Informatica Data Quality: Key to Reliable Data Management

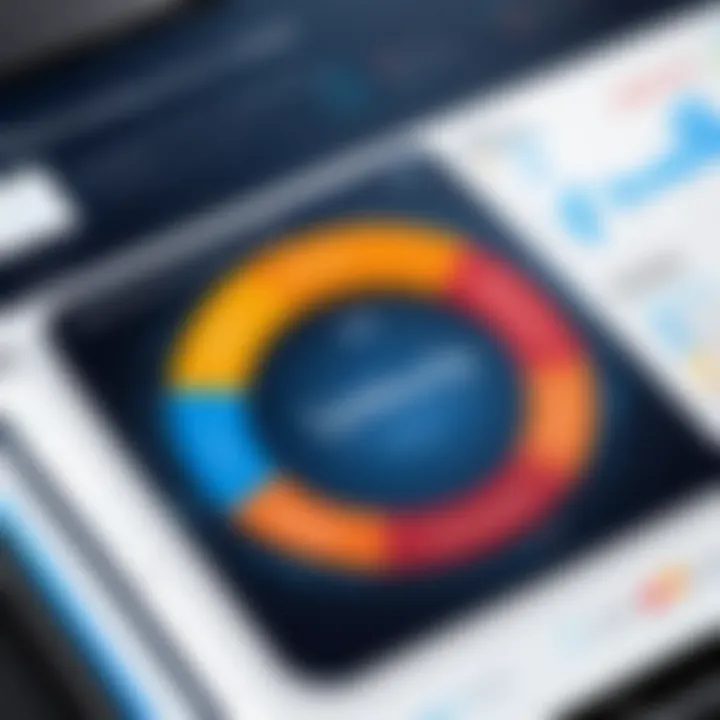
Intro
In the landscape of data management, quality stands as a pillar that supports not just the functionality of systems, but also the integrity of the information they harbor. As organizations dive deeper into data-driven practices, the significance of quality data becomes paramount. Today, we explore how Informatica Data Quality plays a crucial role in this paradigm, ensuring that businesses can rely on their data to pave the way for informed decision-making and operational efficiency.
In an era where data is often referred to as the new oil, organizations are discovering that unrefined, inaccurate, or inconsistent data can lead to significant risks. It is here that Informaticaâs suite of tools comes into play, offering comprehensive solutions designed to enhance the accuracy, consistency, and reliability of data across various systems. From identifying data entry errors to ensuring compliance with regulatory standards, Informatica Data Quality represents a robust framework for achieving reliable data management.
This article aims to shed light on the core functionalities of Informatica Data Quality. Readers will gain insights not only into the solutions offered by this platform, but also into the impact of superior data quality on business operations. By understanding how to harness the full potential of their data assets, organizations can not only boost their efficiency but also gain a competitive edge in today's fast-paced market.
Quality data is the foundation upon which effective strategies and decisions are built. As we delve into the key features, implementation processes, and best practices associated with Informatica Data Quality, expect to glean actionable insights aimed at maximizing data reliability.
Prelude to Informatica Data Quality
In todayâs overload of information, maintaining data integrity is no longer a luxuryâit's a necessity. Organizations are drowning in data, and without a solid framework to assess and improve its quality, the potential for poor decision-making looms large. This precipitates the need for robust tools and methodologies such as those offered by Informatica Data Quality.
Informatica Data Quality serves as a knight in shining armor for professionals grappling with the challenges of unreliable data. With its suite of functionalities, it provides a structured approach to ensure that data is not just plentiful but also credible and consistent. Implementing such a system is paramount for businesses striving for operational excellence and sustainable growth in a data-driven world.
Understanding the pillars of data qualityâaccuracy, completeness, consistency, and timelinessâforms the cornerstone of successful data management. Each dimension contributes to the broader narrative of data quality, driving organizations to make informed decisions, adhere to compliance standards, and optimize operations.
"Quality is not an act; it is a habit."
