Industry Forecast: Insights for Future Success
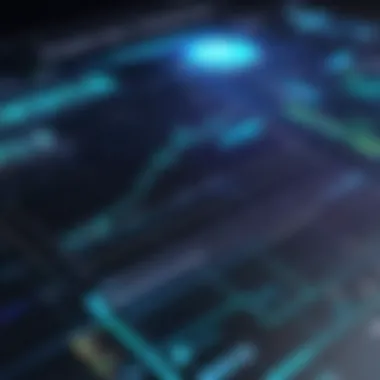
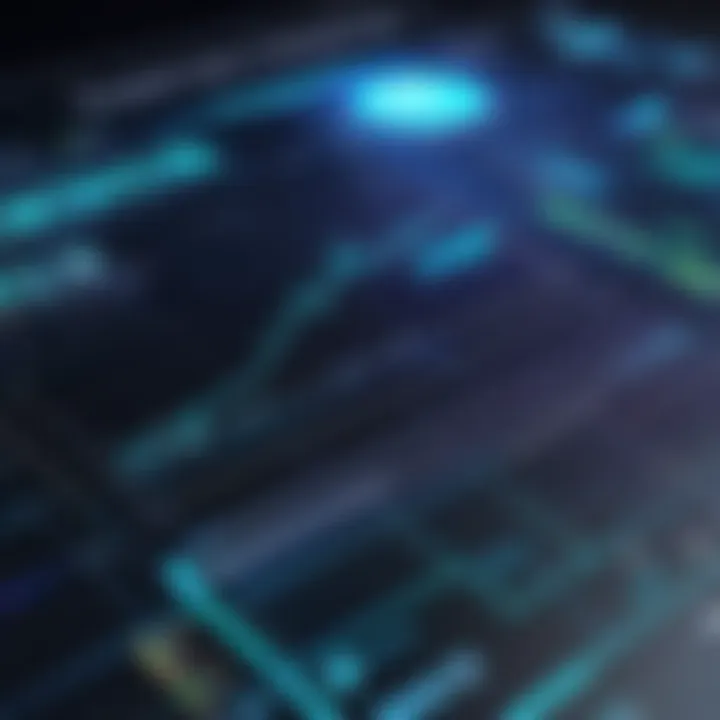
Intro
In a world that keeps spinning faster each day, understanding the shifts and currents of industry landscapes has become crucial. The technological realm is especially dynamic, shaping every facet of business interactions, consumer behavior, and strategic decision-making. This article aims to equip professionals and entrepreneurs with the necessary insights to navigate through changes while harnessing the power of accurate forecasting. As we dive into the various elements that comprise effective industry forecasting, the focus will be on how technological innovations influence business processes and future strategies.
Technological Research Overview
Recent Technological Innovations
The past few years have shown profound shifts within numerous technological sectors, with innovations emerging from unexpected corners. The rapid advancement of augmented reality, for example, is no longer confined to gaming. It's now a tool in training for medical professionals, enabling them to practice intricate procedures without risks associated with real patients.
Moreover, advancements in quantum computing are reshaping data processing capabilities at an unprecedented scale. Companies like IBM and Google are investing heavily in this area, which could revolutionize industries reliant on heavy computations, such as pharmaceuticals and aerospace.
Impact on Business Operations
What’s clear is that these innovations don’t exist in a vacuum; they ripple through entire organizations. Take for instance the mass shift to cloud computing which has transformed both operational efficiency and cost structure for businesses. Companies can scale services up or down swiftly, responding more effectively to market demands.
Furthermore, embracing technologies like Internet of Things (IoT) fosters real-time monitoring and data collection, enabling organizations to enhance productivity and innovate continuously. Businesses stick to their core strategies while remaining agile enough to pivot as necessary based on data-driven insights.
Future Technological Trends
Looking forward, we can expect certain trends to dominate the landscape. Automation will deepen its roots across industries, alleviating tedious tasks and allowing talent to focus on strategic initiatives.
The trend beyond automation hints at a growing reliance on artificial intelligence and machine learning to personalize experiences and forecast needs even further. As businesses increasingly harness these capabilities, the next big question will be whether they can keep ethical considerations front and center.
Data Analytics in Business
Importance of Data Analytics
In today's business environment, data is often referred to as the new oil, but it requires refinement to truly add value. The ability to analyze data effectively allows organizations to make informed decisions rather than relying on gut feelings or outdated information. In particular, industries such as finance and retail are already reaping the benefits of smart data utilization.
