Data Strategy Approach for Informed Decision-Making
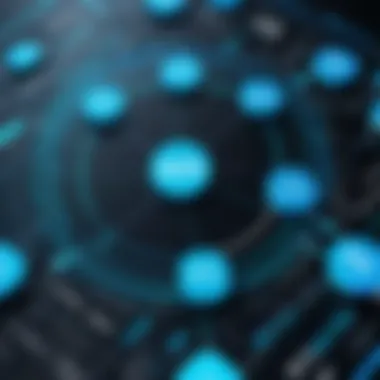
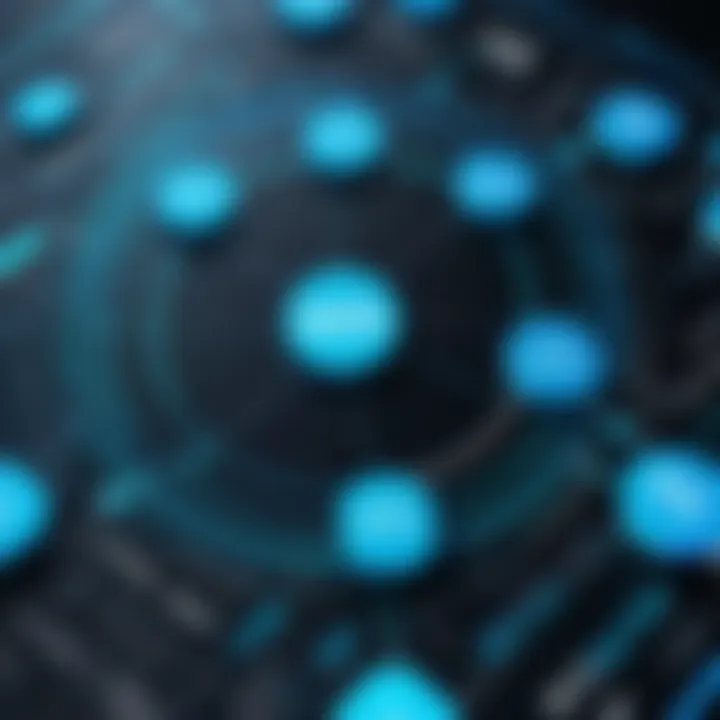
Intro
In today's fast-paced digital environment, businesses face the growing need to develop a robust data strategy. This is essential for managing vast amounts of information effectively. With technology evolving constantly, aligning data initiatives with organizational goals has become a priority for many organizations. Companies that successfully navigate these complexities often gain a competitive edge in the market. As they delve into their data assets, understanding how to leverage these resources for informed decisions is critical.
A well-formulated data strategy serves as a roadmap. It guides organizations in making data-driven decisions, optimizing their operations, and enhancing customer experiences. Key elements include data governance, quality management, and analytics capabilities. These components shape the overall data landscape within a business.
Technological Research Overview
Recent Technological Innovations
The rapid pace of technological innovation has transformed the way businesses operate. Cloud computing and big data technologies play a significant role. These innovations allow companies to store and process vast amounts of data efficiently. Additionally, advancements in machine learning have enabled businesses to analyze data more effectively, drawing insights that inform strategic decisions.
Impact on Business Operations
These technological advancements have had a profound impact on business operations. They facilitate automation, reduce operational costs, and improve efficiency. Companies can now make quicker decisions based on real-time data analysis. Organizations that embrace these changes can adapt rapidly to market fluctuations and customer demands, ensuring sustained growth and competitiveness.
Future Technological Trends
Looking ahead, several trends are likely to define the technological landscape. The integration of artificial intelligence in data management stands out. This can lead to predictive analytics, enabling businesses to anticipate market changes. Furthermore, the focus on data privacy and security will intensify as regulatory pressures mount. Companies must be prepared to adapt to these trends to maintain relevance and compliance.