â Aristotle
Defining Data Quality
Data quality refers to the intrinsic and extrinsic attributes that data must possess to be deemed useful and reliable. At its most basic, it encompasses several critical criteria:
- Accuracy: The data correctly represents the real-world scenarios itâs intended to depict.
- Completeness: All necessary data is present, ensuring no significant gaps that could lead to misleading conclusions.
- Consistency: Data should remain stable across various records and systems, providing the same insights regardless of where it is retrieved from.
- Timeliness: The data is up-to-date and reflects current conditions, particularly crucial in fast-paced sectors where conditions change rapidly.
Moreover, understanding data quality isnât limited to identifying these factors. It's also about appreciating their interdependencies. For example, an increase in accuracy often leads to better completeness and vice versa. Each aspect is like a cog in a machine; if one part is out of whack, the others might struggle to function properly.
Understanding Informatica's Role
Informatica serves as the backbone in the quest for superior data quality. With tools dedicated to data profiling, cleansing, matching, and monitoring, it provides organizations with a comprehensive framework to assess their data landscapes thoroughly. The role of Informatica goes beyond mere analysis; it equips users to enact real change and maintain high standards over time.
Informaticaâs data profiling capabilities allow businesses to better understand their data's current state. By uncovering patterns and anomalies, decision-makers can develop strategies tailored to their unique data issues. This is beneficial not just for initiating improvements, but it also sets the stage for ongoing monitoring and refinement.
Data cleansing tools integrated into the platforms allow for automated correction of inconsistencies or errors, streamlining processes that otherwise might involve tedious manual adjustments. When the data is matched accurately, it ensures that duplicates are minimized, with connections made where necessary, ensuring a seamless flow of reliable information.
In essence, Informatica Data Quality isnât just a tool; itâs a comprehensive strategy to arm businesses with the reliability they need to thrive in a challenging data environment. Its multi-faceted approach enables businesses to not just focus on their data but invest in their future.
Core Components of Informatica Data Quality
In the landscape of data management, the core components of Informatica Data Quality serve as the backbone of robust data practices. Each element plays a pivotal role, creating a synergy that maximizes data accuracy and integrity. Organizations thrive in their objectives when they harness these components effectively, leading to informed decision-making and operational excellence.
Data Profiling
Data profiling is more than just analyzing data; it's about understanding the underlying quality of the data itself. This process involves collecting statistics and assessing the data's structure, content, and relationships within the dataset. For instance, consider a retail company analyzing customer purchase data. By profiling this data, the company can identify anomalies, such as unexpected duplicates in customer IDs or inconsistencies in product categories.
Through data profiling, organizations gain insight into:
- Data distribution and patterns
- Completeness of records
- Data types and formats
This understanding is essential as it sets the stage for subsequent cleansing operations and ensures cleaner, more reliable datasets for further processing.
Data Cleansing
Once data profiling has highlighted the discrepancies, the next step is data cleansing. This step involves correcting or removing inaccurate, incomplete, or irrelevant data from the dataset. Imagine a healthcare provider faced with inconsistent patient recordsânames spelled differently or misregistered birthdates can lead to catastrophic outcomes. Here, data cleansing comes into play.
Informatica provides tools that automate parts of this process, which can involve:
- Standardizing data formats (e.g., dates consistently displayed as MM/DD/YYYY)
- Removing duplicates to ensure unique records
- Correcting misspelled entries or incorrect information
Through effective cleansing, businesses can significantly reduce error rates and enhance the reliability of their data.
Data Matching
Data matching focuses on identifying and linking records that refer to the same entity across different datasets. This process can be akin to solving a puzzleâmatching data from disparate sources to provide a unified view. Consider a bank integrating records from various branches. Without proper matches, a single customer could be registered multiple times with different identifiers, leading to confusion and inefficiencies.
