Unveiling the Profound Influence of Machine Learning on Modern Business Practices
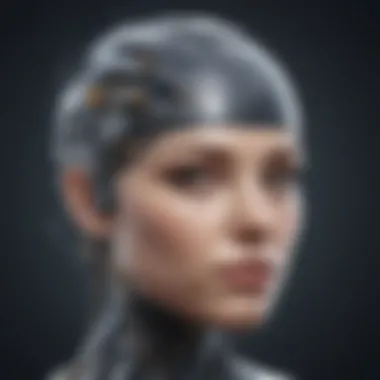
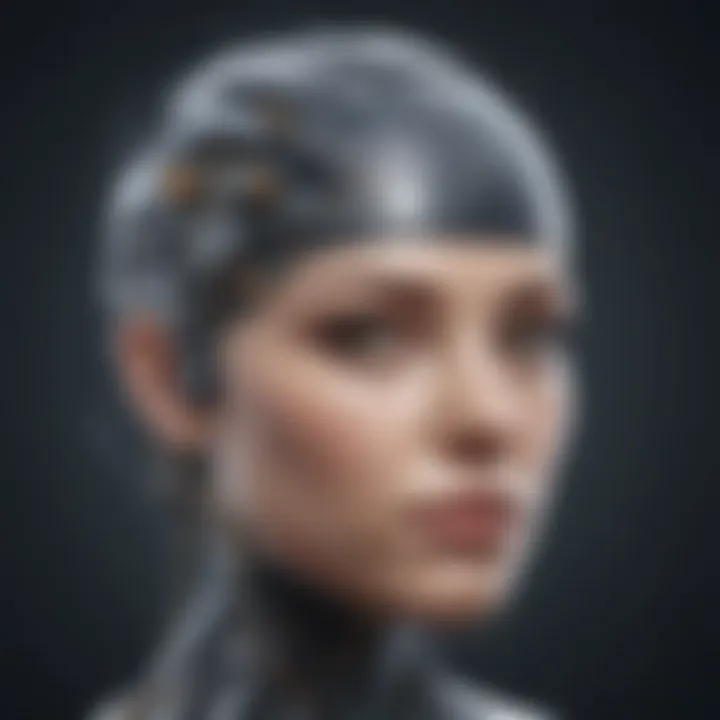
Technological Research Overview
Machine learning, a subset of artificial intelligence, has been revolutionizing various aspects of modern businesses. Recent technological innovations in machine learning have brought forth a seismic shift in how organizations conduct their operations. The impact on business operations is undeniable, with machine learning algorithms powering advanced analytics, forecasting models π, and automation tools π . Looking ahead, future technological trends suggest an even deeper integration of machine learning into business processes, signaling a continued evolution in leveraging data-driven insights π.
Data Analytics in Business
In the realm of business, the importance of data analytics cannot be overstated. Data analytics empowers organizations to extract valuable insights from vast amounts of data, facilitating informed decision-making π§ . Tools for data analysis have become indispensable assets, enabling businesses to visualize trends, identify patterns, and predict outcomes with precision. Case studies on data-driven decisions showcase real-world examples of how leveraging data can drive business strategy, enhance operational efficiency, and foster innovation π.
Cybersecurity Insights
Amid the digital landscape, cybersecurity plays a pivotal role in safeguarding businesses against a dynamic threat landscape. A comprehensive threat landscape analysis is essential for understanding potential vulnerabilities and mitigating risks effectively. Best practices for cybersecurity encompass proactive measures such as regular assessments, robust access controls, and incident response protocols. Moreover, regulatory compliance in cybersecurity ensures that businesses adhere to data protection laws and industry regulations, fostering a secure operating environment π‘.
Artificial Intelligence Applications
Artificial intelligence (AI) is transforming business automation through its ability to replicate human cognitive functions. AI algorithms and applications are restructuring traditional business models by streamlining repetitive tasks, optimizing resource allocation, and enhancing productivity. However, ethical considerations in AI implementation are paramount, necessitating responsible AI governance practices to address concerns regarding bias, privacy, and transparency π€.
