Exploring Data Lake Technology: Architecture and Benefits
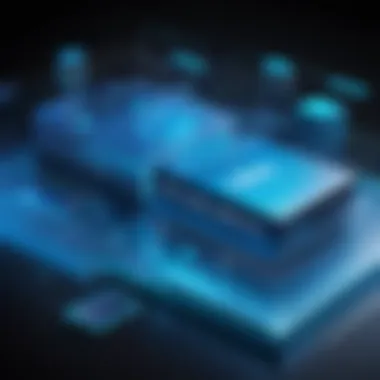
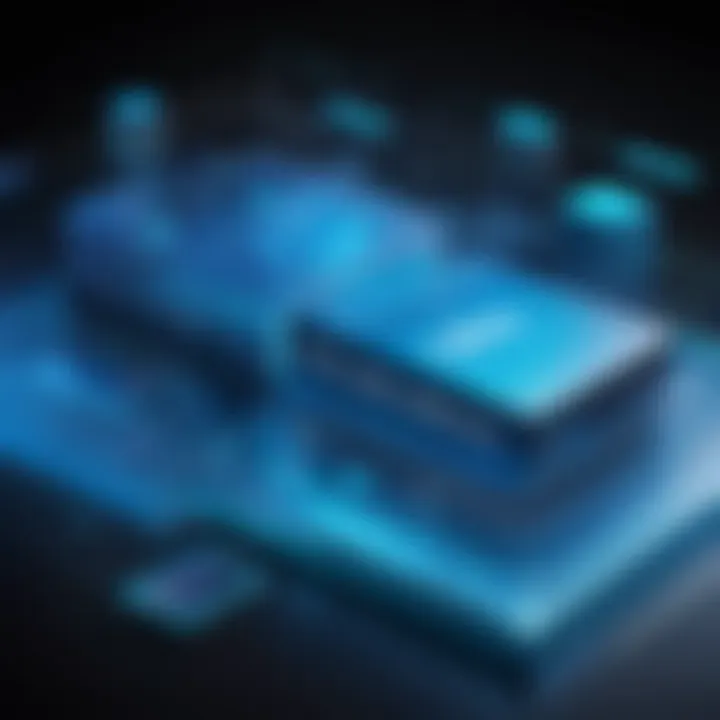
Intro
In an era where data drives decision-making, businesses are continually searching for efficient ways to manage and analyze vast quantities of information. Enter data lakes, a revolutionary approach to data storage and processing that allows organizations to handle both structured and unstructured data with finesse. Rather than relying solely on traditional databases, which can be restrictive and need extensive upfront schema definitions, data lakes offer a more flexible, scalable solution that can adapt as data grows and changes.
Data lakes hold the potential to transform the landscape of data management and analytics. They provide a repository where varied forms of data, from customer interactions to sensor outputs, can coexist without the high-cost overhead often seen in regulated database environments. In this way, they create a treasure trove of information just waiting for businesses to dive in and extract insights.
As we move through this exploration of data lake technology, we will dissect its architecture, weigh the advantages and challenges, and investigate real use cases that spotlight its role in contemporary data strategies. By understanding the nuances of data lakes, organizations can not only enhance their analytical capabilities but also craft informed decisions that resonate across their operational landscapes.
Technological Research Overview
Recent Technological Innovations
The evolution of data lake technology is marked by several innovations that enhance its functionality and accessibility. Artificial intelligence and machine learning, for example, are increasingly integrated into data lakes, providing businesses with tools for advanced predictive analytics. This allows companies to sift through mountains of data in search of patterns and trends quicker and more accurately than ever.
In addition, cloud computing has greatly influenced the deployment of data lakes. Solutions offered by Amazon Web Services, Google Cloud Platform, and Microsoft Azure are setting new standards, making it simpler for organizations to implement and scale their data lake environments according to their growth needs. This shift enables businesses to pay for only what they need and adjust quickly as requirements change.
Impact on Business Operations
Data lakes fundamentally alter how organizations approach data-driven strategies. With the ability to house vast volumes of diverse data types, companies can make decisions based on more comprehensive insights. For instance, a retail company using a data lake may analyze social media sentiment, transaction histories, and customer preferences in a single view, enabling them to tailor marketing campaigns with unmatched precision.
Moreover, the consolidated nature of data lakes minimizes data silos within organizations. When departments have access to a centralized repository of information, collaboration improves and various business units can work in harmony. This, ultimately, leads to better efficiency and productivity.
Future Technological Trends
The future of data lakes appears bright, as more businesses recognize their value. Trends suggest that we may see increased automation in data ingestion processes. Future data lakes could automatically adapt and organize incoming data, using smart algorithms to ensure that structured and unstructured data can be processed without much manual intervention.
Additionally, privacy regulations and ethical data usage are likely to shape the evolution of data lakes. As data governance becomes paramount, companies will need to incorporate robust compliance mechanisms directly within their data lake architectures to protect sensitive information while remaining agile in data usage.