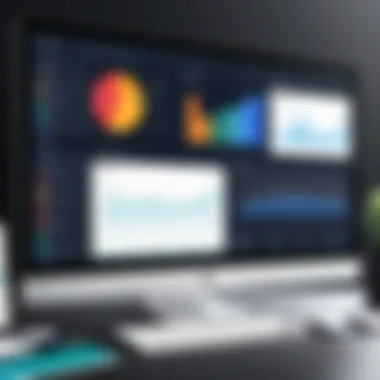
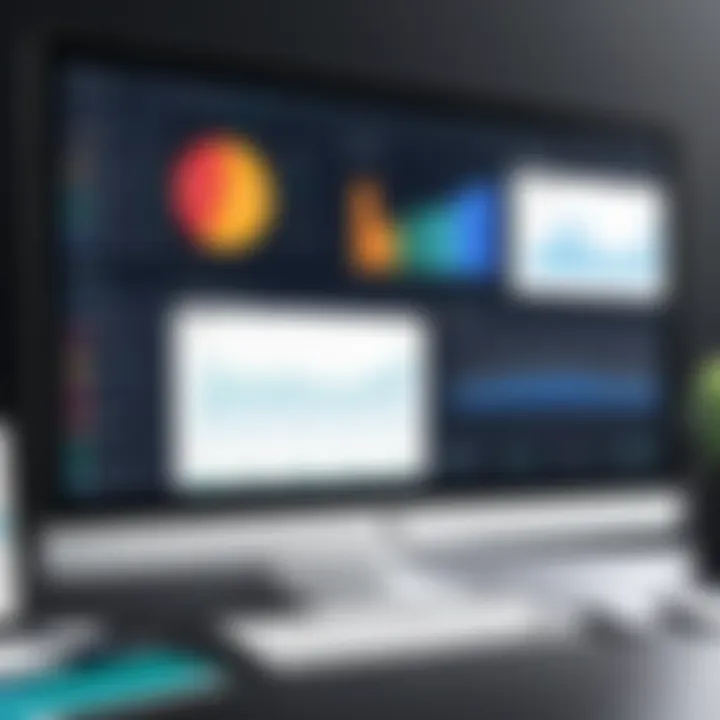
The data matching process involves:
- Defining matching criteria based on attributes like names or addresses
- Evaluating similarity to decide if records qualify as matches
- Merging records once confirmed to be duplicates
By ensuring that data sets are aligned, companies bolster their analytics capabilities, ultimately leading to improved customer insights and more targeted strategies.
Data Monitoring
The final cornerstone is data monitoring. This ongoing process ensures that data quality is maintained over time. It involves regularly inspecting data to catch new issues as they arise. For example, if an organization adds new data sources, it's vital to monitor how these integrate with existing data to safeguard against quality degradation.
Key focus areas of data monitoring include:
- Tracking changes in data quality metrics over time
- Alerting stakeholders to anomalies in data trends
- Verifying adherence to quality standards and compliance regulations
Ultimately, consistent monitoring helps organizations react swiftly to potential data issues, ensuring they maintain a steady course of quality in their data management practices.
"Maintaining high data quality is not a one-time effort, it's an ongoing commitment that paves the way for informed decision-making and operational success."
In summary, the core components of Informatica Data Quality work together like cogs in a well-oiled machine. Each component enhances the overall framework, supporting businesses in fostering a data-centric culture that prioritizes accuracy, consistency, and reliability.
The Importance of Data Quality
In the vast landscape of data management, the importance of data quality cannot be overstated. Reliable data serves as the bedrock for informed decision-making, resource allocation, and strategic planning. In today's fast-paced and data-driven environment, quality data turns into a valuable asset. Businesses that ignore this aspect risk making decisions based on misleading or incorrect information, which can lead to devastating consequences. Therefore, developing a strong grasp of data quality is paramount for companies aiming to thrive.
Effective data management involves more than just mere collection; it includes ensuring that data is accurate, complete, and consistent. Neglecting these fundamentals can result in spending money on flawed metrics or even basing critical decisions on misinformation.
Impact on Business Decisions
When business leaders rely on data to steer their strategies, poor data quality can lead to misguided conclusions. For instance, if sales reports contain discrepancies, a company might misinterpret their market performance. This can precipitate excess inventory or even a redundant marketing push, tying up resources that could otherwise be utilized more effectively.