Tools for Data Analysis
A variety of tools have emerged to aid businesses in this endeavor. Software like Tableau and Power BI empowers teams to visualize trends and derive actionable insights from complex datasets. These tools are vital, allowing for interactivity and real-time data analysis – elements necessary for keeping pace with industry changes.
Case Studies on Data-Driven Decisions
An example to consider is Target’s strategy for predicting consumer purchasing patterns. By using predictive modeling, they effectively identify what products customers will want in advance. Through precise targeting, they enhance customer experiences and increase sales volume, highlighting the power and potential of data analytics.
Data is a precious asset. Harnessing it effectively often distinguishes the leaders from the followers in any industry.
Cybersecurity Insights
Threat Landscape Analysis
As we venture deeper into the digital age, cybersecurity threats continue to evolve, becoming more sophisticated. Ransomware attacks, for instance, have surged, prompting companies to rethink their security frameworks. Cybercriminals are not only targeting large corporations; small businesses are often in their sights, making robust cybersecurity crucial for all.
Best Practices for Cybersecurity
To safeguard against these threats, businesses must adopt several best practices:
- Regularly update software and systems
- Conduct comprehensive vulnerability assessments
- Implement multi-factor authentication
- Train employees on cybersecurity awareness
Regulatory Compliance in Cybersecurity
With regulations like GDPR and CCPA becoming stricter, compliance isn’t just a tick-box exercise anymore. Businesses must weave compliance into their security fabric, recognizing it as both a legal necessity and a trust-building measure with customers. Organizations need to be transparent about data usage and prioritize customer data protection.
Artificial Intelligence Applications
AI in Business Automation
Artificial intelligence has marked its territory as a key player in business automation. From chatbots providing customer service to algorithms optimizing supply chains, the potential is vast. This technology not only improves efficiency but also drives down costs, leading organizations to rethink traditional business models.
AI Algorithms and Applications
Tech giants like Google and Amazon utilize AI extensively, not just in their core products but throughout their operational processes. Machine learning creates fine-tuned recommendations for users, which in turn drives sales and reinforces customer loyalty.
Ethical Considerations in AI
However, with great power comes great responsibility. Ethical concerns regarding bias in AI algorithms and privacy implications are at the forefront of discourse in this space. Companies must navigate these waters carefully, ensuring that while they leverage AI, they are aware of the potential societal impacts.
Industry-Specific Research
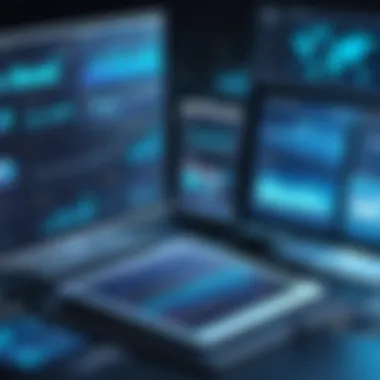
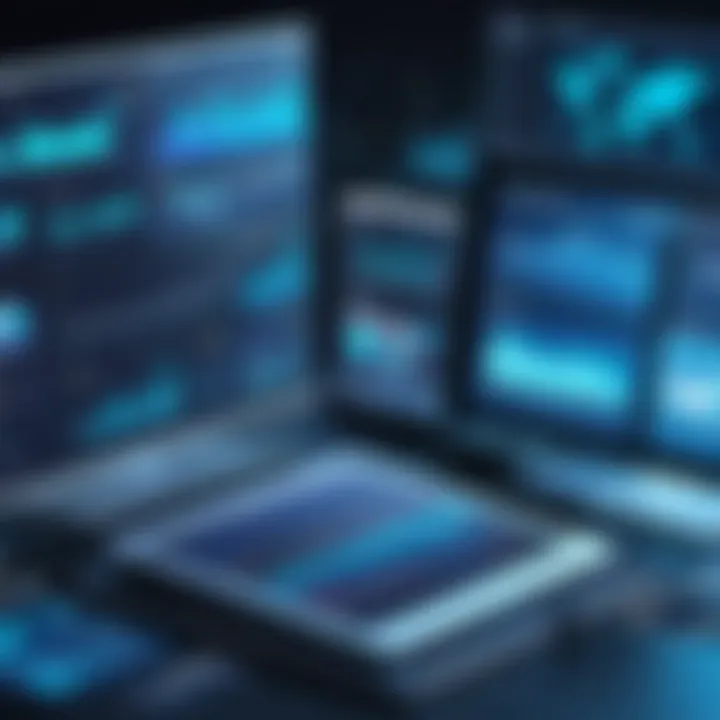
Tech Research in Finance Sector
In finance, firms are investing heavily in fintech innovations. The rise of cryptocurrencies and Blockchain technology is changing how transactions occur, ushering in a new era of transparency and security.