Data Analytics in Business
Importance of Data Analytics
Data analytics has emerged as a cornerstone for modern organizations. It allows companies to retrieve actionable insights from their data, driving informed decision-making. By analyzing patterns and trends, businesses can fine-tune their strategies to meet customer needs more effectively.
Tools for Data Analysis
Several tools are available for organizations seeking to implement data analytics. Popular options include:
- Tableau: Known for its visualization capabilities, it helps users interpret complex data sets.
- Microsoft Power BI: Offers a comprehensive suite of tools for data analysis and visualization.
- Google Analytics: Essential for businesses seeking to understand their online performance and customer behaviors.
- R and Python: Powerful programming languages used for statistical analysis and advanced data manipulation.
Case Studies on Data-Driven Decisions
Numerous organizations have successfully applied data analytics to enhance decision-making. For instance, Netflix employs sophisticated algorithms to recommend content. By analyzing viewer patterns, Netflix has optimized user engagement and retention. Similarly, retailers like Amazon leverage data to customize user experiences, suggesting products based on past purchases.
Cybersecurity Insights
Threat Landscape Analysis
As data becomes increasingly integral to business operations, the threat landscape also evolves. Cybersecurity breaches can have severe consequences, leading to loss of trust and financial damage. Companies must remain vigilant in understanding potential threats, including phishing attacks and ransomware.
Best Practices for Cybersecurity
To mitigate cybersecurity risks, organizations should adopt best practices such as:
- Implementing regular security audits.
- Training employees on recognizing phishing attempts.
- Employing robust encryption methods.
- Establishing incident response plans to manage breaches effectively.
Regulatory Compliance in Cybersecurity
Compliance with regulations like GDPR and CCPA has become crucial. These regulations dictate how organizations must safeguard consumer data. Companies that prioritize compliance not only protect themselves from legal repercussions but also build trust with their customers.
Artificial Intelligence Applications
AI in Business Automation
Artificial intelligence is reshaping business processes. Automation of repetitive tasks improves efficiency and allows employees to focus on higher-value activities. This technology can significantly enhance productivity and reduce operational costs.
AI Algorithms and Applications
Several AI applications are now fundamental to various industries. Common algorithms include neural networks for deep learning and decision trees for predictive modeling. These applications harness data insights to inform business strategies effectively.
Ethical Considerations in AI
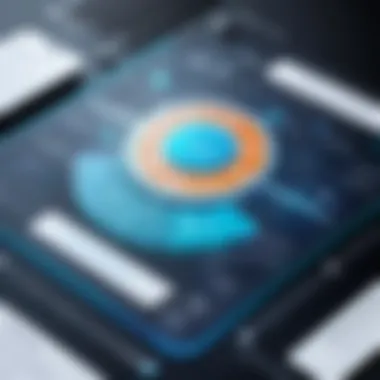
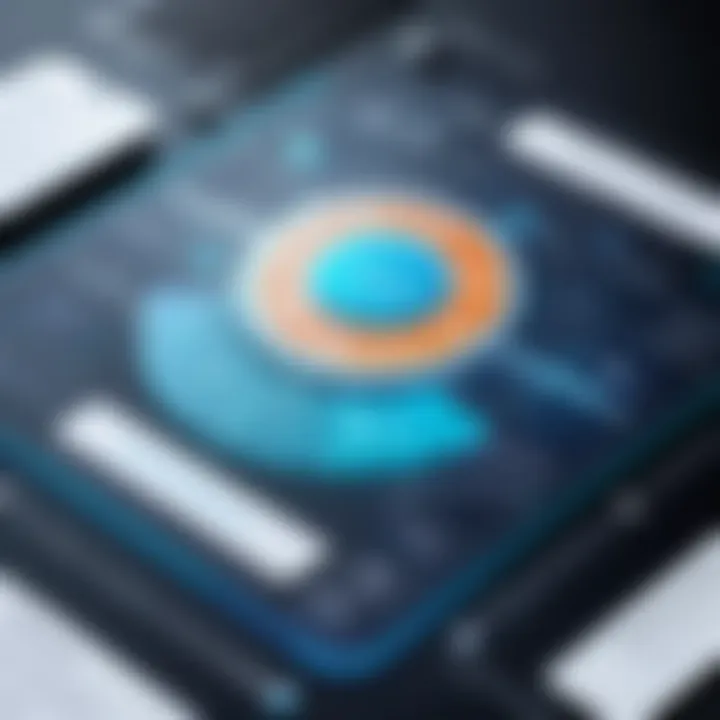
Despite its advantages, the use of AI raises ethical questions. Companies must consider the implications of biased algorithms, data privacy concerns, and transparency. Striking a balance between innovation and ethical responsibility is vital in the AI landscape.