In the realm of analytics, high-quality data enables organizations to accurately identify trends and forecasts. Think of it like using a compass; it only directs you to your destination if itâs calibrated correctly. When data is flawed, the insights drawn become unreliable, which ultimately impedes business growth.
Some core components affecting business decisions include:
- Informed Decision Making: Quality data provides clarity, paving the way for strategic choices that align with company goals.
- Performance Monitoring: Accurate metrics allow leadership to gauge the effectiveness of various initiatives.
- Customer Relationship Management: Properly managed customer data can enhance personal interactions and improve customer satisfaction, thus fostering loyalty.
Regulatory Compliance
Regulatory frameworks often mandate data management practices that prioritize accuracy and consistency. Failing to comply can lead to legal repercussions and substantial penalties. Organizations are increasingly scrutinized regarding the quality of their data, especially in industries like finance and healthcare where trust and accuracy are critical.
Poor quality data can jeopardize compliance efforts, resulting in lost credibility. To counter these risks, ensuring data integrity can often lead to a stronger reputation and trust from both clients and regulatory bodies.
Some key elements regarding compliance include:
- Maintaining Records: Regulatory agencies require precise data for audits, necessitating quality control protocols.
- Data Security: Ensuring only accurate and appropriately handled data improves overall security, minimizing the risk of breaches.
- Transparency: Quality data allows for clear reporting and accountability, which in turn builds credibility with stakeholders.
Operational Efficiency
Organizations that prioritize data quality often experience improved operational efficiency. Quality data permits smoother processes, drives accurate reporting, and facilitates better coordination among departments. For example, when teams are aligned with accurate information, they can collaborate more effectively without wasting time on correcting errors.
Achieving operational excellence with data quality involves:
- Streamlining Processes: Efficient operations stem from reliable data, reducing the scope for manual effort that often creates room for errors.
- Resource Allocation: Accurate data helps in smart allocation, ensuring that resources are utilized where they are most effective.
- Boosting Productivity: Employees can focus on task execution instead of mending data inaccuracies, leading to enhanced productivity.
"Quality is not an act, it is a habit." This proverb rings true in the realm of data management. Emphasizing data quality cultivates a culture of excellence.
In summary, the importance of data quality is deeply entwined with business decisions, regulatory compliance, and operational efficiency. Organizations must recognize that the stakes are high; investing in robust data management processes pays dividends by empowering better decision-making and supporting sustainable growth.
Implementing Informatica Data Quality
Implementing Informatica Data Quality is no small feat, yet its significance in ensuring reliable data management cannot be overstated. Before organizations dive headfirst into this process, understanding the nuances and preparatory steps is crucial. This phase sets the stage for successful data quality initiatives, allowing organizations to harness the immense power that clean, reliable data can bring. From enhancing decision-making processes to boosting business performance, a well-planned implementation strategy paves the way.
