Unveiling the Power of Cloud Data Flow on Google Cloud Platform: A Comprehensive Exploration
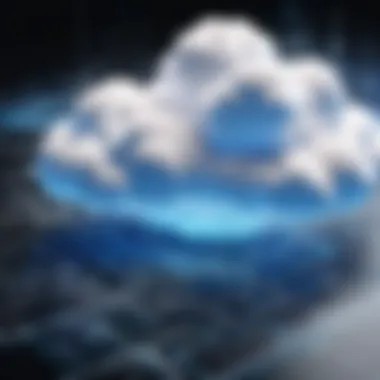
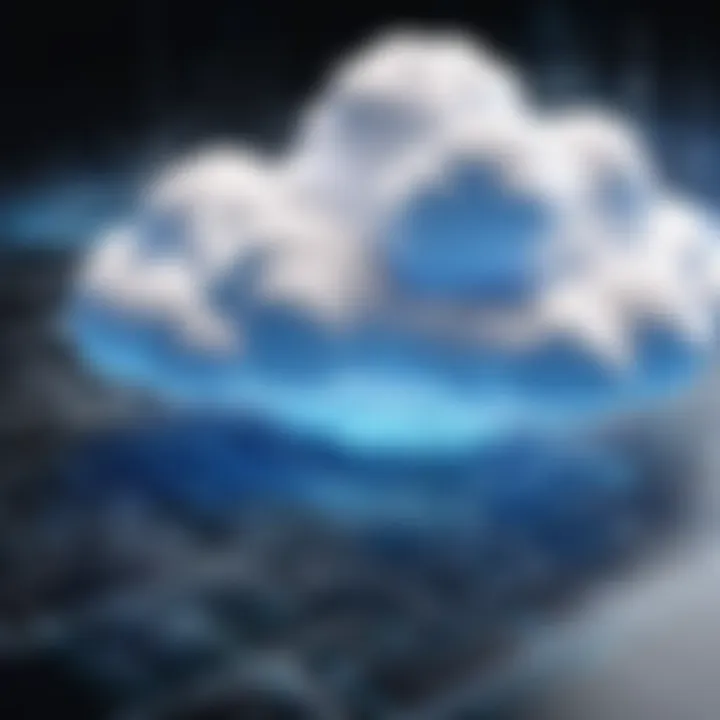
Technological Research Overview
The 21st century has witnessed an unprecedented surge in technological innovations, paving the way for groundbreaking advancements in various industries. When we delve into the realm of cloud data flow on Google Cloud Platform (GCP), we unravel a tapestry of possibilities that redefine data processing and analysis. This section serves as a gateway to understanding the recent technological innovations that have revolutionized cloud computing landscapes. By exploring the impact of these innovations on business operations, we navigate through the intricate web of data-driven decision-making mechanisms that propel organizations towards efficiency and excellence. Furthermore, we peek into the horizon to envisage future technological trends that could potentially shape the trajectory of cloud data flow technologies.
Data Analytics in Business
Cybersecurity Insights
Amidst the digital landscape's relentless evolution, cybersecurity remains a paramount concern for organizations worldwide. Within the context of cloud data flow on Google Cloud Platform, an exploration of cybersecurity insights becomes imperative. This section offers a meticulous analysis of the ever-evolving threat landscape, shedding light on emerging cybersecurity challenges and vulnerabilities. By delving into best practices for cybersecurity, organizations equip themselves with the necessary armor to safeguard against cyber threats effectively. Moreover, we traverse the terrain of regulatory compliance in cybersecurity, emphasizing the pivotal role of adhering to stringent regulations in safeguarding sensitive data and bolstering trust.
Artificial Intelligence Applications
The integration of artificial intelligence (AI) applications reshapes conventional business paradigms, ushering in an era of automation and efficiency. Expanding our discourse on cloud data flow to encompass AI applications on Google Cloud Platform, we navigate through the realm of AI in business automation. By exploring AI algorithms and applications, we unearth the transformative potential of AI in optimizing processes and enhancing user experiences. Furthermore, ethical considerations in AI underscore the significance of responsible AI deployment, fostering a landscape of transparency and accountability in AI-driven solutions.