Industry-Specific Research
Tech Research in Finance Sector
The finance sector has seen immense benefits from data strategies. Advanced analytics allows for improved risk assessment and customer personalization. Financial institutions are now better equipped to mitigate risks and offer tailored financial products.
Healthcare Technological Advancements
In healthcare, data strategy plays a crucial role in patient care optimization. Electronic health records and data analytics pave the way for enhanced treatment plans and patient management, improving overall health outcomes.
Retail Industry Tech Solutions
The retail industry continually integrates technology to enhance customer experiences. From inventory management systems to customer relationship management tools, data-driven solutions help retailers stay competitive in a crowded marketplace.
Implementing a strategic approach to data not only fosters informed decisions but also ensures long-term sustainability in a data-driven world.
Understanding Data Strategy
Understanding data strategy is essential for any organization aiming to thrive in an increasingly data-driven world. A well-structured data strategy provides a framework that aligns data management with business objectives. This alignment allows organizations to make informed decisions and stay competitive. Adopting a coherent data strategy is not just about storage or processing of data but about understanding how data can transform decision-making processes.
Definition of Data Strategy
Data strategy refers to a comprehensive plan that outlines how an organization collects, manages, and utilizes its data to achieve its goals. It encompasses various components, including data governance, data quality, architecture, and analytics. This strategic approach ensures that data is not just available, but also relevant and actionable. Organizations that define their data strategy create a structured method for integrating data into their operations, which enhances their ability to respond to market demands and internal needs. The ultimate aim is to cultivate a data-centric culture that empowers stakeholders at all levels to use data effectively.
Importance in Today's Business Environment
In the current business landscape, the relevance of a strong data strategy cannot be underestimated.
- Organizations that leverage data effectively can uncover insights that drive innovation and efficiency.
- A well-articulated data strategy fosters better decision-making by providing timely and accurate information.
- Companies can identify trends and patterns through data analytics, allowing them to adapt quickly to changing market conditions.
- With data becoming a competitive advantage, firms without a clear data strategy risk falling behind their peers.
The integration of technology has made data more abundant, yet it has also complicated the task of deriving value from it. A sound data strategy helps mitigate the risk of data overload by prioritizing relevant data and establishing clear guidelines for its use. This structured approach is critical in navigating compliance and security challenges that arise in a world where data handling regulations are becoming stricter.
Emphasizing the importance of data strategy, organizations must invest time and resources in developing a clear vision. This vision should define how data will be utilized across the business, emphasizing clarity and collaboration among teams to achieve a unified approach.
Components of a Data Strategy
A well-defined data strategy is essential for businesses looking to navigate the complexities of modern data environments. Each component plays a significant role in ensuring that data serves its purpose effectively. Understanding these components can lead to improved decision-making, operational efficiency, and competitive advantage.
Data Governance
Data governance refers to the policies, procedures, and standards that a business establishes to ensure data is managed properly. It includes defining who is responsible for data at every level. This accountability fosters trust and ensures compliance with regulations, such as GDPR or CCPA.