"Data lakes transform how we think about data. They break down barriers and promote a culture where informed decision-making flourishes."
Data Analytics in Business
Importance of Data Analytics
Data analytics has emerged as a pivotal component in steering businesses toward successful outcomes. With data lakes offering the extensive amounts of data necessary for thorough analysis, companies can draw insights that lead to improved operations, better customer engagement, and enhanced product development.
Tools for Data Analysis
Utilizing several tools can dramatically improve how data lakes function. For example:
- Apache Spark: This open-source framework enhances data processing speeds, allowing companies to analyze big data in a timely manner.
- Tableau: Renowned for its visualization capabilities, this tool helps organizations translate complex data findings into user-friendly visuals.
- Hive: An SQL-like tool that simplifies the querying process over data stored in data lakes, making it accessible to non-technical users.
Case Studies on Data-Driven Decisions
Numerous businesses have successfully leveraged data lakes to make informed decisions that resonate throughout their operations. One notable example includes Netflix, which utilizes user data to customize viewing recommendations, leading to enhanced viewer satisfaction and retention. Another compelling case is that of Airbnb, which analyzes vast amounts of customer behavior data to optimize pricing strategies and enhance host recommendations.
Cybersecurity Insights
Threat Landscape Analysis
In today’s digital world, effective cybersecurity measures are paramount, especially as data lakes store sensitive information. Analyzing the threat landscape reveals that cyber attackers often target large data sources due to their rich content, making it essential for organizations to monitor their data lakes vigilantly.
Best Practices for Cybersecurity
Implementing best practices for cybersecurity in data lakes includes:
- Regular audits to identify vulnerabilities.
- Employing encryption methods to protect sensitive data both at rest and in transit.
- Utilizing access control measures to restrict data access based on user roles.
Regulatory Compliance in Cybersecurity
With the increasing scrutiny on data management practices, organizations must ensure they meet regulatory compliance standards like GDPR or HIPAA. Navigating these regulations requires embedding compliance requirements into the data lake architecture from the get-go, ensuring that data handling processes are transparent and secure.
Artificial Intelligence Applications
AI in Business Automation
Integrating AI into data lakes can streamline several business processes. By automating mundane tasks such as data entry and cleaning, businesses can boost efficiency and redirect human resources toward strategic initiatives.
AI Algorithms and Applications
Various AI algorithms can enhance the functionality of data lakes:
- Clustering Algorithms: Grouping similar data points can identify trends in customer behaviors.
- Predictive Analytics: These models can forecast future outcomes based on historical data, optimizing supply chain operations to match consumer demand.
Ethical Considerations in AI
As businesses utilize AI within data lakes, ethical considerations arise, particularly surrounding data privacy and bias. Organizations must remain vigilant about how they collect, store, and use data to ensure fairness and compliance with ethical standards.
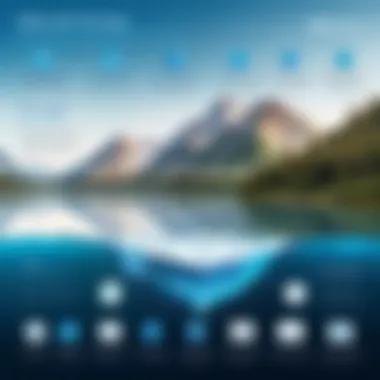
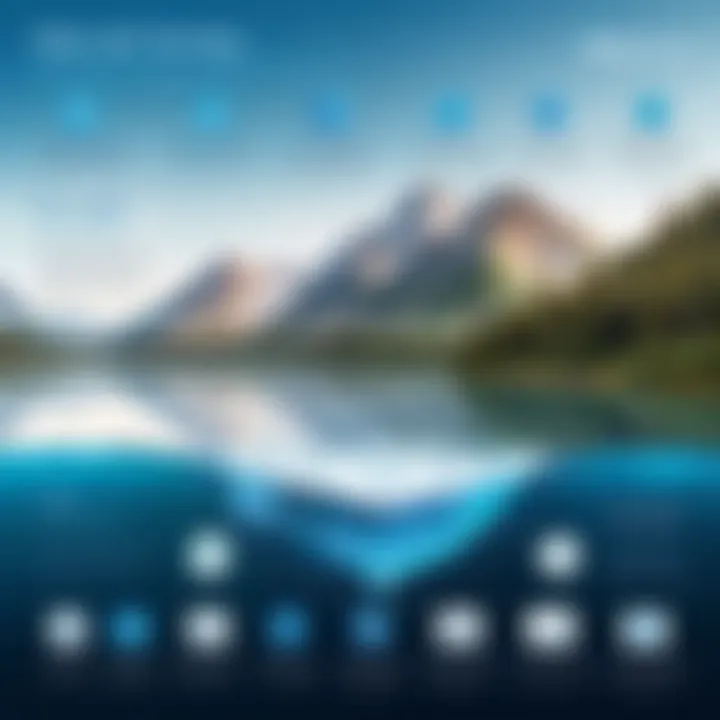
Industry-Specific Research
Tech Research in Finance Sector
Data lakes are rapidly becoming vital in the finance sector. Banks leverage these systems to improve risk management practices, personalizing financial products to better fit customer needs. This data-centric approach not only enhances customer experience but minimizes operational risks.
