Crafting an Effective Data Quality Plan
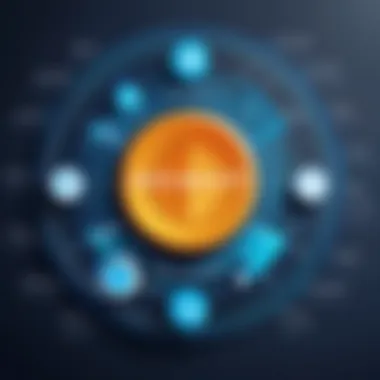
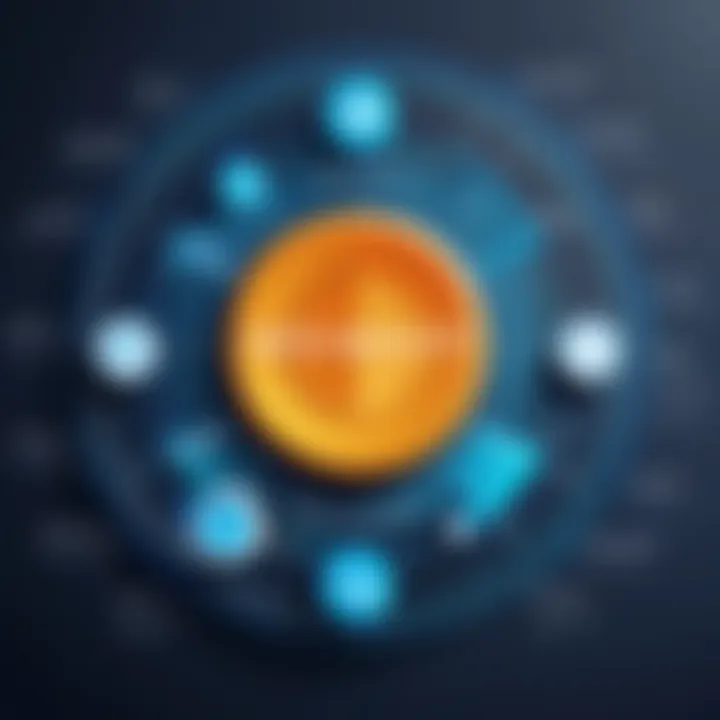
Intro
In the digital age, data is a crucial asset for organizations across all sectors. The ability to harness this data effectively can significantly transform decision-making processes, customer engagement, and operational efficiency. However, the integrity of data often remains compromised due to various issues. Therefore, establishing a solid data quality plan is essential. This article explores the creation of an effective data quality plan, outlining essential components, metrics, and methodologies that ensure data remains accurate and reliable.
Technological Research Overview
Technology has evolved rapidly, impacting the way businesses manage their data. Organizations must stay current with technological innovations to enhance their data quality practices.
Recent Technological Innovations
Recent advancements in big data analytics, cloud computing, and machine learning can help organizations elevate their data quality frameworks. These technologies provide robust tools for gathering, processing, and analyzing large datasets efficiently. Solutions such as Tableau and Microsoft Power BI facilitate data visualization, allowing for more informed decision-making.
Impact on Business Operations
The integration of modern technologies influences business operations. For instance, automated data cleansing tools can reduce human error significantly. By minimizing inaccuracies at the source, organizations can save time and improve overall data reliability. Furthermore, cloud solutions enhance accessibility, allowing stakeholders to access data in real-time, which is critical for agile decision-making.
Future Technological Trends
Looking ahead, several trends will shape the data quality landscape. The adoption of artificial intelligence will likely automate many aspects of data quality assurance. Moreover, the implementation of blockchain technology can enhance data integrity by providing a secure, immutable record of transactions. Organizations must prepare to embrace these changes to stay competitive.
Data Analytics in Business
The importance of data analytics cannot be overstated. As businesses generate more data than ever, the capacity to analyze this information effectively can provide a significant strategic advantage.
Importance of Data Analytics
Data analytics enables organizations to uncover insights that drive informed decision-making. By identifying trends and patterns, businesses can tailor their strategies to meet evolving market demands. Analytics also helps in understanding customer behavior, which can lead to improved service offerings.
Tools for Data Analysis
Numerous tools are available for data analysis. Platforms like IBM Watson Analytics and Google Analytics equip businesses with the capabilities to interpret large datasets efficiently. These tools often come with user-friendly interfaces and extensive support, making them accessible for professionals with varying levels of expertise.
Case Studies on Data-Driven Decisions
Consider the example of Netflix, which leverages data analytics to optimize content recommendations. By analyzing viewer preferences and behaviors, the company continually refines its offerings, keeping customer satisfaction high. Such case studies underscore the importance of data-driven decisions in enhancing customer experience and improving retention rates.
Cybersecurity Insights
As the dependence on data grows, so does the need for cybersecurity. Organizations must ensure that their data quality efforts do not neglect security protocols.
Threat Landscape Analysis
The current threat landscape reveals several vulnerabilities that could compromise data integrity. Cyber threats such as phishing attacks, ransomware, and data breaches pose significant risks. Ongoing awareness of these threats is vital to protect organizational data.