Healthcare Technological Advancements
In healthcare, technological progress is enabling more accurate diagnostics and treatment plans. Wearable devices and telehealth platforms are altering patient interactions and improving overall patient care.
Retail Industry Tech Solutions
Finally, in retail, the integration of technology is transforming shopping experiences, from AI-driven personalized marketing to innovative online interfaces ready for a seamless customer journey. Organizations that embrace these changes will undoubtedly gain an edge in a competitive market.
Understanding Industry Forecasting
Industry forecasting plays a crucial role in shaping strategies and decision-making in various sectors, especially in our tech-driven world. As companies scramble to adapt to the fast-paced changes in technology, a firm grasp of industry forecasting is not just beneficial; it is imperative. The ability to predict market trends, customer behavior, and technological advancements empowers businesses to stay one step ahead.
Definition and Importance
To define industry forecasting, one must consider it as a methodical approach to predicting future economic conditions, market changes, and technology trends based on a blend of qualitative and quantitative analysis. This practice combines various data sources, statistical methods, and analytical tools to produce insights that can significantly influence business strategy and resource allocation.
The importance of industry forecasting cannot be overstated. For businesses, accurate forecasts can minimize risks and reveal opportunities in the marketplace, ensuring a proactive rather than reactive stance. By analyzing data from historical trends, consumer patterns, and technological innovations, organizations can better prepare for inevitable changes.
Here’s a snapshot of why industry forecasting holds such significance:
- Risk Management: Identifying potential challenges early can save companies from costly missteps.
- Competitive Advantage: Understanding market dynamics helps businesses position themselves ahead of the competition.
- Resource Optimization: Allocating resources efficiently is easier with accurate forecasts, reducing wastage.
Industry forecasting also encompasses sector-specific considerations. For example, in the tech industry, the fast evolution of software and hardware paradigms means that stakeholders must constantly reassess their strategies. Market forecasts help gauge adoption rates, shifts in customer needs, or advancements in tech that could influence product development.
Historical Context
To truly appreciate the evolution of industry forecasting, one must take a trip down memory lane. The roots of forecasting trace back centuries to agriculture, where farmers relied on seasonal patterns to plan planting and harvest dates. The flipside of this was economic forecasting, born from the need to predict market reactions and performance under varying conditions.
This historical progression laid the groundwork for today’s sophisticated methods. In the mid-20th century, with the advent of computers and statistical software, forecasting saw a dramatic transformation. Businesses could analyze vast amounts of data more quickly, leading to more accurate predictions than ever before. The rise of the internet further enhanced this evolution—data became more available, and tools more advanced.
Predictive analytics now harness massive datasets from diverse sources, ranging from social media trends to economic indicators. For instance, businesses in the retail sector analyze consumer purchasing behaviors online to forecast future sales accurately. Meanwhile, tech companies utilize machine learning algorithms that continuously update forecasts based on real-time data.
"In the world of business, the only constant is change; forecasting allows us to shape strategy amid uncertainty."
Thus, understanding the history and evolution of industry forecasting not only highlights its importance but also illustrates how far we've come from simple predictions to sophisticated analyses that support strategic decision-making in today's fast-paced arenas.
The Fundamentals of Predictive Analytics
Predictive analytics has become an integral cornerstone in the art of industry forecasting. It allows entities to sift through heaps of data, drawing actionable insights that can assist in anticipating future events with a commendable level of certainty. The rapid pacing of today’s markets, especially in technological sectors, demands not just surface-level analysis but a robust understanding built through fundamental approaches. In this respect, several key elements come into play when discussing predictive analytics within an industry context.
Data Collection Techniques
When it comes to predictive analytics, data is the lifeblood, and how we gather this information can significantly impact the quality of our forecasts. Two distinct methodologies reign when it comes to data collection—quantitative and qualitative approaches.