Pre-Implementation Considerations
Before embarking on the journey of implementing Informatica Data Quality, organizations must take a step back and assess their current data landscape. Identifying the specific data quality challenges faced is essential. These could range from inconsistent data entries to duplicates lurking in the shadows. Here are some key considerations:
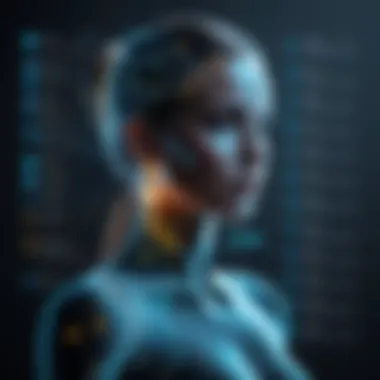
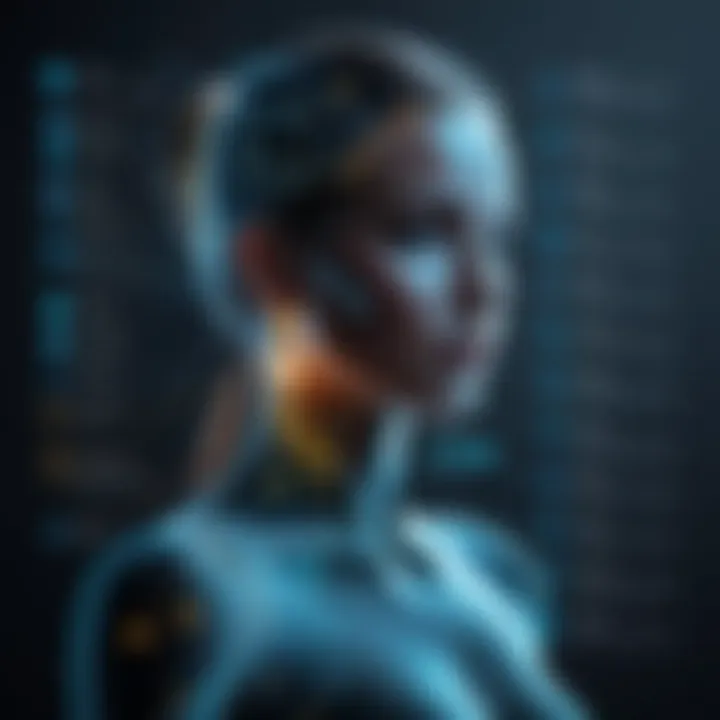
- Current Infrastructure: Evaluate existing data systems and understand how they interact.
- Goals and Objectives: Clearly define what you want to achieve with Informatica Data Quality. Do you aim to improve data accuracy, or are you focusing on operational efficiency?
- Stakeholder Engagement: Include all relevant team members in discussions, ensuring that buy-in is established across the board.
Gathering requirements at this stage ensures that the subsequent steps align with the overall company strategy.
Keys to Successful Deployment
The deployment of Informatica Data Quality systems is crucial in converting theoretical plans into practical success. A few elements stand out as pivotal to achieving this:
- Training and Education: Prepare your team through appropriate training initiatives. This goes beyond basic introductions to the software; itâs about instilling a deeper understanding of how it can be wielded effectively.
- Pilot Projects: Conduct small-scale pilot projects to test out functionalities in a controlled environment. These acts as trial runs, spotlighting potential hiccups and allowing for adjustments before a full rollout.
- Monitoring and Adjustment: Once the system is live, keep a keen eye on its performance. Gather feedback continuously and be ready to make necessary adjustments, whether they come from end-users or predefined metrics.
A holistic view during deployment can prevent roadblocks and ensure data quality practices take root effectively.
Post-Implementation Review
After implementation, itâs essential to pause and assess. A post-implementation review not only gauges the success of the deployed solutions but also highlights areas for improvement. Hereâs what to focus on during this review phase:
- Metrics Evaluation: Measure key performance indicators (KPIs) determined during the planning phase to see if theyâve been met.
- User Feedback: Solicit feedback from users who engage with the data quality tools, understanding their experiences and identifying any pain points.
- Future Improvements: Reflect on what adjustments can be made for better operations down the line, ensuring your data quality initiative evolves.
Best Practices for Maintaining Data Quality
Maintaining data quality is more than just a routine task; it's a strategic necessity. In a world increasingly reliant on data, organizations must prioritize ensuring that their data is accurate, consistent, and trustworthy. Adopting best practices in data quality management can save resources, improve decision-making processes, and ultimately bolster an organizationâs reputation. Here are several key elements that contribute to effective data quality maintenance:
Regular Data Audits
Regular data audits act as a health check on data integrity. Conducting these audits frequently ensures that the data aligns with its intended purpose and is free from discrepancies. Organizations can establish a schedule to review datasets, which can range from weekly to quarterly, depending on data volume and criticality.
- Identify anomalies: By scrutinizing data, teams can catch errors that might otherwise snowball into significant issues.
- Establish baselines: Auditing provides a benchmark for performance, making it easier to track improvements over time.
