Unraveling the Intricacies of the Customer Data Platform Data Model
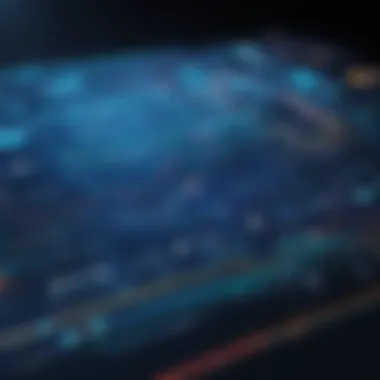
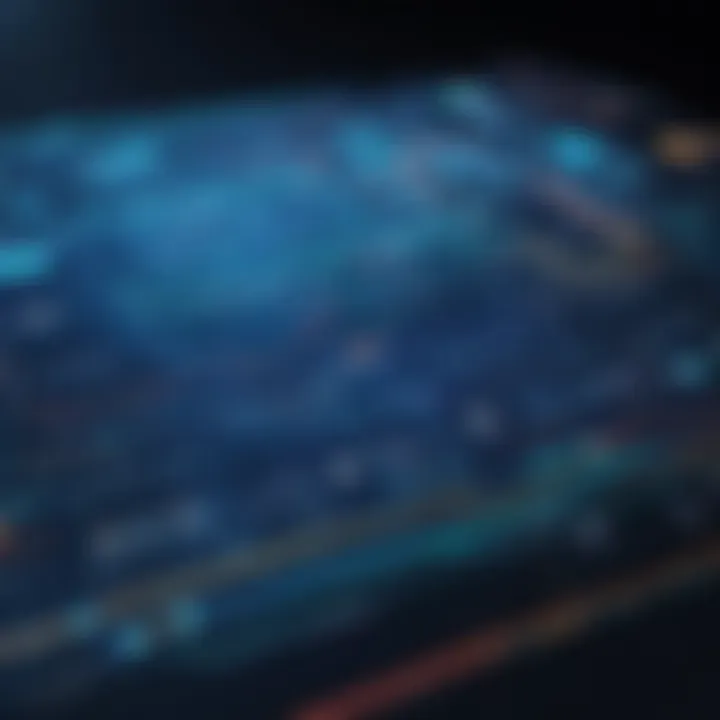
Technological Research Overview
Customer data platforms (CDPs) play a vital role in modern business operations, revolutionizing the way companies collect, manage, and utilize customer data. Recent technological innovations have seen CDP data models becoming increasingly sophisticated, accommodating vast amounts of data from various sources. These advancements have cascading effects on business operations, offering businesses unprecedented insights into customer behavior and preferences. The future of CDP data models promises even greater integration with emerging technologies like AI and machine learning.
Data Analytics in Business
Understanding the importance of data analytics is crucial in leveraging the power of a customer data platform data model. Through tools for data analysis, businesses can extract meaningful insights from the influx of data, guiding strategic decision-making processes. Real-world case studies showcasing data-driven decisions underscore the tangible benefits of implementing robust analytics strategies based on a solid data model.
Cybersecurity Insights
With the proliferation of data collection and analysis, cybersecurity becomes paramount. A thorough threat landscape analysis is necessary to identify potential vulnerabilities within the customer data platform data model. Implementing best practices for cybersecurity ensures that sensitive customer information remains secure, while adhering to regulatory compliance safeguards against data breaches.
Artificial Intelligence Applications
The integration of artificial intelligence into customer data platforms paves the way for new possibilities in business automation. AI algorithms drive predictive analytics and personalized customer experiences, elevating operational efficiency. Ethical considerations in AI implementation underscore the importance of transparency and fairness in leveraging AI within the data model.
Industry-Specific Research
Different industries benefit from tailored technological solutions embedded in customer data platform data models. The finance sector leverages advanced tech research to streamline financial processes and enhance customer service. Similarly, healthcare advancements harness the power of data models to improve patient care and medical research. Retail industries employ tech solutions to optimize inventory management and deliver personalized shopping experiences.
Prelude to Customer Data Platform Data Model
Indian origin writers possess a unique ability to craft compelling narratives that resonate with discerning audiences. In this article, we embark on an exploration of the Customer Data Platform Data Model, delving deep into its intricate structure, functionalities, and profound implications for businesses. By dissecting the key components and best practices associated with this data model, readers will be equipped with invaluable insights to harness data effectively, steering strategic decisions and elevating customer experiences to new heights.