- Quantitative Data: This involves numerical data that can be statistically analyzed. Think of this as collecting information from surveys or customer transactions, where numbers tell a significant story of trends and preferences. These metrics often provide a solid backbone for predictive models.
- Qualitative Data: This type relates to non-numerical insights gathered through interviews, focus groups, or social media evaluations. While this data may seem less tangible, it adds rich contextuality to the numbers.
Incorporating both methodologies may yield the finest results. However, simply gathering data isn't enough; the methods must be robustly defined, ensuring accuracy and relevance. Poor data collection efforts can lead to erroneous conclusions, and as they say,
Key Trends Impacting Industry Forecasts
In today's fast-paced world, trends that impact industry forecasts are not merely footnotes but the main storyline that shapes the future of sectors, especially in technology. Recognizing these trends can enable businesses to adapt and thrive in an environment where change is the only constant. With a deep understanding of what's coming down the pipeline, decision-makers can better position themselves for success. As we dive deeper into the specific trends, we will uncover how they fuel innovation, regulatory compliance, and strategic planning.
Technological Advancements
As the adage goes, "Innovation is the mother of necessity." This may never ring truer than in sectors highly influenced by technology. From artificial intelligence to blockchain, advancements in technology ripple through industries, altering the way organizations forecast and strategize.
Consider the rise of cloud computing. Businesses can now access and interpret vast datasets in real-time, breaking down data silos that may have existed previously. This democratization of access to information encourages agility and informed decision-making, which is particularly crucial for companies aiming to keep pace with competitors.
Moreover, with the advent of predictive analytics, organizations can generate insights that were previously locked away in expansive datasets. For example, a retail company leveraging AI and machine learning can anticipate consumer behavior based on trends and past purchases, allowing for tailored marketing strategies.
Regulatory Changes
Regulatory changes can act as double-edged swords: they may foster innovation but also restrict certain avenues for growth. Take the General Data Protection Regulation (GDPR) as a salient case. Established to protect consumer privacy, this regulation has forced tech companies to rethink data handling and storage. While initially seen as a hurdle, it has also paved the way for advancements in data security and ethical marketing practices.
Organizations must stay abreast of these regulations and adapt their forecasting methods accordingly. An especially forward-thinking enterprise might even engage in proactive compliance, turning regulatory constraints into a competitive advantage by reinforcing trust with consumers through transparent practices.
Market Dynamics
Market dynamics encapsulate everything from shifting consumer preferences to economic instability. For example, the recent global pandemic has reshaped consumer behavior—momentarily scrapping face-to-face transactions in favor of e-commerce. Companies that clung to traditional sales forecasting methods found themselves scrambling as the ground beneath their feet shifted.
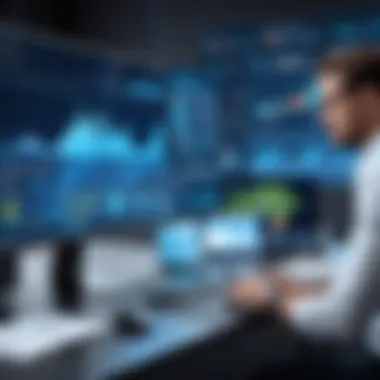
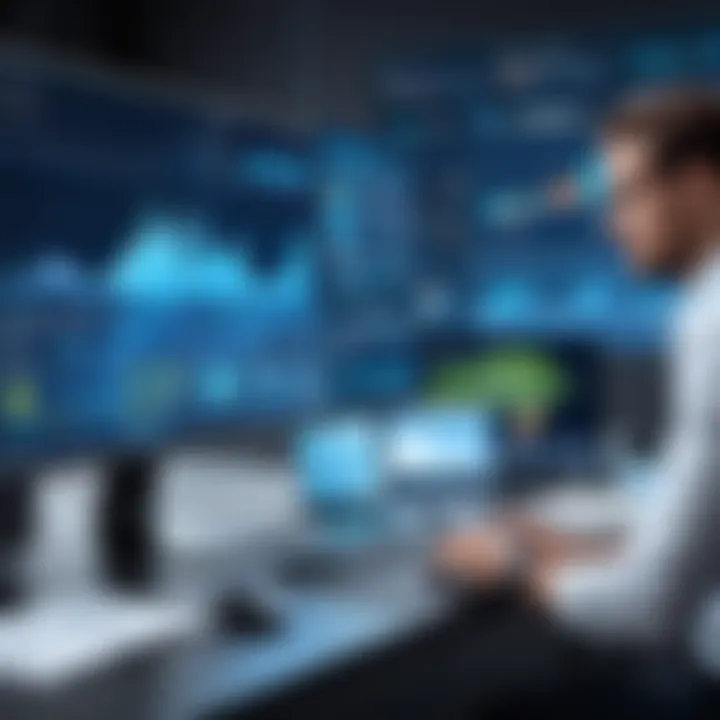
To navigate these changing tides, businesses are now leaning heavily on scenario planning, allowing them to visualize various futures based on current data trends. This practice not only prepares organizations for sudden changes but also fosters a culture of adaptability. Another consideration is supply chain logistics; external disruptions can significantly influence inventory management and logistics strategies, making it essential that businesses evaluate these dynamics as part of their forecasting model.