Effective data governance offers clarity. It helps in aligning data initiatives with business goals, leading to more informed decision-making. Furthermore, it can minimize risks associated with data mishandling. Companies often face tough choices if they neglect this aspect. When data governance is weak, data quality suffers. A structured approach to data governance ensures everyone understands their role. This clarity can enhance collaboration across departments, streamlining data processes.
Data Architecture
Data architecture is the blueprint for managing data assets. It defines the structure, storage, and flow of data within an organization. A well-designed architecture is crucial for efficient data retrieval and use. A clear architecture helps businesses manage vast volumes of information.
Creating a strong data architecture involves frameworks like data models and integration techniques. These frameworks support the organization's data needs while ensuring scalability. An appropriate architecture also enables smooth integration between various data sources, optimizing data utility and functionality. Without it, businesses risk inefficiency and fragmentation, with data scattered across silos.
Data Quality Management
The significance of data quality management cannot be overstated. It entails processes that ensure data is accurate, consistent, and reliable. Quality data is vital for generating insights that drive decision-making. Poor data quality leads to misguided decisions and wasted resources.
Implementing robust data quality management involves establishing metrics and standards for data accuracy. Regular audits and cleansing practices enhance quality and compliance. When data quality is prioritized, organizations experience not just efficiency, but also increased trust from stakeholders and clients. The goal is to create a feedback loop that continually enhances data quality.
Data Analytics Capabilities
Data analytics capabilities are the tools and skills required to extract valuable insights from raw data. The rise of big data demands sophisticated analytics techniques, which can analyze patterns, trends, and behaviors. Implementing strong analytics capabilities allows businesses to become proactive instead of reactive.
Investing in analytics can transform data into actionable insights. It enables organizations to anticipate market trends and understand consumer needs better. Leveraging tools like Tableau or Google Analytics can significantly enhance analytics capabilities.
Employing advanced analytics, such as predictive modeling or machine learning, can further unlock potential value. However, organizations need to ensure they have skilled professionals capable of harnessing these analytics tools effectively.
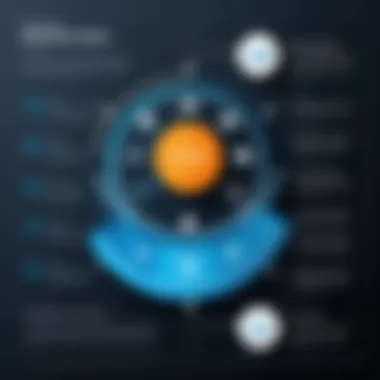
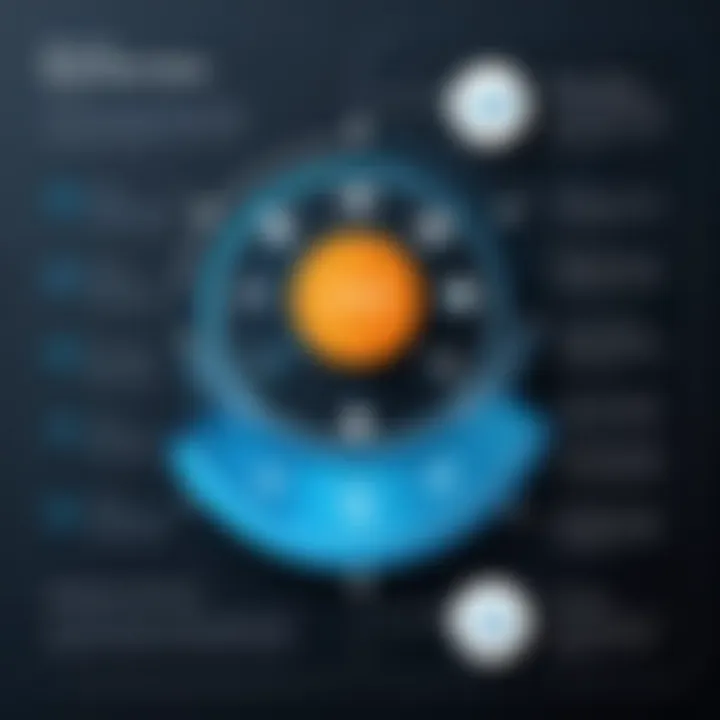
Technology Infrastructure
The technology infrastructure is the foundation upon which a data strategy is built. This includes hardware, software, and network resources essential for data storage, processing, and management. A solid technology infrastructure can enhance data accessibility and security.
