Augmented Data Unveiled: A Strategic Guide for Business Optimization
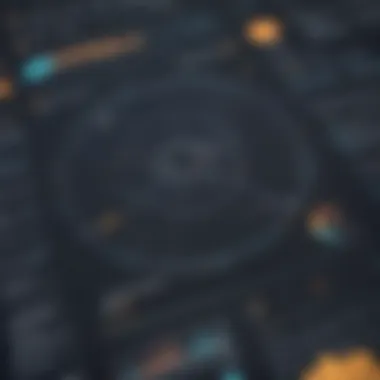
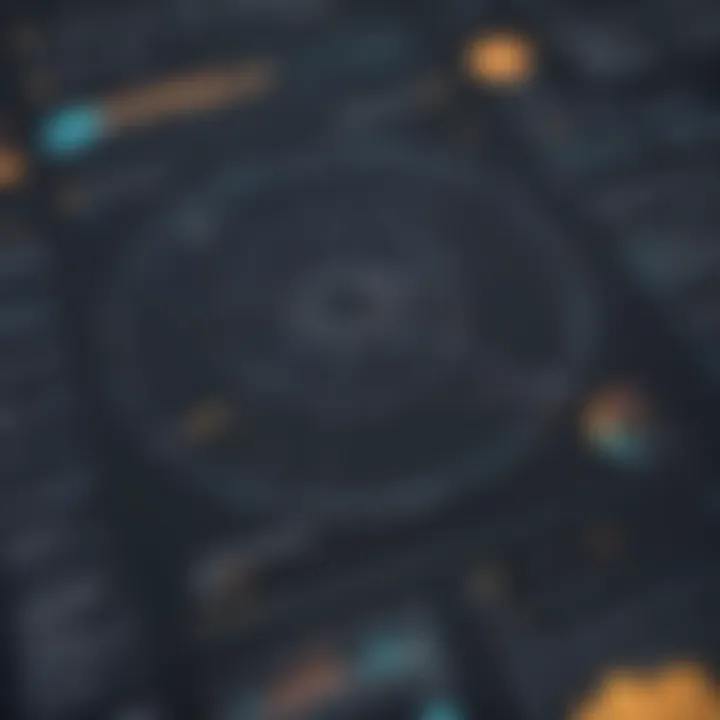
Technological Research Overview
In the realm of technological research, a myriad of recent innovations have paved the way for a significant evolution in augmenting data processes within businesses. These advancements, primarily driven by artificial intelligence (AI) and machine learning (ML) technologies, have revolutionized the quality and relevance of data used in business applications. Such innovations are proving to have a profound impact on business operations by enhancing decision-making capabilities, optimizing processes, and ultimately fostering growth. As businesses tread further into the digital era, exploring these trends in augmented data becomes imperative for staying competitive and harnessing the full potential of technological advancements.
Data Analytics in Business
The significance of data analytics in business cannot be overstated in today's data-driven landscape. It serves as the bedrock for informed decision-making, offering valuable insights that drive strategic initiatives and operational efficiency. Leveraging cutting-edge tools for data analysis empowers businesses to extract meaningful patterns and trends from vast data sets, enabling them to make data-driven decisions with precision and confidence. Through real-world case studies showcasing the power of data analytics, businesses can learn from successful implementations and adapt best practices to optimize their own data strategies.
Cybersecurity Insights
Delving into cybersecurity insights is crucial for businesses looking to safeguard their operations in an increasingly digital world characterized by sophisticated cyber threats. Conducting a thorough analysis of the threat landscape is essential for understanding the dynamics of cyber risks and formulating proactive security measures. By embracing best practices in cybersecurity, organizations can fortify their defenses and mitigate vulnerabilities effectively. Additionally, ensuring compliance with regulatory frameworks is imperative to uphold data protection standards and enhance overall cybersecurity posture.
Artificial Intelligence Applications
Artificial intelligence (AI) applications hold immense transformative potential across various facets of business operations. From enabling business process automation to facilitating predictive analytics, AI algorithms drive innovation and efficiency. However, ethical considerations in AI implementation loom large, necessitating careful navigation of potential risks and considerations around data privacy, bias mitigation, and transparency. Understanding the scope and limitations of AI technologies is crucial for maximizing their benefits while upholding ethical standards and regulatory compliance.
