Unlocking the Potential of Graph Database Technology for Business Success
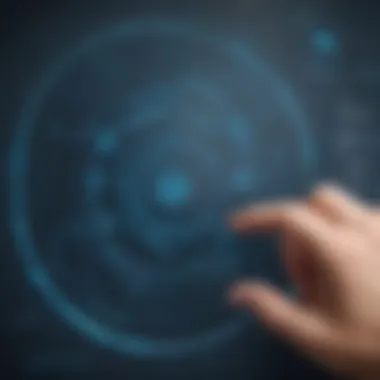
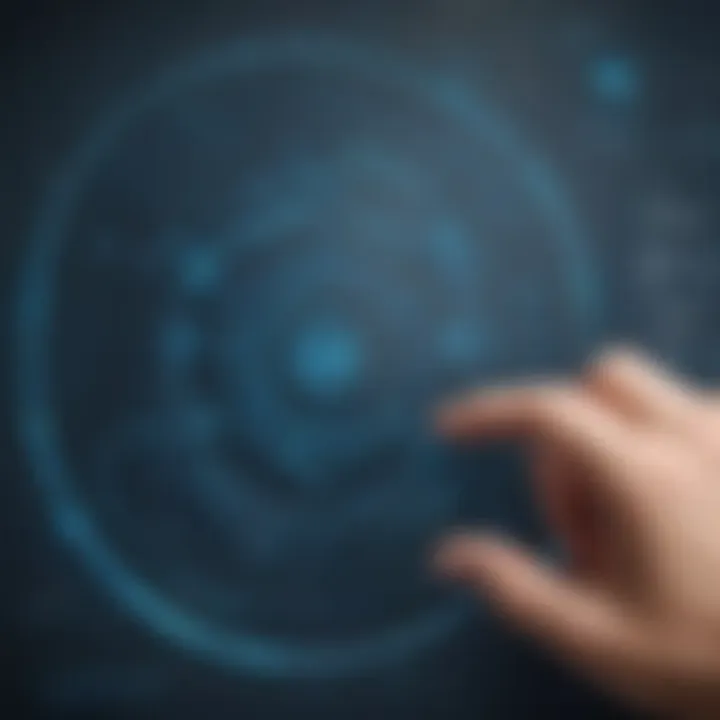
Technological Research Overview
Graph database technology stands at the forefront of modern innovation, reshaping the landscape of data management and analysis. Recent years have witnessed unprecedented advancements in this domain, catapulting businesses into a new era of efficiency and insight. The profound impact of graph databases on various industries is undeniable, revolutionizing the way organizations utilize information to drive growth and competitiveness. As businesses continue to embrace the potential of this technology, it is crucial to explore the key trends and developments shaping its evolution.
Data Analytics in Business
In today's data-driven world, the importance of data analytics cannot be overstated. Businesses rely on data insights to make informed decisions, optimize processes, and gain a competitive edge. Utilizing cutting-edge tools for data analysis empowers organizations to extract valuable information from vast datasets, uncovering hidden patterns and trends. Real-world case studies exemplify the transformative power of data-driven decisions, showcasing how businesses leverage analytics to enhance operations, boost productivity, and drive strategic growth initiatives.
Cybersecurity Insights
Amidst the ever-evolving threat landscape, cybersecurity remains a top priority for organizations across industries. Conducting a thorough analysis of potential risks and vulnerabilities is essential in fortifying digital defenses against cyber threats. Implementing best practices in cybersecurity ensures robust protection of sensitive data and critical infrastructure. Compliance with regulatory standards is imperative, guiding businesses in upholding data security protocols and safeguarding against cyber breaches and intrusions.
Artificial Intelligence Applications
The realm of artificial intelligence (AI) has ushered in a new era of technological innovation, particularly in business automation. Leveraging AI algorithms and applications streamlines workflows, enhances productivity, and empowers organizations to automate repetitive tasks. Ethical considerations in AI deployment are paramount, guiding businesses in maintaining transparency, fairness, and accountability in their utilization of AI technologies. Exploring the diverse applications of AI offers immense potential for improving operational efficiency and driving sustainable growth.
