable advancements in both operational efficiency and data accuracy. One prominent characteristic of the results was a significant reduction in data discrepancies, which ultimately fostered stronger customer relationships. This outcome serves as a popular choice for showcasing the effectiveness of data quality tools.
The unique feature in these results included the ability of the tool to provide real-time data validation, ensuring critical decisions were made based on accurate information. The advantages of these achievements included increased sales and improved customer satisfaction, while the disadvantages revolved around the ongoing need for staff training and support.
Lessons Learned
The implementation success tale highlights crucial lessons pertaining to the importance of stakeholder engagement and continuous improvement in data quality efforts. A key takeaway here is how active involvement of all departments, especially IT and marketing, contributed to a shared understanding of the data's role in driving business value. This aspect serves as a beneficial choice as it emphasizes collaboration in achieving data quality objectives.
The unique feature of lessons learned points to establishing a culture of data accountability within the organization. The advantages include fostering an environment where employees prioritize data integrity, while the disadvantages may encompass the initial resistance to change that can occur when trying to shift mindsets.
Case Study Two: Overcoming Obstacles
Initial Challenges
In another scenario, a healthcare provider faced significant hurdles in data management due to stringent regulatory requirements and the sensitive nature of patient information. The primary characteristic of these initial challenges revolved around the need to maintain compliance while ensuring the accuracy of patient records. This presents a beneficial choice in illustrating the complications that can arise in data-heavy industries.
The unique feature here is the delicate balance organizations must strike between protecting patient privacy and managing comprehensive datasets. The advantages of overcoming these challenges included enhanced operational practices and reduced compliance risks, while the disadvantages involved navigating a labyrinth of policy constraints that could complicate data quality efforts.
Strategic Adaptations
To navigate these initial challenges, the healthcare provider adopted a phased approach to implementing a data quality tool. Strategic adaptations included selecting a vendor experienced in healthcare data solutions and tailoring the tool for specific regulatory needs. The notable characteristic of this adaptation was agility; the organization was able to pivot quickly in response to evolving requirements, which sets it as a beneficial choice in this context.
A unique feature of these adaptations was the thorough training provided to staff on compliance and data management practices, leading to better data stewardship across departments. The advantages included more cohesive team efforts, while the disadvantages highlighted the resource drain associated with ongoing training efforts.
Outcomes
Ultimately, the healthcare provider's targeted approach led to significant enhancements in data quality and compliance adherence. A key characteristic of the outcomes was the marked decrease in audit findings and a boost in operational efficiency. This powerful transformation showcases a popular choice for organizations in similar sectors looking to strengthen their data quality frameworks.
The unique feature of these successful outcomes included developing a comprehensive understanding of how data quality directly impacts patient care and safety. This led to advantages such as improved patient outcomes and reduced operational risks, whereas the perpetual disadvantages entailed the need for ongoing assessments and tool updates to maintain momentum.
Integration with Existing Systems
The integration of data quality tools with existing systems cannot be overstated. It acts as the linchpin that ensures data integrity across operations, forming a seamless connection between various data sources and platforms. When organizations invest in data quality solutions, they aim not solely to clean their data but to embed these tools into their operational fabric. This kind of synergy is essential, as it facilitates effective data governance and empowers decision-making with accurate information.
One of the primary benefits of integration is its ability to streamline workflows. By automating data cleansing and profiling directly within the existing software stack, businesses can reduce the manual overhead involved in data management tasks. This results in not only improved efficiency but also a significant cut in human errors that often plague data entry processes. Furthermore, having a unified data management strategy allows organizations to maintain consistent definitions and standards across all departments.
However, several considerations must be taken into account when prioritizing integration capabilities. Companies should reflect on their current IT infrastructure, scalability, and the potential for future growth. The decision to add a data quality tool also brings with it the challenge of ensuring that the tool can align beautifully with existing technologies without requiring a complete overhaul or causing disruptions.
Ultimately, a thoughtful integration strategy can serve as a force multiplier for data quality initiatives, ensuring that businesses do not simply operate with better data in silos but rather leverage it holistically across their operations.
Compatibility Concerns
When integrating data quality tools, compatibility is where the rubber meets the road. If these tools can't easily communicate with existing databases, applications, and systems, the whole effort may just go up in smoke. Compatibility issues can arise from a variety of sources, including differences in data formats, APIs, and even the underlying architecture of the systems involved.
