Harnessing SAS for Innovation in Data Science
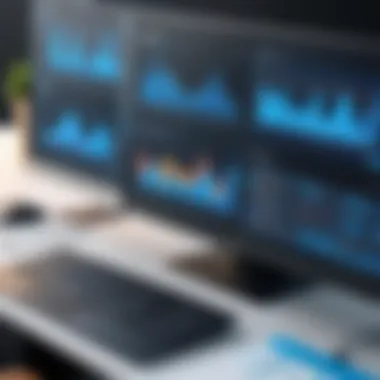
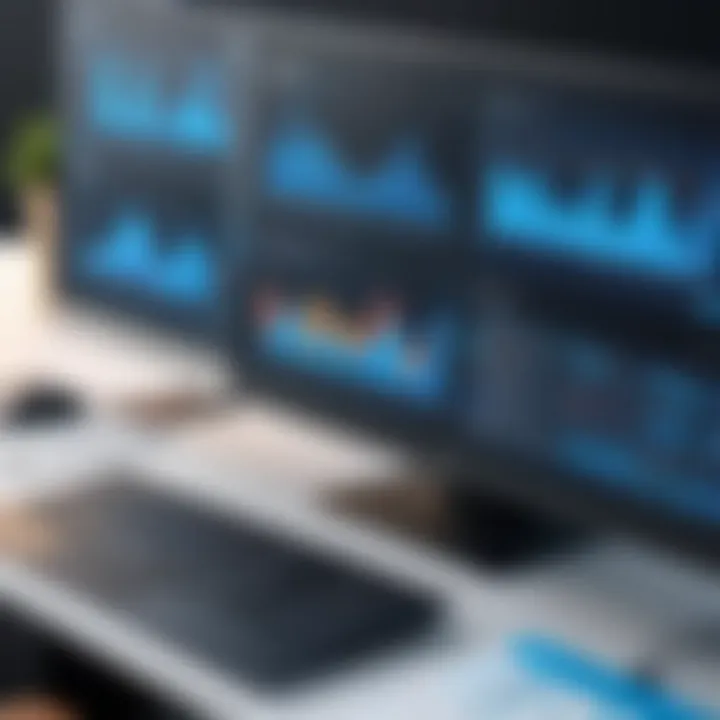
Intro
In today’s fast-paced business landscape, the role of data science has grown beyond mere analysis, becoming a cornerstone of innovation and strategic decision-making. SAS software stands out as a powerful tool, blending statistical analysis with user-friendly interfaces, enabling businesses to interpret vast amounts of data. Its stature in the sphere of data science is not just due to its capability but also because of its ability to intertwine analytics with core business functions.
This narrative explores the essential components of harnessing SAS in data science. It will unfurl the capabilities of SAS, demonstrate its applications in various sectors, and spotlight its influence on driving innovation. That being said, let’s embark on this exploration of technological research, diving into how recent innovations shape business operations and the future trends we can expect to see.
Technological Research Overview
Recent Technological Innovations
The technological landscape is constantly evolving, with SAS at the helm of integrating new capabilities. Recent advancements have included better integration with big data platforms such as Hadoop and cloud environments. These innovations enable businesses to merge structured and unstructured data seamlessly, providing a 360-degree view of performance and insights.
Moreover, advancements in machine learning algorithms within SAS are helping businesses to automate not just analytical tasks but decision processes as well. With features like predictive analytics, organizations can anticipate market shifts and act proactively rather than reactively.
Impact on Business Operations
SAS’s ability to analyze and interpret data influences various aspects of business operations. It enhances decision-making protocols by providing real-time insights and forecasts which were previously unimaginable. Organizations can now rely on data-driven decision-making rather than gut feeling. This reliance significantly improves operational efficiency and enables businesses to identify opportunities for cost-saving or expansion.
Furthermore, SAS boosts collaboration among departments through shared data capabilities. Think about an organization where marketing, finance, and operations have different perspectives; with SAS, they can converge on data to collaboratively generate strategies.
Future Technological Trends
Looking ahead, key trends predict further integration of artificial intelligence with SAS for more robust predictive modeling and enhanced user personalization. On the horizon, incorporating natural language processing will enable non-technical users to engage with data through everyday language, breaking down barriers and democratizing data access.
As businesses continue to navigate complexities within their industries, the strategic advantages gained from tools like SAS will become ever more essential in maintaining a competitive edge.
Data Analytics in Business
Importance of Data Analytics
Data analytics transcends beyond simply parsing numbers; it’s about uncovering stories hidden within the data. Understanding patterns can be pivotal for a business looking to innovate or optimize its offerings. SAS serves as a means of turning raw data into actionable insights, allowing businesses to adapt based on observed trends.
