Unveiling the Diverse Realm of Data Analysis Techniques in Modern Business
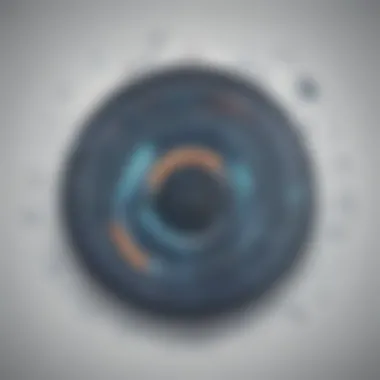
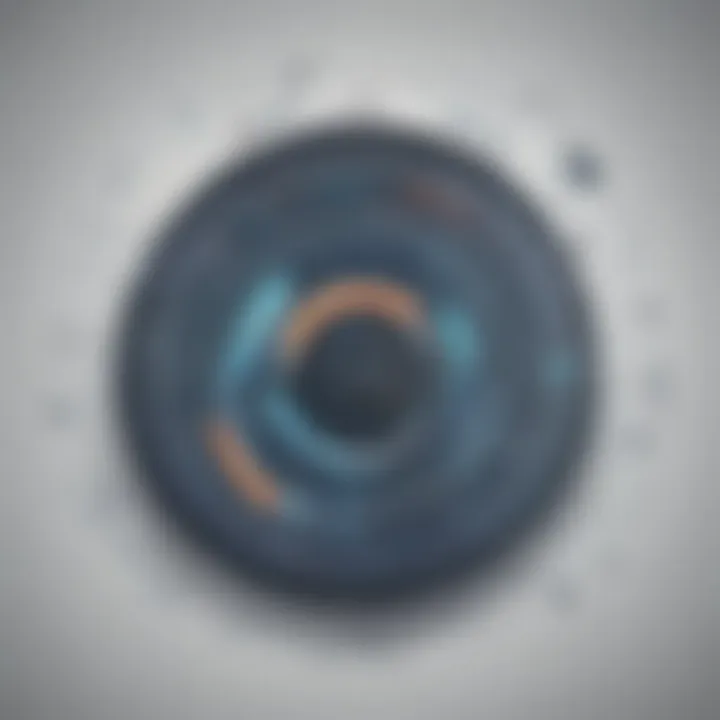
Data Analytics in Business
Data analytics plays a pivotal role in modern business operations. In a data-driven landscape, leveraging the power of analytics offers unparalleled insights essential for informed decision-making and strategic growth. This section delves into the importance of data analytics and its transformative impact on businesses across various industries.
Importance of Data Analytics
The significance of data analytics cannot be overstated in today's competitive market. By harnessing data analytics, businesses can uncover patterns, trends, and correlations within their datasets, enabling them to make data-driven decisions. This data-driven approach empowers organizations to optimize processes, streamline operations, and identify potential areas for growth and improvement.
Tools for Data Analysis
Utilizing cutting-edge tools for data analysis is crucial for businesses aiming to stay ahead of the curve. From traditional statistical methods to advanced machine learning algorithms, a diverse array of tools is available to extract meaningful insights from vast amounts of data. Incorporating efficient data analysis tools enables businesses to enhance operational efficiency, drive innovation, and gain a competitive edge in the market.
Case Studies on Data-Driven Decisions
Real-world case studies offer profound insights into the practical application of data-driven decision-making. By examining successful implementations of data analytics in various business scenarios, organizations can draw inspiration and learn best practices for leveraging data effectively. These case studies illuminate the tangible benefits of data analytics, showcasing how data-driven decisions lead to improved outcomes and sustainable business growth.
Introduction to Data Analysis
Data analysis plays a pivotal role in modern business landscapes. The utilization of data-driven insights has become imperative for organizations seeking to gain a competitive edge and optimize their operations. Through the systematic evaluation of data points, businesses can extract valuable information and make well-informed decisions. This section delves into the integral aspects of data analysis, shedding light on its significance in driving efficiency, identifying opportunities, and mitigating risks.
Importance of Data Analysis
Enhancing Decision-Making
Enhancing decision-making through data analysis involves utilizing statistical tools and techniques to evaluate information critically. By harnessing data, decision-makers can reduce uncertainty, highlight trends, and make strategic choices based on empirical evidence. The ability to process vast amounts of data swiftly empowers organizations to make agile decisions in dynamic markets.