Best Practices for Cybersecurity
To mitigate these risks, businesses should implement best practices, including regular software updates, employee training, and robust access controls. Furthermore, applying encryption methods ensures sensitive data remains protected even if compromised.
Regulatory Compliance in Cybersecurity
Organizations must also stay compliant with regulations such as GDPR and HIPAA. These regulations are designed to protect individuals' data and impose strict guidelines on how businesses collect, store, and manage information. Non-compliance can lead to severe penalties, making adherence essential.
Artificial Intelligence Applications
Integrating artificial intelligence into data quality management can yield significant benefits.
AI in Business Automation
AI has the potential to automate data cleansing processes, reducing the time and resources spent on manual data management. By utilizing machine learning algorithms, organizations can identify anomalies and discrepancies more efficiently than traditional methods.
AI Algorithms and Applications
Applications in AI extend beyond cleansing. Predictive analytics can help organizations foresee potential challenges in data quality before they occur, thus preemptively addressing issues. This predictive capability leads to more reliable datasets, which foster better decision-making.
Ethical Considerations in AI
However, the use of AI raises ethical concerns. Issues related to bias in algorithms can compromise data quality. Organizations must ensure that their AI frameworks are transparent and accountable to maintain trust and integrity.
Industry-Specific Research
Different industries face unique challenges regarding data quality. Tailoring solutions to address these challenges is crucial for effective data management.
Tech Research in Finance Sector
In the finance sector, regulatory compliance and data accuracy are critical. Financial institutions must maintain high data quality standards to meet both internal and external requirements.
Healthcare Technological Advancements
Healthcare organizations rely heavily on accurate patient data to improve outcomes. The integration of electronic health records and advanced analytics tools helps manage and maintain high data quality in this sensitive field.
Retail Industry Tech Solutions
In retail, data quality impacts inventory management and customer satisfaction. Cutting-edge solutions in data analytics can help retailers make better purchasing decisions by accurately predicting consumer trends and preferences.
By understanding the nuances in data quality management, organizations can better equip themselves to face the challenges of an ever-evolving digital landscape.
\n Through continuous monitoring and improvement, organizations can achieve a level of data integrity that not only elevates their internal processes but also enhances their overall strategic direction.
Foreword to Data Quality Planning
In today's data-driven landscape, organizations continually seek to leverage data for competitive advantage. A structured data quality plan is, therefore, essential for ensuring that data remains reliable and actionable. This section delves into the importance of data quality planning, elucidating its benefits, key considerations, and the broader implications for organizations.
Data quality can be defined as the degree to which data is accurate, complete, consistent, and timely. When organizations fail to establish a robust data quality plan, they risk making decisions based on flawed data, which can lead to costly mistakes and missed opportunities. Comprehensively articulating a data quality strategy enhances not only data management practices but also overall operational efficiency.
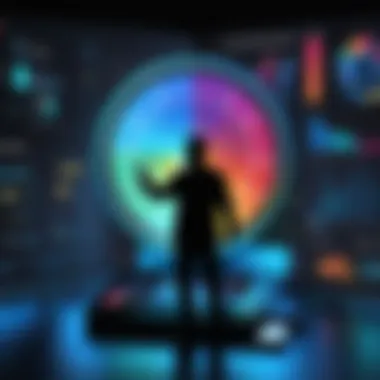
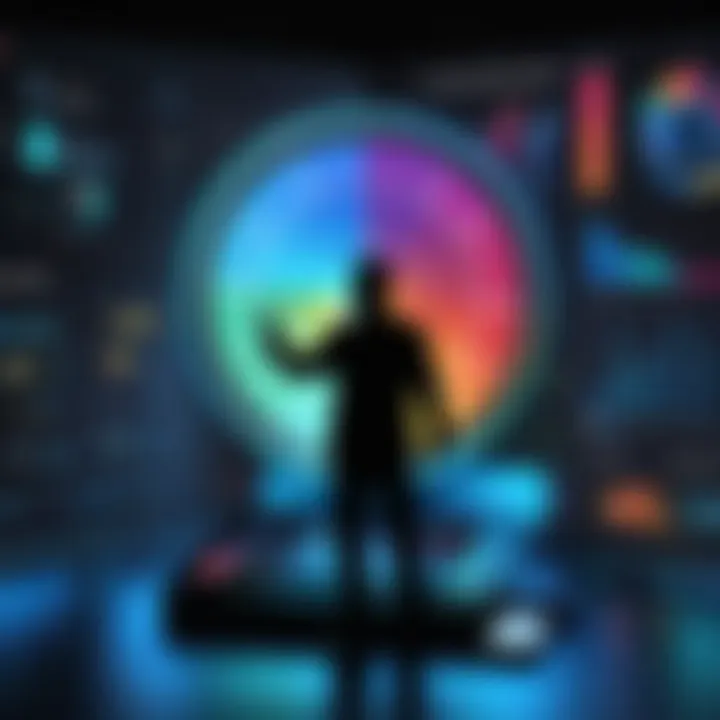
Defining Data Quality
Data quality encompasses various attributes that determine the value of data within an enterprise context. Some core dimensions include accuracy, completeness, consistency, and validity. Attributes like these are crucial because they form the foundation upon which businesses make decisions. Accurate data ensures that insights are reliable; complete data guarantees that decisions consider all necessary information; consistent data maintains uniformity across different systems. Keeping these aspects in check is essential for an effective data strategy.