Tools for Data Analysis
SAS offers a suite of tools catering to a spectrum of analytical needs. For instance:
- SAS Visual Analytics helps users generate reports and dashboards that are intuitive and data-driven.
- SAS Enterprise Miner provides an environment for data mining and creating predictive models.
These tools collectively arm professionals with the means to conduct rigorous analysis, streamline processes, and tailor strategies to better meet customer needs.
Case Studies on Data-Driven Decisions
Particularly in sectors like retail, SAS has revolutionized inventory management. By applying predictive analytics, retailers can maintain optimal stock levels based on sales forecasts. For example, a major grocery chain used SAS to analyze customer purchase patterns, which led to improved product placement and stock management. The result? A significant increase in sales and customer satisfaction.
Finale
SAS embodies a key player in the data science arena, essential for businesses striving to innovate and adapt in an ever-evolving landscape. Whether the focus is on technological advancements, ensuring data analytics serves core business operations, or preparing for the next wave of possibilities, SAS provides the tools necessary for strategic growth and decision-making. As we continue to probe the depths of data, tools like SAS will anchor businesses on their journey towards data-driven excellence.
Prolusion to SAS and its Importance in Data Science
SAS software has become a cornerstone in the world of data science, particularly in how businesses innovate and make decisions. Understanding SAS's role is crucial because it offers a robust platform that simplifies complex analyses and fosters data-driven insights.
Organizations today are inundated with data—from financial transactions to consumer behavior metrics. SAS provides the tools needed to make sense of this wealth of information. It streamlines data management, enhances predictive analytics, and ensures that firms can act on insights swiftly. By leveraging SAS, businesses can not only keep pace with competitors but also set the tone in their respective industries.
A essential element of SAS's importance is its comprehensive analytics capabilities. This software supports everything from initial data exploration to intricate statistical modeling. For decision-makers looking for accurate and timely insights, SAS ensures that the data tells a story that is easily interpretable.
Defining SAS Software
SAS, which stands for Statistical Analysis System, is a software suite developed for advanced analytics, multivariate analysis, business intelligence, and data management. It's not just any old software; it’s highly respected for its ability to manipulate and analyze complex datasets.
At the heart of SAS lies its user-friendly interface, which includes both a point-and-click option for those who prefer a graphical approach and a programming interface for more technical users. It facilitates data integration from various sources, ensuring that users can pull together insights from disparate locations into a cohesive overview.
One major selling point of SAS is its versatility. Companies spanning sectors such as finance, healthcare, and retail utilize SAS to address their unique needs, whether it's assessing risk or optimizing operations.
Historical Context of SAS in Data Science
SAS's journey began in the late 1960s when it was developed to analyze agricultural data. Fast forward to today, it has morphed into a critical player in the digital landscape of data science. Initially, it catered primarily to statisticians, but over the decades, its offerings have expanded massively, incorporating data mining, machine learning, and even artificial intelligence.
The popularization of SAS coincided with the growing need for organizations to harness data effectively. As businesses transitioned into the data era in the 1990s, SAS became increasingly relevant. Its ability to handle large datasets and provide detailed analytics made it the tool of choice for many organizations seeking to leverage data for strategic advantages.
Through the years, SAS has navigated the technological landscape by consistently updating its functionalities. Today, it remains at the forefront of data science tools, evolving with trends like big data and cloud computing. The historical significance of SAS in shaping the data science field cannot be overstated; it paved the way for the automated and integrated approaches we see today in business intelligence.
"SAS has not just adapted to the changes in data science; it has been a catalyst for many of those changes."