Identifying Trends and Patterns
Data analysis aids in identifying trends and patterns, enabling businesses to forecast future developments accurately. By recognizing recurring trends, organizations can adapt their strategies proactively and capitalize on emerging opportunities. This facet of data analysis serves as a cornerstone for strategic planning and fostering innovation within the business ecosystem.
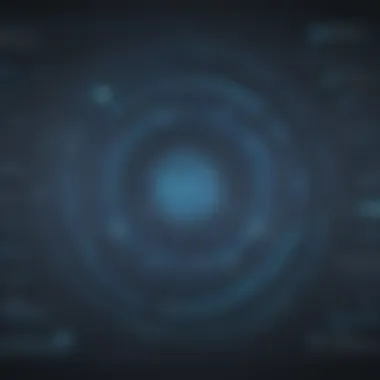
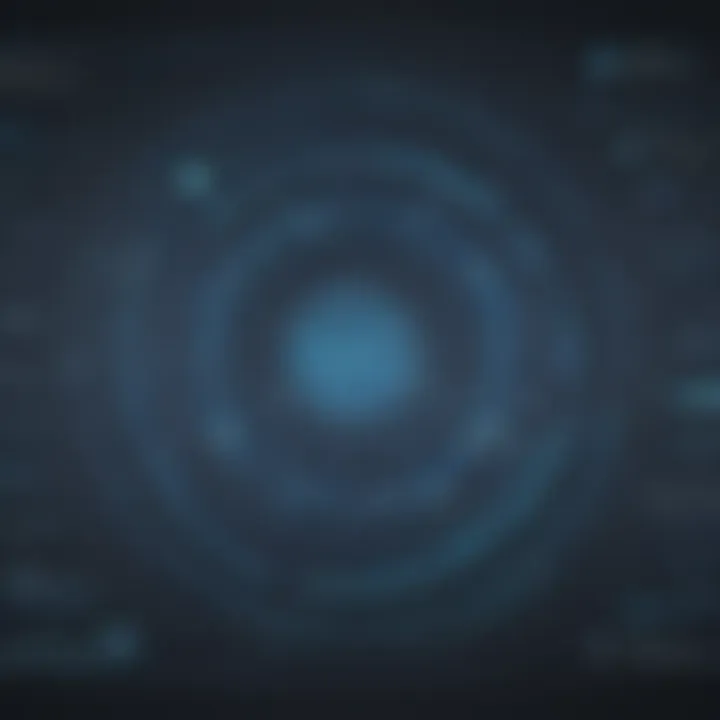
Driving Business Growth
Data analysis serves as a catalyst for driving business growth by providing insights that facilitate expansion and optimization. Through discerning analysis of market trends and consumer behavior, companies can refine their offerings, enhance customer experiences, and streamline operations. The actionable intelligence derived from data analysis empowers organizations to enhance their competitive advantage and sustain long-term growth.
Role of Technology in Data Analysis
Automation and Efficiency
Automation and efficiency in data analysis involve leveraging technological tools to streamline processes and enhance accuracy. By automating repetitive tasks and data cleaning processes, organizations can improve productivity and focus on strategic tasks. The incorporation of automated algorithms drives efficiency in data analysis, reducing manual errors and expediting decision-making processes.
Scalability and Accessibility
Ensuring scalability and accessibility within data analysis frameworks is essential for accommodating growing data volumes and diverse user needs. Scalable solutions enable organizations to handle large datasets efficiently and cater to evolving analytical requirements. Enhancing accessibility ensures that stakeholders across various levels can leverage data insights to inform their decision-making processes effectively.
Advanced Algorithms
The integration of advanced algorithms in data analysis empowers organizations to glean actionable insights from complex datasets. Advanced algorithms like machine learning and artificial intelligence enable predictive modeling, anomaly detection, and personalized recommendations. By harnessing these sophisticated algorithms, businesses can unlock new opportunities, optimize processes, and drive innovations across various domains.