Healthcare Technological Advancements
In healthcare, data lakes serve as integral platforms for research and patient management. They facilitate a comprehensive view of patient data, enabling healthcare professionals to make informed treatment decisions and develop predictive models for patient care.
Retail Industry Tech Solutions
Retailers are utilizing data lakes to mesh sales data with shopping behaviors, allowing for hyper-personalized marketing strategies. This leads to higher conversion rates as customers receive tailored recommendations that resonate with their preferences.
By understanding and implementing data lake technology with a strategic approach, organizations across various sectors can leverage their data more effectively. This lays the foundation for data-driven decision-making that can steer companies toward enduring success.
Understanding Data Lake Technology
In the realm of data management, understanding data lake technology has become increasingly paramount. As organizations grapple with the vast amounts of structured and unstructured information swirling around them, having a grasp of what data lakes offer can set the stage for more informed decision-making. This understanding is not just for IT professionals but extends to entrepreneurs and decision-makers who require insights into data utilization to inform their strategies.
Data lake technology provides a versatile infrastructure that accommodates varied data forms. It enables businesses to harness data for analytics, prevent silos, and enhance real-time decision-making capabilities. As we dive deeper into this subject, let’s dissect some vital aspects that underscore its significance in the modern business landscape.
Definition and Concept
At its core, a data lake can be thought of as a centralized repository that allows businesses to store all their data, regardless of format or structure. The definition seems straightforward, but the implications and functionalities it embodies are much more complex. Essentially, unlike traditional data storages, data lakes can handle raw and unstructured data, providing flexibility that conventional databases often lack.
To put it simply, imagine a large lake fed by various streams—some are clear (structured data like spreadsheets), while others are murky (unstructured data like social media posts or video files). All these streams flow into the lake, making it a rich resource for analytics.
Evolution of Data Storage Solutions
The journey of data storage has evolved significantly over the decades. Initially, businesses relied heavily on relational databases, which were suitable for structured data. Over time, as the internet expanded and data volumes ballooned, traditional systems began to buckle under pressure.
With the rise of Big Data, came a rethinking of storage capabilities. Enter data lakes, which emerged as a response to the limitations of data warehouses. Just like a caterpillar transforms into a butterfly, the evolution from rigid data systems to agile data lakes illustrates a profound shift. Here’s how the landscape has changed:
- From Rigid Systems to Flexibility: Traditional databases required predefined schemas. Data lakes, on the contrary, allow schema-on-read, meaning data can be ingested raw without prior structuring.
- Increased Data Volume: The rise of IoT and digital interactions means data is generated at an unprecedented scale. Data lakes thrive in this environment, ready to take in diverse data rapidly.
- Usability Across Functions: While data warehouses were largely confined to business intelligence needs, data lakes serve broader organizational objectives, catering to data scientists, marketing teams, and more.
By examining these transitions, it’s evident that data lake technology plays a pivotal role in shaping how organizations approach data management and analytics today.
"Understanding data lakes is like having a map in an uncharted territory; it guides you through the data landscape efficiently."
This growing acknowledgment of data lake technology marks a notable point in the vast journey of data storage solutions, sculpting a future where information can be utilized more robustly.
Architecture of Data Lakes
Understanding the architecture of data lakes is paramount in harnessing their full potential within modern data ecosystems. At its core, a data lake is a repository that allows for the storage of vast amounts of raw data in its native format until it's needed. This architecture ensures that organizations can accommodate diverse data types and enable advanced analytics on an unprecedented scale.
Core Components
The building blocks of a data lake can be broken down into several essential constituents:
- Storage: This is where the data physically resides. Often built using distributed file systems like Hadoop Distributed File System (HDFS), these storage solutions ensure scalability and durability.
- Data Ingestion Layer: Functioning as the entry point for data, this layer oversees the collection, processing, and loading of data from various sources into the lake.
- Metadata Management: Understanding what data is available and its schema is critical. Metadata services track the details around data, including where it came from, how it can be used, and its lifecycle.
- Access and Security Controls: These components govern how users can interact with the data lake and help safeguard sensitive information.
Establishing these components properly is vital for optimizing system performance and data retrieval efficacy.
Data Ingestion Processes
Data ingestion is pivotal to the successful functioning of a data lake. It entails the seamless gathering of data from multiple sources which could be anything from databases and APIs to streaming data from Internet of Things (IoT) devices. Understanding the ingestion process has direct implications on data availability and quality.