As businesses collect more data, they need scalable technologies. Cloud computing offers flexibility and efficiency in managing large data sets. Integrating solutions like Microsoft Azure or Amazon Web Services can yield significant benefits. These platforms facilitate easy data sharing, support analytics, and provide robust security measures.
Establishing an adaptable technology framework allows organizations to withstand future challenges. Investing in the right tools ensures that a data strategy remains resilient and poised for growth.
Ensuring that these components are aligned fosters a comprehensive data strategy. By promoting effective data governance, robust architecture, high-quality management systems, advanced analytics capabilities, and solid technology infrastructure, organizations create a strong foundation for reaching their business objectives.
Methodologies for Implementing Data Strategy
Implementing a data strategy requires a structured approach. Methodologies are essential for ensuring that the process is systematic and effective. Organizations must adeptly navigate through multiple facets to realize the value of their data. By employing clear methodologies, businesses gain clarity and direction in their data management efforts. Key elements in this process include assessing the current data landscape, setting clear objectives, developing a roadmap for implementation, and engaging key stakeholders. These components help in maximizing the benefits of data strategy while minimizing risks.
Assessing Current Data Landscape
The first step in implementing a data strategy is to evaluate the existing data landscape. It is crucial to understand what data is available, its sources, and its quality. Organizations often have data scattered across various systems, making it essential to perform an inventory of data assets. This assessment allows businesses to identify strengths and weaknesses in their data practices. Questions to consider include:
- What types of data do we currently collect?
- Are there any gaps in the data we possess?
- How accessible is our data for analysis and decision-making?
By addressing these questions, organizations build a solid foundation for their data initiatives. This step also helps in recognizing potential compliance issues and data silos that could hinder progress.
Setting Clear Objectives
Defining clear objectives is vital for a successful data strategy. Without well-articulated goals, efforts may become disjointed. Objectives should align closely with the organization's overall business strategy. It is beneficial to apply the SMART criteria—Specific, Measurable, Achievable, Relevant, and Time-bound. For instance, a company might aim to enhance customer satisfaction by 20% over the next year through improved data-driven insights. Such clear goals provide guidance in developing data initiatives and focus the organization's resources more effectively.
Developing a Roadmap
A roadmap outlines the strategic plan for implementing data initiatives. It serves as a guide detailing the necessary steps, timelines, and resources required for execution. The development of a roadmap should involve input from multiple departments, as different areas may provide diverse insights into data needs.
When creating a roadmap, organizations should consider the following elements:
- Timeline: Establish a clear timeline for each phase.
- Resources: Identify human, technological, and financial resources.
- Milestones: Set key milestones to assess progress regularly.
Having a clear roadmap helps to prevent potential pitfalls and ensures that projects remain aligned with the overall data strategy.
Engaging Stakeholders
Engaging stakeholders throughout the implementation process is essential. This engagement fosters support and collaboration across departments. Stakeholders often include executives, data analysts, IT personnel, and even end-users. Their perspectives provide valuable insights into what is needed for successful data initiatives.
For effective engagement:
- Identify Key Stakeholders: Recognize who will be impacted by the data initiatives.
- Regular Communication: Maintain open lines of communication to update stakeholders on progress and gather feedback.
- Foster Collaboration: Encourage teamwork in developing and analyzing data projects.
In summary, the methodologies for implementing a data strategy create a structured approach that leads to informed decision-making. By assessing the current landscape, setting clear objectives, developing a detailed roadmap, and engaging stakeholders, organizations can effectively navigate the complexities of data management and governance.