Types of Data Analysis
In the realm of data analysis techniques, understanding different types plays a crucial role in extracting meaningful insights for informed decision-making and fostering business growth. Descriptive Analysis, Diagnostic Analysis, Predictive Analysis, and Prescriptive Analysis represent pillars in this field. Descriptive Analysis involves summarizing data to provide a snapshot of trends and patterns within the dataset, making it an essential step in drawing actionable conclusions. Diagnostic Analysis focuses on identifying causes for past performance, shedding light on factors influencing outcomes and enabling stakeholders to address underlying issues effectively. Predictive Analysis anticipates future trends and behaviors based on historical data, empowering organizations to proactively strategize and mitigate risks. Prescriptive Analysis goes a step further by recommending specific courses of action, optimizing strategies, and utilizing simulation models to simulate various scenarios for decision-makers.
Descriptive Analysis
Summarizing Data
Summarizing data is a critical component of Descriptive Analysis, allowing analysts to condense vast amounts of information into digestible insights. By consolidating data points and metrics, summarization provides a comprehensive view of the dataset's essential characteristics. This method streamlines decision-making processes by highlighting key trends, outliers, and performance indicators. While offering a quick overview, summarizing data may oversimplify complexities within the dataset, potentially missing nuanced details critical for strategic actions.
Visualizing Key Metrics
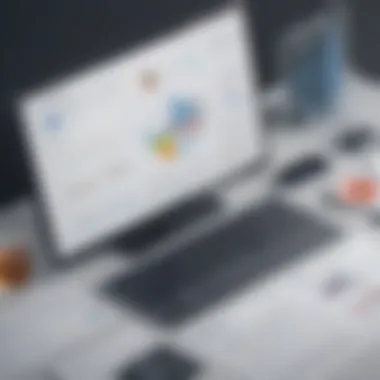
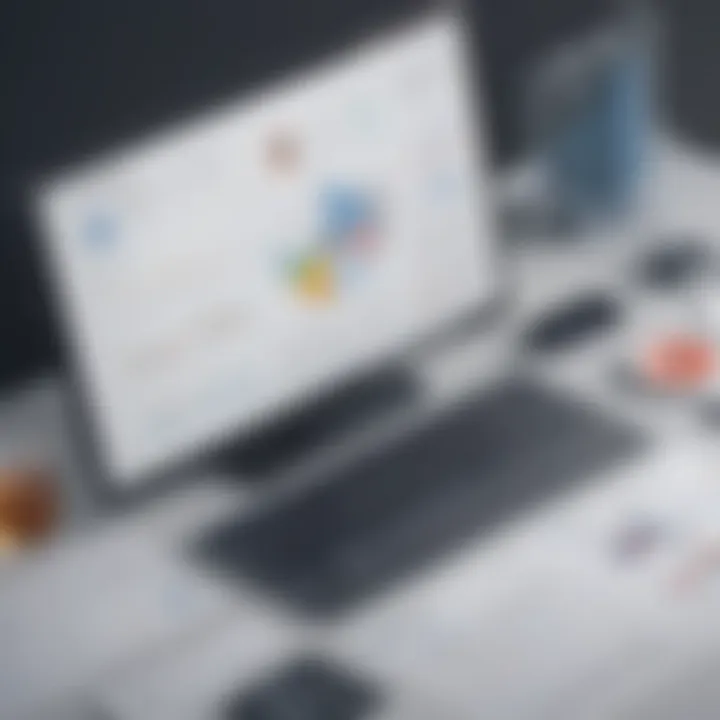
Visualizing key metrics involves representing data through graphs, charts, and other visual aids to enhance comprehension and interpretation. Visualizations help stakeholders grasp trends and patterns quickly, making data more accessible and actionable. They offer a visually appealing means of communicating insights, facilitating better understanding among diverse audiences. However, the efficacy of visualizations relies heavily on the chosen representation method, with suboptimal choices potentially leading to misinterpretation or miscommunication of data.
Identifying Key Performance Indicators (KPIs)
Identifying Key Performance Indicators (KPIs) is crucial for evaluating the success of business operations and strategies. KPIs are specific metrics that measure progress towards predefined goals, enabling organizations to assess performance and track trends effectively. By focusing on KPIs, businesses can align their efforts with overarching objectives, facilitating informed decision-making and driving continuous improvement. However, selecting relevant KPIs requires careful consideration to ensure alignment with organizational priorities and avoid measuring irrelevant or misleading metrics.