"In a world where the only certainties are uncertainty and change, forecasting becomes an act of resilience and foresight."
Sector-Specific Considerations
Understanding various sectors is crucial when discussing industry forecasts. Each sector brings its own unique challenges and opportunities, which can significantly influence predictive outcomes. It’s not just about the numbers; it’s about grasping the subtleties that differentiate these industries.
Technology and Digital Transformation
The tech sector is an ever-evolving landscape, where change is the only constant. Digital transformation isn’t just a buzzword; it’s a necessity. Companies that lag behind in adopting new tools often find themselves quickly outpaced. For instance, businesses embracing cloud computing and artificial intelligence are finding new efficiencies and innovative products, while their competitors may still rely on outdated systems and manual processes.
It is vital to understand how digital transformation impacts forecasting. The integration of tools such as Internet of Things (IoT) devices and big data analytics allows firms not only to collect vast amounts of data but also to analyze patterns and anticipate market shifts. In this digital age, the importance of agility cannot be overstated. Companies must keep their ears to the ground to adjust strategy based on emerging political contexts, consumer behaviors, or technological breakthroughs.
Healthcare Innovations
In the realm of healthcare, forecasting presents unique challenges and opportunities. With advancements in telemedicine and personalized medicine, understanding consumer needs and regulatory frameworks has become of utmost importance. The COVID-19 pandemic underscored the essential role of forecasting in determining resource allocation and response strategies.
Healthcare innovations are changing the way professionals approach forecasting. For example, predictive analytics is used to anticipate patient admission rates based on historical data, seasonal trends, and even weather patterns. Hospitals can now better prepare for patient inflows, which can mean the difference between life and death in critical situations. Thus, sector-specific considerations in healthcare reveal the necessity for a tailored approach to forecasting, engaging with variables unique to this field.
Manufacturing Trends
Manufacturing has seen a seismic shift thanks to technological advancements such as automation and smart factories. It’s one area where forecasting isn't just useful—it's critical. Firms must anticipate shifts in supply chain dynamics, labor costs, and material availability, all of which directly impact production timelines.
As companies move toward lean manufacturing principles, the intricacies of these trends make it paramount to level up predictive capabilities. Utilizing advanced algorithms to assess supply chain risks enables firms to mitigate disruptions proactively. Attention to how global events—like trade policies or natural disasters—can affect local industries further enriches the forecasting narrative in manufacturing.
Financial Services Evolution
The financial sector is undergoing a revolution. Innovations like blockchain technology and fintech applications are reshaping how financial services operate, as well as how they’re forecasted. Decision-makers need to stay ahead of these changes to mitigate risks and explore new markets. For instance, real-time analytics has started to redefine risk assessment in investments, where traditional methods fall short.
Consumer behavior in finance is also rapidly evolving. As more people turn to online banking and investment apps, understanding these shifts provides critical insights into future trends. Financial forecasting, therefore, cannot be a one-size-fits-all approach; it requires an acute awareness of changing consumer preferences, regulatory environments, and technological shifts.
The major takeaway is that industry-specific forecasting is not merely about applying generic models; it’s about adapting to and understanding the nuances that define each sector’s landscape.
Clearly, sector-specific considerations enhance the precision of forecasts, laying the groundwork for strategic decision-making. By focusing on the intrinsic factors unique to each industry, businesses can position themselves better for both anticipated and unforeseeable challenges.