Significance of Data Quality Plans in Business
Data quality plans hold critical importance for organizations seeking to thrive in a competitive environment. A well-crafted data quality plan serves multiple purposes:
- Risk Mitigation: Poor data quality can lead to significant financial and reputational risks. By proactively identifying and addressing data quality issues, organizations can prevent major setbacks.
- Regulatory Compliance: Many industries have regulations that dictate data usage standards. A formal quality plan aids in ensuring compliance, saving organizations from potential fines.
- Enhanced Decision-Making: Accurate data translates into better insights, allowing decision-makers to make informed choices. This fosters organizational agility and responsiveness.
- Customer Satisfaction: Superior data quality directly impacts customer interactions, contributing to a better overall experience. Satisfied customers tend to be loyal, resulting in increased revenue over time.
"Data is a precious thing and will last longer than the systems themselves." – Tim Berners-Lee
In summary, the crafting of an effective data quality plan is integral to improving data management strategies and supporting the broader goals of enterprises. With the right framework in place, businesses can not only enhance their data-related practices but also drive significant growth and success.
Components of a Data Quality Plan
An effective data quality plan is comprised of certain key elements that jointly ensure the quality, integrity, and usability of data across an organization. Such components serve as the backbone for systematic data governance, allowing businesses to make informed decisions based on reliable data. The critical components include understanding different data quality dimensions and establishing a strong governance framework. Each plays a vital role in creating a comprehensive plan that addresses the specific needs of the organization and drives operational success.
Data Quality Dimensions
The data quality dimensions are various criteria that measure specific characteristics of data. They are crucial in assessing the overall quality of data within an organization. These dimensions include Accuracy, Completeness, Consistency, Timeliness, and Uniqueness. Each of these dimensions contributes in its own way to the effectiveness of the data quality plan.
Accuracy
Accuracy refers to the correctness of data and its alignment with the real-world context it represents. High accuracy reduces errors in reporting and decision-making processes. An organization utilizes accurate data for reliable insights.
Key Characteristic:
An important aspect of accuracy is its quantifiable nature, making it easier to assess using statistical measures.
Benefits:
Accurate data leads to trustworthy results and builds credibility within organizational processes.
Unique Feature:
Accuracy often involves regular checks and validations to maintain standards, which can require more resources and time for continuous sustainment.
Completeness
Completeness assesses whether all necessary data is present and can be utilized effectively. Incomplete data can lead to biased analyses and misinformed decisions.
Key Characteristic:
Completeness emphasizes the importance of having a holistic view of the data landscape.
Benefits:
Ensuring completeness enhances the reliability of analyses and outcomes in organizational processes.
Unique Feature:
Completeness can be tricky. Data collection efforts need to be comprehensive, requiring meticulous planning and execution.
Consistency
Consistency checks if data across various databases or systems is uniform. Data should portray a single version of information. Inconsistent data can lead to confusion and errors in judgment.
Key Characteristic:
Consistency is vital for maintaining coherence in reporting across different platforms or departments.
Benefits:
Consistent data fosters trust in reports and insights drawn from that information.
Unique Feature:
Challenges arise when integrating multiple data sources, making data consolidation essential yet complex.
Timeliness
Timeliness refers to the availability of data when it is needed. Outdated or delayed data can significantly impact decision-making processes.
Key Characteristic:
Timeliness ensures that data is actionable and relevant at the moment it is required.
Benefits:
Access to timely data improves responsiveness to market changes and enhances strategy execution.
Unique Feature:
Implementing timely data operations demands an efficient flow of information across relevant stakeholders in real-time.
Uniqueness
Uniqueness assesses that data is not duplicated within the datasets. Redundant data can clutter the landscape and produce misleading outputs.
Key Characteristic:
This dimension ensures that every data entry holds meaningful and non-repetitive information.
Benefits:
Unique data enhances clarity and reduces the ambiguity often caused by duplicate records.
Unique Feature:
Establishing uniqueness can require additional procedures, such as deduplication methods and audits, which may be resource-intensive.
Establishing Data Governance Frameworks
Establishing a strong data governance framework is fundamental in managing the various facets of a data quality plan. This framework delineates roles, responsibilities, and processes, ensuring that data is properly managed throughout its lifecycle. Without a governance structure, organizations risk inconsistency, mismanagement, and a lack of accountability in leadership and processes.
Governance frameworks encompass policies, procedures, and standards that guide data handling and use. Organizations should aim for a clear set of governance strategies detailing not just how data should be managed, but also who should oversee its various dimensions. A formal data governance body can help in making data management a priority, fostering a strength in data-focused culture.
"Data governance is not merely a set of rules; it is an integral structure that nurtures effective data usage within an organization."
Implementing such frameworks encourages a proactive approach, ensuring data quality remains a strategic focal point of high importance.