Industry-Specific Research
Tech research in the finance sector highlights the adoption of cutting-edge technologies like blockchain, AI-powered analytics, and fintech solutions. Healthcare technological advancements focus on innovations such as telemedicine, electronic health records, and AI-driven diagnostics. Retail sector tech solutions encompass customer analytics, inventory management systems, and personalized marketing strategies tailored to consumer preferences π.
Introduction
In this section of the article, we delve into the vital role of Machine Learning in shaping modern businesses. Machine Learning, a subset of artificial intelligence, has garnered immense significance in recent times due to its transformative potential. It fundamentally alters how organizations operate in the digital era, redefining decision-making processes and customer interactions. Hence, understanding its implications is paramount for professionals, entrepreneurs, and tech enthusiasts alike.
Machine Learning serves as the bedrock for data-driven insights, paving the way for enhanced strategic decision-making and operational efficiency within businesses. By analyzing vast datasets with remarkable speed and precision, Machine Learning algorithms offer unparalleled insights, empowering organizations to make informed choices that drive growth and sustainability. Furthermore, the automation of tasks through Machine Learning streamlines operational workflows, optimizing resource utilization and fostering agility in response to market dynamics.
Moreover, the adaptive nature of Machine Learning applications enables businesses to tailor customer experiences with unparalleled personalization and efficiency. Through predictive analytics, organizations can anticipate consumer preferences, personalize recommendations, and enhance customer satisfaction. Additionally, the integration of natural language processing and image recognition technologies facilitates seamless communication and interactive interfaces, augmenting the overall customer engagement and loyalty.
However, amidst its myriad benefits, Machine Learning also poses significant challenges and considerations for businesses. Data privacy and security emerge as critical concerns, necessitating robust measures to safeguard sensitive information and mitigate potential risks. Furthermore, addressing the skill gap and talent acquisition in Machine Learning remains a pressing issue, requiring investments in employee training and recruiting specialized professionals to harness the full potential of this technology.
Understanding Machine Learning
In this article, Understanding Machine Learning holds pivotal significance as it forms the foundation for comprehending the transformative power of machine learning in business operations. Delving into the intricacies of machine learning concepts and types provides a solid framework for exploring its applications and implications in the corporate landscape. By unraveling the core principles of machine learning, we illuminate how this technology drives innovation and efficiency within organizations, revolutionizing decision-making processes and customer experiences.
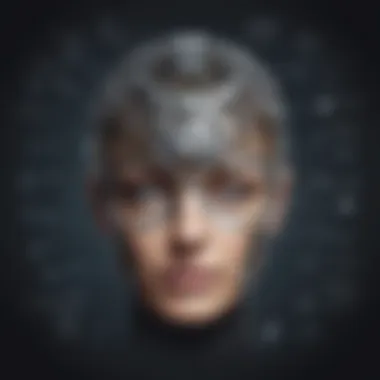
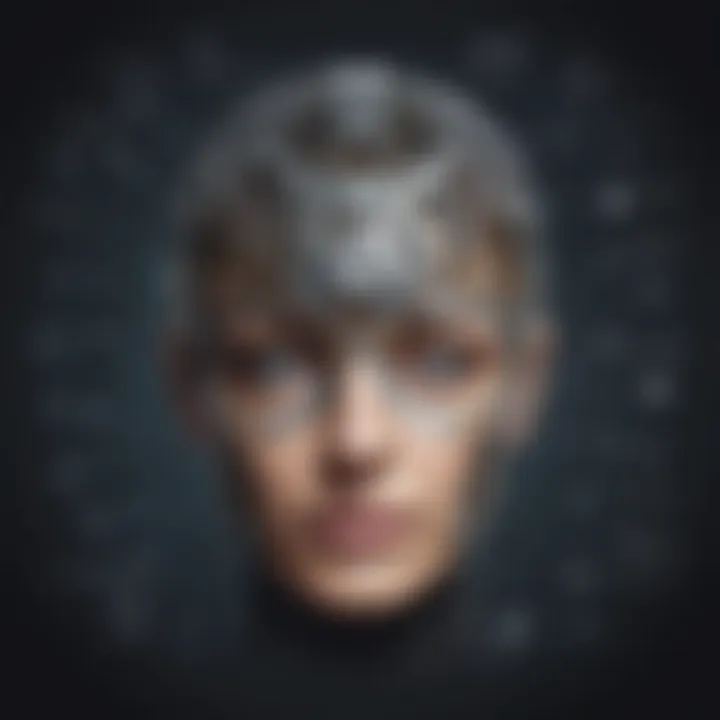
Defining Machine Learning
Machine Learning Concepts
Machine learning concepts are the building blocks of this article, shedding light on how algorithms learn from data to make informed decisions. The key characteristic of machine learning concepts lies in their ability to adapt and improve performance without explicit programming. This characteristic makes them a favorable choice for businesses seeking data-driven insights and predictive analytics. Despite their complexity, machine learning concepts offer unique advantages in enhancing operational efficiency and streamlining processes by leveraging large datasets intelligently.