Industry-Specific Research
Industry-specific research offers tailored insights into the technological landscape across various sectors. In the finance sector, technological research is driving advancements in predictive analytics, risk assessment, and personalized financial services. Healthcare is witnessing a technological revolution with innovations in telemedicine, electronic health records, and healthcare data analytics. Retail industry tech solutions range from AI-powered customer analytics to inventory management systems, shaping personalized retail experiences and optimizing supply chain management.
Introduction to Augmented Data
Augmented data is a cutting-edge concept that combines artificial intelligence (AI) and machine learning to elevate the quality and relevance of data in business applications. In the digital era, where data reigns supreme, understanding and harnessing the power of augmented data can provide businesses with a significant competitive advantage. By infusing AI and machine learning into traditional data sets, companies can make more informed decisions, streamline operations, and fuel growth. This article serves as a comprehensive guide to elucidate the intricacies of augmented data and its ramifications for businesses in various industries.
Understanding the Concept of Augmented Data
Defining augmented data
Augmented data, at its core, involves enhancing existing datasets with AI algorithms and machine learning models. This integration empowers organizations to derive deeper insights, predict trends, and optimize business strategies with unprecedented precision. The key characteristic of augmented data lies in its ability to enrich raw information, enabling businesses to extract value from large and complex datasets efficiently. This approach is particularly advantageous in scenarios where traditional data analytics fall short, offering a proactive and dynamic framework for decision-making.
The role of AI and machine learning
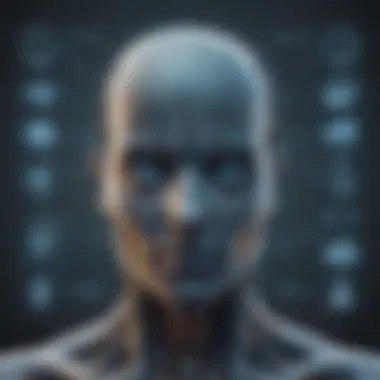
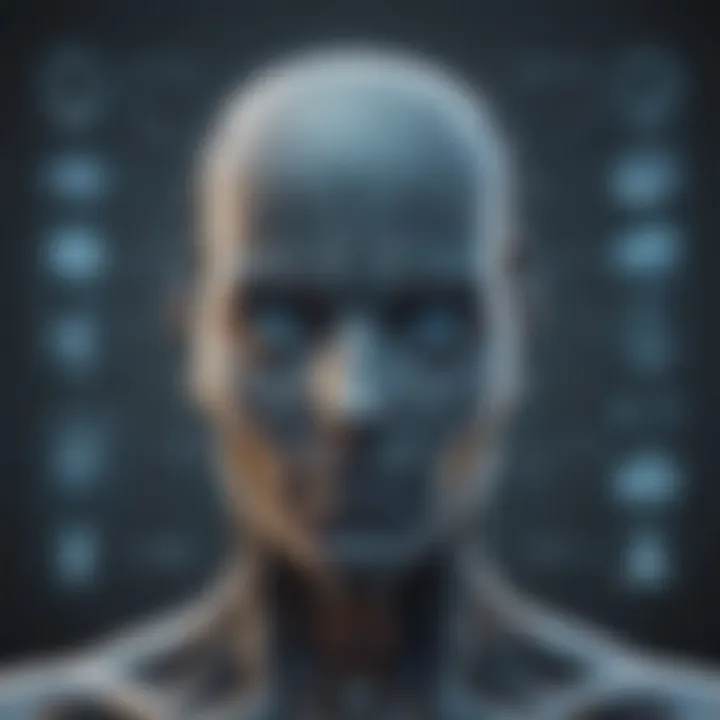
AI and machine learning play a pivotal role in augmenting data by enabling automation, pattern recognition, and predictive analytics. By leveraging AI-powered algorithms, businesses can distill vast amounts of data into actionable intelligence, driving operational efficiencies and innovation. The unique feature of incorporating AI and machine learning in data augmentation is the scalability and adaptability it provides. However, challenges such as algorithm bias and data privacy concerns must be carefully navigated to harness the full potential of this technology.
