Unveiling the Advanced Technology Behind Artificial Intelligence Evolution
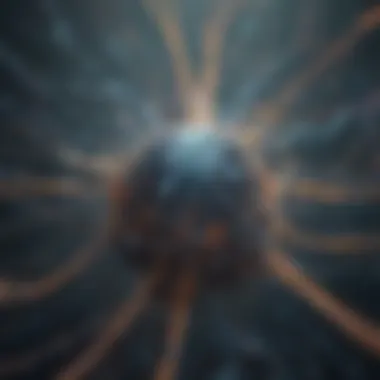
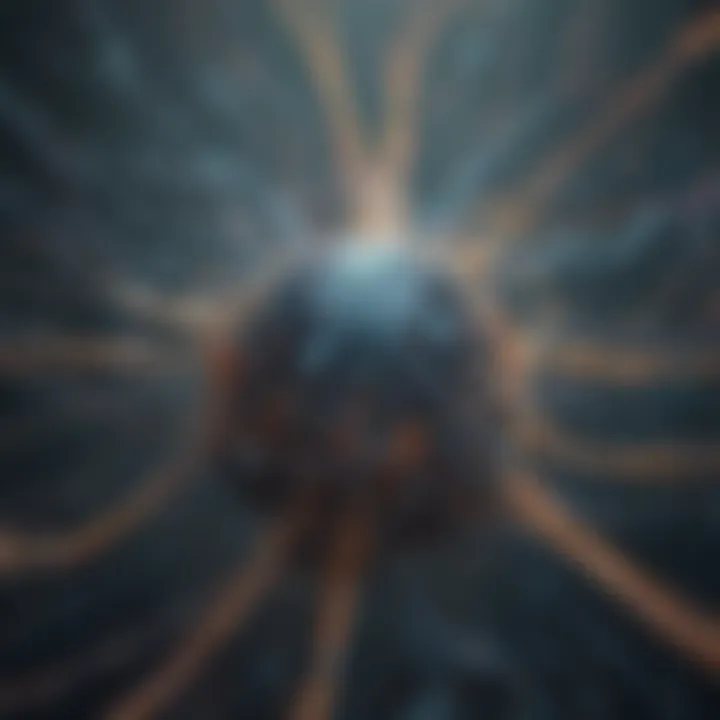
Technological Research Overview
The realm of artificial intelligence (AI) is a captivating blend of cutting-edge technology and innovative applications. This section pries into the intricate web woven by AI technology, unraveling the fundamental components shaping the landscape of artificial intelligence innovation. From the intricate networks of neural pathways to the sophisticated algorithms powering machine learning capabilities, a detailed exploration awaits the reader.
Recent Technological Innovations
AI's journey is peppered with momentous technological breakthroughs, from the inception of neural networks to the evolution of deep learning frameworks. Attend closely as we dissect these milestones and their significance in the upward trajectory of artificial intelligence advancement.
Impact on Business Operations
The marriage of AI technology and business operations has heralded a new era of efficiency and productivity. We unveil the transformative impact AI exerts on streamlining processes, enhancing decision-making, and fostering unparalleled growth within diverse industries.
Future Technological Trends
Gaze into the crystal ball of AI innovation as we forecast the imminent trends poised to revolutionize the technological landscape. From the integration of AI in everyday operations to the prolific rise of data-driven decision-making, the horizon gleams with promises of unprecedented transformations.
Introduction
Artificial intelligence (AI) is a transformative technology that has revolutionized the way we approach problem-solving and decision-making. In today's fast-paced world, where data is abundant and complex, AI serves as a beacon of innovation, offering capabilities that go beyond human capacity. This section will serve as a gateway into the intricate world of AI technology, shedding light on its components and mechanisms.
Defining Artificial Intelligence
Understanding AI in Modern Context
When delving into the realm of AI in a modern context, it becomes evident that the field has evolved significantly, witnessing remarkable advancements in recent years. The focus here lies on the application of AI in real-world scenarios, where machines mimic human cognitive functions to perform tasks efficiently. Leveraging algorithms and data, AI has become instrumental in predictive modeling, automation, and problem-solving. The essence of understanding AI in a modern context lies in its ability to adapt and learn from vast datasets, enabling machines to make accurate decisions swiftly. This section will explore the significance of this adaptability and its impact on various industries.