- Engage stakeholders: Involving various departments in the audit process fosters a culture of accountability.
In short, audits not only highlight errors but can also unveil opportunities for process improvements.
User Training and Awareness
Investing in user training is paramount for sustainable data quality. Tools like Informatica Data Quality are sophisticated, and without proper understanding, users might not utilize them effectively. Itâs crucial for organizations to:
- Educate employees: Regular training sessions can empower users to understand data quality principles and the importance of their roles in maintaining it.
- Create awareness: It's essential to promote a culture that values data integrity; awareness campaigns can ensure data quality remains top of mind for all employees.
When teams are well-informed about data quality procedures, they tend to make smarter decisions that reflect positively on overall organizational performance.
Automation of Processes
Automating data quality processes can be a game changer. Manual interventions, though sometimes necessary, often introduce errors or delays. Automation ensures a streamlined approach which brings with it several benefits:
- Consistency: Automated processes deliver uniform outcomes consistently, reducing chances of human error.
- Efficiency: Repetitive tasks can become time-consuming. Automation frees up human resources to focus on higher-level analytical tasks.
- Real-time monitoring: Automated systems can provide continuous oversight of data, ensuring immediate identification of quality issues.
"In the realm of data, speed and accuracy are king; automation places your organization at the throne."
Embracing automation in data quality practices not only enhances precision but also supports agile responses to data shifts.
In summary, adopting these best practices lays a strong foundation for maintaining data quality. Regular audits keep data in check, user training fosters a culture of accountability, and automation streamlines processes. Each element reinforces the other, creating a robust system that champions reliable data management.
Challenges in Data Quality Management
Managing data quality isnât just a walk in the park. Setting aside the tangled web of data systems, organizations face multifarious challenges that can, if ignored, lead to a quagmire of inaccuracies and inefficiencies. In order to truly understand the significance of data quality management, it is critical to navigate through these challenges and realize their implications on both the data itself and the broader organizational landscape.
Data Complexity
The modern digital landscape has bolstered the volume, variety, and velocity of data. This complexity can become a double-edged sword; while big data presents vast opportunities for insightful decision-making, it also complicates the data quality landscape significantly.
When organizations collect data from diverse sources â think customer transactions, social media interactions, and IoT devices â it creates a potpourri of formats and structures. For example, a name could appear as "John Smith" in one database and "Smith, John" in another. Such inconsistencies can lead to challenges in data integration and reporting. Additionally, this complexity often necessitates sophisticated data governance frameworks, which, if not properly implemented, can spiral into chaos.
One way organizations can tackle complexity is by investing in robust data profiling tools. These tools can help to identify inconsistencies and gaps within datasets, allowing data custodians to address issues proactively before they snowball into larger problems.
Integration Issues
With numerous data sources come a myriad of integration challenges. Perhaps youâve heard the saying, "Squaring the circle," which is very apt here. Integrating disparate systems often feels like trying to fit a square peg in a round hole. If you donât have a strategy in place, you could end up with siloed data that is both incomplete and unreliable.