Industry-Specific Research
While the implications of cloud data flow on Google Cloud Platform reverberate across diverse sectors, industry-specific research sheds light on tailored technological advancements. In the finance sector, tech research unveils innovative solutions that streamline financial processes and optimize decision-making mechanisms. Healthcare technological advancements harness the power of cloud data flow to revolutionize patient care delivery and clinical outcomes. On the frontier of retail industry tech solutions, organizations leverage cloud data flow to enhance customer experiences and drive personalized engagements, redefining the retail landscape.
Prelims to Cloud Data Flow
Cloud Data Flow on Google Cloud Platform is a crucial element in modern computing. Understanding the basics of data flow and its application in cloud computing is essential for maximizing data processing and analysis efficiency. This section will delve deep into the intricacies of Cloud Data Flow, highlighting its significance and benefits.
Defining Cloud Data Flow
Conceptual Overview
Cloud Data Flow provides a structured approach to data processing, facilitating seamless workflows and scalability. Its key characteristic lies in its ability to process data in real-time, enabling swift decision-making based on up-to-date information. This feature is particularly advantageous for businesses seeking timely insights from their data. However, the extensive resources required for real-time processing may pose challenges in terms of cost and infrastructure.
Key Components
The components of Cloud Data Flow, including parallel processing and resource allocation, play a vital role in optimizing performance. Parallel processing allows for efficient data handling by dividing tasks among multiple processing units. Resource allocation ensures that the necessary resources are allocated dynamically, enhancing overall system efficiency. While these components improve data processing speed and reliability, improper configuration can lead to resource wastage and underutilization.
Importance in Modern Computing
Real-time Data Processing
Real-time data processing is a critical aspect of modern computing, enabling businesses to react promptly to changing market trends and consumer behavior. With Cloud Data Flow, real-time processing becomes more accessible, providing continuous data insights for informed decision-making. The ability to process data instantaneously enhances operational efficiency, but it also requires robust infrastructure to support the constant influx of data.
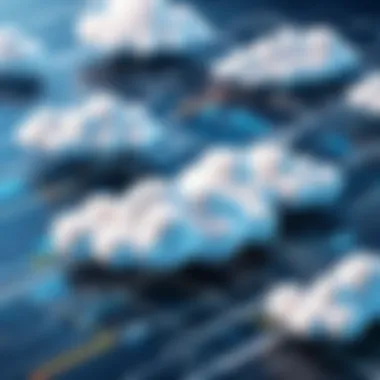
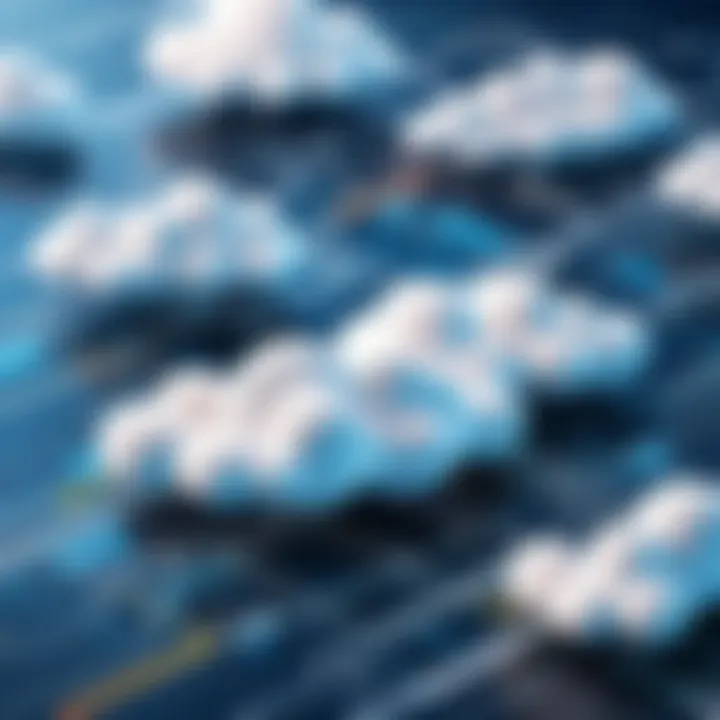
Scalability Benefits
Scalability is a key advantage of Cloud Data Flow, allowing organizations to expand their data processing capabilities as needed. The ability to scale up or down based on demand ensures optimal resource utilization and cost-effectiveness. Scalability benefits enable businesses to handle varying workloads without over-provisioning resources, thus enhancing operational flexibility. However, achieving seamless scalability requires careful monitoring and optimization to avoid unnecessary expenses.