Understanding the Fundamentals
As we unravel The Role of Customer Data Platforms within the overarching realm of data modeling, it becomes apparent that these platforms serve as the linchpin for consolidating and analyzing vast amounts of customer data. The key characteristic of Customer Data Platforms lies in their ability to orchestrate diverse data sources into a unified view, enabling organizations to extract actionable insights for enhanced decision-making. Despite their complexities, these platforms offer unprecedented agility and scalability, empowering businesses to stay ahead in the data-driven landscape.
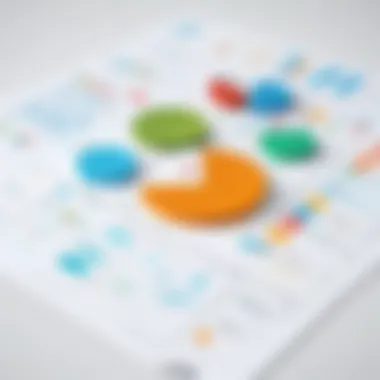
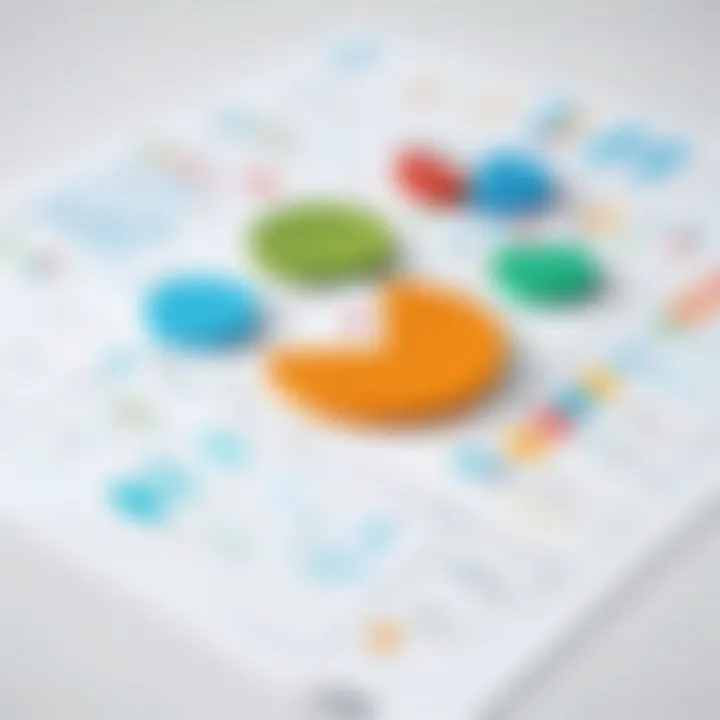
In exploring the Data Model Overview, we uncover a panoramic view of the foundational framework that underpins customer data platforms. The focal point of this overview is the structured representation of data entities, relationships, and processes within an organization. This structured approach not only facilitates seamless data utilization but also lays the groundwork for advanced analytics and predictive modeling, propelling businesses towards data-driven success.
Turning our attention to the pivotal aspect of Importance of Data Modeling, we encounter the cornerstone of efficient data management practices. Data modeling serves as the compass that steers organizations towards a clearer understanding of their data assets, ensuring structural integrity, integration, and quality control. By embracing robust data modeling strategies, businesses can harness the full potential of their data assets, fostering informed decision-making and strategic agility.
Key Components
Delving deeper into the realms of Data Collection Methods unveils a tapestry of innovative techniques employed to gather, standardize, and store data efficiently. The crux of data collection methods lies in their adaptability to diverse data sources and formats, providing organizations with a comprehensive data repository. This versatility not only enhances data validity and completeness but also fortifies organizations against data silos and inconsistencies.
Exploring the intricate landscape of Data Storage Architecture reveals a nuanced interplay between data repositories, processing systems, and data access protocols. The key characteristic of data storage architecture lies in its ability to optimize data retrieval and storage processes, ensuring rapid access to crucial insights. This streamlined approach not only enhances data resilience but also fosters a secure data environment, safeguarding critical information from potential threats.
Segueing into the realm of Data Integration Strategies, we unearth a trove of methodologies employed to harmonize disparate data sources and systems. The essence of data integration strategies lies in their ability to create a cohesive data ecosystem, enabling seamless data flow across organizational interfaces. Through strategic integration initiatives, organizations can enhance data consistency, accuracy, and accessibility, laying the foundation for agile decision-making and operational excellence.