Key Features of SAS for Data Analysis
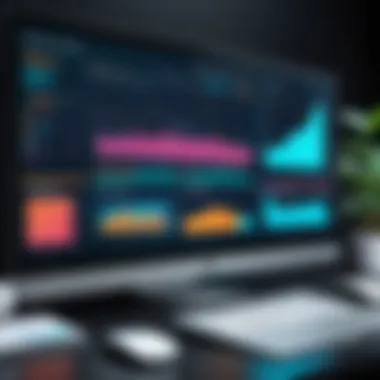
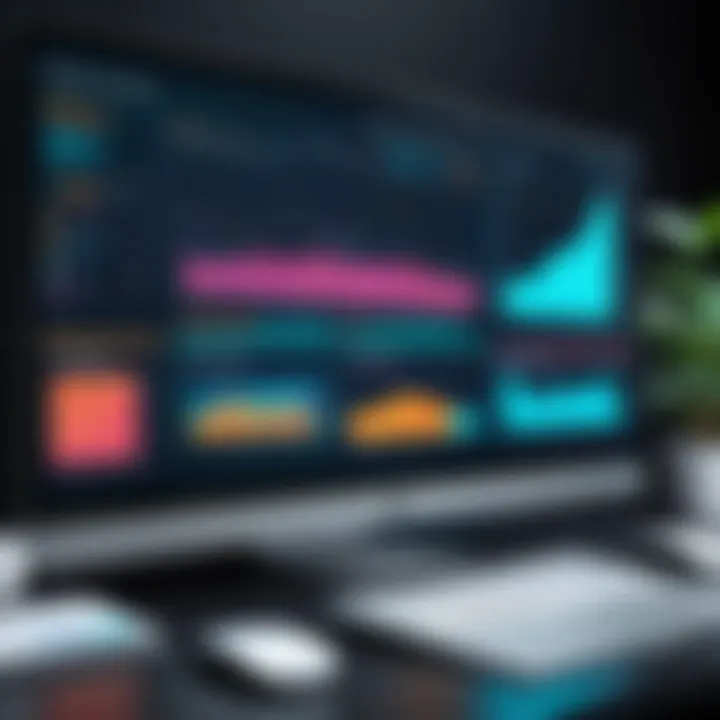
SAS holds a significant position in the arena of data analytics, offering a robust suite of tools tailored to meet the diverse needs of businesses today. The key features of SAS resonate well with decision-makers seeking to harness the power of data, enabling them to drive innovation and improve outcomes across various functions. By understanding these features, businesses can use SAS effectively to enhance their data analysis capabilities.
Data Management Capabilities
One of SAS's standout aspects is its data management capabilities. The software excels in organizing, cleaning, and transforming data into useful formats, facilitating easy analysis. Here are a few specific elements that highlight SAS's prowess in data management:
- Data Integration: SAS has the ability to connect to multiple data sources, including databases, spreadsheets, and big data environments. This means businesses can pull together information from various places to get a comprehensive view.
- Data Preparation: Data never comes in a neat package. SAS provides numerous tools for data cleaning and wrangling. It helps users to deal with missing values, duplicate records, and inconsistent formatting. This preparatory work is essential to ensure that subsequent analyses yield reliable results.
- Scalability: As businesses grow, so does their data. SAS can handle vast datasets with ease, making it suitable for companies of all sizes—from startups to enterprises. Its performance remains steady, even when the volume of data soars.
These capabilities allow users to ensure that they're working with clean, integrated datasets, which is critical for any successful data analysis project.
Advanced Analytics Functions
SAS isn't just about crunching numbers; it offers a spectrum of advanced analytics functions. This feature becomes crucial when diving into more sophisticated analyses. Here's a closer look at what SAS provides:
- Predictive Analytics: With built-in techniques for forecasting and regression analysis, SAS equips users to predict future trends based on historical data. This can be invaluable for strategic planning.
- Machine Learning: The platform supports machine learning algorithms that can automate decision-making processes and identify patterns in data that may not be immediately apparent.
- Text Analytics: SAS can also analyze unstructured data, like text from surveys or social media. This can help businesses glean insights into customer sentiments, market trends, or operational issues that require attention.
These advanced features empower organizations to not only analyze data but also to derive actionable insights critical for innovation and competitiveness.
Statistical Analysis and Reporting
SAS has a rich legacy in statistical analysis, which is a core strength of the platform. The statistical analysis and reporting features provide users with the ability to make sense of their data through rigorous testing and validations. Some important elements include:
- Statistical Procedures: SAS comes equipped with a wide range of statistical functions, from descriptive statistics to more complex inferential statistics. This versatility allows users to conduct thorough analyses.
- Visualization Tools: Equally important is the ability to visualize data findings. SAS offers tools for creating graphs and charts, making insights more accessible for stakeholders. A well-crafted visual can often convey a message more powerfully than numbers alone.
- Automated Reporting: SAS allows users to automate the reporting process. This means that businesses can generate periodic reports easily, enabling quick decision-making based on current data insights.
Being able to conduct robust analyses and translate those into clear reports is key for business leaders aiming to foster data-driven cultures.
"In today's fast-paced business world, having the right insights at your fingertips can make all the difference between success and stagnation."
The combination of these features sets SAS apart in the realm of data analytics. With a strong foundation in data management, advanced analytical capabilities, and comprehensive reporting tools, SAS remains a cornerstone for businesses looking to leverage data effectively.
SAS in Predictive Analytics
In today's rapidly changing business world, predictive analytics has become a cornerstone for organizations aiming to make informed decisions. SAS offers powerful tools designed to harness the potential of predictive modeling, allowing businesses to forecast trends, understand customer behavior, and uncover hidden insights. Being able to leverage predictive analytics effectively can be the difference between getting left behind and staying ahead of the competition.