Types of Machine Learning
The categorization of machine learning into types plays a significant role in shaping its practical applications and outcomes. By understanding the different types of machine learning, such as supervised, unsupervised, and reinforcement learning, businesses can tailor their approaches to suit specific objectives. Each type brings its unique features to the table, from supervised learning's guidance by labeled data to unsupervised learning's ability to identify patterns autonomously. Recognizing the advantages and disadvantages of each type empowers organizations to harness the full potential of machine learning in addressing varying business needs.
Applications of Machine Learning
Predictive Analytics
Predictive analytics epitomizes the marriage between data and foresight, enabling businesses to forecast future trends and behaviors with precision. The key characteristic of predictive analytics lies in its proactive nature, guiding strategic decisions based on actionable insights derived from historical data. By incorporating predictive analytics, organizations can mitigate risks, seize opportunities, and optimize resource allocation effectively. Despite its complexities, predictive analytics offers a competitive edge by empowering businesses to anticipate market shifts and customer preferences accurately.
Natural Language Processing
Natural language processing embodies the convergence of machine learning and linguistics, facilitating seamless communication between humans and machines. The key characteristic of natural language processing lies in its capability to interpret and generate human language fluently. This attribute makes it a valuable asset for businesses aiming to automate customer interactions, improve sentiment analysis, and enhance text summarization processes. Despite the challenges, natural language processing enhances operational efficiency by enabling accurate language translation and sentiment analysis on a large scale.
Image Recognition
Image recognition symbolizes the evolution of machine learning in visual analysis, revolutionizing how businesses interpret and categorize visual data. The key characteristic of image recognition lies in its ability to identify patterns, objects, and faces within images accurately. This feature makes it a valuable choice for applications such as security surveillance, medical diagnostics, and autonomous driving systems. Despite its computational intensity, image recognition offers unparalleled advantages in automation, quality control, and image retrieval tasks, accelerating the pace of innovation and decision-making.
Benefits of Machine Learning in Business
In examining the benefits of integrating machine learning into business operations, it becomes evident that this technology plays a pivotal role in enhancing various facets of organizational efficiency and effectiveness. By harnessing the power of machine learning algorithms, businesses can delve into the realm of data-driven insights, empowering them to make well-informed decisions based on comprehensive analysis rather than intuition or historical trends alone. Moreover, machine learning facilitates risk management by providing predictive analytics that identify potential risks before they escalate, allowing organizations to proactively address challenges and minimize negative impact. The incorporation of machine learning in business not only streamlines processes but also fosters a culture of continuous improvement and innovation, essential for sustainability in today's competitive landscape.
Enhanced Decision Making
Data-driven insights
The utilization of data-driven insights within the context of business decision-making heralds a paradigm shift towards evidence-based strategies rather than conjecture. Data-driven insights leverage historical and real-time data to identify patterns, trends, and correlations that influence operational outcomes, enabling organizations to optimize their processes and capitalize on emerging opportunities. This emphasis on empirical evidence not only enhances the accuracy of decision-making but also instills a sense of confidence and transparency within the decision-making framework.