Industry-Specific Research
Tech research within specific industries provides invaluable insights into the unique challenges and opportunities facing various sectors. In the finance industry, technological advancements have revolutionized financial services, offering agile solutions for modern banking and investment practices. Healthcare sectors benefit from the latest tech innovations, enhancing patient care, medical research, and day-to-day operations. Similarly, the retail industry leverages tech solutions to deliver personalized customer experiences, optimize supply chains, and drive digital transformations. Industry-specific research highlights the adaptability and innovation sparked by technological advancements within key sectors of the economy.
Introduction
Graph database technology has emerged as a powerful tool in the realm of data management, revolutionizing how companies leverage information. This article offers a deep dive into the intricacies of graph databases, shedding light on their applications, benefits, and impact on businesses. By unearthing crucial insights and optimizing data management processes, graph databases have paved the way for innovation and growth within the corporate landscape.
Understanding Graph Database Technology
The Basics of Graph Databases
Graph databases operate on the premise of graph theory, where nodes represent entities, and edges depict the relationships between these entities. This unique structure allows for highly flexible and interconnected data models, enabling more nuanced representation of complex relationships compared to traditional relational databases. The graph database's key characteristic lies in its ability to efficiently traverse relationships between data points, facilitating quick and seamless data retrieval. This feature makes graph databases the preferred choice for scenarios requiring real-time querying and analysis of interconnected data sets
Graph Database Structure
The structure of a graph database consists of nodes, relationships, properties, and labels. Nodes serve as the fundamental entities in the database, representing data points such as people, products, or locations. Relationships define the connections between nodes, indicating how the entities are related. Properties are key-value pairs attached to nodes and relationships, offering additional context or information. Labels categorize nodes based on shared characteristics, facilitating efficient data organization and retrieval. While the flexibility of this structure allows for rich data representation and querying capabilities, managing large-scale graph databases can pose challenges in terms of scalability and performance.
Graph Database Fundamentals
Nodes and Relationships
Exploring Nodes in Graph Databases:
Exploring nodes in graph databases is a fundamental aspect that underpins the entire data modeling process. Nodes represent entities or data points, each possessing unique identifiers and properties. By exploring nodes, users can navigate through the intricate network of connections within a graph database, unveiling valuable insights and patterns. The key characteristic of exploring nodes lies in its ability to traverse the graph efficiently, uncovering relationships and facilitating data discovery. This exploration process is highly beneficial in discerning complex data interrelationships and extracting meaningful information efficiently.
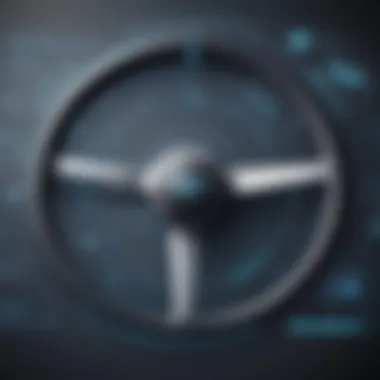
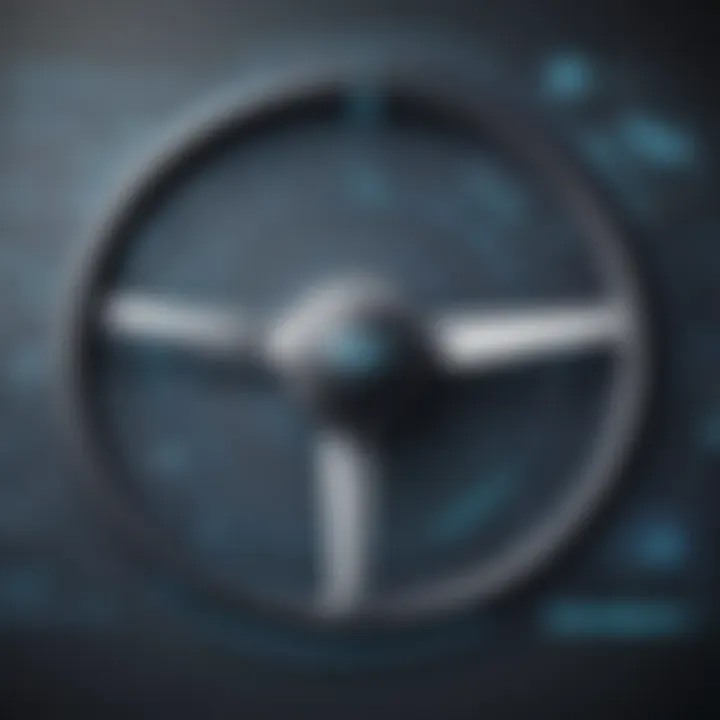
Relationships in Graphs:
Relationships in graphs define the connections or interactions between nodes, establishing the core essence of graph database technology. These relationships depict how different entities are linked, providing context and significance to the data elements. The key characteristic of relationships lies in their ability to capture the nuances of data relationships, fostering a holistic view of the interconnected data points. Leveraging relationships in graphs enhances data querying and analytical capabilities, enabling users to extract valuable insights from complex datasets effectively. Despite some complexities in managing intricate relationships, the advantages of relationships in graph databases outweigh the challenges, offering a robust framework for data representation.