Importance of Augmented Data for Businesses
Enhanced decision-making
Augmented data empowers businesses to make informed decisions based on real-time insights and predictive analytics. By integrating AI-driven models, organizations gain a competitive edge in strategic planning and risk management. The key characteristic of enhanced decision-making through augmented data is the agility and accuracy it offers, enabling agile responses to changing market dynamics and consumer behavior.
Improved data accuracy
Enhancing data accuracy is a critical aspect of augmented data, as it ensures that business decisions are founded on reliable and up-to-date information. By leveraging machine learning algorithms for data cleansing and validation, organizations can minimize errors and optimize data quality. The unique feature of improved data accuracy lies in its ability to enhance data integrity and credibility, fostering trust among stakeholders and facilitating data-driven decision-making.
Driving business growth
The primary goal of augmented data in the business context is to drive growth and scalability. By harnessing AI and machine learning for predictive analytics and market segmentation, companies can identify new opportunities, optimize processes, and personalize customer experiences effectively. The key characteristic of driving business growth through augmented data is the transformative impact it has on operational efficiency and revenue generation. However, addressing challenges such as data integration complexities and talent acquisition is essential to maximizing the growth potential of augmented data initiatives.
Applications of Augmented Data
Augmented Data plays a crucial role in reshaping the landscape of modern businesses by integrating cutting-edge technologies like artificial intelligence and machine learning. This innovative approach revolutionizes decision-making processes, elevates data accuracy, and uncovers growth opportunities in the digital era. Businesses can leverage augmented data to refine their operations, gain competitive advantages, and unlock new possibilities through enhanced data quality, relevance, and application.
Utilizing Augmented Data in Various Industries
E-commerce
E-commerce sector benefits significantly from augmented data utilization due to its reliance on consumer insights and personalized experiences. By harnessing augmented data, e-commerce businesses can enhance customer segmentation, optimize product recommendations, and streamline order fulfillment processes. The key characteristic of augmented data in e-commerce lies in its ability to predict consumer behavior accurately, enabling precise targeting strategies and tailored promotional offers. However, challenges such as data privacy concerns and algorithm bias need careful consideration.
Healthcare
Augmented data plays a pivotal role in the healthcare industry by improving patient care, treatment outcomes, and operational efficiency. Healthcare providers leverage augmented data for precision medicine, disease prediction, and resource management. The unique feature of augmented data in healthcare is its potential to revolutionize diagnostic accuracy and treatment efficacy. Despite its advantages, concerns regarding data security and ethical implications must be addressed to ensure trustworthy implementation.
Finance
The finance sector embraces augmented data to optimize risk assessment, fraud detection, and investment strategies. Augmented data empowers financial institutions to analyze market trends, predict economic shifts, and personalize customer experiences. The distinctive feature of augmented data in finance is its capability to improve decision-making accuracy and speed, leading to enhanced portfolio management and reduced risks. Nevertheless, challenges related to data governance and regulatory compliance require meticulous attention.