Evolution of AI Technology
The evolution of AI technology traces a fascinating journey from the initial concept of artificial intelligence to its current sophisticated applications. Over the decades, AI has transitioned from rule-based systems to machine learning and deep learning algorithms, embodying a new era of intelligence. Understanding the trajectory of AI's evolution is crucial in grasping the complexities and potentials of modern AI systems. Despite challenges and controversies, the evolution of AI continues to shape the technological landscape significantly, paving the way for groundbreaking innovations. This section will delve deep into the historical aspects of AI's evolution, highlighting key milestones and technological shifts.
Significance of AI in Today's Tech Landscape
AI's Impact on Industries
Artificial intelligence's impact on industries is undeniable, with its ability to streamline operations, improve efficiency, and drive innovation. Across sectors such as healthcare, finance, and manufacturing, AI is transforming traditional processes, offering new insights through data analytics and automation. The incorporation of AI technologies has revolutionized production lines, customer service, and decision-making, fostering a competitive edge for organizations embracing these innovations. This section will elucidate the multifaceted impact of AI on various industries, showcasing real-world examples of successful AI integration.
Benefits of Implementing AI
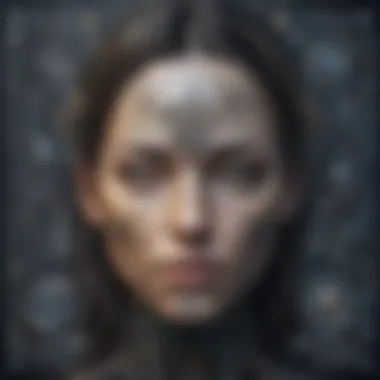
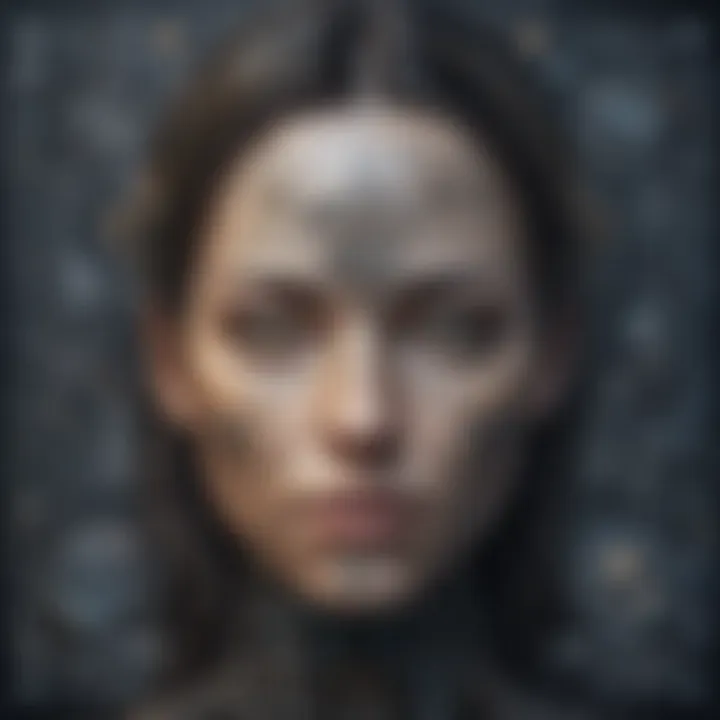
Implementing AI systems unlocks a plethora of benefits for organizations, ranging from enhanced productivity to superior customer experiences. By automating repetitive tasks, AI frees up human resources to focus on higher-value activities, fostering creativity and strategic thinking. Additionally, AI-powered insights enable data-driven decision-making, leading to improved outcomes and reduced operational costs. The benefits of implementing AI extend beyond efficiency gains, offering organizations a strategic advantage in a data-driven economy. This section will explore the tangible advantages of integrating AI solutions and the considerations associated with such implementations.
Overview of AI Technology
Foundational Concepts of AI
At the core of AI technology lie foundational concepts that form the basis of intelligent systems. From problem-solving algorithms to pattern recognition techniques, these concepts define the capabilities of AI systems. Understanding the foundational concepts of AI is crucial for grasping the underlying mechanisms that enable machines to exhibit cognitive abilities. Key topics such as neural networks, expert systems, and natural language processing form the building blocks of AI technology, shaping its diverse applications across industries. This section will provide a comprehensive overview of these foundational concepts, elucidating their significance in the AI landscape.