Concept of Predictive Modeling
Predictive modeling is the process where statistical techniques are used to create models that predict future outcomes based on historical data. It’s fundamentally about making educated guesses using data. Picture this: a retail company looks at its sales data from the past five years. By applying predictive modeling, they can forecast which products might fly off the shelves next season based on trends, consumer behavior, and market conditions.
In essence, predictive modeling transforms raw data into actionable insights. Important techniques often employed include regression analysis, decision trees, and neural networks. Each technique serves a unique purpose, contributing to a more comprehensive understanding of the dynamics at play in various sectors. With SAS, organizations can build and refine these models with relative ease. The user-friendly interface combined with robust analytical capabilities simplifies what could otherwise be a complicated process.
Implementation of Predictive Models with SAS
Implementing predictive models using SAS is more than a simple task; it's a systematic approach that intertwines various features of the software for optimal results. First off, data preparation stands as a critical step in this process. Data must be cleaned and formatted correctly, ensuring that it's ready for analysis. SAS provides extensive data management capabilities, allowing users to quickly manipulate and organize data sets, thereby giving them the groundwork they need for modeling.
Once the data is prepped, users can employ SAS's suite of statistical tools to kickstart their predictive modeling journey. The capabilities extend to:
- Regression Analysis: Useful for predicting a dependent variable, such as sales figures, based on one or more independent variables like marketing spend.
- Decision Trees: Providing a visual representation of decisions and their possible outcomes, assisting in understanding complex data interactions.
- Neural Networks: Ideal for identifying patterns and making predictions in large datasets, particularly useful in customer segmentation.
After developing these models, validation is key. SAS allows for various testing methods, including cross-validation, ensuring that the model performs well not just on training data but also on unseen data. Moreover, built-in functionality helps visualize results, allowing teams to interpret them easily and make strategic decisions based on solid evidence.
"Predictive analytics is not about predicting the future; it is about making the future predictable."
In essence, by integrating SAS into the predictive modeling process, businesses can enhance their forecasting abilities, turning data into a strategic asset that drives innovation and growth.
Applications of SAS in Business
SAS software plays a crucial role in transforming data into actionable insights across various industries. By leveraging the capabilities of SAS, businesses can not only enhance operational efficiency but also drive innovation through informed decision-making. Each industry has its unique set of applications, yet the core benefits remain consistent: improved analysis, prediction, and overall strategy alignment.
Financial Services Industry
Risk Management
Risk management is one of the standout applications of SAS in the financial services sector. Effective risk management is vital for banks and financial institutions to ensure compliance and safeguard against potential losses. SAS allows users to analyze vast datasets, identifying potential risks tied to credit, market fluctuations, or operational vulnerabilities.
The key characteristic that makes risk management a popular choice for this article is its comprehensive approach. SAS provides tools that facilitate both quantitative and qualitative assessment of risks. A unique feature of SAS in this space is its ability to simulate scenarios based on historical data, helping organizations foresee potential pitfalls and prepare accordingly. The advantage of such capabilities is clear: businesses can mitigate unexpected shocks and maintain stability in uncertain environments.
Fraud Detection
Fraud detection represents another critical application, especially in financial sectors vulnerable to illicit activities. This aspect focuses not just on identifying fraudulent transactions post-factum but on employing predictive analytics to spot them before they happen. SAS provides a robust toolkit for analyzing transaction patterns and flagging anomalies.
The key characteristic here lies in continuous learning systems—SAS can adapt and evolve by utilizing machine learning techniques. This results in a dynamic approach to fraud detection, making it a go-to choice for many organizations. The unique feature of this tool is its ability to integrate real-time data analysis, enabling swift responses to fraudulent activities. However, the downside might be the reliance on historical data, which could potentially miss emerging fraud patterns.
Healthcare Analytics
Patient Data Analysis
In the healthcare field, patient data analysis has become indispensable. Transforming raw data from various sources into understandable formats can lead to better patient outcomes and more effective treatments. SAS empowers healthcare providers to analyze longitudinal data, identifying patient trends and outcomes.