Identifying and Defining Key Data Sources
By pinpointing the sources of data, organizations can gain insights into its quality and relevance. Evaluating the data sources delivers clear visibility into potential issues that may affect downstream operations. Furthermore, clarity around data sources helps create trust among stakeholders. They will have confidence regarding the datasets driving business decisions.
Mapping Data Sources
Mapping data sources involves an intricate examination of all potential repositories of data within an organization. This task requires systematic documentation. Organizations must categorize the data based on its type and usage. This mapping can take several forms:
- Internal sources: Data generated from within the organization, such as customer relationship management (CRM) systems, financial records, and operational databases.
- External sources: Third-party data that offers additional insights, such as market research reports or demographic information from reputable services.
- Public sources: Government databases and academic publications that can provide valuable information.
Creating a clear visual representation of data flows can be beneficial. This not only illuminates points of integration but also identifies redundancies. Using tools like Microsoft Visio or Lucidchart can aid in this process. Visuals support understanding and communication of the data flow across systems, enhancing the overall quality management strategy.
Evaluating Data Source Quality

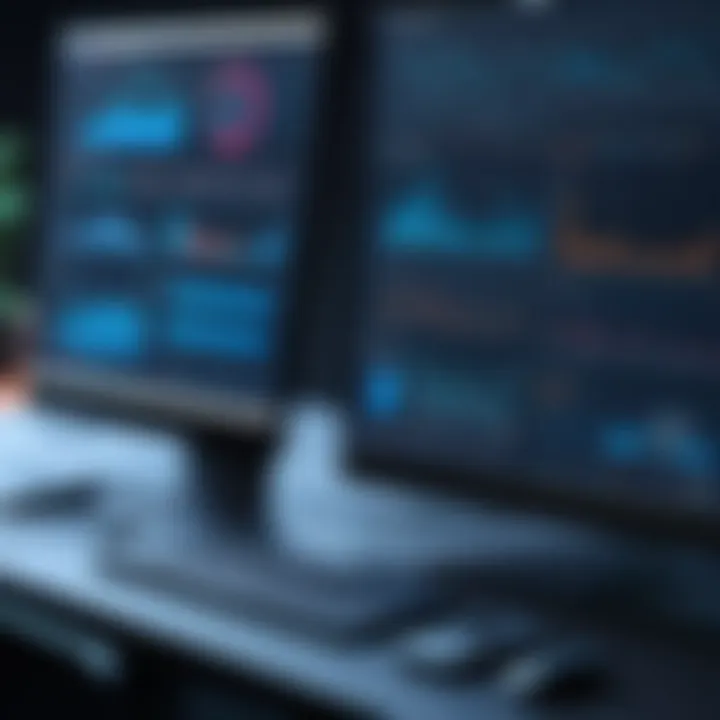
Evaluating data source quality is an essential step that allows organizations to assess the reliability and validity of their data. There are several aspects to this evaluation task that require careful consideration:
- Data accuracy: This measures how closely the data aligns with the true values. For example, if a dataset claims to contain sales figures, those numbers must be verified against source documents.
- Data completeness: It refers to whether all necessary data is present. Missing values can lead to skewed analyses.
- Data consistency: This checks if data across different sources align with one another. Conflicting information can cause confusion and misinformed decisions.
To systematically evaluate data quality, organizations can employ a scoring system that rates each data source against these criteria. Tools like Talend or Informatica can also help streamline this process by providing automated data quality checks.
"A strong understanding of data provenance protects against the blind spots that can arise from poor data sourcing."
This overview of mapping and evaluating data sources illustrates the pathway toward enhanced data quality. A grounded approach facilitates ongoing improvements and shifts the focus toward data-driven success.
Setting Metrics for Data Quality Assessment
Establishing metrics for data quality assessment is an essential aspect of any data quality plan. Metrics provide the foundation for understanding and evaluating the state of data within an organization. Without measurable parameters, it becomes challenging to identify gaps or areas for improvement. Metrics offer a way to quantify data quality, allowing organizations to take informed actions that enhance data integrity and reliability.
When crafting metrics, it is crucial to focus on specific elements that directly impact data quality. These include accuracy, completeness, consistency, timeliness, and uniqueness. Each dimension serves a purpose, helping organizations to monitor their data effectively. For instance, accuracy measures how closely data values align with reality, while completeness assesses whether all necessary data is available. Consistency checks for uniformity across different datasets, and timeliness deals with how up-to-date the data is. Uniqueness ensures there are no duplicated records, which can skew analysis and decision-making.
The benefits of developing robust metrics are manifold. First, they enable organizations to have a clear benchmark for data quality. This means organizations can gauge their current data status against the established parameters. Second, they provide insights into trends over time, helping stakeholders understand whether efforts to improve data quality are effective. Third, they facilitate accountability by assigning responsibilities tied to specific metrics, ensuring that teams are engaged in maintaining quality standards.
Developing Quality Metrics
Creating quality metrics requires a systematic approach. Initially, stakeholders should agree on the definitions of each metric. This clarity avoids confusion and misinterpretation down the line. After definitions are established, the next step is to determine the formulas or methods for calculating each metric. For example, accuracy can be measured as the percentage of correct entries against total entries.