Key Components of AI Systems
AI systems consist of intricate components that work synergistically to process information, learn from data, and make informed decisions. The key components of AI systems encompass hardware, software, algorithms, and data, each playing a vital role in the functioning of intelligent systems. Understanding these components is essential for developing robust AI solutions that meet the requirements of diverse use cases. From data preprocessing to model training and deployment, each component contributes to the overall performance and efficacy of AI systems. This section will dissect the key components of AI systems, highlighting their interdependencies and implications for AI development.
Core Technologies in Artificial Intelligence
This section of the article focuses on the core technologies that drive advancements in artificial intelligence (AI). Understanding these technologies is pivotal in grasping the underlying mechanisms of AI systems. Core technologies such as neural networks, machine learning algorithms, natural language processing (NLP), and computer vision play a fundamental role in shaping the capabilities of AI. By delving into these technologies, readers can gain valuable insights into the foundation of modern AI innovations, making this section crucial for comprehending the intricacies of AI development. Exploring the significance of these technologies unveils their profound impact on various industries and highlights the benefits they offer in enhancing operational efficiency and decision-making processes within businesses. Additionally, considering the ethical implications of integrating these core technologies in AI systems is essential to ensure responsible and ethical AI deployment.
Neural Networks
Neural networks are a critical component of AI systems, mimicking the human brain's neural structure to process complex data and extract meaningful patterns. Their structure and functionality are key aspects that enable the learning capabilities of AI models, allowing them to recognize patterns, make predictions, and optimize outcomes. Highlighting the importance of neural networks in AI underscores their role in enhancing machine learning processes and achieving remarkable accuracy in tasks such as image and speech recognition. The different types of neural networks, including convolutional neural networks (CNNs) and recurrent neural networks (RNNs), offer unique features that cater to specific AI applications. Understanding the various types of neural networks equips developers with the knowledge to choose the most suitable architecture for their AI projects based on performance requirements and data characteristics.
Structure and Functionality
The structure and functionality of neural networks encompass interconnected layers of artificial neurons that transmit and process information through weighted connections. This architecture allows neural networks to learn from data inputs, adjust their internal parameters, and improve accuracy over time. The ability of neural networks to adapt to changing data dynamics and optimize predictive outcomes makes them a versatile and powerful tool in AI development. Leveraging the parallel processing capabilities of neural networks enhances computational efficiency and enables complex pattern recognition tasks, making them a valuable asset in various industry domains.
Types of Neural Networks
The diverse types of neural networks, including feedforward neural networks, recurrent neural networks, and self-organizing maps, cater to specific AI applications by offering distinct benefits and functionalities. Feedforward neural networks are primarily used in pattern recognition tasks, while recurrent neural networks excel in sequence modeling and time-series analysis. Self-organizing maps are instrumental in clustering and dimensionality reduction tasks, providing efficient solutions to complex data processing challenges. Understanding the nuances of each neural network type empowers practitioners to design AI models that align with project requirements and target outcomes effectively.
Machine Learning Algorithms
The integration of machine learning algorithms is a cornerstone of AI development, enabling systems to learn from data, adapt to new information, and improve performance autonomously. Supervised learning, unsupervised learning, and reinforcement learning represent key categories of machine learning algorithms, each contributing unique capabilities to AI systems. Exploring the intricacies of these algorithms shed light on how AI models leverage data to make informed predictions, discover hidden patterns, and optimize decision-making processes. Understanding the merits and limitations of each algorithm category guides practitioners in selecting the most suitable approach for their AI applications based on data availability and learning objectives.
Supervised Learning
Supervised learning utilizes labeled data to train AI models, allowing them to map input features to output labels and make accurate predictions on unseen data. The key characteristic of supervised learning lies in its ability to learn from annotated examples, enabling systems to generalize patterns and extrapolate insights from training data. This approach is beneficial in tasks where clear outcome labels are available, such as image classification and regression analysis. While supervised learning excels in scenarios with well-defined target variables, it may face challenges in handling unstructured data or complex decision-making processes that require extensive labeled datasets for training.