Types of Data Ingestion:
- Batch Ingestion: This method collects data at scheduled intervals, making it suitable for historical data analysis. Organizations might load data nightly or weekly to reduce the processing load during peak hours.
- Streaming Ingestion: In contrast, streaming ingestion allows for real-time data processing, capturing data as it flows in from sources, creating opportunities for instantaneous insights, especially in the realms like fraud detection or real-time marketing analytics.
These processes have to be meticulously designed to ensure that data quality and transfer speed do not compromise. Without a well-defined ingestion process, organizations may end up with a data stew that could lead to chaos rather than clarity.
Storage Management
The management of storage resources within a data lake is one of the most critical aspects of the architecture. Without efficient storage management, organizations risk overspending on capacity while underutilizing their stored data.
Data lakes thrive on the ability to scale out—adding more cheap storage when necessary. However, merely piling on more disks doesn’t cut it. Here are some strategies that promote effective storage management:
- Data Lifecycle Management: Placing a strategy on how long data is retained can significantly reduce storage costs. Organizations should classify data into tiers based on usage and importance.
- Data Compression Techniques: Using compression algorithms can save enormous amounts of storage space. When millions of records are involved, even small percentage gains can translate into substantial savings.
- Data Quality Checks: Implement regular quality audits to cleanse the data stored in the lake. This helps to maintain the integrity of the data and ensures that only relevant and quality data is active in the system.
The organization’s approach to these elements shapes the responsiveness and efficiency of the overarching data architecture while influencing decision-making capabilities.
Key Advantages of Data Lakes
Data lakes have emerged as a vital resource in today’s data-driven landscape. Recognizing their advantages allows businesses to harness unparalleled capabilities in managing data. The importance of understanding these merits cannot be understated, as it lays the groundwork for practical applications and sound strategic decisions. Here, we’ll dive into three significant advantages: scalability and flexibility, the handling of diverse data types, and the facilitation of advanced analytics.
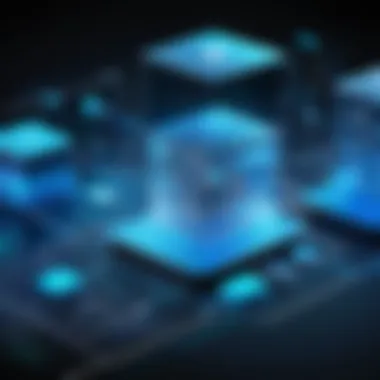
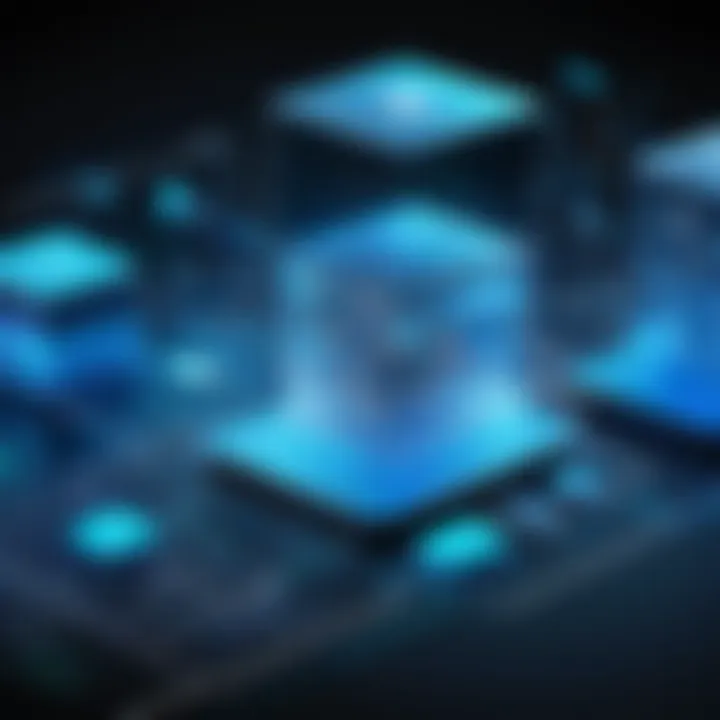
Scalability and Flexibility
One of the primary benefits of data lakes is their scalability. As organizations grow, so do their data needs. Traditional databases often encounter a bottleneck, sluggishly plodding along as data piles up. With a data lake, this issue dissipates. Organizations can store vast amounts of data without worrying about the constraints of a rigid schema. This flexibility means you don’t have to throw out data just because it doesn’t fit neatly into predetermined categories.
In practical terms, companies can scale their storage capacity up or down based on immediate needs. For instance, a retail enterprise may see a predictive surge in online shopping during a seasonal event. Instead of investing heavily in temporary infrastructure, they can leverage a data lake. This kind of agility separates the wheat from the chaff, as companies that can adapt quickly are often the ones that stay ahead in competitive markets.