Properties and Labels
Understanding Properties in Graphs:
Properties in graph databases encapsulate the attributes or characteristics of nodes and relationships, enriching the dataset with meaningful information. Understanding properties is paramount in distinguishing and categorizing data points based on their specific features. The key characteristic of properties lies in their ability to facilitate precise data retrieval and manipulation, streamlining data management processes. Embracing properties in graph databases enhances data organization and fosters streamlined data access, promoting efficient data analysis and decision-making.
Labels in Graph Databases:
Labels in graph databases categorize nodes based on common characteristics or attributes, enabling systematic data classification and retrieval. Utilizing labels offers a structured approach to organizing data elements within a graph database, simplifying data operations and enhancing data querying efficiency. The unique feature of labels lies in their role in grouping related nodes, allowing for targeted data retrieval and manipulation. Despite potential complexities in managing a diverse range of labels, their benefits in enhancing data organization and retrieval make them a valuable asset in optimizing graph database operations.
Applications of Graph Databases
In this segment, we delve into the crucial realm of Applications of Graph Databases, shedding light on its profound significance within the broader context of this article. By exploring the various specific elements, benefits, and considerations related to Applications of Graph Databases, we aim to provide a comprehensive understanding of how this technology is reshaping industries. From optimizing social networking platforms to revolutionizing fraud detection mechanisms, graph databases offer a multitude of applications that are instrumental in driving innovation and efficiency.
Social Networks
Enhancing Social Networking Platforms
Delving into the sphere of Enhancing Social Networking Platforms, we uncover a key aspect of leveraging graph databases. This specific component plays a pivotal role in enhancing the overall functionality and user experience of social networking platforms. The unique characteristic of Enhancing Social Networking Platforms lies in its ability to personalize recommendations and connections based on intricate network patterns, thus fostering deeper user engagement. Despite certain limitations, such as scalability challenges with vast user bases, Enhancing Social Networking Platforms remains a popular choice for companies seeking to enhance user interactions and content relevance.
Recommendation Systems
Exploring the domain of Recommendation Systems within graph databases, we uncover a vital tool for enhancing user experience and driving business success. The core feature of Recommendation Systems lies in their capacity to analyze vast amounts of user data to generate personalized recommendations, thus increasing user retention and satisfaction. While these systems offer numerous advantages, such as improved customer engagement and sales, they may face challenges in handling diverse data sets and ensuring data privacy. Nevertheless, Recommendation Systems stand out as a valuable asset for companies looking to boost user engagement and loyalty.
Fraud Detection
Utilizing Graph Databases for Fraud Detection
Within the realm of fraud detection, the utilization of graph databases presents a powerful solution for identifying and preventing fraudulent activities. The key characteristic of leveraging graph databases for fraud detection lies in their ability to uncover complex relationships and irregular patterns within vast data sets, facilitating proactive risk mitigation. Despite their efficacy, challenges such as data integration issues and real-time processing constraints may pose hurdles in implementing these systems effectively. Nonetheless, the advantages of utilizing graph databases for fraud detection outweigh the drawbacks, making them a strategic choice for organizations aiming to safeguard their operations.
Network Analysis
Examining the significance of Network Analysis in the context of graph databases, we unveil a critical methodology for deciphering interconnected data points and structures. The primary benefit of Network Analysis lies in its capacity to reveal hidden connections and anomalies within complex networks, enabling organizations to enhance decision-making and streamline operations. While Network Analysis offers numerous benefits, such as improved risk assessment and predictive insights, organizations may encounter challenges related to data accuracy and visualization complexities. Nevertheless, the inclusion of Network Analysis in graph databases showcases its value in facilitating informed decision-making and optimizing business processes.