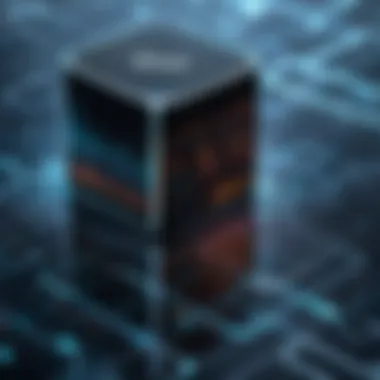
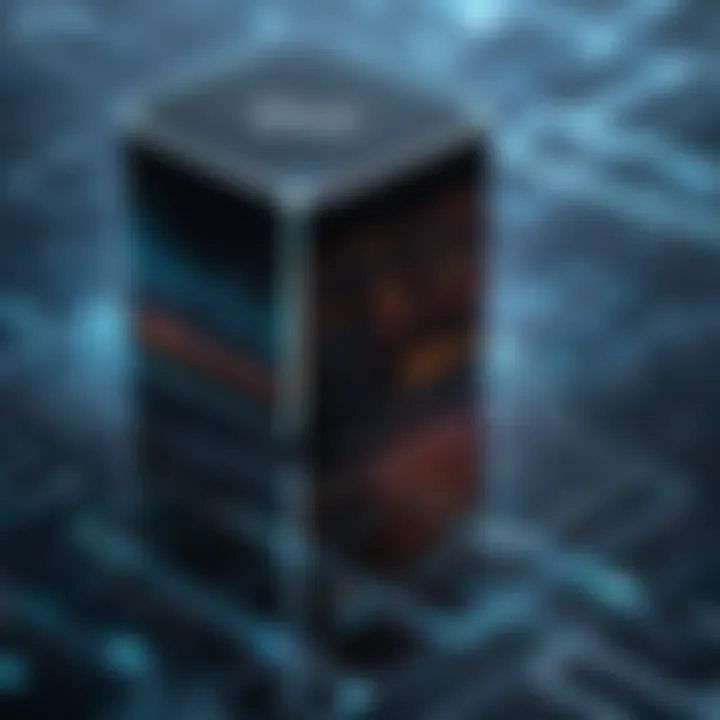
The notable aspect of patient data analysis is its role in facilitating personalized medicine. Hospitals can tailor treatments based on patient history and genetic information, thus improving recovery rates. A unique feature of SAS is its capability to manage and integrate data from various systems. However, efficiency can be hampered if the data is not consistently of high quality.
Operational Efficiency
Operational efficiency is at the heart of healthcare analytics. By optimizing workflow and resource allocation, healthcare institutions can provide better care while reducing costs. SAS tools allow for the identification of bottlenecks and inefficiencies in existing systems.
The key characteristic that makes operational efficiency a focus in this article is its potential to enhance overall healthcare delivery. By accurately predicting patient inflow, facilities can better manage staff assignments, equipment usage, and space allocation. A unique feature of using SAS here is the predictive modeling of patient volumes based on historical data, which enables better staffing. Yet, this predictive accuracy can sometimes be affected by unpredictable external factors like public health crises.
Retail and E-commerce
Customer Behavior Modeling
Understanding customer behavior is paramount for retailers and e-commerce businesses. SAS aids in analyzing purchasing patterns and preferences, enabling businesses to tailor their marketing strategies effectively. This application enhances customer engagement and retention.
The key aspect of customer behavior modeling is its focus on personalization. Organizations can segment their customer base and design targeted campaigns that resonate on an individual level. A unique feature is the advanced analytics that allows for real-time adjustments depending on customer actions. Nevertheless, high reliance on data might lead to privacy concerns among consumers.
Inventory Management
Efficient inventory management is critical for optimizing operational costs and meeting customer demand. SAS tools provide insights into stock levels and sales forecasts, enabling retailers to manage their inventory effectively.
The salient characteristic of inventory management is its dual focus on minimizing excess stock and ensuring product availability. SAS enables a dynamic inventory system that adjusts based on real-time sales data. A distinct feature of SAS in this context is its integration capability with e-commerce platforms, making data-driven decisions more fluent. However, incorrect data inputs can lead to misinformation, causing stocking issues.
The integration of SAS into various business applications provides a dynamic and powerful toolset for organizations looking to innovate and navigate the complexities of data-driven decision-making.
Integrating SAS with Other Technologies
In today’s data-driven landscape, combining SAS with other technologies has become increasingly vital. This integration enables organizations to harness the full potential of their data resources, unlocking deeper insights and driving innovative business solutions. It serves not just to enhance analytical capabilities, but also to improve operational efficiencies. Here, we will look specifically at how SAS can work hand-in-hand with big data technologies and machine learning tools, with a keen eye on their unique contributions.
SAS and Big Data Technologies
Hadoop Integration
When we talk about Hadoop integration, we delve into the world of distributed storage and processing. Hadoop allows SAS to manage enormous datasets effectively, making it a vital player in the realm of data science. One notable characteristic is its ability to handle unstructured data, a feature that many traditional systems struggle with. The synergy between SAS and Hadoop can enhance the data mining processes significantly, which in turn leads to sharper, data-driven insights.
One of the great advantages of this integration is the scalability it offers. Businesses can store and analyze massive amounts of data in a cost-effective manner. Plus, Hadoop's flexibility allows for varied data types, whether it be text, images, or structured numerical data. However, with benefits come challenges—implementing Hadoop alongside SAS can involve a learning curve, especially for teams unaccustomed to big data environments.