Benefits of a Robust Data Model
Enter the realm of Enhanced Customer Insights, where the power of robust data models shines through in generating profound customer understanding. The key characteristic of enhanced customer insights lies in their ability to unveil intricate consumer behaviors, preferences, and sentiments, equipping organizations with unparalleled personalization capabilities. By leveraging these insights, businesses can tailor their products and services to meet individual customer needs, fostering long-lasting relationships and brand loyalty.
Embark on a journey through Improved Personalization, where the finesse of data modeling crafts bespoke customer experiences tailored to individual preferences. The crux of improved personalization lies in its seamless integration of customer data, enabling dynamic segmentation and targeted marketing initiatives. This personalized approach not only enhances customer engagement but also drives customer loyalty and advocacy, propelling businesses towards sustainable growth.
Uncover the transformative potential of Streamlined Marketing Campaigns, where data-driven strategies converge to create impactful marketing initiatives. The key characteristic of streamlined marketing campaigns lies in their ability to optimize marketing efforts through targeted segmentation, personalized messaging, and real-time analytics. By harnessing the power of data modeling in marketing campaigns, organizations can drive ROI, optimize campaign performance, and achieve unprecedented levels of customer engagement.
Optimizing Data Model for Business Success
Optimizing Data Model for Business Success is a pivotal aspect of this article. It serves as the bedrock for businesses aiming to harness the potential of their data infrastructure efficiently. By optimizing the data model, companies can streamline operations, improve decision-making processes, and enhance the overall customer experience. This section delves into the best practices and considerations critical to achieving success through data optimization.
Best Practices
Data Quality Management
Data Quality Management plays a crucial role in ensuring the integrity and accuracy of data within the customer data platform. By focusing on data quality, organizations can enhance the reliability of their insights and decision-making processes. The meticulous attention to detail in data quality management is paramount for deriving meaningful and actionable conclusions from the vast amounts of data at hand. While data quality management may entail rigorous processes, its impact on the overall data model effectiveness cannot be overstated.
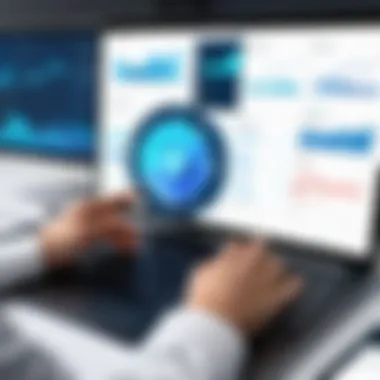
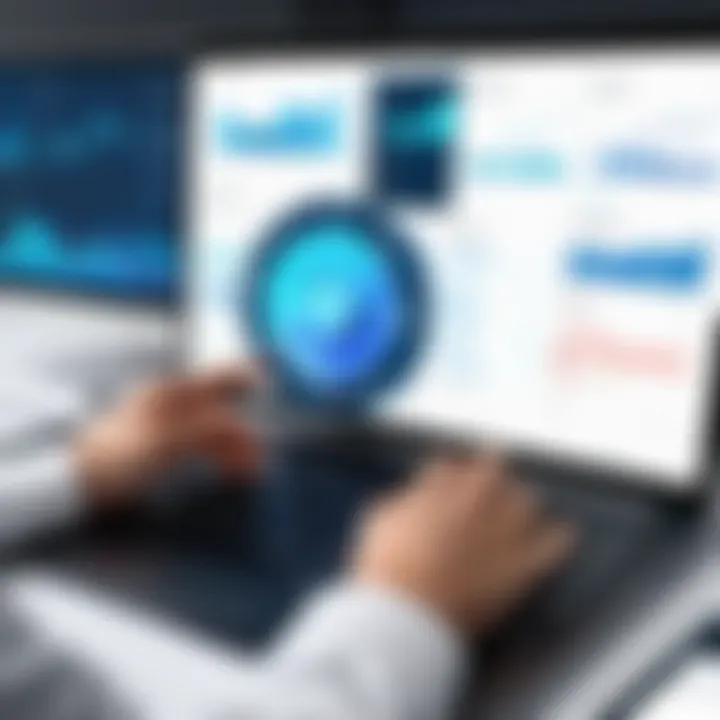
Scalability and Flexibility
Scalability and Flexibility are key factors to consider when optimizing the data model for business success. The ability of the data infrastructure to scale with increasing volumes of data while remaining flexible enough to adapt to changing business needs is paramount. Scalability ensures that the data model can grow seamlessly as the business expands, while flexibility allows for adjustments to be made without disrupting operations. Balancing scalability and flexibility is crucial for long-term success in data management.