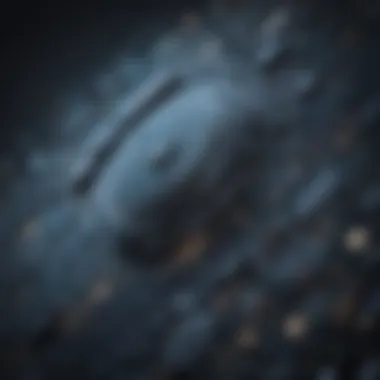
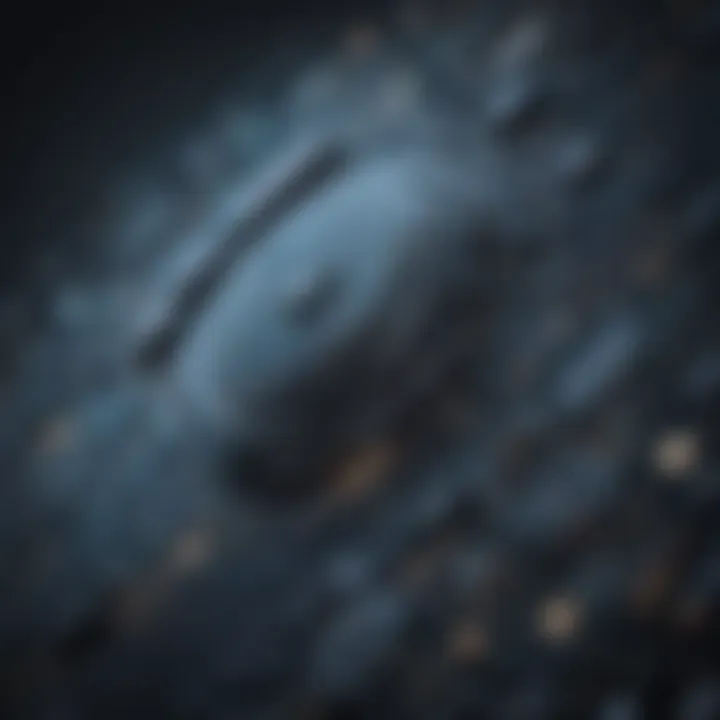
Manufacturing
Augmented data drives innovation and efficiency across the manufacturing sector by enhancing product quality, supply chain management, and predictive maintenance practices. Manufacturers utilize augmented data for demand forecasting, quality control, and process optimization. The crucial aspect of augmented data in manufacturing is its role in enabling predictive analytics for proactive problem-solving and resource allocation. While the benefits include improved production outcomes and cost savings, integrating augmented data seamlessly into existing systems poses integration challenges and workforce upskilling requirements.
Enhancing Customer Experience with Augmented Data
Personalized Recommendations
Personalized recommendations powered by augmented data transform customer engagement strategies, driving increased conversions and loyalty. Businesses leverage personalized recommendations for cross-selling, upselling, and content customization. The key characteristic of personalized recommendations is their ability to deliver relevant and timely suggestions based on individual preferences and behaviors. Despite the advantages in boosting revenue and customer satisfaction, concerns over privacy and data security underline the need for transparent data handling practices.
Predictive Analytics
Predictive analytics fueled by augmented data empowers businesses to forecast trends, mitigate risks, and seize opportunities. Organizations apply predictive analytics for demand forecasting, inventory management, and customer churn prediction. The unique feature of predictive analytics is its capacity to enable proactive decision-making and strategic planning, enhancing operational efficiency and competitive advantage. However, challenges related to data silos and model accuracy persist, calling for continuous validation and refinement.
Targeted Marketing
Targeted marketing strategies underpinned by augmented data enable businesses to tailor campaigns, enhance audience targeting, and measure campaign effectiveness. Marketers leverage augmented data for segmentation, personalization, and performance optimization. The distinctive feature of targeted marketing is its ability to deliver tailored messages to specific audience segments, driving engagement and conversion rates. Yet, issues like data privacy regulations and ad fatigue necessitate a balanced approach to maintain consumer trust and compliance with evolving standards.
Implementing Augmented Data Strategies
Implementing Augmented Data Strategies is a crucial aspect of this article, focusing on how businesses can leverage augmented data effectively. By integrating AI and machine learning techniques, businesses can enhance data quality, accuracy, and relevance in various applications. Implementing these strategies allows businesses to optimize processes, make informed decisions, and drive growth in the digital landscape. Understanding the significance of implementing augmented data strategies is paramount for businesses aiming to stay competitive and innovative in today's data-driven market.
Steps to Successfully Implement Augmented Data
Data integration
Data integration plays a pivotal role in the successful implementation of augmented data strategies. It involves combining data from multiple sources to provide a unified view for analysis and decision-making. The key characteristic of data integration lies in its ability to streamline data workflows and enhance data accessibility. This integration is a popular choice for businesses looking to improve operational efficiency and gain deeper insights from their data. While data integration offers numerous advantages such as improved data consistency and simplified data management, it may also pose challenges related to data compatibility and integration complexities.
Model development
Model development is another critical aspect of implementing augmented data strategies, contributing to enhancing data processing and predictive analytics capabilities. The key characteristic of model development is its ability to create machine learning models tailored to specific business needs and objectives. This process is a beneficial choice for businesses seeking to automate decision-making processes and improve overall performance. The unique feature of model development lies in its iterative nature, allowing for continuous improvements based on feedback and new data. While model development offers advantages like increased efficiency and accuracy, it may also present challenges related to model interpretability and scalability.