Cloud Technologies
In the context of data science, cloud technologies present an appealing option for many organizations. Integrating SAS with cloud services provides a pathway to on-demand resources and enhanced computational power. One key feature of cloud technology is the ease of access; users can seamlessly access SAS analytics tools from anywhere with internet access. This accessibility is crucial for decision-makers who need real-time data insights.
Moreover, cloud services often come with built-in security features, which can ease minds concerning data privacy and risks of breaches. The unique selling point here is the capability of elastic resources. As demand surges, cloud technologies allow businesses to scale up their services swiftly without the hefty investments needed for on-premises infrastructure. On the flip side, dependence on third-party cloud providers does pose risks related to uptime and performance, which organizations must weigh carefully when planning their data strategies.
Collaboration with Machine Learning Tools
As businesses continue to embrace the potential of machine learning, the collaboration between SAS and these tools positions organizations to innovate like never before. SAS can serve as a robust foundation, providing a stable environment to build and deploy machine learning models.
By leveraging SAS's comprehensive suite of analytics, businesses can ensure that their machine-learning projects are grounded in solid data insights. This combination not only augments predictive accuracy but also enhances the interpretability of results, aiding stakeholders in making informed decisions. Moreover, the ability to integrate with various programming languages and frameworks often used in machine learning projects enhances SAS's utility, allowing teams to harness the best of both worlds.
Best Practices for Using SAS in Data Science Projects
Leveraging SAS effectively in data science projects can be the linchpin for achieving innovation and efficiency. This section examines crucial best practices that ensure organizations get the most out of their SAS investment. By adhering to these guidelines, businesses can not only streamline their processes but also extract meaningful insights to drive decision-making. The importance lies in establishing a structured approach that mitigates risks and optimizes outcomes. In a rapidly evolving landscape where data is the currency, these practices outline a path for success.
Developing a Data Strategy
A solid data strategy is the backbone for any effective SAS project. It involves outlining clear objectives and understanding the types of data at your disposal. Organizations must prioritize defining their goals, whether to boost customer retention or streamline operations. If the aim is to enhance customer targeting, teams need to map that against the data they can access. Here are a few steps to consider:
- Identify Objectives: Determine what you hope to achieve with SAS.
- Assess Data Sources: Examine existing data and look for new avenues for data capture.
- Align Stakeholders: Ensure that all relevant parties understand the data strategy and their roles within it.
Incorporating these elements can lay a foundation that guides teams throughout the project, ensuring alignment between business goals and data capability.
Ensuring Data Quality
Data quality is non-negotiable in any SAS initiative. Poor-quality data can lead to misguided strategies and decisions, wreaking havoc across operations. Consistency, accuracy, and relevance should be the watchwords during data preparation. To uphold high data standards, consider these approaches:
- Data Cleansing: Regularly clean your data to remove duplicates, errors, and inconsistencies.
- Validation Processes: Implement checks to validate the accuracy of your data continually.
- Metrics for Quality: Establish key performance indicators (KPIs) to measure data quality over time.
By taking data quality seriously, organizations not only empower their SAS capabilities but also contribute to building a culture of data integrity.
Building a Skilled Team
No technology or software can replace the human insight essential for making effective decisions. Building a skilled team for SAS is critical. This involves not just ensuring that your team members are technically proficient, but also fostering an environment where continuous learning is encouraged. Here’s a breakdown of effective strategies:
- Training Programs: Invest in regular training to keep your team up to speed with SAS updates and best practices.
- Cross-Functional Teams: Encourage collaboration between data scientists, analysts, and business professionals to foster diverse perspectives.
- Recruitment Focus: Look for candidates who possess analytical skills along with a solid understanding of your industry’s specific challenges.
Creating a knowledgeable and versatile team is crucial for not just leveraging SAS tools but also driving the innovative solutions that come with it.