"Data isn’t just about numbers. It’s about making informed decisions that can drive business success."
This quote underscores the importance of having a well-thought-out data strategy in place.
Aligning Data Strategy with Business Goals
Data strategy must not exist in a vacuum. Its alignment with business goals is essential to ensure that every data initiative serves a clear purpose. Organizations that align their data strategies with their business objectives can significantly improve operational efficiency, enhance decision-making capabilities, and drive sustainable growth. The harmonization of these two elements creates a framework where data becomes an asset rather than merely a byproduct of business processes. Therefore, establishing this alignment is not just beneficial but necessary for modern enterprises.
Understanding Business Objectives
To effectively align data strategy with business goals, the initial step is to comprehend what those goals are. Business objectives can range from expanding market share to enhancing customer satisfaction or improving operational efficiency. Understanding these goals involves engaging with various stakeholders throughout the organization.
Some key actions to consider include:
- Stakeholder Engagement: Talk to department heads and team leaders to understand their priorities.
- Market Analysis: Assess the external environment and competitor strategies to define your organization's positioning.
- SWOT Analysis: Identify strengths, weaknesses, opportunities, and threats that may influence business goals.
By accumulating this information, an organization can develop a clear picture of where it wants to go, which inform the necessary data initiatives. This understanding is an essential precursor to creating effective data strategies.
Translating Objectives into Data Initiatives
Once business objectives are clear, the next step is translating these goals into actionable data initiatives. This translation requires a strategic approach, ensuring that data efforts directly support organizational aims.
To facilitate this translation, consider the following:
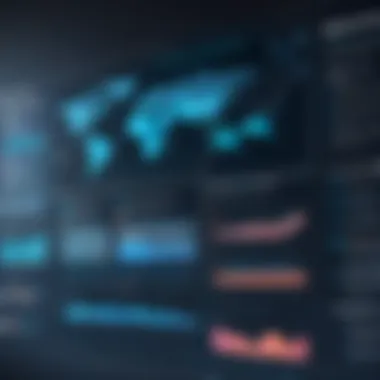
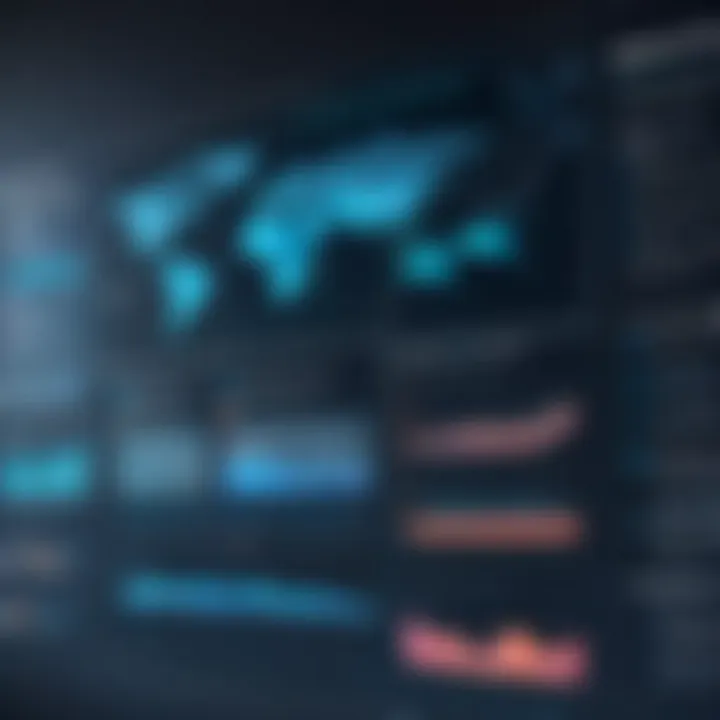
- Mapping Data to Goals: Determine how specific data points relate to each objective. For example, if customer satisfaction is a goal, focus on customer feedback and journey data.