Comparison with Traditional Data Processing
Efficiency
Cloud Data Flow surpasses traditional data processing methods in terms of efficiency by offering parallel processing and resource allocation features. These functionalities streamline data workflows and minimize processing times, resulting in higher operational efficiency. The efficiency of Cloud Data Flow lies in its ability to handle large datasets with speed and accuracy, enhancing overall data processing capabilities. However, ensuring optimal efficiency requires continual performance tuning and monitoring to adapt to changing workload requirements.
Flexibility
The flexibility of Cloud Data Flow grants organizations the freedom to adjust processing resources based on workload fluctuations. This adaptability ensures that data processing tasks are completed efficiently without resource bottlenecks or delays. Cloud Data Flow's flexibility allows businesses to scale operations up or down seamlessly, responding to market dynamics with agility. Nonetheless, maintaining flexibility necessitates ongoing evaluation of resource usage patterns to optimize performance and minimize operational costs.
Exploring Google Cloud Platform (GCP)
Exploring Google Cloud Platform (GCP) in the context of unleashing the power of cloud data flow is a pivotal aspect of this article. By delving into the intricacies of GCP, we illuminate the pathway for optimizing data processing and analysis on the cloud. The significance of comprehending GCP lies in its foundational role in enabling seamless data management and utilization for businesses across diverse sectors. As we explore GCP further, we uncover its core services and integration capabilities with cloud data flow, shedding light on the transformative potential it holds for modern computing landscapes.
Overview of GCP
Core Services
When dissecting the core services of GCP, a standout feature emerges in its robust foundation for scalable and secure cloud operations. GCP's core services encompass a wide array of tools and functionalities designed to streamline processes and enhance efficiency in data handling. The key characteristic of GCP's core services lies in its adaptability to varying business needs, making it a versatile and reliable choice for harnessing the power of cloud data flow. Additionally, the unique feature of seamless integration within the GCP ecosystem offers substantial advantages in terms of agility and performance optimization. While there may be minor drawbacks related to complexity for beginners, the benefits of GCP's core services far outweigh any initial challenges, positioning it as a frontrunner in cloud computing solutions.
Advantages
In exploring the advantages that GCP brings to the table, a compelling narrative unfolds around its unparalleled reliability and scalability factors. The key characteristic of GCP's advantages lies in its ability to provide a high level of flexibility and customization, catering to unique project requirements with ease. Evident in its seamless integration with cloud data flow, GCP's advantages manifest through streamlined data processing workflows and advanced analytics capabilities. The unique feature of GCP's advantages is its focus on performance optimization and cost-efficiency, making it a must-have for organizations looking to drive innovation through cloud computing. While there may be slight limitations regarding learning curves for intricate features, the advantages of GCP stand as a testament to its position as a frontrunner in the cloud services domain.
Optimizing Data Flow on GCP
Optimizing Data Flow on Google Cloud Platform (GCP) plays a pivotal role in maximizing the efficiency and effectiveness of data processing activities. By delving into various performance enhancement techniques and cost-efficient strategies, businesses can streamline their operations and achieve unparalleled results. With a focus on enhancing data flow mechanisms, this section explores key elements such as parallel processing and resource allocation to elevate the data processing capabilities on GCP.