Unsupervised Learning
In contrast to supervised learning, unsupervised learning focuses on extracting patterns and relationships from unlabeled data without predefined target labels. The key characteristic of unsupervised learning lies in its ability to uncover hidden structures within data autonomously, enabling AI models to identify clusters, anomalies, or correlations without explicit guidance. This approach is advantageous in scenarios where the underlying data patterns are unknown or when exploring intrinsic data properties is the primary objective. However, unsupervised learning may struggle with interpretability issues and scalability concerns, particularly in complex datasets with high dimensionality.
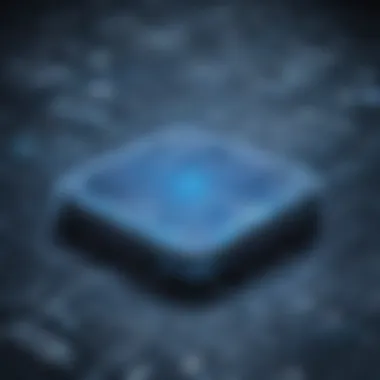
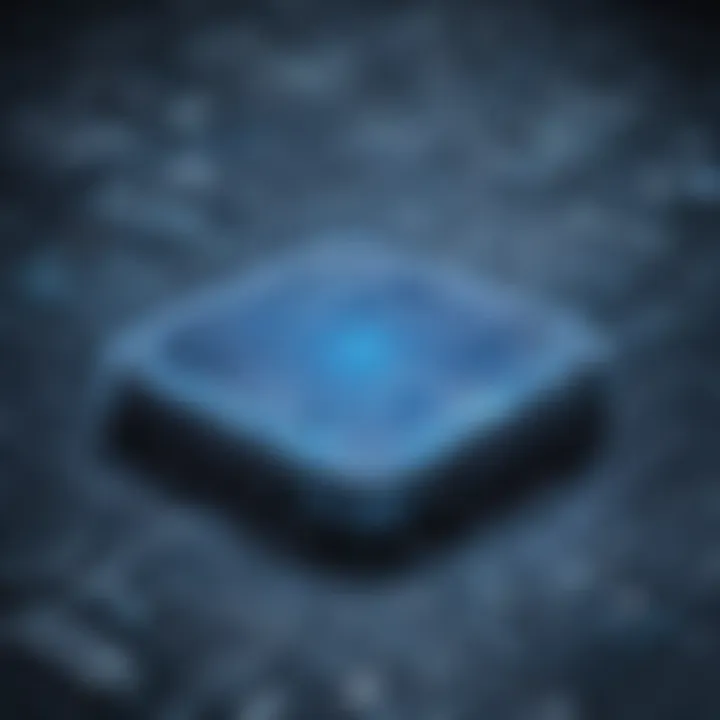
Reinforcement Learning
Reinforcement learning operates on a reward-based system, where AI agents learn optimal behavior through trial and error interactions with an environment. The key characteristic of reinforcement learning lies in its ability to balance exploration of new actions and exploitation of known strategies to maximize cumulative rewards. This approach is beneficial in dynamic environments where decisions have delayed consequences or when there is a trade-off between short-term gains and long-term objectives. Reinforcement learning excels in tasks such as game playing, robotics control, and resource allocation, where adaptive decision-making and continuous learning are essential for achieving desired outcomes.
Natural Language Processing (NLP)
Natural language processing (NLP) is a specialized branch of AI that focuses on enabling machines to understand, interpret, and generate human language. The mechanisms underlying NLP algorithms facilitate text processing, sentiment analysis, language translation, and speech recognition, revolutionizing communication and content analysis capabilities. Examining the intricate workings of NLP mechanisms unveils the underlying principles that drive language processing tasks, enabling AI systems to extract semantic meaning, context, and sentiment from textual data. The applications of NLP in AI systems span a wide range of domains, including chatbots, virtual assistants, sentiment analysis tools, and language translation services, demonstrating the versatility and impact of NLP technologies in reshaping human-machine interactions and content processing workflows.