- Prioritization of Initiatives: Identify which data initiatives can deliver the most significant impact based on the established objectives. Not all data is equally valuable; prioritization helps allocate resources effectively.
- Establishment of KPIs: Set key performance indicators specific to each initiative to monitor progress toward business objectives. Without these metrics, evaluating the success of data initiatives becomes challenging.
"Aligning your data strategy to business goals transforms data from a mere tool to a pivotal driver of organizational success."
Challenges in Data Strategy Implementation
Implementing a data strategy is fraught with numerous challenges that can undermine the effectiveness of the overall approach. These challenges need to be recognized and addressed to ensure that data is leveraged effectively for informed decision-making. Several focal points exist here, such as data silos, compliance issues, and skill gaps in the workforce. Not only do these elements inhibit progress, but they can also lead to missed opportunities.
Data Silos
One of the most significant barriers to a successful data strategy is the presence of data silos. These occur when data is isolated in different departments or systems, preventing easy access and integration. Data silos not only fragment valuable information but also limit collaborative efforts within an organization. The disconnection can lead to inconsistent insights and hinder the ability to make well-informed decisions. To mitigate this, businesses may need to invest in centralized data management systems that promote accessibility and encourage cross-departmental communication. Identifying areas where silos exist is essential for creating transparency.
"Data silos present a serious risk to the integrity of decision-making processes within organizations."
Compliance Issues
The complexity of regulatory compliance cannot be overstated, and it poses a substantial challenge in the implementation of data strategies. Organizations must navigate various laws and regulations regarding data protection, such as the General Data Protection Regulation (GDPR) or the California Consumer Privacy Act (CCPA). Non-compliance can lead to hefty fines and damage to an organization’s reputation. Hence, integrating compliance measures into the data strategy from the outset is vital. Regular audits and trainings on data regulations can help ensure that all stakeholders understand their responsibilities. Moreover, establishing clear protocols for data handling will aid companies in avoiding legal pitfalls.
Skill Gaps in the Workforce
Another noteworthy hurdle is the skill gaps within the workforce. As data strategy becomes more complex, the demand for skilled professionals grows. Many organizations may find it challenging to fill these positions, especially as technology evolves rapidly. This skill gap can affect the ability to analyze data effectively and translate findings into actionable strategies. To combat this, companies must prioritize continuous learning and professional development. Investing in training programs or collaborating with educational institutions can help bridge skill deficiencies and create a workforce adept at handling the evolving data landscape.
In summary, confronting the challenges in data strategy implementation is critical for any organization looking to leverage data effectively. By addressing data silos, compliance requirements, and workforce skill gaps, companies can create a robust framework that supports data-driven decision-making.
Evaluating the Success of Data Strategy
Assessing the effectiveness of a data strategy is crucial for sustained growth and informed decision-making. Without thorough evaluation, organizations risk investing in initiatives that do not align with their goals or yield the anticipated value. This section delves into methods of evaluating success, focusing on specific elements like Key Performance Indicators and feedback mechanisms, which together create a framework for measuring performance.
Key Performance Indicators (KPIs)
Key Performance Indicators are quantifiable measures that help track an organization's performance against its strategic objectives. Defining appropriate KPIs is critical for determining if a data strategy is meeting its goals. Here are some important KPIs to consider:
- Data Usage Rates: Monitoring how often data is accessed by different teams can reveal whether employees leverage data effectively.
- Data Quality Scores: Assessing the accuracy and consistency of the data collected is essential. Low-quality data can lead to misguided decisions.
- User Satisfaction Levels: Gathering feedback from users about data tools and resources can highlight areas for improvement in data accessibility and usability.
- Cost Savings Achieved: Tracking savings due to efficiencies gained from data-driven decision-making can illustrate the tangible impact of a data strategy.
The selection of KPIs should relate directly to the goals set during the planning phase of the data strategy. Aligning these indicators with organizational objectives ensures that the evaluation is relevant and useful.