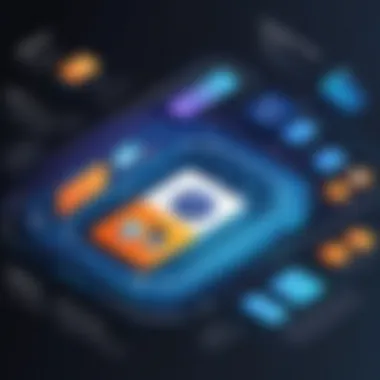
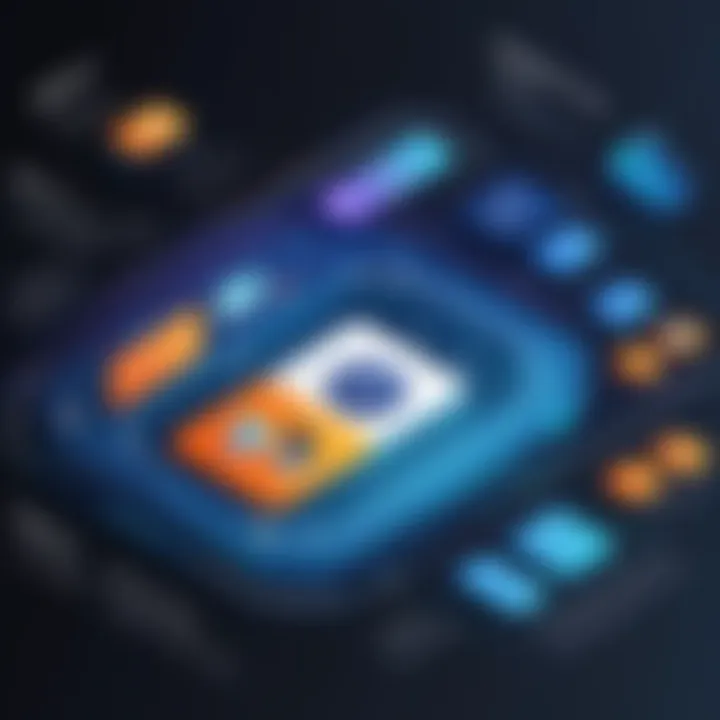
"Data without action is just numbers; action without data is just guesswork."
In summary, best practices surrounding SAS involve careful strategy development, stringent data quality measures, and the cultivation of a skilled workforce. By focusing on these core areas, businesses can fully harness the power of SAS for impactful outcomes.
Challenges in Implementing SAS Solutions
Implementing SAS solutions in a corporate environment isn’t all sunshine and rainbows. While the platform offers powerful tools for data analysis and insights, there are significant challenges that organizations must navigate. These challenges can influence the overall effectiveness of SAS in driving business innovation. Grasping the complexities involved in cost, user adaptation, and compliance is crucial for anyone looking to integrate SAS into their data science strategy.
Cost Considerations
The financial aspect of deploying SAS solutions can be daunting. Organizations often find themselves grappling with initial licensing fees, ongoing maintenance costs, and expenses related to training and support. SAS software is a premium product, and this premium experience comes at a price.
- License Fees: Companies must understand the licensing model for SAS, which usually involves a hefty upfront investment. This can be a deal-breaker for smaller organizations trying to stretch their budget.
- Training Expenses: To fully utilize the features of SAS, investing in training for staff is a must. While SAS does offer documentation, hands-on training often requires external consultants, further inflating costs.
- Hidden Costs: There may be unexpected expenses related to software integration or custom development work that can add up quickly.
Understanding these cost considerations is vital for any business that wishes to leverage SAS effectively.
Learning Curve for Users
SAS, despite its robust capabilities, has a reputation for being somewhat complex. New users may encounter a steep learning curve, which can hinder adoption.
- Interface Complexity: The user interface is rich in features, but it might overwhelm those accustomed to more straightforward tools.
- Programming Knowledge Required: While some functions are user-friendly, many advanced features necessitate a solid understanding of programming within the SAS environment. Users may struggle if they lack this expertise.
- Training Requirements: As mentioned before, committing time and resources to upskill team members is not just beneficial but often mandatory for optimally leveraging SAS.
The proficiency gap can lead to underutilization of the software, making it crucial to consider user training early in the implementation process.
Data Privacy and Compliance Issues
In today’s data-driven landscape, privacy isn’t just a concern; it’s a necessity. SAS users must navigate a maze of data privacy regulations, especially when dealing with sensitive customer information.
- Regulatory Compliance: Depending on the industry, businesses might face strict regulations like GDPR or HIPAA. SAS users need to know how to configure their solutions to meet these requirements.
- Data Management Practices: Ensuring proper data management practices are in place is essential. Poor practices can lead to breaches, risking the integrity of sensitive data and possibly leading to legal issues.
- Ethical Considerations: There’s an ethical obligation to protect data. Organizations should clearly communicate how they handle data to both their internal teams and external clients.
"Navigating the regulations and ethical responsibilities surrounding data is as crucial as understanding the software itself. Successful implementation hinges on balancing innovation with compliance."
In summary, while SAS presents organizations with a world of opportunity in data analytics, overcoming the associated challenges is no small feat. By understanding the cost implications, providing adequate training, and ensuring compliance with data regulations, businesses can better position themselves to reap the full benefits of SAS solutions.
Future Trends in SAS and Data Science
Understanding the future trends in SAS and data science is crucial for staying ahead in an increasingly data-driven business landscape. As companies continually harness data to drive decision making and innovation, embracing advancements in technology becomes imperative. The interplay between SAS tools and emerging trends not only enhances efficiency but also expands the analytical capabilities available to businesses.