Performance Enhancement Techniques
Parallel Processing
Parallel processing emerges as a fundamental technique in optimizing data flow on GCP. It revolutionizes the speed and efficiency of data processing tasks by enabling multiple operations to execute simultaneously. This parallel execution significantly reduces the processing time for complex computations, enhancing the overall throughput of data pipelines. One of the key characteristics of parallel processing is its ability to divide tasks into smaller sub-tasks that can be processed concurrently, leveraging the full computational power of the underlying infrastructure. Despite its advantages in improving performance and scalability, parallel processing may pose challenges in managing dependencies and ensuring consistent results across parallel threads.
Resource Allocation
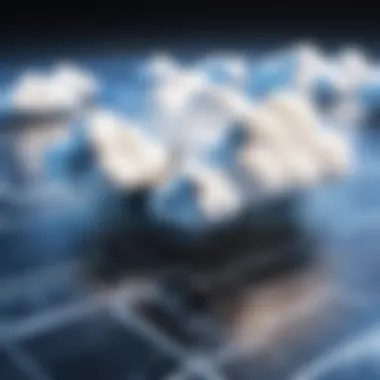
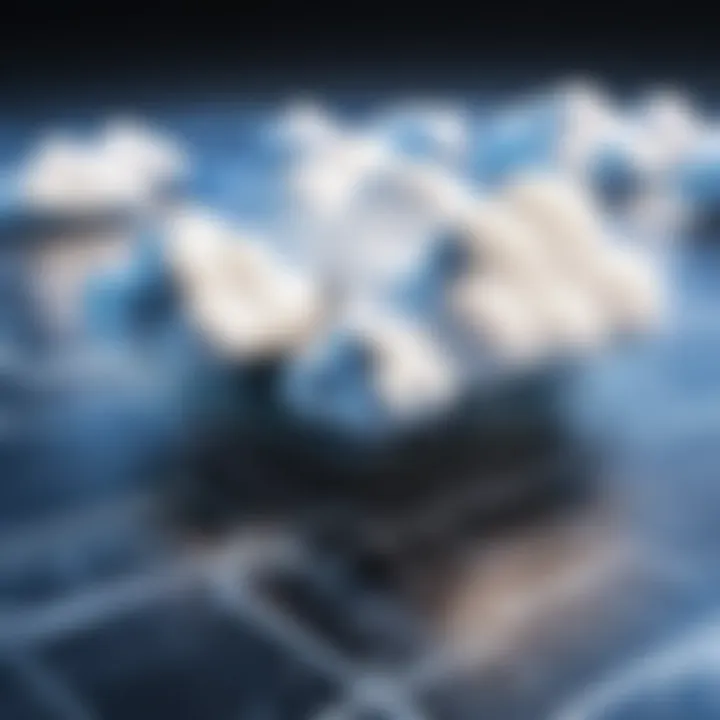
Resource allocation stands as a critical aspect in optimizing data flow on GCP, influencing the system's performance and cost-effectiveness. Effective resource allocation involves allocating computing resources such as CPU and memory according to the workload demands, ensuring optimal utilization and performance efficiency. By dynamically allocating resources based on the data processing requirements, businesses can prevent underutilization or overprovisioning of resources, thereby optimizing the cost-effectiveness of data processing operations. The unique feature of resource allocation lies in its ability to adapt to fluctuating workloads in real-time, maintaining an optimal balance between resource utilization and operational costs.
Cost-Efficient Strategies
Resource Utilization Optimization
Resource Utilization Optimization emerges as a key strategy in enhancing cost-efficiency and performance in data flow on GCP. By optimizing resource utilization, businesses can maximize the output of data processing tasks while minimizing resource wastage. This strategy involves continuous monitoring of resource usage, identifying bottlenecks, and optimizing resource allocation to enhance overall system performance. The unique feature of resource utilization optimization lies in its ability to leverage insights from resource usage patterns to fine-tune resource allocation, ensuring efficient utilization of computing resources while minimizing costs.