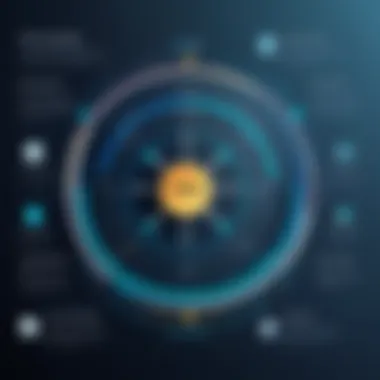
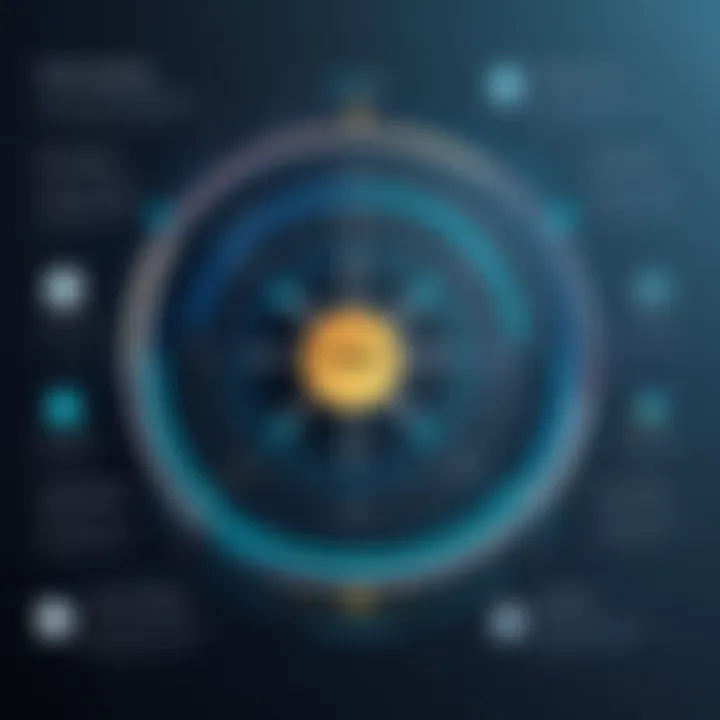
Organizations must carefully evaluate the following aspects:
- Data Formats: Data might be structured in different formats across systems. Ensuring that the new tool can work with existing formats is vital.
- API Support: An effective data quality tool should provide robust API integration to facilitate seamless data exchange and communication.
- Legacy Systems: Many businesses have legacy systems housing crucial data. These systems may not support newer tools unless proper bridges or middleware are developed.
Neglecting any of these compatibility concerns could lead to inconsistent data, which ultimately undermines the objectives of employing a quality tool in the first place. In turn, organizations may find themselves staring at an unnecessary budget crunch, not to mention wasted time.
Data Migration Strategies
Following compatibility analysis, the next logical step is establishing effective data migration strategies. Successful data migration is not just about transferring data; it’s also about ensuring that data quality is maintained throughout the process. The following strategies can mitigate common pitfalls:
- Assess and Cleanse Before Migration: It is sensible to clean data before migration. Any inaccuracies should be rectified beforehand to prevent the transfer of bad data into the new system.
- Phased Migration: Instead of evolving everything in one go, a phased approach allows for testing and debugging at each stage. This limits risks significantly while making it easier to manage changes without overwhelming the team.
- Data Backup: Always have a fallback plan. Regular backups before migration prevent data loss should anything go south during the process. It also provides peace of mind.
- Employee Training: Ensure teams involved in migration are trained adequately on the new systems and data handling procedures. Knowledge and comfort with new technologies reduce errors and speed up the adaptation process.
Adopting these strategies results in higher success rates for data migrations and helps establish a solid foundation for embedding data quality tools in operational workflows.
"Integration without consideration is like building a house on sand. You need a solid foundation for success."
Future Trends in Data Quality Tools
In an era where data drives decision-making, understanding the future trends in data quality tools becomes paramount for businesses striving to maintain a competitive edge. The evolution of these tools mirrors the shifting landscape of technology and data processing practices, ensuring they remain relevant and effective. By anticipating these trends, companies can prepare to adapt their data management strategies. In this section, we explore how emerging technologies and anticipated market changes will shape data quality.
Emerging Technologies
Artificial Intelligence
Artificial Intelligence (AI) stands out as a revolutionary force in the realm of data quality. Its ability to learn from vast amounts of information and identify patterns enhances accuracy in data processing. A key characteristic of AI is its capacity for automation—something that helps reduce the time and effort required for data quality tasks.