Understanding NLP Mechanisms
The complexity of NLP mechanisms lies in their ability to deconstruct and analyze linguistic patterns, syntactic structures, and semantic relationships within textual data. By leveraging techniques such as tokenization, part-of-speech tagging, and named entity recognition, NLP models can extract meaningful insights from unstructured text and derive actionable intelligence for various applications. Understanding the intricacies of NLP mechanisms equips developers with the knowledge to design robust language processing pipelines, ensuring accurate and efficient text analysis in AI systems. The evolution of NLP mechanisms reflects continuous improvements in language understanding capabilities, driving advancements in machine translation, sentiment analysis, and contextual language modeling.
Applications in AI Systems
The integration of NLP applications in AI systems enhances human-machine interactions by enabling natural language understanding and generation functionalities. Chatbots powered by NLP algorithms can engage in seamless conversations with users, addressing queries, providing recommendations, and offering personalized assistance based on user input. Virtual assistants leverage NLP technologies to interpret voice commands, perform automated tasks, and access relevant information sources, streamlining daily activities and enhancing user productivity. The widespread applications of NLP in sentiment analysis tools enable businesses to analyze customer feedback, social media interactions, and textual content sentiment, allowing for data-driven decision-making, targeted marketing strategies, and sentiment-based insights into customer preferences.
Computer Vision
Computer vision is a cutting-edge field of AI that focuses on enabling machines to interpret and analyze visual information from the surrounding environment. Image recognition and object detection are key components of computer vision systems, allowing AI models to understand and process digital images or video streams effectively. Exploring the capabilities of computer vision unveils its role in diverse applications such as autonomous vehicles, facial recognition systems, healthcare imaging, and quality control measures. The advancements in computer vision technologies have revolutionized industries by offering automated image analysis, visual inspection tools, and intelligent surveillance systems that enhance efficiency, accuracy, and decision-making processes.
Image Recognition
Image recognition algorithms enable AI systems to identify and categorize objects, patterns, or features within digital images, enabling automated analysis and classification of visual content. The key characteristic of image recognition lies in its ability to extract visual features, detect spatial relationships, and classify image contents based on learned patterns. This capability is crucial in applications such as medical imaging diagnosis, security surveillance, and product identification, where accurate visual understanding is essential for making informed decisions. Image recognition algorithms continue to evolve, leveraging deep learning architectures and computer vision techniques to achieve state-of-the-art performance in image classification, object localization, and semantic segmentation tasks.
Object Detection
Object detection algorithms empower AI systems to locate and track specific objects or attributes within images or video frames, contributing to enhanced situational awareness and object localization tasks. The key characteristic of object detection lies in its ability to identify multiple objects in complex scenes, assign bounding boxes, and classify object categories with high precision. This functionality is vital in applications such as autonomous driving, retail analytics, and industrial inspection, where accurate object detection and recognition are paramount for ensuring safety, efficiency, and compliance. Object detection algorithms leverage deep learning frameworks, such as Faster R-CNN and YOLO, to achieve real-time object localization and detection capabilities, enabling rapid decision-making in dynamic visual environments.
Advanced AI Technologies
In the landscape of artificial intelligence, advanced AI technologies play a pivotal role in the progression and application of cutting-edge innovations. These technologies encompass sophisticated algorithms and models that enable AI systems to perform intricate tasks and learn from data effectively. Understanding advanced AI technologies is imperative for professionals and decision-makers seeking to leverage the full potential of artificial intelligence in various industries. By exploring deep learning, reinforcement learning, and generative adversarial networks (GANs), individuals can grasp the depth and breadth of AI capabilities and their impact on technological advancements.
Deep Learning
Deep Neural Networks
Deep neural networks form the backbone of deep learning, a subset of advanced AI technologies. These networks are structured to mimic the human brain's neural connections, allowing for complex data processing and pattern recognition tasks. The key characteristic of deep neural networks lies in their ability to learn hierarchical representations of data, enabling them to handle intricate tasks such as image and speech recognition. The unique feature of deep neural networks is their capacity to automatically extract meaningful features from raw data, leading to superior performance in tasks requiring sophisticated analysis. While deep neural networks offer unparalleled capabilities in handling complex data, they often require vast amounts of labeled data for training, which can be a drawback in certain scenarios.