Auto-scaling Features
Auto-scaling features offer dynamic scalability options, allowing businesses to automatically adjust resource capacity based on workload fluctuations. This flexibility ensures optimal resource allocation during peak loads, preventing performance degradation or resource shortages. The key characteristic of auto-scaling features is their ability to seamlessly adapt to varying workloads without manual intervention, enhancing operational efficiency and cost-effectiveness. While auto-scaling features provide agility and scalability benefits, improper configurations may lead to unexpected cost escalations or resource inefficiencies, highlighting the need for careful planning and monitoring.
Implementing Data Flow Solutions
As we delve into the intricate realm of cloud data flow on Google Cloud Platform (GCP), the section of Implementing Data Flow Solutions stands as a crucial pillar in this article. It encapsulates the practical application of theoretical knowledge, bridging the gap between concept and implementation. Understanding how to effectively implement data flow solutions is paramount in maximizing the efficiency of data processing and analysis within the cloud computing landscape. By exploring use cases and best practices, readers can grasp the nuances of executing data flow solutions in real-world scenarios.
Use Cases
Real-time Analytics
Real-time analytics, a cornerstone of contemporary data processing, plays a pivotal role in facilitating instantaneous insights and decision-making. Its ability to process data at the moment of input ensures that businesses can respond swiftly to changing trends and scenarios. The distinctive feature of real-time analytics lies in its timely delivery of actionable intelligence, enabling organizations to optimize operations and enhance customer experiences. While offering unparalleled agility, real-time analytics may pose challenges in terms of data volume and processing speed, warranting meticulous attention to infrastructure and algorithms for optimal performance.
Data Migration
Data migration, a fundamental aspect of modern data management, holds significance in the context of implementing data flow solutions on GCP. It involves the seamless transfer of data from one system to another, ensuring continuity and accuracy in information flow. The key characteristic of data migration lies in its ability to enable transition without disruption, consolidating data across platforms for unified access. However, challenges such as data integrity and compatibility issues may arise during the migration process, necessitating thorough planning and validation mechanisms for successful outcomes.
Best Practices
Data Flow Design Patterns
Data flow design patterns serve as architectural blueprints for structuring data pipelines efficiently and cohesively. By establishing standardized methods for data transformation and movement, design patterns enhance the scalability and maintainability of data flow solutions. Their unique feature lies in promoting reusability and standardization, reducing development time and complexity. While advantageous in promoting consistency and efficiency, design patterns require careful consideration of specific use cases and customization to ensure optimal functionality.
Error Handling
Error handling mechanisms play a critical role in maintaining data integrity and operational stability within data flow solutions. By implementing robust error detection and recovery strategies, organizations can mitigate risks associated with data inconsistencies and processing failures. The key characteristic of error handling lies in its ability to identify and address deviations from expected outcomes, ensuring continuous operation and error resolution. Despite its indispensable role, error handling may introduce overhead in terms of resource utilization and system complexity, necessitating a balanced approach towards fault tolerance and performance optimization.