It is also important to consider the data sources that will be used for measurement. Understanding the structure and nature of the data sources aids in setting realistic targets. The actual calculation should be automated as much as possible to reduce human error and ensure timely reporting. Tools such as Tableau or Microsoft Power BI can be instrumental in visualizing these metrics.
Benchmarking and Evaluating Metrics
Once quality metrics are established, the benchmarking process begins. Benchmarking involves comparing data quality metrics against industry standards or practices of similar organizations. This activity offers an external perspective, helping to identify whether current metrics are aligned with best practices.
Evaluation of metrics should not be a one-time effort. It is essential to continuously assess the relevance and effectiveness of the metrics. As organizational goals change or new data sources become available, metrics may need adjustment. Regular reviews ensure that metrics remain aligned with the organization’s evolving needs.
Businesses should also look to leverage feedback from various teams within the organization. User experience with data can provide insights into the practicality of the metrics established. Ultimately, consistent benchmarking and evaluation allow organizations to maintain high data quality standards and adapt proactively to any emerging challenges.
Tools and Technologies for Data Quality Management
In today's data-driven world, the management of data quality is paramount for organizations seeking to optimize their operations and enhance decision-making. Tools and technologies play a critical role in this process. They facilitate the systematic identification, assessment, and rectification of data quality issues. Organizations that embrace these technologies tend to benefit from increased efficiency and improved accuracy in outcomes.
Specific elements within data quality management tools encompass data profiling, cleansing, monitoring, and reporting. Data profiling allows organizations to examine their data assets and assess their quality. Data cleansing tools help in correcting inaccuracies and inconsistencies. Monitoring technologies provide continuous oversight of data quality, which is vital for maintaining integrity over time. Finally, reporting tools enable organizations to visualize their data quality status, fostering better decision-making and accountability.
The benefits of implementing data quality tools are manifold. They streamline processes that would otherwise be very time-consuming and manual. By automating data quality checks, organizations can ensure ongoing compliance with established standards, ultimately leading to enhanced trust in the data. However, organizations must consider the specific needs of their operations when selecting these tools.
Overview of Data Quality Tools
Several data quality tools exist to help organizations with their data management needs. Some prominent examples include Informatica, Talend, and IBM InfoSphere Quality Stage. Each of these tools provides distinct features aimed at addressing different aspects of data quality.
- Informatica: Known for its robust data integration capabilities, Informatica offers strong data profiling and cleansing functionalities. This tool is often favored by large enterprises for its scalability and comprehensive feature set.
- Talend: An open-source option, Talend is popular among organizations that prefer cost-effective solutions that don't compromise on quality. Its community edition allows for basic data quality management, while the paid versions offer advanced features.
- IBM InfoSphere Quality Stage: A powerful solution for managing data quality at enterprise levels. It provides sophisticated tools for profiling, cleansing, and monitoring data effectively.
- SQL Server Data Quality Services: Integrated into Microsoft SQL Server, this tool allows organizations to manage their data quality using familiar SQL environments.
Implementing these tools results in an organized approach to identifying data issues and rectifying them, leading to better overall data quality.
Evaluating Tools for Organizational Needs
Choosing the right data quality management tool requires an evaluation of specific organizational needs. Key considerations include functionality, scalability, integration capabilities, and cost.
- Functionality: Assess what features are essential for your organization. Does your team need extensive data cleansing options, or is profiling sufficient?
- Scalability: Ensure the tool you choose can adapt as your organization's data grows. A tool that works well for a small data set may not be suitable for larger volumes.
- Integration capabilities: Data quality tools must work seamlessly with existing systems. If a tool cannot integrate with databases and other software your organization uses, it may create more issues.
- Cost: Evaluate the total cost of ownership. Consider initial purchase price, maintenance fees, and potential training costs for personnel.
Choosing the right tool can make the difference between a robust data management strategy and one that fails to deliver on its promises.
Stakeholder Involvement in Data Quality Processes
In the pursuit of reliable data quality management, the engagement of stakeholders becomes crucial. Stakeholders provide unique perspectives, insights, and the practical experience needed to shape an effective data quality plan. Their involvement can enhance the accuracy of data, ensure the appropriateness of metrics, and foster a culture of accountability. Creating a data quality plan is not merely a technical challenge; it is an exercise in collaboration and communication that can significantly impact organizational outcomes.
Identifying Key Stakeholders
Determining which stakeholders to engage is the first step in the process. Key stakeholders typically include:
- Data Owners: Usually from departments that generate and maintain the data. Their knowledge of data handling practices is invaluable.
- Data Consumers: Users who rely on data for making decisions. Their feedback on the functionality and relevance of data is crucial.
- IT Personnel: They manage the technical side, like data storage and data management systems. Their input is essential for ensuring appropriate tools and technologies are employed.
- Compliance Officers: Their role is to ensure that data management practices comply with regulations. Their insights help structure data governance frameworks.
- Business Analysts: They often analyze trends and insights from the data. Their understanding of business needs informs the criteria for quality standards.
Identifying these stakeholders ensures a comprehensive approach to data quality. A diverse group will lead to a multi-dimensional understanding of data requirements and challenges.