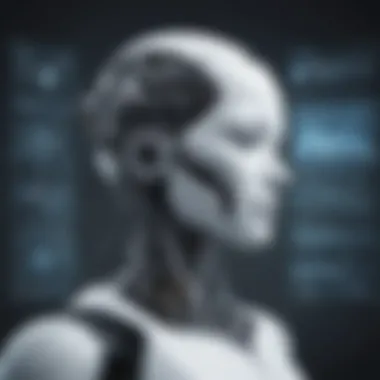
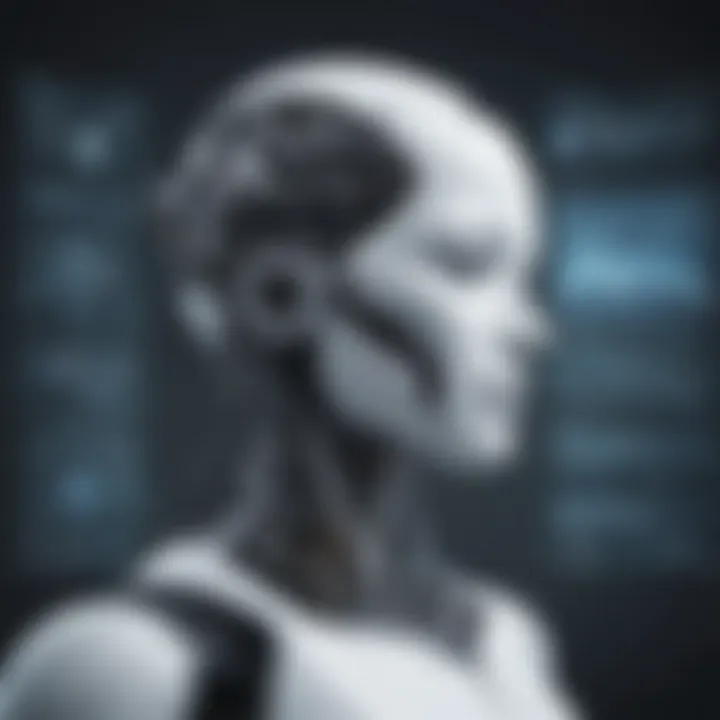
Risk management
Risk management, a critical component of strategic planning, is significantly bolstered by the integration of machine learning capabilities. By employing advanced algorithms to assess risk factors and predict potential threats, businesses can preemptively mitigate risks and fortify their resilience against unforeseen events. The proactive nature of machine learning in risk management equips organizations with the foresight and agility required to navigate complex business landscapes while maintaining operational stability.
Improved Customer Experiences
Personalization
Personalization, a cornerstone of modern customer engagement, is elevated through the implementation of machine learning algorithms that tailor products and services to individual preferences and behaviors. By analyzing customer data and interactions, businesses can craft personalized recommendations, offers, and experiences that resonate with each customer on a personal level, fostering loyalty and brand affinity. The personalized approach facilitated by machine learning not only enhances customer satisfaction but also cultivates long-term relationships built on trust and mutual understanding.
Customer service automation
The automation of customer service processes through machine learning technologies revolutionizes the efficiency and responsiveness of customer interactions. Automated chatbots equipped with natural language processing capabilities can address customer queries and issues round-the-clock, ensuring prompt and personalized support. By relieving human agents of routine inquiries, customer service automation frees up valuable resources, allowing organizations to allocate human capital towards more complex tasks that require emotional intelligence and critical thinking.
Operational Efficiency
Automation of repetitive tasks
Streamlining operational workflows and reducing manual intervention is a core benefit of leveraging machine learning for automating repetitive tasks. By automating routine processes such as data entry, scheduling, and reporting, organizations can enhance efficiency, accuracy, and scalability across multiple functions. The automation of repetitive tasks not only minimizes human error but also accelerates time-to-market and enables employees to focus on value-adding activities that drive strategic objectives.
Supply chain optimization
Supply chain optimization, a strategic imperative in ensuring seamless operations and cost-effectiveness, is intricately enhanced through machine learning interventions. By analyzing vast amounts of supply chain data in real time, machine learning algorithms can optimize inventory management, demand forecasting, and logistics routing to mitigate disruptions and enhance overall productivity. The predictive capabilities of machine learning enable businesses to anticipate supply chain bottlenecks and proactively adjust their strategies, fostering resilience and agility in a dynamic marketplace.