Knowledge Graphs
Building Knowledge Graphs
The creation of Knowledge Graphs emerges as a fundamental aspect of graph databases, unlocking a reservoir of interconnected information for enhanced decision-making. The key characteristic of building Knowledge Graphs lies in their ability to connect disparate data points to form a cohesive knowledge framework, empowering organizations to derive valuable insights and correlations. Despite challenges in managing evolving data sources and ensuring data quality, the advantages of Knowledge Graphs in fostering innovation and enabling smarter data-driven decisions are indisputable. As organizations strive to harness the power of data, building Knowledge Graphs stands out as a strategic approach for fostering knowledge discovery and organizational growth.
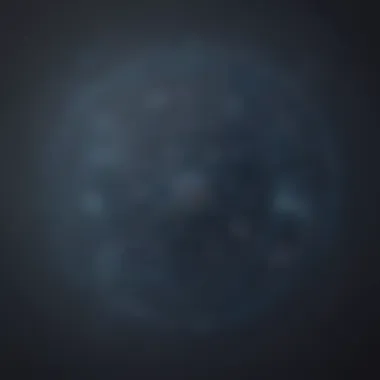
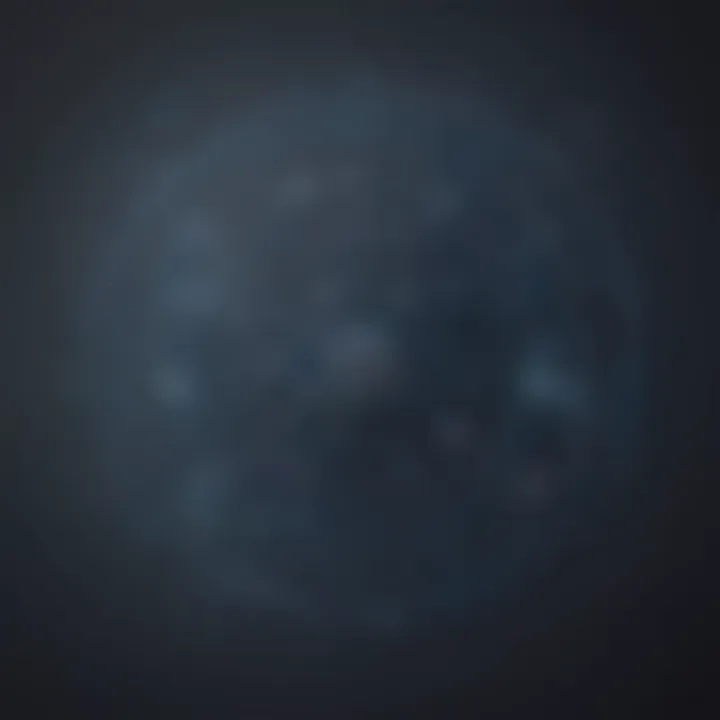
Semantic Search
Exploring the realm of Semantic Search within graph databases, we unveil a powerful tool for enhancing information retrieval and semantic understanding. The primary feature of Semantic Search lies in its capability to interpret user queries contextually and deliver relevant results based on semantic relationships, thereby improving search accuracy and user experience. While Semantic Search offers notable benefits, such as enhanced query precision and semantic enrichment, challenges such as ambiguous query interpretation and limited language support may pose obstacles. Nonetheless, the integration of Semantic Search in graph databases showcases its potential in revolutionizing information retrieval and knowledge discovery processes.
Benefits of Graph Database Technology
In the realm of database technology, the benefits of utilizing graph databases cannot be overstated. Graph databases offer a unique approach to data management, allowing for the representation of complex relationships between entities with unmatched efficiency. One crucial aspect to consider when delving into the benefits of graph database technology is its ability to enhance scalability and performance while maintaining flexibility and agility in data operations.
Scalability and Performance
Enhanced Scalability
When we speak of enhanced scalability in the context of graph database technology, we are highlighting the exceptional capability of these databases to handle a growing amount of data without compromising performance. Unlike traditional relational databases, graph databases excel in scaling horizontally as data volumes increase, ensuring that operations maintain optimal speed and efficiency. The key characteristic that sets enhanced scalability apart is its ability to seamlessly accommodate additional nodes and relationships, making it a popular choice for dynamic and expanding datasets. This unique feature not only ensures consistent performance but also enables businesses to adapt to evolving data requirements efficiently.