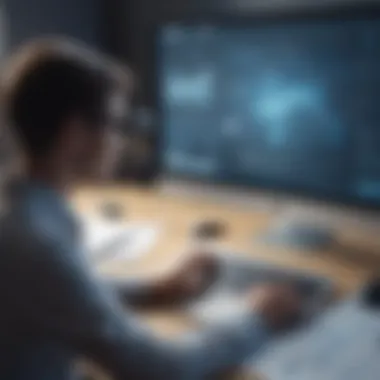
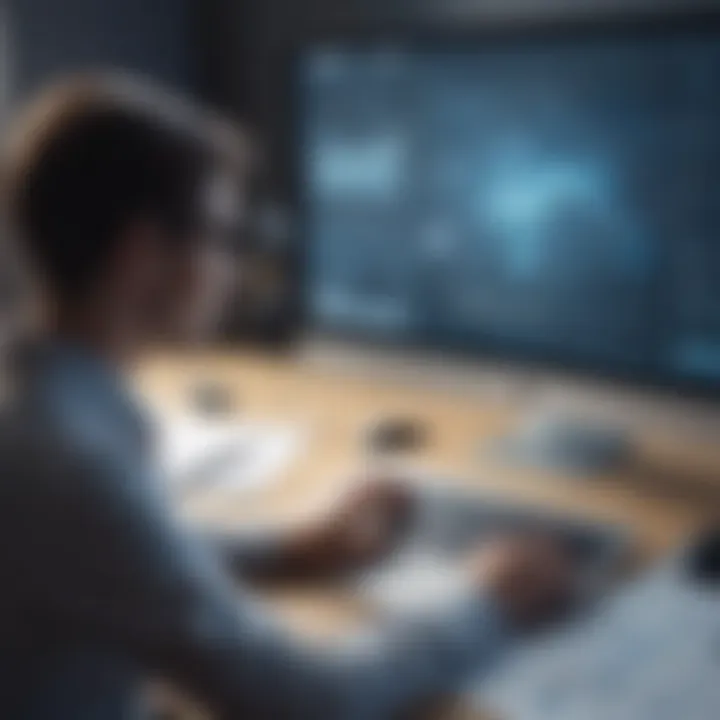
Training algorithms
Training algorithms are essential for implementing augmented data strategies as they are responsible for teaching machine learning models to make predictions based on input data. The key characteristic of training algorithms is their adaptability to varying data patterns and complexities, enabling machines to learn and evolve over time. This aspect is a popular choice for businesses aiming to leverage data-driven insights for better decision-making and business outcomes. The unique feature of training algorithms is their ability to handle large volumes of data efficiently while continuously refining predictive models. Despite offering benefits such as improved accuracy and scalability, training algorithms may encounter challenges related to computational resources and algorithm biases.
Evaluation and optimization
Evaluation and optimization are integral steps in implementing augmented data strategies as they ensure the performance and effectiveness of machine learning models. The key characteristic of evaluation and optimization is their focus on assessing model accuracy, reliability, and robustness. This process is a beneficial choice for businesses looking to fine-tune their algorithms and applications for optimal results. The unique feature of evaluation and optimization lies in their iterative nature, allowing for continuous model enhancements and adjustments. While providing advantages like improved model performance and decision-making accuracy, evaluation and optimization may also pose challenges related to data bias and overfitting issues.
Future Trends and Innovations in Augmented Data
Future Trends and Innovations in Augmented Data play a pivotal role in shaping the landscape of data utilization for businesses in the digital age. By exploring the advancements and potential developments in this field, businesses can stay ahead of the curve and capitalize on emerging opportunities. These trends encompass cutting-edge technologies and innovative approaches that can revolutionize how data is processed, analyzed, and leveraged for business success. Understanding and adapting to these trends is paramount for organizations striving to maintain a competitive edge in today's data-driven environment.