Challenges in Industry Forecasting
In the realm of industry forecasting, the challenges faced can often resemble navigating a maze with shifting walls. As businesses strive to anticipate market movements and enhance strategic decisions, it's crucial to understand the hurdles that may arise. This section zeroes in on three core challenges: data integrity issues, volatility and uncertainty, and bias in models. Each of these elements not only impacts the accuracy of forecasts but also shapes the operational decisions that can make or break an entity's success.
Data Integrity Issues
Data integrity stands as a cornerstone in the world of forecasting. It refers to the accuracy and consistency of data over its entire lifecycle. When businesses use faulty or incomplete data as a base for their predictions, they might as well be casting darts in the dark. For example, consider a company investing heavily in a new product line based on faulty sales data from an unreliable source. If the data reflects inflated projections, that company might find itself in hot water, faced with overstock and reduced profits.
To mitigate these issues, organizations must prioritize rigorous data validation processes. Implementing data cleaning methods—such as reconciling records and eliminating duplicates—can enhance the integrity of their datasets. Furthermore, establishing a culture of accountability around data management ensures that the sources remain reliable and trustworthy, enabling forecasts to thrive on solid ground.
Volatility and Uncertainty
In today’s fast-paced digital landscape, volatility and uncertainty have become ubiquitous. Markets can shift on a dime, thanks to myriad factors including economic changes, technological advancements, or even global health crises. This fluctuating environment complicates forecasting models immensely. For instance, a sudden spike in raw material costs due to geopolitical tensions can upend production timelines and reorder priorities.
Dealing with such unpredictable elements often requires a multi-faceted approach. Incorporating scenario planning could help organizations navigate through potential outcomes. This strategic method involves creating various plausible futures and assessing impacts to ensure that when the next storm brews, businesses are not left scrambling. Embracing flexibility in strategic plans equips organizations to adjust their forecasts with the winds of change.
Bias in Models
All models are simplifications of reality, and this is where bias creeps in. Bias can arise from many sources, such as the data used, the methods of analysis chosen, or even the inherent perspectives of the analysts themselves. For instance, a forecasting model built predominantly on past successes may overlook emerging trends that deviate from established paths. This could result in a skewed understanding of market trajectories and potentially lead to lost opportunities.
To combat bias, transparency in model development is essential. Analysts should regularly review their assumptions and methods, employing diverse teams to bring varying viewpoints into the mix. Moreover, introducing alternate forecasting methods helps neutralize reliance on a single paradigm, enhancing overall accuracy.
"Forecasters must embrace the complexity of their craft, continually evaluating and refining their tools to better meet the demands of a rapidly evolving landscape."
By acknowledging the challenges posed by data integrity, market volatility, and bias, organizations can better prepare themselves to forecast with precision and agility, ensuring they remain one step ahead in their respective industries.
The Role of Machine Learning
Machine learning (ML) is an essential component in the realm of industry forecasting, especially as we live in a time when data is produced exponentially. Its significance stems from its ability to analyze massive datasets, uncover patterns, and derive insights that traditional methods might overlook. In the context of forecasting, ML not only enhances the accuracy of predictions but also enables businesses to preemptively adjust their strategies based on anticipated market changes. Here, we’ll explore how machine learning integrates with traditional forecasting methods, its numerous benefits, and the limitations it faces in application.
Integration with Traditional Methods
Integrating machine learning with traditional forecasting techniques is like blending oil and vinegar; at first glance, they seem mismatched, but with the right approach, they can create something exceptional. Traditional methods often rely on historical data and straightforward statistical approaches, which can sometimes miss the nuances in complex datasets.
On the other hand, machine learning thrives on complexity and can incorporate vast amounts of data that include unstructured forms like text, images, and even social media feeds. When these two approaches are fused, it can lead to more reliable forecasts. For instance, a company might utilize linear regression for its basic analysis but then apply a machine learning model like random forests to refine the results, capturing more intricate relationships that may influence the market.