Handling Diverse Data Types
Data lakes shine when it comes to managing different types of data. In an age where businesses capture structured, unstructured, and semi-structured data, having a single repository that can accommodate all forms is indispensable. From text and images to video and sensor data, a data lake serves as a one-stop shop.
For example, an automotive company may collect everything from engine performance logs to social media insights about customer sentiment. Traditional systems might struggle to aggregate this wealth of information. A data lake, however, welcomes all types without excessive preprocessing. This opens the door for rich analytical insights that would be impossible to capture otherwise.
Facilitating Advanced Analytics
The role of data lakes extends beyond mere storage; they are a breeding ground for advanced analytics. As companies collect more diverse datasets, the potential for in-depth analysis skyrockets. These insights can inform everything from high-level strategy to granular operational adjustments.
With the inclusion of machine learning algorithms and artificial intelligence, data lakes empower analysts to explore patterns and correlations, even in vast datasets. They effectively act as analytical playgrounds where organizations can experiment without the constraints often found in more traditional environments.
"Data lakes aren’t just vast reservoirs—they’re the engines of insight, delivering what you need to stay competitive and innovate."
Challenges in Implementing Data Lakes
Implementing a data lake is not a walk in the park. Organizations need to navigate through various challenges that can potentially hinder the effectiveness of this technology. Understanding these challenges is crucial for proper planning and execution, ensuring that businesses leverage their data effectively and meet their strategic objectives.
Data Governance and Security
Data governance refers to the management of data availability, usability, integrity, and security within an organization. It’s the backbone that ensures data is handled correctly, but it's especially complex when dealing with a data lake, where data types and sources are diverse.
- Sensitive Information: Data lakes can house a mix of sensitive and non-sensitive data. Without stringent governance, sensitive data may slip through the cracks, leading to compliance issues, especially with regulations like GDPR or HIPAA.
- User Access Control: The sheer volume of data in lakes can make managing user access a daunting task. Organizations might struggle to assign permissions correctly, allowing unauthorized users to access information they shouldn't.
In the face of these challenges, implementing robust access controls and auditing mechanisms can help ensure that data governance remains stringent. It’s like having a strong lock on your front door; it keeps out unwanted visitors and protects what’s inside.
Data Quality Issues
When it comes to data lakes, the saying "garbage in, garbage out" could not be more relevant. With multiple sources feeding data into a lake, maintaining quality is key.
- Inconsistent Formats: Different sources may send data in various formats. This inconsistency can lead to confusion and errors when trying to analyze or integrate the data later on. Imagine trying to fit a square peg into a round hole; it simply doesn’t work!
- Lack of Cleansing: Unlike traditional databases where data is cleansed and processed before entry, data lakes often store raw data. This can result in lots of noise, making it hard to locate valuable insights.
To combat data quality issues, businesses must establish rigorous data cleansing protocols and metadata management strategies. Integrating tools that allow for automatic monitoring and cleansing of data can prove beneficial.
Integration with Existing Systems
Integrating a data lake with existing systems can be like trying to mix oil and water; the results may not always be favorable. Organizations often find themselves at a crossroads where legacy systems and newer technologies don’t play well together.
- Legacy System Constraints: Older systems may not be built to handle the unstructured data that data lakes typically manage. This incompatibility can slow down operations or even lead to data losses if not handled correctly.
- APIs and Middleware: Without the right middleware or Application Programming Interfaces (APIs), data can be trapped in silos, meaning it may not flow freely between systems. This bottleneck can greatly reduce the effectiveness of data analytics efforts.
Successful integration requires thoughtful planning. Organizations should evaluate the capabilities of their existing systems and consider investing in tools that enhance data interoperability. Moreover, adjusting workflows to incorporate the data lake efficiently can streamline the transition and utilization of the data.
Successfully navigating these challenges can lead to effective data lakes that drive powerful insights, but being aware of potential pitfalls from the get-go saves organizations from future headaches.
Implementation Strategies for Businesses
Implementing data lake technology demands careful consideration and planning to ensure that it aligns with the broader business objectives. A well-articulated strategy not only maximizes the potential of data lakes but also addresses potential pitfalls. Successful implementation involves a few crucial elements, namely assessing business needs, choosing the right tools, and establishing a governance framework.
Assessing Business Needs
Before jumping into the implementation of a data lake, it is vital for businesses to first evaluate their specific requirements. This process goes beyond a mere evaluation of current data volumes and dives deeper into understanding what insights the organization seeks to derive from its data.
- Define Objectives: Businesses need clarity on their data goals. Are they aiming to enhance customer experience, improve operational efficiency, or cultivate a competitive edge? Knowing these objectives informs how the data lake should be structured and utilized.