Applications of Deep Learning
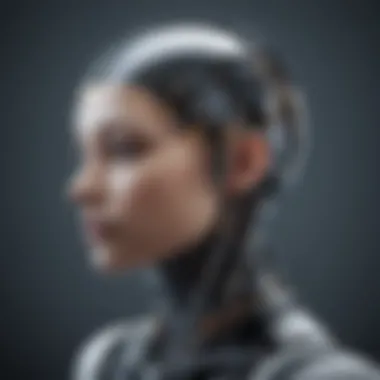
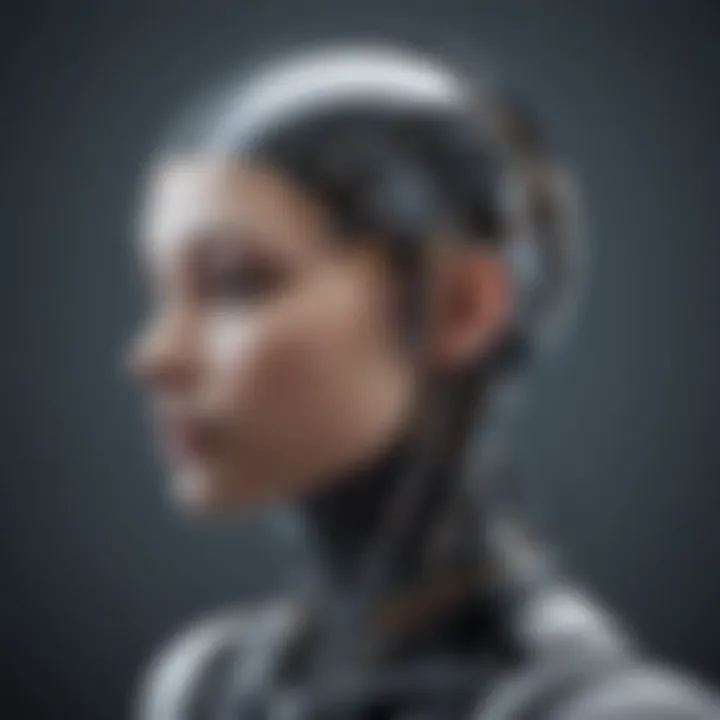
Applications of deep learning span various domains, including image recognition, natural language processing, and autonomous driving. The key characteristic of these applications lies in their ability to learn from large datasets and make intelligent decisions based on patterns and correlations within the data. Deep learning is a popular choice in AI systems for its superior performance in tasks such as image classification and sentiment analysis. The unique feature of deep learning applications is their adaptability to new data and scenarios, making them highly versatile in dynamic environments. However, the significant computational resources required for training deep learning models can pose challenges in resource-constrained settings.
Reinforcement Learning
Exploration vs. Exploitation
Reinforcement learning entails the balance between exploration (trying out new actions to gather more information) and exploitation (leveraging known information to maximize rewards). The key characteristic of this balance lies in optimizing long-term outcomes by deciding when to explore and when to exploit. In this context, exploration allows for discovering potentially better strategies, while exploitation focuses on exploiting known strategies for immediate gains. The unique feature of exploration vs. exploitation is its dynamic nature, where the agent adapts its behavior based on the observed rewards and exploration outcomes. While reinforcement learning offers significant potential in complex decision-making scenarios, the trade-off between exploration and exploitation can be a critical factor in achieving optimal performance.
Reward Systems in RL
Reward systems in reinforcement learning serve as the feedback mechanism that guides the learning process towards desired outcomes. The key characteristic of reward systems lies in their ability to reinforce or discourage specific actions taken by the learning agent based on the received rewards. Rewards act as signals indicating the desirability of a particular action, shaping the agent's future decision-making processes. The unique feature of reward systems is their adaptability to diverse environments and tasks, allowing for flexible learning based on varying reward structures. However, designing effective reward systems that align with the desired behavior can be a challenging aspect of reinforcement learning, requiring careful consideration of task objectives and agent capabilities.
Generative Adversarial Networks (GANs)
GAN Architecture
Generative adversarial networks (GANs) consist of two neural networks, a generator, and a discriminator, engaged in a competitive training process. The key characteristic of GAN architecture lies in its ability to generate realistic data samples by synthesizing information learned from training data. The generator network creates new instances of data, while the discriminator network evaluates the generated samples, fostering a competitive learning dynamic. The unique feature of GAN architecture is its proficiency in generating diverse and high-quality data samples, making it ideal for tasks like image generation and data augmentation. However, ensuring stable training and mitigating issues like mode collapse presents ongoing challenges in leveraging GANs effectively.