Optimized Performance
Optimized performance is another critical aspect that distinguishes graph databases from other conventional options. The primary focus here is on maximizing query speed and resource utilization to deliver rapid responses to complex data queries. By employing index-free adjacency, graph databases streamline query processing by directly traversing relationships between nodes, resulting in expedited retrieval of interconnected data points. This approach boosts overall system performance and lowers latency, making optimized performance a preferred solution for applications demanding real-time insights and rapid data processing. Despite its advantages in query optimization, it is essential to consider potential trade-offs such as increased storage requirements when leveraging optimized performance capabilities in graph database technology.
Flexibility and Agility
Adaptable Data Modeling
Flexibility is a cornerstone of effective data modeling, and graph databases excel in providing adaptable structures for diverse datasets. Adaptable data modeling allows businesses to define and modify relationships between entities on-the-fly, enabling seamless adjustments to accommodate shifting business requirements. The key characteristic of adaptable data modeling lies in its schema-free nature, empowering users to modify data models without the constraints of predefined schemas. This flexibility not only enhances data agility but also caters to changing needs in dynamic environments, making adaptable data modeling a preferred choice for organizations seeking nimble and scalable data management solutions.
Agile Querying
Agile querying plays a pivotal role in ensuring responsive data retrieval and analysis within graph database environments. By leveraging powerful query languages like Cypher, users can swiftly extract valuable insights from interconnected data sets with intuitive and efficient queries. The key characteristic of agile querying lies in its ability to express complex relationships and patterns succinctly, facilitating seamless data exploration and decision-making processes. This method not only accelerates query performance but also fosters a user-friendly querying experience, enhancing overall data accessibility and usability. Despite its advantages in query optimization, considerations such as query complexity and performance overhead should be weighed when implementing agile querying strategies in graph database technology.
Challenges and Considerations
In this section, we delve into the crucial aspect of challenges and considerations within the realm of graph database technology. Understanding and addressing these challenges are paramount for organizations seeking to harness the full potential of graph databases. By highlighting the key obstacles and nuances involved, companies can navigate effectively through the intricacies of implementing and utilizing graph databases. These considerations play a significant role in shaping strategies, optimizing performance, and ensuring data integrity, ultimately impacting the success of graph database utilization in various applications.
Data Consistency
Maintaining Data Integrity
Exploring the facet of maintaining data integrity unveils a fundamental pillar in the graph database landscape. Data integrity signifies the accuracy, consistency, and reliability of information stored within the database, ensuring that the data remains trustworthy and valid. Maintaining data integrity is crucial for organizations relying on precise insights for their operations and decision-making processes. The unique characteristic of maintaining data integrity lies in its ability to uphold the sanctity of data throughout its lifecycle, fostering confidence in the information presented. Despite its proactive nature, challenges may arise in upholding data integrity in dynamic environments, necessitating robust mechanisms for validation and correction. By prioritizing data integrity, businesses can fortify the foundation of their analytical frameworks and enhance overall efficiency.
Consistency Models
Delving into consistency models illuminates the diverse strategies employed to maintain data accuracy and synchronization in graph databases. Consistency models dictate how and when updates to the database are propagated across the system, ensuring a harmonized view of data for all users and applications. The key characteristic of consistency models lies in balancing data consistency with system performance, striking a delicate equilibrium between real-time updates and operational efficiency. Adopting suitable consistency models is vital for streamlining data access, minimizing conflicts, and optimizing the scalability of graph database deployments. However, different consistency models present varying trade-offs, influencing factors such as latency, fault tolerance, and resource utilization. Understanding the nuances of consistency models is critical for effectively managing data consistency in diverse operational scenarios and tailoring solutions to meet specific business requirements.