Facilitating Communication and Collaboration
Once stakeholders are identified, fostering effective communication and collaboration is critical. This can be achieved through various methods:
- Regular Meetings: Schedule consistent check-ins to allow stakeholders to discuss progress, challenges, and insights related to data quality.
- Workshops and Training: Organizing sessions to train stakeholders on the importance of data quality and their roles can promote a shared understanding of goals.
- Collaborative Tools: Utilize platforms that enable shared documentation and real-time updates, helping stakeholders remain aligned in their efforts. Tools like MS Teams, Slack or Asana can be beneficial.
- Feedback Mechanisms: Create channels for stakeholders to provide feedback on the data quality initiatives. Surveys or feedback forms can solicit valuable insights.
- Clearly Defined Roles: Ensuring every stakeholder knows their responsibilities avoids confusion and promotes accountability.
By establishing these communication channels, organizations enable a more collaborative environment. This engagement not only enhances data quality but also facilitates a culture where data integrity is prioritized.
Effective stakeholder involvement in data quality processes can lead to a substantial reduction in data errors, ultimately enhancing decision-making capabilities throughout the organization.
Implementation of the Data Quality Plan
The implementation of a data quality plan is crucial to its success. A well-structured plan ensures that data remains reliable and valuable for decision-making processes. Effective implementation involves several steps that require careful consideration and alignment with organizational goals. By prioritizing this process, businesses can minimize errors and enhance data integrity.
Pilot Testing and Iteration
Pilot testing serves as the foundation for implementing the data quality plan. This initial phase is about validating the effectiveness of the proposed strategies and methods on a smaller scale. During pilot testing, teams should focus on specific data sets or areas likely to showcase the plan’s impact quickly. This initial roll-out allows organizations to identify gaps or challenges in their approach.
- Feedback Collection: Establish channels for stakeholders to provide insights and feedback. Regular updates help address issues early, ensuring modifications can be made swiftly.
- Performance Metrics: Evaluate data quality dimensions such as accuracy and completeness during this phase. Real-time data collection can reveal whether the deployment meets established metrics.
- Iterative Improvements: Use feedback and performance evaluations to refine processes continuously. The iterative nature of this step allows for gradual but impactful changes.
Full-Scale Deployment
Following a successful pilot test, organizations can proceed to full-scale deployment. This stage encompasses a broader implementation of the data quality plan across the enterprise. It is essential to maintain the lessons learned from the pilot phase and apply them to this more extensive execution.
- Comprehensive Training: Ensuring that all team members are educated about the data quality plan is vital. This includes understanding their roles and the importance of maintaining data integrity.
- Integration with Existing Systems: The plan must harmonize with current data infrastructure. Identify potential challenges and solutions early in this phase to ensure a smooth transition.
- Monitoring Mechanisms: Establish robust monitoring systems to ensure ongoing adherence to data quality standards. These mechanisms should facilitate adjustments and foster continuous improvement based on real-time insights.
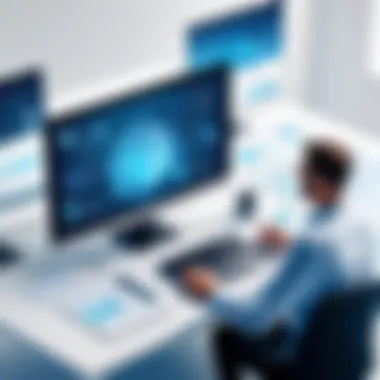
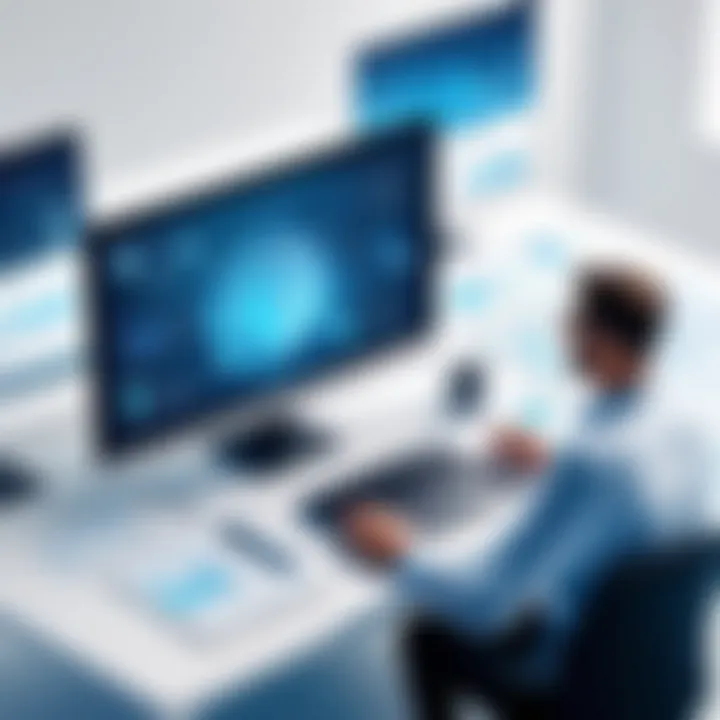
The deployment of a data quality plan should not be viewed as a one-time effort but a continuous endeavor to refine and enhance data practices.