Challenges and Considerations
In addressing the challenges and considerations surrounding the integration of machine learning in business operations, it is crucial to delve into various key elements that play a significant role in shaping the landscape of this technological advancement. One paramount aspect that demands attention is data privacy and security, which forms the cornerstone for maintaining trust and confidentiality in a data-driven business environment. Without a robust framework in place to safeguard sensitive information, businesses risk not only violating regulations but also jeopardizing their reputation and customer trust.
Data Privacy and Security
Ethical implications:
Ethical implications within the realm of data privacy and security underscore the moral responsibility of organizations to handle data ethically and transparently. This facet addresses issues such as consent, data usage, and the potential societal impact of leveraging machine learning technologies. Ethical considerations are imperative in ensuring that data practices align with ethical standards, respect user privacy, and mitigate any discriminatory outcomes.
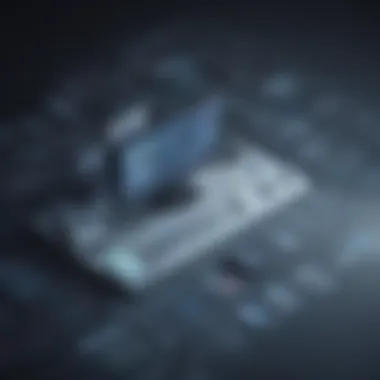
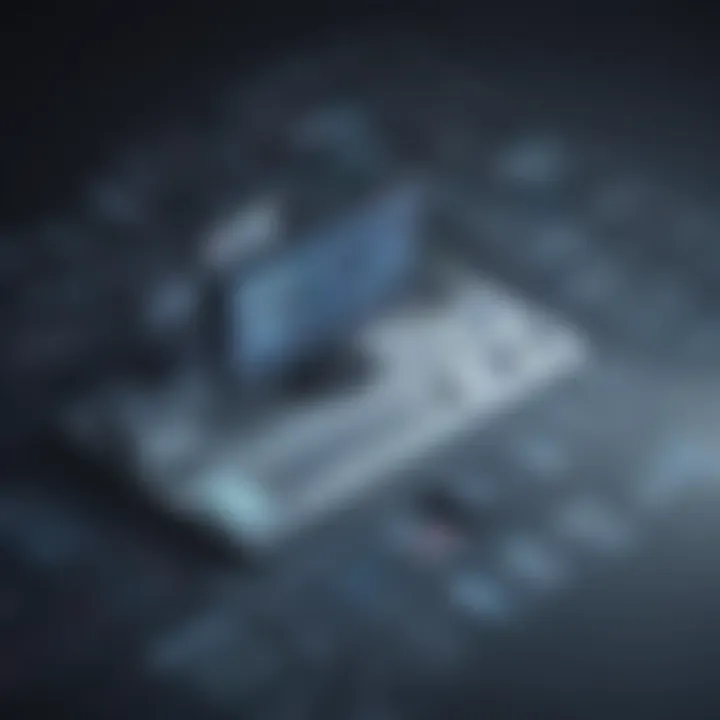
Cybersecurity concerns:
Cybersecurity concerns represent a critical component of data protection when implementing machine learning technologies. With the increasing sophistication of cyber threats, businesses face the challenge of safeguarding their systems from malicious attacks and data breaches. Addressing cybersecurity risks involves implementing robust security protocols, encrypting sensitive data, and continuously monitoring for any vulnerabilities to prevent unauthorized access and data compromises.
Skill Gap and Talent Acquisition
Shifting focus to the skill gap and talent acquisition, businesses encounter the imperative of training employees to harness the full potential of machine learning tools effectively. Ensuring that staff possesses the necessary skills to leverage data analytics and machine learning algorithms is essential for maximizing the benefits derived from these technologies. Strategic training programs can empower employees to understand the complexities of machine learning and apply them adeptly within their respective roles.
Training employees:
Training employees encompasses education initiatives aimed at enhancing employees' understanding of machine learning concepts, data analysis techniques, and algorithmic principles. By equipping staff with the requisite knowledge and skills, organizations can cultivate a data-literate workforce capable of leveraging data-driven insights to drive strategic decision-making and operational efficiencies.