- Identify Data Sources: Companies should take stock of existing data inputs, both structured and unstructured. This could include customer transaction records, social media interactions, or even Internet of Things (IoT) data.
- Scoped Resources: Understanding the necessary infrastructure is crucial. This includes analyzing the available resources in terms of budget and human expertise. Many businesses underestimate the resources required to effectively manage a data lake.
Choosing the Right Tools and Platforms
Once the business needs have been established, it’s time to explore the tools and platforms that can support the data lake architecture. The market has a plethora of options, and choosing tools that suit your specific requirements can make or break your implementation.
- Evaluate Compatibility: The selected tools must work well with existing systems. Whether considering cloud services like Amazon Web Services or Azure, it’s crucial to ensure seamless integration into the existing ecosystem.
- User-Friendly Interface: Tools should not only be powerful but also user-friendly. A steep learning curve can deter teams from utilizing these systems effectively.
- Scaling Capabilities: As the business grows, so too will the data. It’s vital to choose platforms that can scale efficiently without hitting performance snags.
Establishing a Governance Framework
The success of a data lake doesn’t merely hinge on its architecture and tools; it’s also deeply rooted in governance. A robust framework for governance facilitates data security, quality management, and compliance with regulations.
- Data Stewardship: Assigning dedicated data stewards can help maintain control over data quality. Their responsibilities include monitoring data accuracy and ensuring adherence to compliance.
- Access Management: Implementing stringent access controls is essential for data protection. Not all employees require access to all data. Establish clear policies to define who can view or manipulate the data.
- Documentation and Auditing: Regularly updating documentation and conducting audits helps in maintaining the integrity of data practices. These processes also ensure that the data lake remains compliant with any applicable regulations.
A well-implemented governance framework is indispensable for the long-term success of data lake technology. Without governance, even the best architectures can fall prey to inefficiencies and security risks.
In summary, businesses must embrace a strategic approach when implementing data lake technology. Each step — from specifying business needs to tool selection and governance — contributes significantly to unlocking the value hidden within data.
Comparative Analysis with Data Warehousing
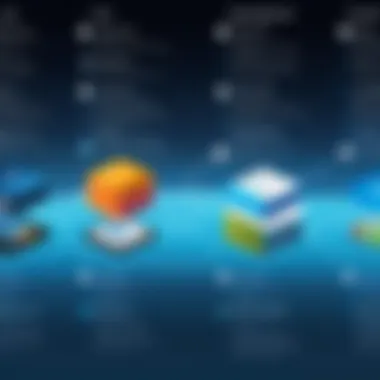
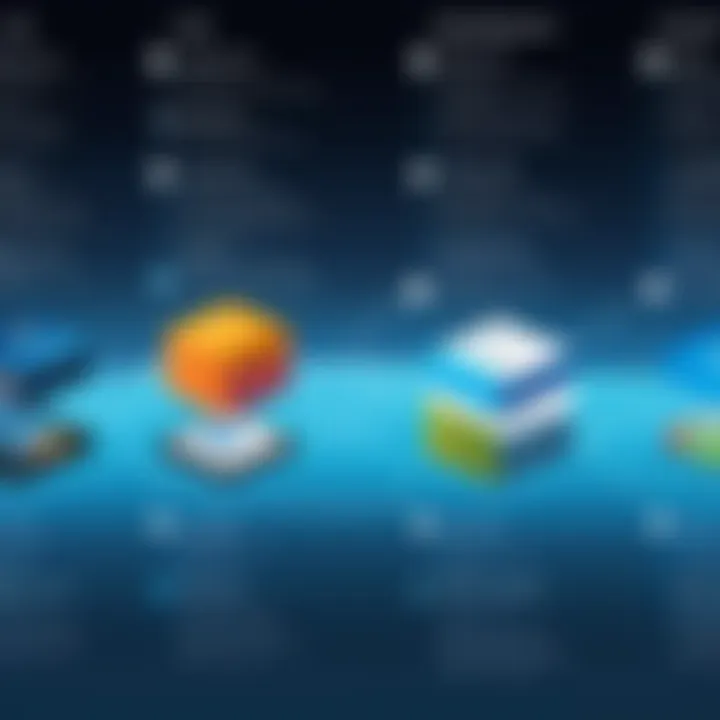
Understanding the differences between data lakes and data warehouses is crucial for organizations that aim to maximize their data management strategies. While both play important roles in data storage, they serve different purposes and are optimized for distinctive use cases. This section will unpack the similarities and differences between the two, alongside guidelines on when to use each solution.