Emerging Technologies Shaping Augmented Data
Edge computing
Edge computing, a key player in the realm of augmented data, focuses on processing data closer to its source, enabling real-time insights and actions without the need to send information to a centralized server. This decentralized approach enhances data processing speed and minimizes latency, making it a preferred choice for applications requiring instant decision-making capabilities. The unique feature of Edge computing lies in its ability to enhance data processing efficiency by distributing computing tasks closer to where the data is generated. While it offers notable advantages in terms of speed and responsiveness, challenges such as data security and network connectivity may arise in certain scenarios, necessitating careful consideration in its implementation.
Explainable AI
Explainable AI stands out for its emphasis on transparency and interpretability in artificial intelligence systems. This attribute is crucial, particularly in critical decision-making processes where understanding the rationale behind AI-generated outcomes is essential. By providing clear explanations of AI's decisions and predictions, Explainable AI enhances trust and fosters confidence in automated processes. Its unique feature lies in the ability to demystify complex AI algorithms, allowing stakeholders to comprehend and validate the reasoning behind AI-driven results effectively. While Explainable AI boosts credibility and comprehension in AI applications, challenges such as model interpretability and performance trade-offs may arise, requiring careful evaluation and balancing of factors for optimal implementation.
Quantum computing
Quantum computing represents a paradigm shift in data processing capabilities, leveraging quantum phenomena to perform complex calculations at unprecedented speeds. Its key characteristic lies in harnessing quantum bits (qubits) to process vast amounts of data simultaneously, surpassing the limitations of traditional binary systems. Quantum computing's advantage stems from its potential to solve highly intricate problems efficiently, making it an attractive choice for tackling advanced analytical tasks and optimization challenges. The unique feature of Quantum computing lies in its capacity to explore multiple solutions concurrently through quantum superposition, paving the way for groundbreaking advancements in data processing and encryption. However, practical implementation challenges such as qubit error rates and scalability hurdles underscore the need for ongoing research and development to harness Quantum computing's full potential.
Potential Impact of Augmented Data on Industries
Automation of decision-making processes
Automation of decision-making processes revolutionizes how businesses formulate strategies and execute operational tasks by leveraging AI algorithms to analyze data and recommend optimal actions. The key characteristic of this approach lies in its ability to streamline decision-making workflows, minimize human biases, and accelerate response times to real-time challenges. By automating repetitive tasks and extracting insights from vast datasets, businesses can enhance operational efficiency and agility while mitigating risks associated with manual decision-making. The unique feature of Automation of decision-making processes is its capacity to scale across diverse industries and domains, driving innovation and fostering competitiveness. However, challenges related to ethical considerations, algorithmic biases, and regulatory compliance necessitate a balanced approach to ensure responsible and effective deployment.
Enhanced data security measures
Enhanced data security measures encompass a proactive strategy to safeguard digital assets, prevent unauthorized access, and mitigate cybersecurity threats in a data-centric environment. The key characteristic of this approach involves employing encryption, access controls, and monitoring mechanisms to fortify data integrity and confidentiality across all touchpoints. By implementing robust security protocols and staying abreast of evolving cyber threats, businesses can instill trust among stakeholders and uphold regulatory compliance standards. The unique feature of Enhanced data security measures lies in their adaptability to dynamic cyber threats and the ability to proactively identify and address vulnerabilities before they escalate. While enhancing data security is paramount for safeguarding sensitive information, considerations such as usability, scalability, and cost-efficiency must be balanced to achieve a resilient security posture.
Transformation of business operations
The Transformation of business operations signifies a fundamental shift in how organizations leverage augmented data to optimize processes, drive innovation, and enhance customer experiences. This approach emphasizes reimagining traditional workflows, embracing digital technologies, and fostering a data-driven culture to adapt to changing market dynamics. The key characteristic of this transformation lies in its ability to catalyze organizational agility, efficiency, and resilience through data-driven insights and predictive analytics. By integrating augmented data into core business functions, organizations can achieve operational excellence, create new revenue streams, and gain a competitive edge in the digital landscape. The unique feature of Transformation of business operations is its potential to catalyze organizational growth, foster cross-functional collaboration, and enable continuous improvement through iterative refinement and innovation. However, challenges such as organizational change management, skill gaps, and legacy system integration present hurdles that necessitate strategic planning and stakeholder alignment to ensure successful transformation.