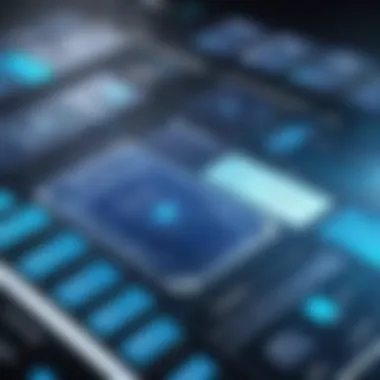
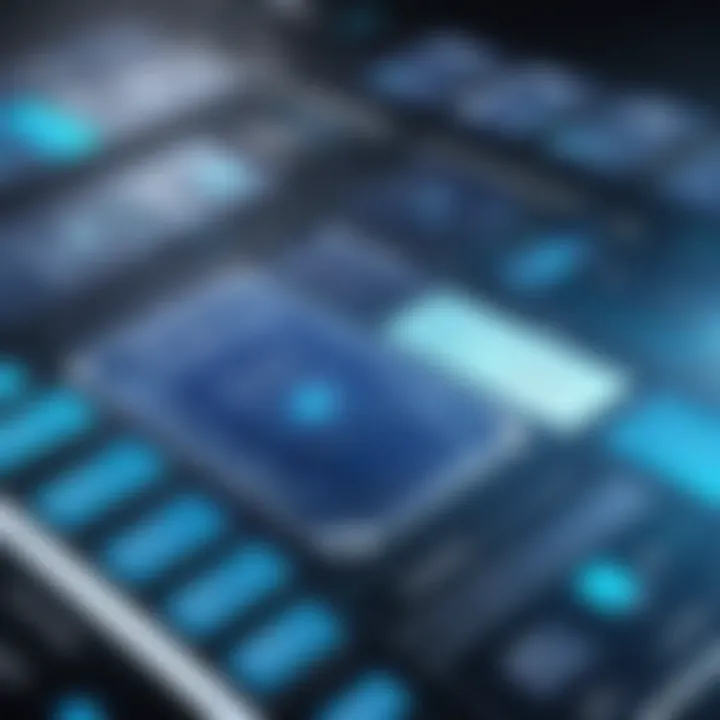
- Practical example: In the retail sector, predictive models using both time series analysis and neural networks could forecast demand more accurately, thus aiding inventory management strategies.
This synergy allows forecasters to develop a more holistic view of potential future states, making the iterative process of refining forecasts more effective.
Benefits of Machine Learning
The benefits of machine learning in industry forecasting are as numerous as they are impactful. Here are some of the key advantages:
- Enhanced Prediction Accuracy: By analyzing numerous variables and correlations, ML models can improve prediction accuracy significantly compared to traditional methods.
- Real-time Insights: Machine learning algorithms can process and analyze data in near real-time, allowing companies to adjust their strategies dynamically. This responsiveness is crucial in rapidly changing environments.
- Scalability: ML models can scale up to accommodate larger datasets without a loss in performance. This is particularly beneficial in sectors like financial services, where data grows continuously.
- Automation of Analysis: By automating the analysis process, businesses can free up resources that would otherwise be spent on manual data handling.
- Pattern Recognition: Machine learning excels at recognizing patterns that might not be obvious to human analysts, thus revealing hidden opportunities or risks.
"In the landscape of industry forecasting, machine learning isn't merely a tool; it's a transformative power that drives innovation and decision-making."
These benefits are particularly relevant for organizations that need to make data-driven decisions quickly and accurately.
Limitations of AI in Forecasting
Despite its clear advantages, relying solely on machine learning for forecasting does come with its own set of limitations. It’s essential to approach this technology with a discerning eye. Some pertinent limitations include:
- Data Quality Dependency: The effectiveness of ML models heavily relies on data quality. Poor or biased data can lead to inaccurate predictions.
- Interpretability Issues: Many machine learning models, especially deep learning algorithms, often operate as a ‘black box,’ making their predictions hard to interpret, which can hinder decision-makers' trust in the output.
- Overfitting Risk: Machine learning models can sometimes overfit to historical data, meaning they might perform well on training data but poorly on new, unseen data.
- Cost and Resources: Implementing machine learning solutions often requires significant investment in technology and expertise, which can be a barrier for smaller businesses.
Recognizing these limitations is crucial for businesses that wish to incorporate machine learning into their forecasting processes. By understanding both the strengths and weaknesses, organizations can make more informed decisions on how to proceed.
In summary, the role of machine learning in industry forecasting is multifaceted, blending seamlessly with traditional methods to enhance accuracy and insights. While it offers substantial benefits, being aware of its limitations is equally important. Moving forward, organizations must find a balanced approach that leverages machine learning while navigating its inherent pitfalls.
Best Practices for Effective Forecasting
Effective forecasting is not merely a routine task—it’s a pivotal component for any business aiming to thrive amid uncertainty. Best practices in forecasting ensure that organizations are equipped with the tools and knowledge to anticipate market shifts, manage risks, and seize opportunities before they vanish into thin air. Understanding how to harness these practices helps professionals make strategic decisions that could very well define the trajectory of their enterprises.