One unique feature of AI in data quality tools is its predictive analytics capability. This allows organizations to foresee potential data issues before they arise. For instance, AI algorithms can analyze historical data trends to reveal anomalies and alert users proactively. However, the downside lies in the complexity of implementation, requiring skilled personnel to manage and tune AI systems effectively.
Machine Learning
Machine Learning (ML) contributes significantly to the landscape of data quality by offering advanced analytical abilities that constantly improve over time. A noteworthy aspect of ML is its adaptability; it can seamlessly adjust to new datasets and learn from them. This feature makes it a compelling choice for firms looking to enhance data integrity.
The unique strength of machine learning is its ability to handle large datasets with multiple variables, making it easier to cleanse and normalize data effectively. However, the reliance on historical data for training can lead to inappropriate conclusions if past patterns do not hold. Therefore, businesses need to cautiously evaluate the application of ML in relation to their specific data contexts.
Blockchain
Blockchain technology has emerged as a valuable asset in ensuring data quality through its decentralized and immutable nature. With its ability to create transparent records of transactions, blockchain offers an unprecedented level of security and traceability. A standout characteristic of blockchain is that it minimizes the risk of data tampering, a common concern for organizations.
The unique feature of blockchain in data quality tools is its consensus mechanism, which assures all parties involved in a transaction that the data is valid and resistant to alteration. Although there are significant benefits, the complexity of blockchain integration into existing systems can pose a challenge. Moreover, necessary changes in organizational culture and processes for adoption might slow down its implementation.
Anticipated Market Changes
The data quality tools market is on the brink of transformative changes driven by the rapid advancements in technology and shifting business needs. One of the primary anticipated changes is the growing focus on interoperability among different data quality tools. As organizations often utilize a mix of systems, the ability for these tools to work seamlessly together becomes critical.
In addition, the increasing regulatory scrutiny surrounding data protection and privacy will demand more robust data quality solutions. Companies must adapt to comply with regulations, which may push them to invest in tools that not only maintain data integrity but also ensure security and compliance.
As data landscapes evolve, so must the tools that shape them. Embracing emerging technologies while staying vigilant about market shifts will help organizations navigate the complexities of data quality effectively.
Overall, staying ahead of these trends is not just advantageous; it is essential for businesses aiming to sustain operational excellence and thrive in a data-driven world.
Finale and Recommendations
As the data landscape becomes increasingly complex, the significance of choosing the right data quality tools cannot be overstated. The insights provided in this article lay a foundation for effectively navigating these options, ensuring that businesses are equipped to make informed decisions about data integrity and utility. Ultimately, these decisions not only affect data management strategies but also influence overall business outcomes and customer satisfaction.
Summary of Findings
Throughout the exploration of various data quality tools, several key points emerge that deserve attention:
- Features and Functionality: Each tool brings a unique set of features tailored to specific organizational needs. From data profiling to monitoring metrics, distinguishing these functionalities is crucial in aligning tools with business goals.
- Market Trends: Staying attuned to trends such as artificial intelligence and machine learning integration can offer leverage in optimizing data management processes.
- Real-World Impact: Case studies reinforce that effective implementation can lead to tangible improvements in data accuracy and operational efficiency, and consequently boost the bottom line.
These findings advocate for a thorough evaluation process that includes assessing technical requirements, cost considerations, and long-term scalability to ensure that the chosen tools provide the most value.
Guidance for Decision Makers
Making decisions regarding data quality tools demands a strategic approach combined with an understanding of the organization’s specific requirements. Here are some recommendations for decision makers:
- Define Requirements Clearly: Before diving into the selection process, it is essential to pinpoint what data quality challenges the organization faces. Whether it’s data cleansing or matching, understanding these needs is pivotal.
- Involve Cross-Functional Teams: Engaging various stakeholders can provide a holistic view of needs that might be overlooked by a singular viewpoint. Input from IT, marketing, and compliance can illuminate differing perspectives on essential features.
- Assess Flexibility and Integration: As future needs evolve, tools that offer flexibility and compatibility with existing systems are more advantageous. Ensure the selected tools can effectively integrate into current data ecosystems.
- Cost-Benefit Analysis: Beyond the mere acquisition costs, conduct a detailed analysis of potential financial and operational impacts. Even the priciest tool might be economical in the long run if it significantly improves data quality and decision-making efficiency.
- Prioritize Training and Support: Implementing new tools is only half the battle; adequate training and support are crucial for successful adoption within teams.