Recruiting data scientists:
In the realm of talent acquisition, recruiting data scientists stands out as a pivotal strategy for organizations looking to augment their analytical capabilities and derive actionable insights from big data sets. Data scientists play a crucial role in developing and implementing machine learning models, conducting in-depth data analysis, and extracting valuable business intelligence. However, the recruitment of data scientists presents challenges in identifying qualified professionals with a blend of technical expertise, domain knowledge, and problem-solving acumen necessary to thrive in a data-centric environment.
Future Trends in Machine Learning
In the continually evolving realm of technology, staying abreast of future trends in machine learning is imperative for businesses aiming to remain competitive. The adoption of AI technologies is reshaping traditional business practices, with machine learning spearheading this paradigm shift. Understanding the nuances of upcoming trends in machine learning equips organizations to leverage cutting-edge advancements effectively. By delving into trends such as Explainable AI and AI-Powered Automation, companies can harness the potential of these innovations to drive growth and efficiency.
Explainable AI
Interpretable machine learning models
Interpretable machine learning models play a pivotal role in enhancing the transparency and explainability of AI-driven decision-making processes. These models prioritize clarity, enabling stakeholders to comprehend the decision-making rationale behind AI algorithms. The key characteristic of interpretable machine learning models lies in their ability to provide actionable insights in a comprehensible manner, promoting trust and accountability within organizations. This feature makes interpretable models a favored choice in critical domains where transparency and interpretability are paramount. Despite their benefits, interpretable machine learning models may have limitations, such as potentially sacrificing nuanced complexity for clarity. Nevertheless, their advantages in fostering trust and understanding make them invaluable tools in augmenting decision-making processes.
AI-Powered Automation
Robotic process automation
Robotic process automation (RPA) revolutionizes operational workflows by automating repetitive tasks, streamlining processes, and reducing human error. The key characteristic of RPA is its capacity to mimic human actions in software applications, enhancing efficiency and scalability. This automation approach is immensely beneficial for organizations seeking to optimize resource utilization and drive operational productivity. The unique feature of RPA lies in its adaptability across diverse functions, from finance to customer service, making it a versatile solution for various business verticals. While RPA offers significant advantages in enhancing operational agility, it may pose challenges in terms of initial setup complexities and integration with existing systems.
Intelligent chatbots
Intelligent chatbots represent a sophisticated application of AI technology in automating conversational interactions with users. Key characteristics of intelligent chatbots include natural language processing capabilities and contextual understanding, facilitating seamless engagement with customers. The appeal of intelligent chatbots in modern business scenarios stems from their role in augmenting customer service operations, improving response times, and personalizing user experiences. The unique feature of intelligent chatbots lies in their ability to learn from interactions, continuously enhancing their conversational abilities. Despite their advantages, intelligent chatbots may encounter limitations in handling complex queries or emotional nuances effectively. Overall, their benefits in enhancing customer engagement and operational efficiency make them indispensable tools for businesses embracing AI-driven automation.
Conclusion
Machine learning has undeniably become a cornerstone in shaping modern business landscapes. In this article, we navigated through the intricate web of advancements brought about by machine learning within the business domain. Its pivotal role in enhancing decision-making processes cannot be overlooked. By leveraging data-driven insights, companies can now make strategic decisions backed by robust analytics, leading to increased operational efficiency and competitive advantage. Moreover, the impact of machine learning on customer experiences has been profound. Through personalization and automation, businesses can tailor their offerings to meet individual needs, leading to heightened customer satisfaction and loyalty. However, amidst all these benefits, it is pivotal to consider the ethical implications and cybersecurity concerns that accompany the widespread adoption of machine learning. Safeguarding data privacy and security must remain a top priority for businesses venturing into the realm of AI technologies. Addressing the skill gap and talent acquisition challenges by investment in training programs and recruitment strategies is vital to fully capitalize on the potential of machine learning. As we look to the future, concepts like Explainable AI and AI-powered automation stand out as potential game-changers, paving the way for even more innovation and transformation in the business landscape. In essence, the journey of machine learning in business is just beginning, promising a future filled with endless possibilities and opportunities for those daring enough to embrace its potential.