Feedback Mechanisms
Feedback mechanisms are an integral part of evaluating a data strategy's success. They provide insight into how stakeholders interact with data initiatives and can reveal opportunities for improvement. Key considerations for implementing effective feedback mechanisms include:
- Regular Surveys: Surveys can gather input from various stakeholders regarding their experiences with data tools and processes. It's important to ask clear questions that yield actionable insights.
- Focus Groups: Engaging small groups of users in structured discussions can uncover deeper insights into their needs and challenges related to data usage.
- Performance Reviews: Including data-related performance metrics in employee reviews can motivate teams to engage with data more actively and responsibly.
- Iteration Based on Feedback: The data strategy should not be static. Adjustments based on feedback ensure the initiative continues to evolve and meet changing needs.
"Success is not just about having a data strategy in place; it is about continuously refining it through feedback and measurable outcomes."
Future Trends in Data Strategy
In the ever-evolving landscape of technology, staying ahead of future trends in data strategy is essential for businesses. The integration of modern technologies and evolving user expectations can significantly impact decision-making processes. Understanding these trends enables organizations to leverage data effectively, leading to enhanced decision-making and competitive advantage. As we explore this section, we will focus on two key trends: the integration of AI and machine learning, and an increased emphasis on data ethics.
Integration of AI and Machine Learning
The integration of Artificial Intelligence (AI) and Machine Learning (ML) into data strategies is not just a trend; it is becoming a necessity. Companies that adopt these technologies can analyze vast amounts of data at a speed and accuracy unattainable by human capabilities alone. AI and ML drive automation, predictive analytics, and improved data processing efficiencies.
- Benefits:
- Enhanced data insights through more complex algorithms.
- Better customer experiences by tailoring services based on data-driven understanding.
- Increased operational efficiency as routine tasks are automated.
Organizations should consider the following when integrating AI and machine learning:
- Assessing their existing data quality and infrastructure.
- Ensuring alignment with business goals for maximum impact.
- Training staff on new technologies to bridge skill gaps.
"Integrating AI in strategy not only transforms data handling but also reshapes business models and industry dynamics."
Emphasis on Data Ethics
As organizations rely more on data, the importance of data ethics becomes apparent. Ethical considerations surrounding data collection and use are essential in building trust with clients and consumers. Data strategies must ensure compliance with regulations such as GDPR and CCPA, while also fostering a culture of ethical data use. This includes being transparent about data collection practices and utilizing data responsibly.
- Considerations for ethical data use:
- Implementing robust data governance frameworks.
- Regularly assessing compliance with legal and ethical standards.
- Promoting a culture of responsibility among employees regarding data handling.
Organizations focusing on ethical data practices not only mitigate risks but also enhance their image in the eyes of customers and stakeholders. As businesses acknowledge the significance of data ethics, it can lead to stronger, trust-based relationships, and ultimately a more sustainable business model.
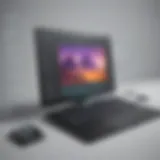
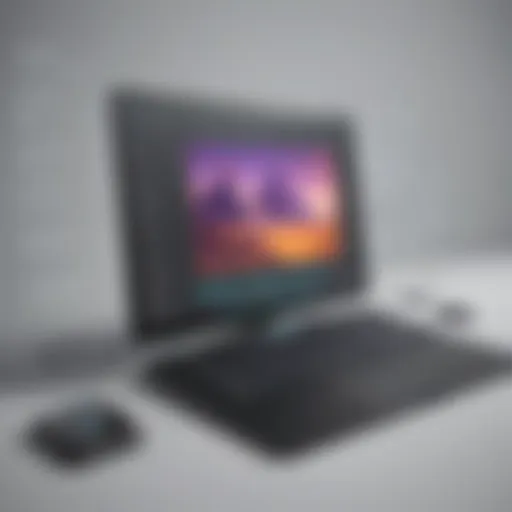