Security Considerations for Cloud Data Flow
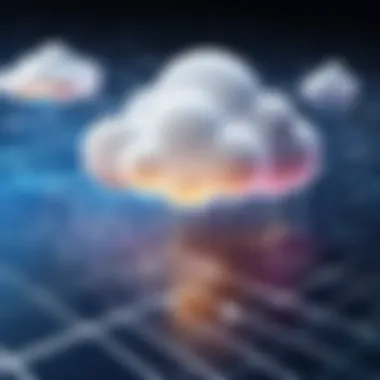
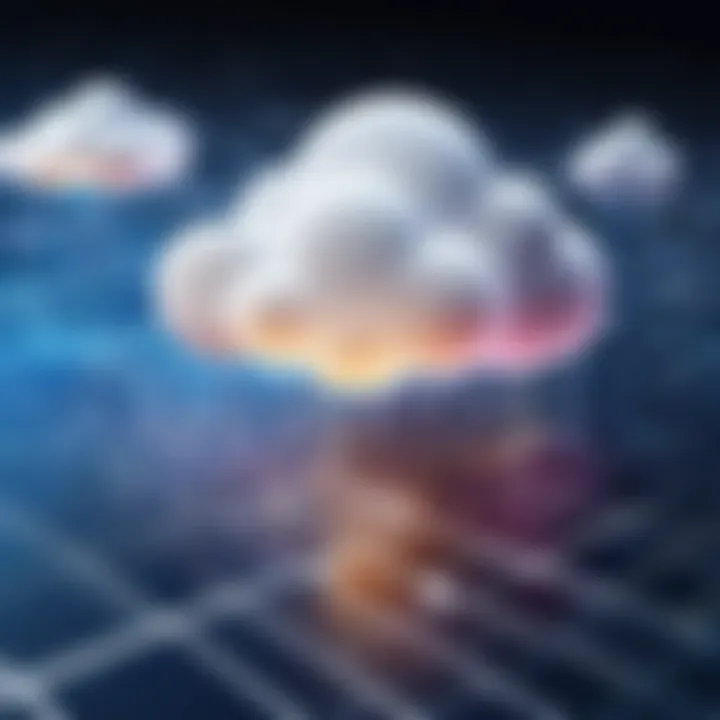
In the ever-evolving landscape of cloud computing, security considerations stand paramount as organizations harness the power of data flow on Google Cloud Platform (GCP). Ensuring the integrity, confidentiality, and availability of data is crucial in this digital era where cyber threats loom large. Security Considerations for Cloud Data Flow encompass a multifaceted approach to safeguarding sensitive information amidst data processing and analysis. By implementing robust security measures, businesses can mitigate risks and uphold trust in their cloud infrastructure.
Data Encryption
End-to-End Encryption
End-to-End Encryption emerges as a pivotal aspect within Security Considerations for Cloud Data Flow. This encryption methodology secures data throughout its journey from source to destination, thwarting unauthorized access attempts. The distinctive feature of End-to-End Encryption lies in its ability to encode data in transit and at rest, ensuring a comprehensive shield against data breaches. End-to-End Encryption serves as a stalwart defender of data integrity and confidentiality, making it a preferred choice for organizations leveraging cloud data flow on GCP. While its advantages are clear in bolstering data security, potential drawbacks may include increased computational overhead for encryption and decryption processes.
Key Management
Within the realm of Security Considerations for Cloud Data Flow, Key Management assumes a critical role in safeguarding encryption keys vital for data protection. Effective Key Management entails the secure generation, distribution, and storage of cryptographic keys, ensuring seamless encryption and decryption operations. The key characteristic of Key Management lies in its capacity to centralize key administration, simplifying key rotation and access control. Its unique feature lies in enhancing data security by enforcing strict key access policies and comprehensive key lifecycle management. Despite its advantages in fortifying data encryption, complexities may arise in managing a large number of keys and ensuring key availability without compromising security.
Access Control Policies
Role-Based Access
Access Control Policies play a pivotal role in governing user permissions within Cloud Data Flow environments. Role-Based Access delineates user roles based on responsibilities and authorization levels, limiting unauthorized access to sensitive data. The key characteristic lies in its granularity, allowing organizations to tailor access rights according to specific job functions. This tailored approach enhances data security by restricting unnecessary data exposure, making Role-Based Access an essential choice for mitigating insider threats. The unique feature of Role-Based Access lies in its scalability, enabling seamless management of access permissions as organizational structures evolve. While its advantages are evident in ensuring data integrity, challenges may surface in defining precise role assignments and managing access complexities.
Audit Trails
In the realm of Security Considerations for Cloud Data Flow, Audit Trails serve as a vital mechanism for monitoring and tracking user activities. These trails provide a comprehensive record of data access, modifications, and system events, aiding in forensic investigations and compliance adherence. The key characteristic of Audit Trails lies in their transparency, offering organizations insights into data interactions and potential security incidents. Its unique feature lies in enhancing accountability and traceability, empowering organizations to identify security breaches promptly. Despite its advantages in bolstering data governance, complexities may arise in managing and analyzing vast volumes of audit data efficiently.