Diagnostic Analysis
Understanding Causes for Past Performance
Understanding causes for past performance involves delving into historical data to ascertain the factors influencing outcomes. By analyzing past trends and events, stakeholders can gain insights into the root causes behind success or failures, informing future strategies. This analysis helps in identifying patterns and correlations that contribute to outcomes, enabling targeted interventions and process improvements. However, retrospective analysis may overlook external factors or emergent trends that could impact future performance.
Root Cause Analysis
Root Cause Analysis aims to identify the fundamental reasons responsible for specific outcomes or occurrences. By exploring the underlying issues that lead to desired or undesired results, organizations can implement targeted corrective actions and preventive measures. This method focuses on digging deep into the data to uncover systemic issues, fostering long-term solutions rather than superficial fixes. However, root cause analysis can be time-consuming and resource-intensive, requiring comprehensive data collection and analysis.
Identifying Anomalies
Identifying anomalies involves detecting irregularities or deviations from expected patterns within the data. By flagging outliers and discrepancies, analysts can pinpoint data errors, fraud, or system malfunctions that require attention. Anomaly detection is essential for maintaining data integrity and ensuring the reliability of analytical insights. However, differentiating between genuine anomalies and random fluctuations can be challenging, potentially leading to false alarms or overlooking genuine anomalies.
Predictive Analysis
Forecasting Future Trends
Forecasting future trends entails leveraging historical data and statistical models to predict upcoming patterns and behaviors. By extrapolating past trends and accounting for influencing factors, organizations can anticipate market dynamics and consumer preferences. This proactive approach allows businesses to adapt strategies preemptively, capitalizing on emerging opportunities and mitigating potential risks. However, forecasting accuracy relies on the quality and relevance of historical data, making continuous data collection and analysis imperative.
Predicting Customer Behavior
Predicting customer behavior involves using predictive models to anticipate consumer actions and preferences. By segmenting customers based on historical interactions and attributes, businesses can personalize offerings and marketing strategies, enhancing customer satisfaction and loyalty. Predictive analytics enables targeted marketing campaigns and tailored service delivery, optimizing customer experiences. However, predictions may sometimes be inaccurate due to changing consumer preferences or unforeseen market shifts.
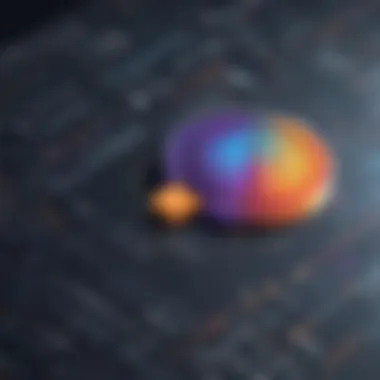
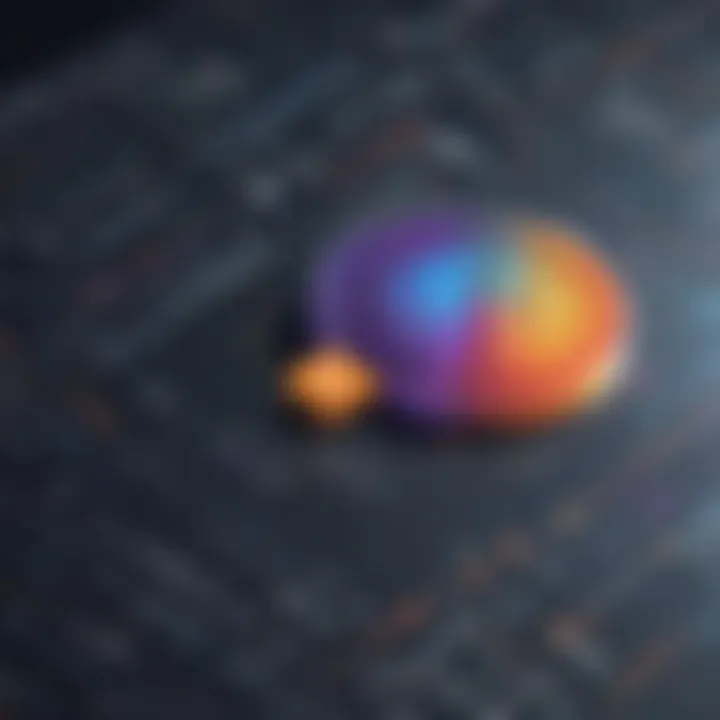
Risk Assessment
Risk assessment evaluates potential threats and vulnerabilities within business operations, guiding risk mitigation strategies and contingency planning. By quantifying risks and assessing their potential impact, organizations can prioritize resources and interventions to minimize adverse outcomes. This systematic approach enables proactive risk management and resilience-building, safeguarding business continuity and reputation. However, risk assessment involves inherent uncertainties and assumptions, requiring periodic reassessment and adaptation to evolving risk landscapes.