In summary, implementation requires thorough planning and dedicated execution. Organizations must recognize the interplay between pilot testing and full-scale deployment as interconnected steps in a continuous process. Ensuring all team members are aligned with the data quality objectives can lead to significant improvements in data quality and overall business performance.
Monitoring and Maintaining Data Quality
Monitoring and maintaining data quality is a critical part of any effective data quality plan. Without this continuous oversight, organizations risk allowing data integrity issues to proliferate, leading to poor decision-making and potentially significant business impacts. This section explores the elements that make continuous monitoring essential, the benefits it offers, and considerations for businesses embarking on this necessary journey.
One of the key aspects of data quality is its dynamic nature. Data landscape evolves – new data is collected, existing data is modified, and systems change. Thus, a one-time assessment is not sufficient. In fact, it is paramount to have robust processes in place that allow for ongoing evaluation of data quality. Organizations can benefit by establishing clearly defined metrics to gauge various dimensions of quality such as accuracy, completeness, and consistency. This should be part of a larger strategic framework.
Establishing Continuous Monitoring Processes
To ensure data quality is maintained over time, organizations must establish continuous monitoring processes. These processes involve automated tools and manual checks that regularly assess data against established quality metrics.
- Automation - Leveraging technology can streamline monitoring efforts. Automated systems can run checks at set intervals, flagging any anomalies or deviations from expected quality standards.
- Data Profiling - Assessing the data's current state before and after any changes can uncover quality issues. This often involves profiling data to understand its distribution, trends, and patterns. Data profiling tools can be invaluable in this aspect.
- Alerts and Notifications - By implementing alert systems, stakeholders can be notified promptly if data quality falls below acceptable thresholds. This proactive measure helps in addressing issues before they escalate.
- Documentation and Process Logging - Keeping detailed logs helps teams track changes and understand the impact of various operations on data. This is crucial for audits and compliance purposes.
Through these methods, organizations can stay vigilant, ensuring that their data remains reliable and trustworthy over time.
Feedback Loops for Continuous Improvement
Incorporating feedback loops into the data quality monitoring process enhances the overall ability to make informed decisions. This involves collecting insights from the monitoring processes and using them to inform and revise data quality strategies.
- Regular Reviews - Scheduled assessments of the monitoring processes can reveal gaps or areas for improvement. Implementing a feedback mechanism where insights are shared with relevant stakeholders fosters accountability.
- Stakeholder Input - Engage key users and data stewards to gather feedback on data usability and quality. Their practical insights can guide necessary adjustments, ensuring that the processes align with business needs.
Continuous improvement is not a one-time initiative; it's a culture that needs to be fostered. Regularly integrating feedback creates a cycle of enhancement that aligns with organizational goals.
- Iterative Changes - Address any identified issues with actionable changes. Adapt the monitoring parameters based on emerging needs or technological advancements in the field of data management.
Establishing a culture of continuous improvement not only helps maintain high data quality standards but also promotes a proactive approach to data management across the organization. This ongoing commitment enhances overall operational efficiency and decision-making capabilities.
Challenges in Data Quality Management
A well-formulated data quality plan is vital for organizations aiming to leverage their data assets to drive informed decision-making. However, the journey toward achieving high data quality is fraught with challenges that, if ignored, threaten the integrity and reliability of data-driven strategies. Understanding these challenges is crucial as it allows companies to devise effective solutions and reinforce their data governance frameworks.
One prominent challenge lies in data integration. As organizations often pull data from diverse systems, inconsistencies may arise. Integrating data sources without a thorough quality assessment can lead to discrepancies, eventually undermining trust in the resulting analyses.
Another challenge is data volume. The rapid growth of data can overwhelm existing quality management processes. Organizations frequently underestimate the complexity involved in managing large datasets, leading to overlooked errors and quality issues.
Furthermore, there is the risk of changing business requirements. As organizations evolve, their data needs may shift. A quality plan fixed in its approach may become obsolete, resulting in data that no longer supports current business objectives. Organizations must maintain agility in their data quality strategies to adjust to these changes effectively.
Key takeaway: The hurdles in data quality management must be approached systematically. Organizations should not only identify these challenges but also anticipate their impacts on overall data management objectives.
Common Pitfalls in Data Quality
Organizations often encounter several common pitfalls in their pursuit of data quality. One of the foremost issues is a lack of clear ownership and responsibility concerning data quality initiatives. Without designated roles for managing data quality, it becomes challenging to hold anyone accountable for data problems.
In addition, teams may suffer from insufficient training. Data users typically require training to understand data quality principles. A workforce untrained in data quality best practices can lead to errors and misinterpretations that further degrade quality.
Another frequent pitfall is inadequate resources. Organizations may allocate insufficient funds or personnel to their data quality initiatives, which ultimately leads to subpar implementation of quality measures. Without robust resources, the likelihood of failure increases. Factors such as these can cause a downward spiral in data reliability, leading to larger systemic issues.
Strategies to Overcome Challenges
To counteract these challenges, organizations must prioritize several strategic approaches. Firstly, establishing clear roles and responsibilities is crucial. When employees understand their specific roles in maintaining data quality, accountability increases.