Similarities and Differences
At first glance, data lakes and data warehouses may seem like two peas in a pod, but the nuances in their design and utility separate them significantly. Here are some key points of comparison:
- Data Structure:
Data warehouses are typically structured for query efficiency, which means data is usually organized into tables and schemas. In contrast, data lakes embrace a more flexible architecture, allowing for unstructured, semi-structured, and structured data, all in one place. This results in a broader data consumption capability when utilizing a data lake. - Storage Costs:
Data lakes often leverage cheaper storage solutions, making them cost-effective for vast volumes of data. Conversely, data warehouses require higher-quality storage infrastructure, which can bump up expenses significantly. - Use Cases:
Data lakes are well-suited for raw data analytics, providing a playground for data scientists to experiment and innovate. Whereas data warehouses shine in business intelligence situations, where structured data is crucial for generating reports and dashboards to inform decision-making.
One might say that data lakes offer the wild west of data exploration while data warehouses deliver an organized library for business insights. This difference underscores the fundamental roles each solution plays within an organization.
When to Use Each Solution
Choosing between a data lake and a data warehouse boils down to understanding specific business requirements and the types of analysis one intends to perform. Here are some considerations that could help in making that decision:
- Use a Data Lake When:
- Opt for a Data Warehouse When:
- Your organization is dealing with vast amounts of unstructured or semi-structured data, such as clickstream data, social media interactions, or log files.
- You want to empower data scientists and analysts to explore data in its raw form and experiment with machine learning models.
- Cost is a primary concern, as data lakes can keep storage expenses in check by using affordable technologies like Amazon S3 or Google Cloud Storage.
- Your needs revolve around operational reporting and business intelligence, requiring well-structured data for analysis.
- You need to ensure compliance with data governance and quality standards, which might be more challenging in an unstructured environment.
- Real-time analytics is essential, as data warehouses often provide more efficient querying capabilities compared to lakes.
In summary, both data lakes and data warehouses have their place in the landscape of data management. The choice between them should stem from a thorough analysis of your organization’s requirements, whether that means diving into extensive and varied data or necessitating structured and organized datasets for immediate decision-making insights.
"Data lakes are the chaotic oceans of information, promising discovery, while data warehouses act as well-regulated rivers guiding organizations toward informed decisions."
Ultimately, recognizing the complementary nature of these solutions can lead organizations to implement a hybrid approach, marrying the adaptability of data lakes with the stability of data warehouses for a holistic data strategy.
Case Studies and Real-World Applications
Understanding the practical applications of data lake technology is crucial for grasping its full potential. Though the concept might seem abstract, real-world case studies breathe life into theory, showcasing how various industries are adopting data lakes. Not only do these applications illustrate the benefits of data lakes in action, but they also emphasize what businesses need to consider when integrating such systems into their existing frameworks. This section dives into industry-specific use cases and success stories, presenting a clearer picture of data lakes' impact across diverse sectors.
Industry-Specific Use Cases
Data lakes have emerged as versatile solutions for organizations seeking to handle complex data environments. Here are a few notable examples across different industries:
- Healthcare: Large hospitals and research institutions are using data lakes to compile patient records, genomic data, and clinical insights. For instance, Mount Sinai Health System integrates electronic health records with genomic data stored in their data lake to improve patient outcomes through personalized medicine.
- Finance: Financial institutions utilize data lakes to enhance fraud detection and risk management. An example can be seen in American Express, which leverages its data lake to analyze transaction patterns in real-time, allowing for immediate responses to potential fraud.
- Retail: Companies like Walmart have adopted data lakes to analyze customer behavior and optimize inventory. By aggregating data from point-of-sale systems, online platforms, and customer loyalty programs, Walmart can better predict trends and enhance customer engagement.
- Telecommunications: Telecom companies are harnessing data lakes for network optimization. A case study of Verizon shows how they store vast amounts of call detail records to analyze network performance and improve service quality.
These examples underscore the data lake's ability to store and analyze diverse data types, enabling organizations to draw actionable insights.
Success Stories and Learnings
Exploring the success stories of companies that have effectively implemented data lakes reveals valuable lessons and strategies that can guide other organizations. Here are some insights drawn from notable success:
- Netflix's Personalized Recommendations: Netflix has developed a sophisticated data lake that aggregates user activity and viewing preferences. This highly personalized recommendation system not only keeps users engaged but also drives subscriptions by suggesting relevant content. Netflix’s continuous investment in its data lake demonstrates the need for ongoing optimization and scalability to handle growing volumes of data.
- The Coca-Cola Company: Coca-Cola's data lake initiative aimed at improving operational efficiencies. By leveraging its lake to analyze data from IoT devices in their bottling plants, the company gained insights into production line performance. The lesson here is the importance of a cross-departmental approach, as different business units can extract value from the same pool of data, leading to holistic improvements.
- Capital One: As a pioneering bank in the digital space, Capital One has utilized data lakes for customer insights and predictive analytics. Their focus on data governance ensured compliance with regulations while maximizing value from customer data. This emphasizes that a strong governance framework is essential when managing sensitive data within a data lake.