Prescriptive Analysis
Recommendation Systems
Recommendation systems utilize algorithms to offer personalized suggestions or content to users based on their preferences and behaviors. By analyzing historical data and user interactions, these systems predict relevant items or services that align with individual needs, enhancing user engagement and satisfaction. Recommendation systems enhance customer experiences and drive sales by guiding informed decision-making and fostering repeat interactions. However, effectiveness depends on the quality of data inputs and algorithmic accuracy, influencing the relevance and impact of recommendations.
Optimization Strategies
Optimization strategies focus on maximizing efficiency and performance through systematic planning and resource allocation. By identifying bottlenecks and inefficiencies, organizations can streamline processes and enhance productivity. Optimization strategies aim to meet objectives with minimal resources, balancing trade-offs and constraints to achieve optimal outcomes. However, implementing optimization strategies requires careful analysis, rigorous testing, and continual monitoring to ensure effectiveness and sustainability.
Simulation Models
Simulation models create virtual representations of real-world scenarios to simulate outcomes and assess various strategies. By modeling complex systems and interactions, organizations can test different approaches and predict potential consequences before implementation. Simulation allows stakeholders to evaluate risks, evaluate decisions, and optimize strategies in a controlled environment, minimizing real-world impacts. However, constructing accurate simulation models demands detailed data inputs and precise assumptions, affecting the reliability and applicability of simulation results.
Applications of Data Analysis
In the realm of data analysis, businesses leverage various techniques to extract insights for informed decision-making and growth. Understanding different types of data analysis methods is crucial for professionals looking to harness technology effectively. Within the context of this article, the section on Applications of Data Analysis explores the pivotal role data analysis plays in enhancing business strategies and operational efficiencies. By delving into Business Intelligence, Financial Analysis, Healthcare Analytics, and Marketing Optimization, this segment sheds light on how data-driven approaches can revolutionize diverse sectors.
Business Intelligence
Market Segmentation
Market Segmentation within the realm of Business Intelligence is a critical tool for businesses to segment target audiences based on shared characteristics. By identifying specific market segments, organizations can tailor their strategies to meet the unique needs and preferences of distinct customer groups. The key characteristic of Market Segmentation lies in its ability to enhance personalized marketing efforts, leading to more effective customer engagement. Despite its advantages in optimizing marketing campaigns, organizations must navigate challenges such as data accuracy and segmentation implementation to maximize the benefits of this approach.
Competitor Analysis
Competitor Analysis is an integral component of Business Intelligence that enables companies to gain insights into their competitors' strategies and performances. By studying competitors' strengths and weaknesses, organizations can refine their own tactics to stay ahead in the market. The essence of Competitor Analysis lies in its capacity to drive competitive advantage and innovation through benchmarking against industry peers. While this analysis empowers businesses to make informed decisions, companies must navigate concerns such as data collection reliability and competitive landscape dynamics for accurate evaluations.
Performance Monitoring
Performance Monitoring, a cornerstone of Business Intelligence, entails tracking key performance indicators (KPIs) to assess organizational performance. By monitoring metrics such as sales figures, customer satisfaction levels, and operational efficiency, companies can gauge their progress towards strategic objectives. The significance of Performance Monitoring rests in its ability to foster continuous improvement and align business activities with desired outcomes. Despite the advantages of this monitoring approach in enhancing decision-making, organizations need to address challenges related to data integration and real-time reporting for comprehensive analyses.