Use Cases of GANs
GANs find applications across various domains, including image generation, style transfer, and data synthesis. The key characteristic of GAN applications lies in their capability to generate realistic data samples indistinguishable from authentic data sources, enhancing data diversity and quality. GANs are a popular choice in AI systems for tasks like image synthesis and anomaly detection due to their generative capabilities. The unique feature of GAN applications is their adaptability to diverse data types, allowing for the creation of novel data instances useful in creative tasks. However, training stable GAN models and ensuring convergence remain ongoing challenges in the practical deployment of GANs in real-world scenarios.
Ethical Considerations in AI Development
Exploring the ethics in AI development is crucial in this article. Understanding the ethical implications surrounding artificial intelligence is paramount in today's technological landscape. It is imperative to delve into the ethical considerations that shape how AI impacts industries and society. By critically analyzing the ethical frameworks within which AI operates, we can ensure that the benefits of AI are maximized while potential risks are mitigated. Ethical considerations guide the responsible development and deployment of AI technologies, fostering trust and reliability in these systems.
Bias and Fairness in AI Systems
Addressing Algorithmic Bias
Delving into addressing algorithmic bias is essential for ensuring the integrity of AI systems. Addressing algorithmic bias involves identifying and rectifying prejudices or discriminatory outcomes that may arise from AI algorithms. By actively combating bias in AI systems, we can promote fairness and inclusivity. The key characteristic of addressing algorithmic bias lies in its capacity to enhance the accuracy and fairness of AI technologies. Addressing algorithmic bias is a vital aspect of AI development, as it ensures that AI systems treat users with equity and objectivity.
Ensuring Fairness in AI
Discussing fairness in AI is pivotal in maintaining ethical standards within AI systems. Ensuring fairness in AI involves implementing mechanisms that guarantee unbiased and equitable treatment for all individuals interacting with AI technology. The key characteristic of ensuring fairness in AI is its ability to uphold ethical principles and prevent discriminatory practices. By prioritizing fairness, AI developers can build trust and credibility in their technologies. Ensuring fairness in AI is a cornerstone of responsible AI development, shaping the societal impact of artificial intelligence.
Transparency and Accountability
Exploring transparency and accountability in AI systems is essential for fostering trust and understanding. Interpretable AI models offer insights into how AI reaches decisions, enabling users to comprehend the reasoning behind AI-generated outcomes. The key characteristic of interpretable AI models is their ability to provide clarity and justification for AI algorithms' behavior. Regulatory frameworks establish guidelines and standards for AI development, ensuring that ethical and legal considerations are upheld. The unique feature of regulatory frameworks lies in their capacity to promote adherence to ethical norms and prevent misuse of AI technologies. Both transparency and regulatory frameworks are pivotal in creating responsible and accountable AI ecosystems.
Data Privacy and Security
Understanding data privacy and security is paramount in safeguarding user information within AI systems. Protecting user data involves implementing measures to prevent unauthorized access or misuse of personal information. The key characteristic of protecting user data is its ability to uphold privacy standards and build user trust. Cybersecurity measures are essential in mitigating potential threats and vulnerabilities within AI systems. The unique feature of cybersecurity measures is their role in enhancing the resilience and security of AI infrastructure. Prioritizing data privacy and cybersecurity is crucial for maintaining the integrity and trustworthiness of AI technologies.