Future Trends and Innovations
In the landscape of technology and data processing, Future Trends and Innovations play a pivotal role in shaping the direction of advancements. Looking ahead is not merely about speculation but a strategic move towards embracing cutting-edge solutions. In this article, Future Trends and Innovations are a beacon guiding readers towards the upcoming waves of progress in cloud data flow on Google Cloud Platform. By delving into trends like AI Integration and Blockchain Applications, we pave the way for a deeper understanding of how technology evolves and impacts our digital ecosystem.
AI Integration
Machine Learning Models
Machine Learning Models form the cornerstone of AI Integration in modern data processing frameworks. These models are designed to analyze data patterns, learn from them, and make predictions without being explicitly programmed. The beauty of Machine Learning lies in its ability to adapt and improve over time, enhancing the accuracy and efficiency of data analysis. In this article, the discussion revolves around the role of Machine Learning Models in enhancing the predictive capabilities of cloud data flow on Google Cloud Platform. The unique feature of these models lies in their capacity to handle vast amounts of data swiftly, providing valuable insights for decision-making. While they offer unparalleled benefits in terms of automated analysis and decision support, challenges like data bias and model interpretability remain areas of concern that readers need to consider.
Predictive Analytics
Predictive Analytics stands at the forefront of harnessing the power of data to forecast future trends and behaviors. By utilizing statistical algorithms and machine learning techniques, Predictive Analytics enables organizations to anticipate outcomes based on historical data patterns. Within the realms of this article, Predictive Analytics emerges as a vital component of strategic planning and resource optimization in cloud data flow implementations on Google Cloud Platform. Its key characteristic lies in its proactive approach towards identifying trends and anomalies, empowering businesses with strategic foresight. While facilitating data-driven decision-making, Predictive Analytics comes with the challenge of data quality and model accuracy, urging readers to balance the benefits with potential limitations.
Blockchain Applications
Smart Contracts
Smart Contracts revolutionize traditional contract processes by automating and enforcing agreements through self-executing codes. These contracts eliminate the need for intermediaries, enhancing transparency and security in transactions. In the context of this article, Smart Contracts are highlighted for their efficiency and reliability in data transactions within cloud data flow operations on Google Cloud Platform. The key characteristic of Smart Contracts lies in their tamper-resistant nature and the ability to execute predefined actions automatically. While their advantages include reduced transaction costs and streamlined processes, issues such as smart contract bugs and legal enforceability present challenges that demand attention.
Cryptocurrency Transactions
Cryptocurrency Transactions redefine the way financial transactions are conducted, utilizing digital assets for secure and seamless payments. These transactions operate on decentralized blockchain networks, ensuring transparency and immutability of transaction records. Expounded within this article, Cryptocurrency Transactions showcase their relevance in enhancing data security and transactional efficiency in cloud data flow environments on Google Cloud Platform. The key characteristic of cryptocurrency transactions is their decentralized nature, enabling peer-to-peer exchanges without intermediaries. While offering benefits like global reach and reduced fraud risks, concerns around regulatory compliance and market volatility call for a balanced view when integrating cryptocurrency transactions into data flow solutions.
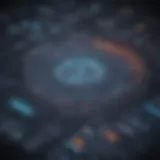
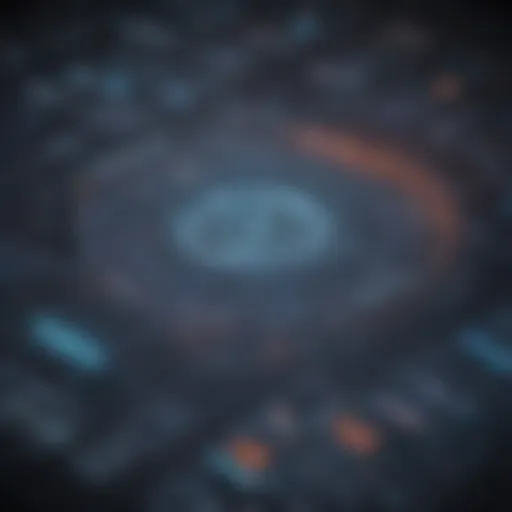