Investing in comprehensive training programs can address knowledge gaps within teams. By fostering an environment where data quality knowledge is shared, organizations can mitigate the impact of human error and enhance their overall data management capabilities.
Moreover, allocating adequate resources towards data governance cannot be understated. This might include investing in advanced data quality tools, as well as ensuring that there is sufficient personnel to monitor data quality standards continuously.
Additionally, adopting a flexible approach to the data quality plan is essential. Organizations should regularly review and revise their plans to reflect changing business requirements. This ensures that data remains aligned with organizational goals and continues to provide meaningful insights.
In essence, recognizing these common pitfalls and implementing thoughtful strategies will lead to more effective data quality management. Organizations will create a robust foundation to support their data-driven initiatives.
Case Studies of Successful Data Quality Plans
Case studies play a critical role in understanding the practical applications and success of data quality plans in various organizations. They provide real-world examples of how companies face their data challenges. By examining these instances, organizations can glean valuable insights into the formulation and execution of effective data quality strategies. Success stories not only highlight the benefits associated with strong data quality practices but also illustrate specific considerations and adaptations required for unique organizational contexts.
Incorporating case studies in the discourse about data quality plans allows businesses to see proven methodologies at work. Such examples act as a guide for companies seeking to improve their own data management practices. They demonstrate how data quality planning can result in enhanced operational efficiency, better decision-making, and ultimately, improved business outcomes.
Industry-Specific Examples
Different industries encounter diverse challenges related to data quality. For instance:
- Healthcare: A leading hospital implemented a data quality plan that enhanced patient record accuracy. They introduced real-time data validation systems which significantly reduced errors related to patient information. This not only improved treatment outcomes but also ensured compliance with regulatory standards.
- Finance: A prominent financial services firm developed a rigorous data quality framework to manage risk and ensure compliance. By establishing strict data governance practices, they effectively minimized discrepancies in transaction records, leading to a more reliable audit process.
- Retail: A large retail chain adopted a comprehensive data quality strategy focusing on inventory data. They integrated predictive analytics to forecast demand accurately, which reduced stock-outs and overstock situations. This approach boosted customer satisfaction and optimized supply chain operations.
These examples exhibit how tailored data quality plans can address sector-specific challenges and leverage data as a strategic asset.
Lessons Learned from Implementations
Analyzing successful case studies offers various lessons that organizations can adopt for their initiatives. Key takeaways include:
- Customization is Crucial: Each organization has unique data challenges. Tailoring a data quality plan to fit specific business needs and industry standards is essential for success.
- Engage Stakeholders Early: Effective communication and collaboration among stakeholders enhance the likelihood of successful implementation. Involving users from the outset helps form a quality plan that truly reflects the organization’s objectives.
- Iterate and Adapt: Continuous improvement is a fundamental concept in effective data quality management. Organizations that frequently review and iterate their plans adapt better to changing business landscapes.
- Invest in Training: Empower employees with the knowledge and tools necessary to manage data effectively. Training helps in cultivating a culture of data quality that persists over time.
"Successful data quality management is not just about the tools but also about the people and processes that drive it."
The End
The conclusion of this article serves as a pivotal component in reinforcing the overarching themes of data quality planning. A well-crafted data quality plan not only enhances a company's data management strategies, but it also drives integrity and precise decision-making. It synthesizes various aspects mentioned throughout the article, creating a cohesive understanding of the topic.
Recap of Key Points
- Importance of Data Quality: Highlighted the critical roles that accuracy, completeness, and consistency play in maintaining high data standards.
- Components of an Effective Plan: Discussed the necessity of establishing governance frameworks and evaluating key data sources.
- Stakeholder Engagement: Emphasized that stakeholder involvement is vital for successful implementation and monitoring of the data quality plan.
- Technology and Tools: Addressed the selection of appropriate tools and technologies tailored for specific organizational needs to enhance data quality.
- Continuous Improvement: Underlined the significance of establishing feedback loops for ongoing maintenance of data integrity.
This recap emphasizes the requirement for organizations to actively engage with these critical elements in order to forge meaningful improvements in their data quality efforts.
Forward-Looking Strategies
Organizations aiming to develop an effective data quality plan should consider several strategies moving forward:
- Adopt a Proactive Approach: Rather than waiting for data errors to surface, organizations should anticipate potential issues through planned assessments and regular audits.
- Leverage Advanced Technologies: Explore options like Artificial Intelligence and Machine Learning for predictive analytics in data quality management.
- Foster a Data-Centric Culture: Encourage all employees to prioritize data integrity, ensuring that quality is a shared responsibility across all departments.
- Regular Training and Development: Invest in ongoing education for staff to keep them updated on best practices and tools for maintaining data quality.
- Iterate and Adapt: Be flexible in adjusting the data quality plan based on changing business needs or emerging technology trends.
The successful execution of these strategies will build a solid foundation for sustainable data quality management, thereby yielding long-term benefits for the organization. This conclusion encapsulates the necessity of a structured approach toward data quality, urging professionals to prioritize its strategic importance.