Query Complexity
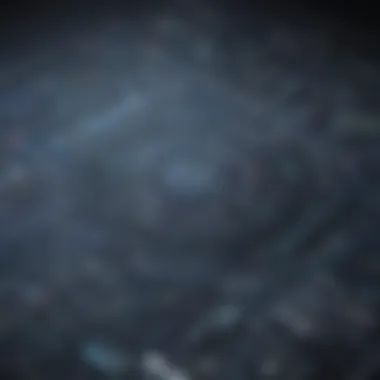
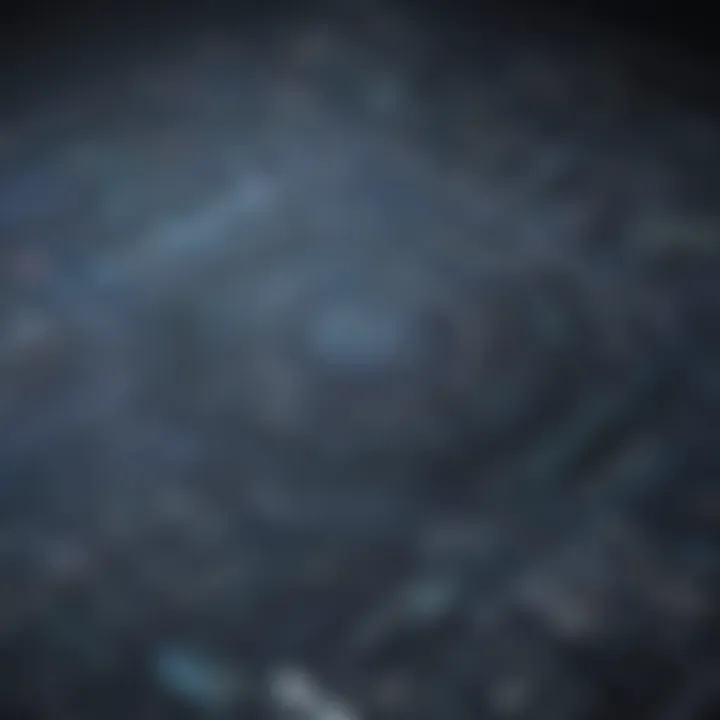
Complex Query Patterns
Navigating complex query patterns unveils the intricate nature of extracting meaningful insights from graph databases efficiently. Complex query patterns encompass sophisticated data retrieval strategies, encompassing a diverse range of queries spanning multiple nodes and relationships. The key characteristic of complex query patterns lies in their ability to unearth interconnected insights, revealing intricate relationships and patterns within the data landscape. Optimal utilization of complex query patterns empowers organizations to uncover hidden correlations, streamline decision-making processes, and drive strategic initiatives. However, the complexity of these queries can pose challenges in terms of performance optimization, resource allocation, and query execution time. Balancing the intricacy of query patterns with system responsiveness is essential for maximizing the value derived from graph database queries and unlocking actionable intelligence.
Performance Optimization
Delving into performance optimization sheds light on strategies aimed at enhancing the efficiency and responsiveness of graph database operations. Performance optimization encompasses a spectrum of techniques designed to streamline query processing, minimize response times, and maximize resource utilization. The key characteristic of performance optimization lies in its focus on iterative refinement, continual monitoring, and adaptive tuning to meet evolving performance demands. Implementing robust performance optimization strategies is imperative for ensuring the scalability, agility, and competitiveness of graph database applications. However, optimizing performance entails a nuanced balance between resource allocation, query complexity, and system constraints, calling for a systematic approach to identifying bottlenecks and implementing targeted enhancements. Embracing performance optimization best practices enables organizations to leverage the full potential of graph databases, bolstering decision-making capabilities and driving innovation across diverse domains.
Real-world Implementations
In this section, we delve into the practical applications of graph database technology, shedding light on how various industries leverage this innovative tool to revolutionize their operations. Real-world implementations play a pivotal role in highlighting the adaptability and versatility of graph databases in different organizational settings. By examining how leading companies incorporate graph database technology into their systems, we can grasp the tangible benefits and emerging trends in the field.
Case Studies
Leading Industry Use Cases
Exploring leading industry use cases provides invaluable insights into the diverse ways graph databases are utilized across different sectors. These case studies exemplify the strategic advantages gained by companies adopting graph database technology, showcasing its ability to enhance data analysis, streamline processes, and improve decision-making. By closely analyzing these use cases, we can draw parallels between their success stories and the potential opportunities graph databases present for businesses seeking data-driven solutions.