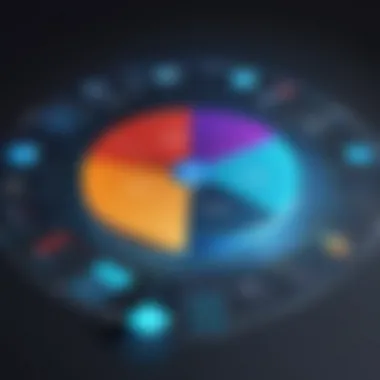
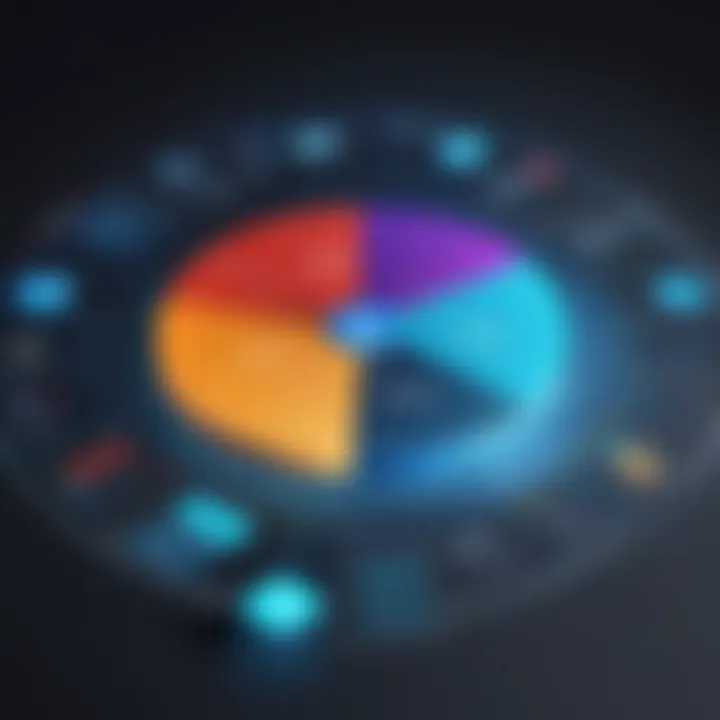
Issues such as incompatible data formats or different storage architectures can create bottlenecks that slow down the entire data pipeline. Moreover, any discrepancies arising from misaligned data standards further complicate these integration efforts. For instance, a retail company may utilize point-of-sale systems that record customer purchases differently than its e-commerce platform. This raises the risk of having conflicting customer data, leading to a poor understanding of customer behavior.
Addressing integration issues may involve employing ETL (Extract, Transform, Load) tools like Informatica PowerCenter. These tools can help streamline the process by transforming and consolidating data into a unified format, thus fostering seamless integration across systems.
Resource Constraints
Lastly, it's worth noting that resource constraints can pose a significant barrier to achieving quality data management. Many organizations donât have unlimited budgets or staffing resources to devote solely to data quality initiatives. This can result in under-resourced teams that struggle to keep up with the demands of managing vast amounts of data.
A common scenario is that companies may prioritize immediate operational tasks over long-term data quality strategies. Budget constraints might lead to decisions such as opting for cheaper, less comprehensive data quality solutions. Consequently, this can create a vicious cycle where poor data quality persists due to insufficient investment in tools and training.
Overcoming resource constraints revolves around smart choices. Organizations might consider engaging in training programs for existing staff, effectively utilizing in-house talents. Additionally, adopting cost-effective data management solutions can help businesses stretch their budgets further, ensuring that the essential processes remain intact without breaking the bank.
"Data quality challenges donât just stem from the data itself, but from the systems, processes, and people handling that data."
By addressing these challenges head-on, organizations can lay the groundwork for effective data quality management that not only augments data reliability but enhances decision-making processes across the board.
Future Trends in Data Quality
In today's fast-paced digital environment, the stakes for maintaining data integrity are higher than ever. Companies are constantly looking for ways to improve their data quality strategies. The emerging trends in data quality management are critical for addressing the challenges businesses face in this rapidly evolving landscape. By understanding new insights and technologies, organizations can ensure they maintain a competitive edge, streamline their operations, and make informed decisions based on reliable data.
AI and Machine Learning in Data Quality
The integration of artificial intelligence (AI) and machine learning into data quality processes is reshaping the way businesses manage their data assets. AI can analyze massive datasets and identify patterns at a speed and accuracy that is beyond human capacity. This technology enables automated anomaly detection, recognizing outliers or errors that traditional methods might miss. Furthermore, machine learning algorithms can continuously improve themselves by learning from past mistakes, ultimately making the data management process smarter over time.
- Benefits:
- Automated data cleansing processes that drastically reduce manual effort.
- Real-time data quality monitoring allows for prompt corrections.
- Enhanced decision-making capabilities through predictive analytics.
The bottom line? AI and machine learning are not merely trends; they are pivotal enablers of a data-driven culture, ensuring that the data businesses rely on every day is not only accurate but also enriched with insights.