Security and Compliance Measures
In the realm of data security, implementing robust security and compliance measures is indispensable. Protecting sensitive customer data and ensuring compliance with relevant regulations are non-negotiable aspects of data optimization. Security breaches can have detrimental effects on a business's reputation and finances, making stringent security and compliance measures vital components of the data model optimization process.
Implementing a Data Governance Framework
Establishing a robust data governance framework is instrumental in maintaining data quality and integrity. By defining clear data policies, organizations can ensure that data is used ethically, securely, and in alignment with business objectives. Data accuracy is a cornerstone of data governance, necessitating measures to verify and validate data across the organization. Enforcing data privacy standards is equally crucial, especially in an era where data privacy is a significant concern for customers and regulatory bodies. By prioritizing data governance, businesses can build trust with their customers and stakeholders while maximizing the value of their data assets.
Challenges and Solutions
Exploring the challenges and solutions within the domain of the Customer Data Platform Data Model is crucial for businesses aiming to optimize their data operations. By addressing pressing issues and devising effective strategies, organizations can enhance their data management practices and overall performance. This section dives deep into key elements like data quality, governance, and silos, offering insights for decision-makers to navigate these challenges successfully.
Data Quality Issues
Within the realm of data quality issues, certain factors significantly impact the efficacy of the customer data platform data model. The first crucial aspect to consider is the presence of inconsistent data sources. These disparate sources of information contribute to the overall complexity and accuracy of data within the model. Highlighting the variability and discrepancies stemming from inconsistent sources sheds light on the challenges faced by organizations in maintaining data integrity and reliability. Although popular for diverse data inputs, inconsistent data sources pose challenges in ensuring cohesive data processing and analysis within this context.
Another critical data quality issue to address is poor data governance. Poor governance practices hinder the effective utilization of data assets, resulting in compromised decision-making processes. By emphasizing the lack of standardized protocols and policies governing data management, we recognize the dire consequences of inadequate data governance on organizational performance. While being a prevalent issue, poor data governance underscores the necessity for streamlined processes and frameworks to regulate data handling and storage within the customer data platform data model.
Lastly, the presence of data silos emerges as a significant concern impacting data quality within the model. These isolated repositories of information restrict data flow and accessibility, leading to inefficiencies in data utilization and analysis. Highlighting the detrimental effects of data silos on operational efficacy and strategic insights underscores the importance of breaking down these barriers for seamless data integration and utilization. Despite being common in organizational settings, data silos highlight the imperative need for holistic data management and integration strategies.
Mitigation Strategies
To combat the challenges posed by data quality issues, implementing robust mitigation strategies is essential for organizations leveraging the customer data platform data model. The first strategy entails implementing data cleaning processes to enhance data quality and consistency. By emphasizing regular data cleansing routines, organizations can ensure data accuracy and reliability, supporting informed decision-making and analysis. Despite potential drawbacks such as resource intensiveness, the benefits of data cleaning processes in refining data quality outweigh the associated costs.
Another vital mitigation strategy involves deploying automation tools to streamline data processing and analysis. Automation enhances operational efficiency by reducing manual intervention in data tasks, improving data processing speed and accuracy. Highlighting the scalability and speed benefits of automation tools underscores their relevance in optimizing data operations within the customer data platform data model. While introducing automation may require initial investments, the long-term benefits in operational efficiency and data accuracy justify its integration.