Impact on Business Operations
Discussing the impact of graph databases on business operations is essential to understanding their transformative influence on organizational efficiency and performance. By examining specific instances where graph databases have optimized workflows, enhanced customer experiences, and facilitated data-driven decision-making, we gain a comprehensive view of their strategic importance. Highlighting the practical implications of integrating graph database technology in day-to-day operations underscores its role in driving sustainable business growth and fostering competitive advantages in the marketplace.
Future Trends and Innovations
In this section on Future Trends and Innovations, we delve into the critical aspects that shape the trajectory of graph database technology. As the digital landscape rapidly evolves, staying abreast of emerging trends is paramount for businesses seeking a competitive edge. Understanding the future trends equips organizations to adapt, innovate, and optimize their data strategies effectively. By discerning the direction in which graph databases are heading, companies can harness their full potential and drive sustainable growth. Emphasizing the significance of trend forecasting and proactively integrating innovations into existing infrastructures can catalyze organizational success in a dynamic market environment.
Emerging Technologies
Integration with AI
Integration with AI stands as a pivotal domain intersecting with graph database technology. This symbiotic relationship between AI and graph databases empowers organizations to extract profound insights and unlock new opportunities. The integration enhances data processing capabilities, enabling sophisticated pattern recognition, and predictive analytics. AI augments the querying and analytical prowess of graph databases, enabling deeper understanding and decision-making. The key characteristic of integrating AI with graph databases lies in the synergy between machine learning algorithms and graph patterns, fostering enriched data exploration and knowledge discovery. Despite the nuanced complexities inherent in deploying AI within graph databases, the benefits encompass heightened accuracy in predictive modeling, trend analysis, and anomaly detection, enriching the data interpretation landscape.
Blockchain Integration
Blockchain integration presents a transformative juncture within the realm of graph database technology. The amalgamation of blockchain and graph databases revolutionizes data security, transparency, and decentralization paradigms. A fundamental aspect of blockchain integration lies in immutability and consensus mechanisms, ensuring data integrity and audit trails within interconnected networks. The indelible nature of blockchain transactions, coupled with graph database structures, amplifies trust and reliability in data interactions. This integration not only fortifies data integrity but also streamlines processes by eliminating intermediaries and enhancing peer-to-peer transactions. While blockchain integration offers unparalleled data security benefits, challenges like scalability and interoperability warrant meticulous considerations to optimize its utilization within graph database ecosystems.
Conclusion
Graph database technology stands at the forefront of innovation, heralding a new era in data management and analysis. As we journeyed through the intricate web of nodes, relationships, and properties, it became evident that the essence of graph databases lies in their ability to unearth valuable insights and optimize information flow within organizations. The importance of our conclusion extends beyond mere data manipulation; it signifies a paradigm shift towards more agile and efficient data modeling and querying processes. By delving deep into the core principles and practical applications of graph databases, we have unraveled the potential they hold for transforming businesses across various sectors.
The Future of Graph Databases
Continued Evolution
Continued evolution within the realm of graph databases epitomizes the constant strive for optimization and enhancement. This perpetual process aims to refine the existing structures, algorithms, and functionalities of graph databases to meet the ever-evolving demands of modern enterprises. The significance of continued evolution lies in its iterative nature, fostering a culture of continuous improvement and adaptation. By integrating new features, addressing performance bottlenecks, and enhancing scalability, continued evolution ensures that graph databases remain at the cutting edge of technological innovation. While the evolving landscape of graph databases presents unique challenges, such as compatibility issues during upgrades and potential disruptions to established workflows, the benefits outweigh the risks by ushering in a new era of efficiency and effectiveness.
Impact on Businesses
The impact of graph databases on businesses resonates with the core essence of driving growth, fostering innovation, and empowering decision-making processes. Businesses leveraging graph databases witness a transformation in data clarity, actionable insights, and strategic decision-making capabilities. The key characteristic of this impact lies in its ability to streamline operations, enhance customer experiences, and uncover hidden patterns within vast datasets. By enabling faster query executions, facilitating complex data connections, and fostering a deeper understanding of business dynamics, the impact on businesses catalyzes a data-driven culture that propels enterprises towards sustainable growth and competitive advantage. While integrating graph databases into existing infrastructures may pose initial integration challenges and require upskilling of personnel, the long-term advantages translate into enhanced efficiency, improved decision-making, and accelerated innovation within businesses.