Self-Service Data Quality Tools
Empowering users with self-service data quality tools is another significant trend making waves in data management. Traditionally, data quality tasks fell into the lap of IT departments, which often led to bottlenecks. Today, self-service tools allow end-users to take charge.
With tailored interfaces, users can monitor, cleanse, and validate their own data without needing extensive technical knowledge. This democratization of data quality management brings a host of advantages:
- Advantages:
- Increased efficiency: End-users can resolve data issues promptly, seeing the benefits without the lengthy queues for IT assistance.
- Heightened ownership: Users take responsibility, promoting accountability across the organization.
- Cost savings: Reducing reliance on IT for routine data quality tasks lowers operational expenses.
Data Governance Integration
As organizations navigate the complexities of data governance, integrating data quality management becomes crucial. Strong data governance frameworks ensure data is not only accurate but also secure and compliant with regulations.
In todayâs landscape, where data breaches and regulatory scrutiny are at an all-time high, having robust data governance is essential. Organizations are beginning to recognize data quality as a fundamental aspect of governance, not a separate discipline. Key considerations for integrating data quality into governance include:
- Collaboration: Between various departments; cross-functional teams must work together to align data quality efforts with governance mandates.
- Policy Development: Clear policies must be established that outline data quality measures, standards, and expectations.
- Ongoing Training and Support: Providing continuous education on data quality and governance issues ensures all staff are aligned and aware of their responsibilities.
In summary, acknowledging the trends shaping data quality management is not simply about keeping up with the latest technologies. Itâs about fostering a resilient data culture that promotes accuracy, enhances trust, and ultimately guides organizational success.
"Data quality is not a destination but a journeyâa continuous effort toward improvement and reliability."
By embracing these upcoming trends, organizations can lay a strong foundation for future advancements in their data quality strategies.
The End
Understanding the pivotal role of data quality is essential for organizations navigating the modern data-centric environment. Informatica Data Quality equips businesses with robust tools to not only manage their data but also ensures the integrity and reliability of the information that drives decision-making. This article highlights various aspects of data quality, emphasizing its importance across multiple dimensions of business operations.
Summary of Key Points
In summary, the exploration of Informatica Data Quality reveals several critical insights:
- Importance of Data Quality: Reliable data forms the bedrock of informed decision-making. When organizations implement stringent data quality measures, they significantly boost their operational efficiency and effectiveness.
- Core Components: Key functionalities such as data profiling, cleansing, matching, and monitoring emerge as essential to maintaining high-quality data standards. By leveraging these capabilities, businesses can effectively manage the complexities associated with data.
- Challenges and Solutions: Data complexity, integration issues, and resource constraints are indeed significant hurdles. However, recognizing these challenges allows organizations to proactively address and mitigate potential setbacks.
- Best Practices: Regular audits, user training, and process automation stand as best practices that help in upholding data quality over time. By instilling a culture centered around data quality, organizations can ensure their data remains an asset rather than a liability.
- Future Trends: The integration of AI and machine learning, coupled with self-service tools and robust data governance, points to a future where data quality management is becoming increasingly sophisticated. Keeping an eye on these trends will be crucial.
The Path Ahead
Looking towards the future, professionals and decision-makers must acknowledge that the landscape of data quality is rapidly evolving. Organizations should prioritize investing in cutting-edge technologies that enhance data reliability. Training staff on the significance of data integrity is equally vital; a knowledgeable team can be the difference between success and failure in data quality initiatives.
As companies adapt to emerging trendsâsuch as artificial intelligence making data processes more intuitiveâstaying informed about advancements is crucial. Data governance will play an important part in ensuring that all data management practices align with organizational goals and compliance regulations. In essence, laying down a solid foundation now, grounded in the principles of Informatica Data Quality, will pave the way for future successes in data management.
As we move forward, embracing these technologies and methodologies not only allows for better operational decision-making but also provides businesses the ability to thrive in a competitive marketplace.