Future of Data Lake Technology
As the digital landscape continues to evolve at a breakneck speed, understanding the future of data lake technology becomes crucial for any organization looking to remain competitive. This section examines upcoming trends and transformative influences shaping the realm of data lakes. The significance of these trends cannot be overstated, as they offer valuable insights into how businesses can leverage data more effectively, improve decision-making, and harness advanced analytics to fuel growth.
Trends in Data Management
In the world of data management, a few unmistakable trends are emerging that stand to reconfigure how organizations approach data lakes. Here’s a look at some of these prevailing themes:
- Increased Adoption of Cloud Solutions: More and more organizations are migrating to cloud-based data lakes. This shift facilitates scalability and reduces infrastructure costs. Cloud storage offers elasticity that on-premises solutions struggle to match.
- Real-Time Analytics: The demand for instant insights is growing. Data lakes are evolving to accommodate streaming data, enabling businesses to gain real-time visibility into operations and make quicker decisions.
- Data Democratization: The idea here is pretty straightforward: make data accessible to everyone in an organization, not just data scientists. User-friendly tools and interfaces are being developed, allowing non-technical users to query data lakes without needing specialized skills.
- Interoperability and Integration: Organizations are investing in solutions that ensure data lakes can easily integrate with existing systems and applications, creating a seamless workflow. This shift minimizes friction and enhances data governance.
- Focus on Data Governance: As data volumes explode, the importance of maintaining high data quality and governance becomes paramount. Future data lake technologies are likely to incorporate advanced governance frameworks that allow businesses to manage their data responsibly.
"Anticipating the future means recognizing the patterns of today. Data lakes are not just storage solutions; they are the backbone of tomorrow's analytics architecture."
The Role of Artificial Intelligence
The influence of artificial intelligence (AI) on data lake technology is undeniable. As businesses visualize how to harness the power of data lakes, they can’t overlook AI's remarkable capabilities. Here’s how AI is steering the ship:
- Automated Data Curation: With vast volumes of data flowing into data lakes, AI algorithms will increasingly handle data cleansing and organization. This automation reduces manual effort and minimizes errors, allowing analysts to focus on deriving insights instead of spending time preparing data.
- Enhanced Predictive Analytics: AI-driven tools can sift through massive datasets, identifying trends and patterns unrecognizable to the human eye. Businesses can predict future behaviors or outcomes, such as customer churn or market dynamics, giving them a strategic advantage.
- Natural Language Processing (NLP): As NLP technologies advance, querying data lakes will become easier for non-technical users. Conversational interfaces powered by AI allow users to ask complex questions in plain language and receive accurate insights instantly.
- Proactive Security Measures: AI can also play a vital role in securing data lakes. By employing machine learning to detect anomalies or potential breaches, organizations can respond proactively, enhancing cybersecurity and safeguarding sensitive information.
In summary, the future of data lake technology is anything but static. Trends like cloud adoption, real-time analytics, and AI integration collectively indicate a more agile, user-friendly, and secure approach to data management. Organizations that adopt these changes will not just keep pace—they will thrive in an increasingly data-driven world.
The End
As we draw the curtains on our examination of data lake technology, it's essential to underscore the importance of this innovative solution in today’s data-driven landscape. Businesses are continually inundated with a myriad of data types, often stored in disparate systems that hinder effective analysis. Data lakes present a robust pathway to address this issue, serving as a centralized repository for structured and unstructured data.
Summary of Key Insights
Throughout this article, we have navigated various aspects of data lakes, from their architecture to real-world applications. Some pivotal insights include:
- Scalability and Flexibility: Data lakes allow organizations to scale easily as their data needs grow, without the constraints often experienced with traditional data warehousing solutions.
- Enhanced Analytics Capabilities: They facilitate sophisticated analytics, empowering businesses to derive actionable insights from vast datasets. The ability to process diverse data types unleashes the potential for advanced analytics like machine learning and predictive modeling.
- Challenges in Implementation: Despite their advantages, businesses must be prepared to tackle challenges like data governance, security, and integration issues. Establishing a robust governance framework is crucial to unlocking the true benefits of a data lake.
These takeaways highlight the significant benefits of adopting data lake technology while also emphasizing the need for strategic planning during implementation.
Final Thoughts on Adoption and Implementation
For organizations contemplating the adoption of data lakes, it’s vital to consider both the potential and the pitfalls. It’s not merely about accumulating data; it's about creating a strategy that aligns with business goals, promotes data accessibility, and ensures governance. A thoughtful approach encourages:
- Tailored Solutions: Each business has unique needs; therefore, customizing a data lake strategy to align with those specific requirements is imperative.
- Continuous Evaluation: The tech world is ever-evolving, and so should your data strategy. Regular assessments of data governance and security policies can make all the difference in maintaining the integrity of your data lake.
In sum, adopting data lakes is akin to laying down new tracks for a train destined for the future of data management. With careful consideration and implementation, organizations stand to benefit immensely, ensuring that they not only stay afloat in the wave of data but also ride it towards success.