In this 5th section of the article, we will explore the Future Trends in AI Technology to provide a comprehensive understanding of the advancements shaping the AI landscape. Discussing Explainable AI (XAI) is crucial as it highlights the significance of developing AI models that are interpretable and transparent. Interpretable Machine Learning Models play a key role in enhancing the explainability of AI systems by allowing stakeholders to understand the decision-making process. Their unique characteristic lies in providing insights into how AI algorithms reach specific conclusions. Despite their benefits, these models may have limitations in handling complexity and large datasets, making them a vital but nuanced choice. Additionally, AI Explainability focuses on making AI decisions understandable and revealing the reasoning behind AI-driven outcomes. By emphasizing transparency, AI Explainability promotes trust and accountability in AI applications. Its distinctive feature lies in demystifying black-box AI models to enable users to comprehend how decisions are made. However, the trade-off may involve compromising some predictive accuracy for interpretability, making AI Explainability a strategic yet balanced approach in AI development. Consequently, understanding Explainable AI concepts is pivotal in navigating the ethical and operational considerations associated with AI implementation. Moving on to Edge AI, the discussion delves into Decentralized AI Processing, a concept that revolutionizes AI systems by enabling data processing and decision-making at the edge, closer to where the data is generated. Its key characteristic lies in reducing latency and bandwidth requirements by localized data processing, making it a strategic choice for applications demanding real-time insights. The unique feature of Decentralized AI Processing is its ability to empower edge devices with intelligence, enhancing autonomy and operational efficiency. Despite its advantages in efficiency and speed, challenges such as network reliability and security risks may arise, underscoring the importance of careful implementation. Furthermore, exploring the Benefits of Edge Computing in AI illuminates how decentralized data processing enhances AI innovation by enabling distributed computing resources for faster decision-making and lower operational costs. Highlighting the key characteristic of reduced data transfer and enhanced privacy, Edge Computing in AI emerges as an advantageous solution for resource-constrained environments. Its unique feature lies in optimizing resource utilization and scalability by leveraging edge devices for computation, enabling AI applications at the network periphery. However, potential disadvantages include increased complexity in managing distributed systems and ensuring data consistency across varied edge nodes, requiring meticulous design and operational considerations. Considering the integration of Edge AI into diverse industries, understanding its benefits and challenges is crucial for informed decision-making. As we explore the application of AI in Healthcare, Diagnostic AI Systems receive significant attention for their transformative role in enhancing medical diagnosis and decision-making processes. Their key characteristic lies in leveraging AI algorithms to analyze medical data and images for accurate patient diagnosis, making them a popular choice for improving healthcare outcomes. The unique feature of Diagnostic AI Systems is their ability to detect patterns and anomalies in medical data with unprecedented precision, leading to timely and accurate diagnostic results. While their advantages include improved diagnostic accuracy and efficiency, challenges such as data bias and regulatory compliance need careful consideration to ensure ethical and reliable implementation. Likewise, delving into Personalized Medicine Advancements sheds light on how AI technologies are revolutionizing medical treatments by tailoring therapies to individual patients based on genetic, lifestyle, and environmental factors. The key characteristic of Personalized Medicine Advancements lies in providing customized treatment plans that optimize patient outcomes and reduce adverse effects, positioning them as a beneficial choice for fostering precision medicine. The unique feature of Personalized Medicine Advancements is their ability to identify personalized treatment options through advanced data analytics and machine learning algorithms, enhancing treatment efficacy and patient satisfaction. Despite the clear advantages of personalized medicine, challenges related to data privacy, regulatory compliance, and treatment costs necessitate a holistic approach to implementation and oversight. Exploring AI's role in addressing Climate Change, Environmental Applications of AI emerges as a pivotal area for leveraging AI technologies to combat environmental challenges. Its key characteristic lies in enabling data-driven decision-making for sustainable resource management and conservation efforts, making it imperative for promoting environmental sustainability. The unique feature of Environmental Applications of AI is its capacity to analyze vast datasets for insights into climate patterns, ecosystem changes, and pollution levels, facilitating informed environmental policies and actions. While the benefits of environmental applications include improved resource efficiency and environmental monitoring, challenges such as data accuracy and model reliability underline the importance of robust data validation and model calibration. Concomitantly, advancing Sustainable Technology Solutions through AI innovation underscores the transformative potential of AI in developing eco-friendly technologies for sustainable development. Emphasizing the key characteristic of enhancing energy efficiency and reducing environmental footprint, Sustainable Technology Solutions represent a promising choice for fostering green technologies and reducing carbon emissions. The unique feature of Sustainable Technology Solutions is their capacity to drive innovation in renewable energy, waste management, and environmental monitoring, contributing to a greener and more sustainable future. However, challenges such as scalability, integration with existing infrastructure, and economic viability warrant strategic planning and cross-sector collaborations to ensure the successful adoption of sustainable technology solutions.