Continuous Learning and Adaptation
In today's fast-paced business climate, companies must constantly recalibrate their strategies through continuous learning. This means developing methodologies that not only allow for collecting vast amounts of data but also include the ability to interpret and act upon it.
To effectively implement this practice, consider the following steps:
- Stay Informed: Keenly watch industry trends and how they evolve over time. Subscribe to journals, attend workshops, and participate in forums.
- Feedback Loops: Regularly solicit feedback from key stakeholders, customers, and employees. Use this input to refine forecasting models.
- Training Programs: Invest in training for staff on new analytical tools and data interpretation methods. An empowered team is an innovative team.
"Adaptive learning isn’t a one-and-done. It’s an ongoing journey that keeps businesses one step ahead of the competition."
Collaboration Across Teams
Collaboration is the backbone of effective forecasting. It’s like a well-conducted symphony—every section plays its part. When teams from different departments band together, they create a richer and more nuanced perspective on data.
Key elements to encourage collaboration include:
- Cross-Disciplinary Meetings: Regular sessions that bring different teams together to exchange insights ensure that everyone is on the same wavelength.
- Shared Platforms: Utilize collaborative software that allows real-time data sharing and analysis. Tools like Slack or Trello can bridge gaps between departments and foster unified efforts.
- Joint Responsibility: By making different teams accountable for various parts of the forecasting process, you inject a sense of ownership and commitment into the whole operation.
Documentation and Review Processes
Documenting processes and maintaining robust review channels is essential to creating accurate forecasts. If data isn’t carefully recorded and considered, it can lead to assumptions that miss the mark—throwing all previous calculations into disarray.
Consider incorporating these best practices:
- Standard Operating Procedures (SOPs): Outline and follow consistent steps for collecting and analyzing data. This creates a clear roadmap for all involved.
- Monthly Reviews: Set up regular reviews of past forecasts versus actual results. Analyzing missed predictions can lead to insightful lessons and help fine-tune future efforts.
- Centralized Documentation: Maintain a centralized database that everyone in the organization can access. This ensures that all team members are using the same information and bases their decisions on verified data.
By adopting these best practices, organizations can navigate the tricky waters of forecasting. It’s not just about having data at hand but also about understanding it, implementing changes, and continuously seeking to improve—like tending to a garden that flourishes under the right conditions.
End and Future Directions
The conclusion and future directions of industry forecasting serve as a pivotal segment in this article, highlighting the significance of synthesizing knowledge and looking ahead in an inherently volatile landscape. This section invites professionals, entrepreneurs, and decision-makers to take stock of insights gleaned while setting the stage for ongoing exploration and adaptation. Each of the previously discussed components plays a crucial part in developing a robust forecasting model that not only reacts but anticipates market trends.
Recap of Insights
In summarizing the insights shared throughout this article, it becomes clear that understanding the foundations of industry forecasting is essential for navigating complexities. Key elements include:
- The integration of predictive analytics and data collection techniques.
- The influence of technological advancements on various sectors, from healthcare to manufacturing.
- Challenges, such as data integrity and model bias, that industry forecasters must confront.
- The role of machine learning, which adds layers of sophistication to traditional forecasting methods.
Each of these insights provides a framework that firms can apply. They also reiterate the manifold benefits derived from a proactive forecasting approach.
Emerging Areas for Research
As industries evolve, so too should the methods used to forecast them. The following areas represent fertile ground for future research:
- Interdisciplinary Approaches: Combining insights from economics, behavioral sciences, and data analytics can yield unique perspectives on market trends.
- Real-time Data Utilization: The demand for instant insights is growing. Exploring how to leverage real-time data more effectively will be crucial.
- Sustainability Trends: Understanding how the shift towards sustainability impacts various sectors invites rich analysis and will be a focal point in coming years.
- Consumer Behavior Analytics: With consumer preferences shifting rapidly, going deeper into behavioral analytics may provide an edge.
As we put a curtain down on the current insights, the landscape of industry forecasting is characterized by flux and potential. To thrive, organizations must remain vigilant, embrace learning, and drive innovation, catering to the ever-present winds of change in the digital arena.
"The future belongs to those who prepare for it today."
Continually revisiting and refreshing one's approach to forecasting will be how businesses not just survive, but flourish.