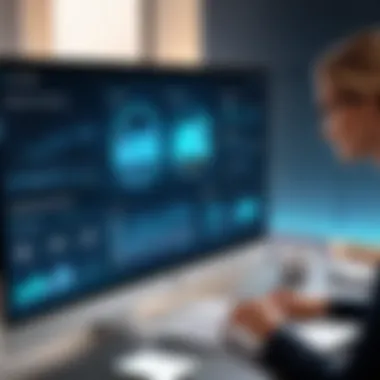
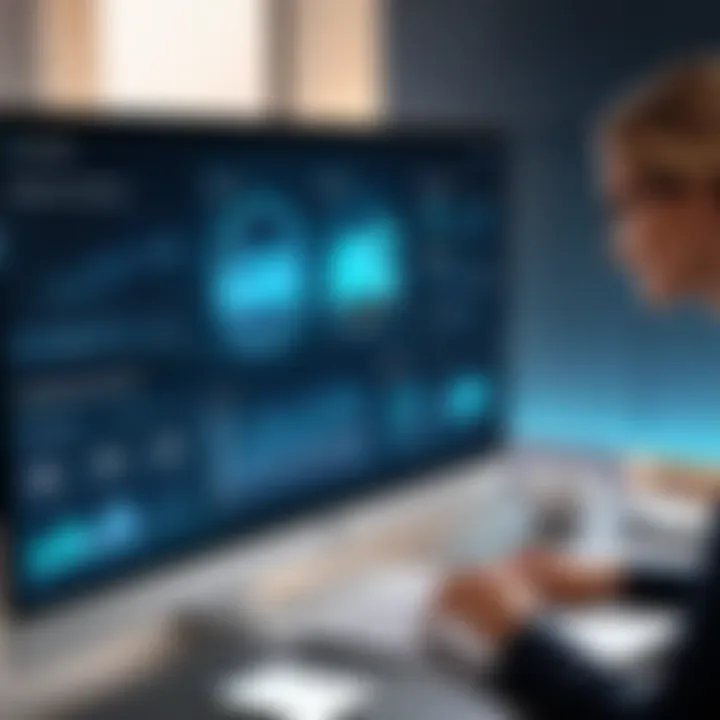
Enhancing cross-department collaboration serves as a final crucial mitigation strategy to address data quality issues within the model. By fostering collaboration across departments, organizations can promote data sharing and transparency, ensuring a unified approach to data management. Emphasizing the communication and knowledge-sharing aspects of cross-department collaboration showcases its role in breaking down organizational silos and promoting data integration. Despite potential challenges in aligning departmental objectives, the collective benefits of enhanced collaboration in improving data quality and operational efficiency are substantial.
Future Trends in Data Modeling
In this intricate exploration of the customer data platform data model, it is crucial to consider the future trends shaping the landscape of data modeling. Understanding the evolution of data modeling is key for businesses striving to stay ahead of the curve. By focusing on the advancements in AI and machine learning integration, companies can harness powerful tools to drive strategic decision-making and enhance customer experiences.
AI and Machine Learning Integration
Predictive Analytics
Delving into the realm of predictive analytics within the data modeling sphere, we encounter a potent tool for forecasting future trends and customer behavior patterns. The essence of predictive analytics lies in its ability to unravel hidden insights from data, enabling organizations to anticipate market shifts and tailor their strategies accordingly. The allure of predictive analytics lies in its predictive accuracy and capacity to transform data into actionable insights, facilitating informed decision-making in the dynamic business landscape.
Behavioral Segmentation
Unpacking the significance of behavioral segmentation, we uncover a methodology that leverages customer behavior data to create targeted marketing strategies and personalized customer interactions. By categorizing customers based on their behaviors and preferences, businesses can tailor their offerings to meet individual needs effectively. The strategic advantage of behavioral segmentation lies in its ability to enhance customer engagement and drive impactful marketing campaigns, fostering sustainable customer relationships.
Automated Decision-Making
Exploring the realm of automated decision-making, we encounter a revolutionary approach to streamlining operational processes and enhancing efficiency. Automated decision-making empowers businesses to make data-driven decisions in real-time, optimizing resource allocation and response mechanisms. The distinctive feature of automated decision-making lies in its capability to minimize human errors and maximize operational speed, offering organizations a competitive edge in an increasingly digital ecosystem.
Data Monetization Strategies
Unveiling the realm of data monetization strategies, it is essential to understand the mechanisms through which organizations can extract value from their data assets. By capitalizing on innovative revenue generation streams, businesses can diversify their income sources and unlock untapped growth opportunities.
Creating New Revenue Streams
Exploring the concept of creating new revenue streams through data monetization, organizations can tap into novel ways of monetizing their data assets. By identifying new market opportunities and consumer niches, businesses can leverage their data resources to generate additional revenue streams effectively. The appeal of creating new revenue streams lies in its potential to drive incremental revenue and expand business horizons through innovative data utilization.
Enhancing Customer Value Proposition
Delving into the realm of enhancing customer value proposition, businesses can elevate their offerings by providing personalized and tailored products and services. By refining the customer experience through data-driven insights, organizations can cultivate loyal customer relationships and enhance brand loyalty. The strategic advantage of enhancing customer value proposition lies in its power to differentiate businesses in competitive markets, resonating with customers on a deeper level and fostering long-term loyalty.
Unlocking Business Growth Opportunities
Unlocking the potential of business growth opportunities through data monetization strategies, organizations can capitalize on market trends and consumer behaviors to drive expansion. By harnessing data analytics and insights, businesses can identify growth avenues and strategic partnerships to scale their operations effectively. The distinctive feature of unlocking business growth opportunities lies in its ability to fuel sustainable growth and innovation, positioning organizations for long-term success in dynamic market environments.