AI and automation are reshaping how data is processed, analyzed, and interpreted. As these technologies mature, they promise to streamline processes, reduce human error, and expedite decision-making. Adapting to these changes requires organizations to rethink their data strategies and invest in skills that leverage these innovations effectively.
AI and Automation in SAS
AI's integration into SAS represents a significant leap towards operational excellence. With SAS’s analytics solution, businesses can automate repetitive tasks which significantly minimizes the manual workload. This automation does not merely enhance speed; it brings consistency and reliability to the data analysis process. Furthermore, machine learning capabilities within SAS allow for predictive analytics models that evolve with the data they analyze.
In practical terms, this means that companies can anticipate market trends, improve customer satisfaction, and refine product offerings based on real-time data insights. For instance, if a retail firm uses SAS with AI, it can analyze past buying patterns and optimize inventory accordingly, thus preventing overstock and improving cash flow.
Some benefits of AI and automation within SAS include:
- Enhanced Efficiency: Automated data cleaning and transformation save significant amounts of time.
- Improved Accuracy: Reducing human involvement decreases the risk of errors in data reporting.
- Robust Insights: AI algorithms can uncover patterns humans might miss, leading to more informed decision making.
Adoption of AI also requires a robust framework for integrating these technologies. Organizations need to ensure their data pipelines are equipped for the scale and complexity of AI applications.
Emerging Data Science Practices
The practice of data science is evolving, driven by leaps in technology and changing market demands. The emergence of various frameworks and methodologies reshapes how data analyses are conceived and executed within SAS.
In particular, concepts such as data ethics, collaborative analytics, and real-time processing are becoming increasingly pivotal. These practices resonate with the growing emphasis on responsible data usage while simultaneously ensuring timely insights for competitive advantage.
With real-time analytics, organizations can respond swiftly to emerging trends. When integrated with SAS, this capability allows for a dynamic approach to business challenges; rather than relying on historical data, firms can adapt to changes in real-time.
Additionally, the concept of collaborative analytics is gaining momentum, where data scientists and business stakeholders work closely to glean insights from data analytics. This collaboration can foster a deeper understanding of the analytics narrative and drive the implementation of strategies that better align with business goals.
In summary, the future trends in SAS and data science are not just about tools; they are about redefining how organizations interact with data. As AI and automation grow, accompanied by new data science practices, firms that adapt will lead the charge in innovation and effective decision making.
"Embracing the changes in data science practices lays the groundwork for significant breakthroughs in business strategy.
Finale: The Role of SAS in Shaping Data-Driven Decision Making
Data-driven decision-making is no longer just a trend; it’s an essential component of business strategy in today’s fast-paced market. SAS plays a pivotal role in this paradigm shift. By providing advanced analytical capabilities, it allows organizations to make sense of vast amounts of data, turning raw information into actionable insights. This section delves into the significance of SAS within the context of data-driven decision-making, emphasizing its benefits and core considerations.
The heart of SAS lies in its ability to perform extensive data analysis, which is crucial for creating sound business decisions. Companies that leverage SAS can identify patterns, track performance metrics, and forecast future trends more accurately. These advantages lead to a competitive edge, facilitating more informed investment opportunities and strategic planning initiatives.
Key Benefits of SAS in Decision-Making:
- Enhanced Predictive Analytics: SAS enables organizations to move from traditional methods to predictive analytics. Predictive modeling can forecast outcomes, thereby helping businesses prepare for future challenges.
- Informed Risk Management: Through its comprehensive statistical analysis, SAS helps organizations assess risks before they manifest. This proactive approach is vital for financial institutions and sectors like healthcare.
- Unified Data Management: SAS's data management capabilities ensure that diverse data sources are processed seamlessly. This interoperability streamlines workflows, making data access and processing more efficient.
"In an age where data is deemed the new oil, SAS serves as the refinery, transforming crude data into valuable insights for decision-making."
However, harnessing these benefits comes with its challenges. Organizations must invest in building a skilled workforce capable of utilizing SAS tools effectively. Training can be costly, and the learning curve can be steep. Yet, the reward of strategic decision-making driven by data far outweighs these initial hurdles.
Moreover, businesses must be aware of data privacy regulations as they navigate the complexities of data management. SAS tools must be implemented with strict adherence to compliance standards to avoid legal repercussions.
In summary, SAS is more than just software; it is a comprehensive suite that empowers organizations to essentially shape their future. The capabilities offered by SAS enable businesses to approach decision-making with confidence, backed by data insights and analytical rigor. As firms continue to embrace data-driven strategies, the integration of SAS will likely define all future innovations in their operational practices. This makes understanding and utilizing SAS not just beneficial, but indispensable for any organization looking to thrive in the digital era.