Master Data Management vs Data Warehouse Explained
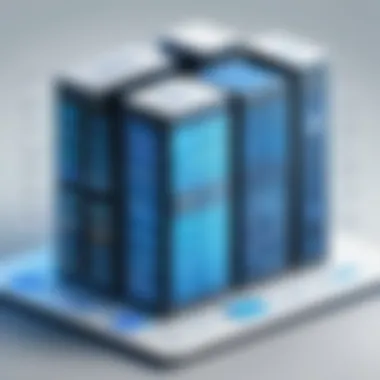
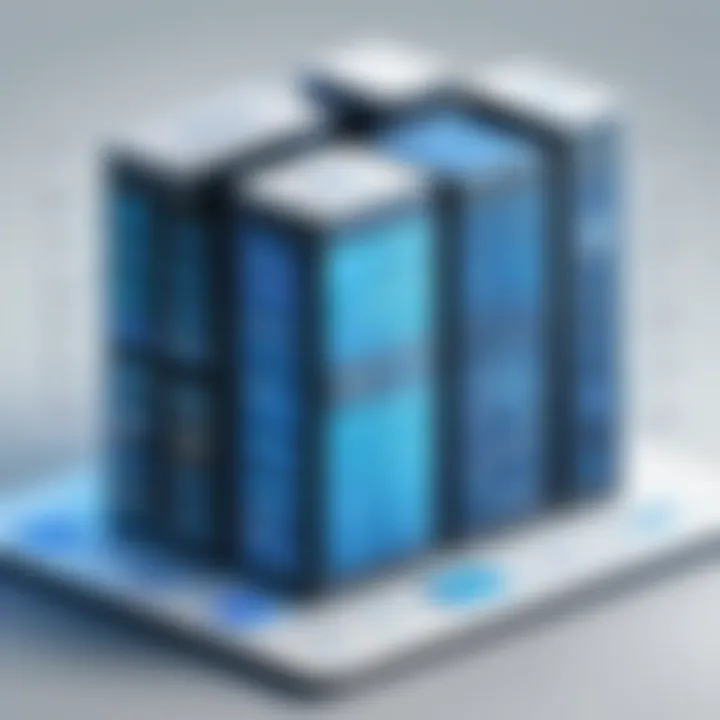
Intro
In today's data-driven world, organizations face a dilemma when it comes to managing information. Two prominent strategies surface: Master Data Management (MDM) and Data Warehousing. Understanding the differences between these systems is essential for making informed decisions regarding data governance, analytics, and overall business strategies.
While MDM focuses on ensuring the accuracy and consistency of key business information, Data Warehousing emphasizes the storage and retrieval of vast amounts of historical data for analysis and reporting purposes. This article delves into the essential aspects of both MDM and Data Warehousing, offering insights into their functionalities, methodologies, and practical applications.
Technological Research Overview
Recent Technological Innovations
With the rapid advancement of technology, both MDM and Data Warehousing have evolved significantly. Innovations such as cloud computing have provided organizations with scalable solutions for data storage and management. Furthermore, the integration of real-time data processing enables businesses to leverage insights rapidly, enhancing decision-making processes.
Organizations increasingly adopt MDM tools like Informatica Master Data Management and SAP Master Data Governance. These tools not only simplify data management but also ensure compliance with data quality standards. Meanwhile, Data Warehouse solutions such as Snowflake and Amazon Redshift offer robust analytics capabilities, making them popular choices for handling large volumes of data.
Impact on Business Operations
The integration of MDM and Data Warehousing fundamentally transforms business operations. MDM helps to create a single source of truth, eliminating data silos and ensuring that all departments share accurate information. This uniformity fosters collaboration and enables companies to respond more effectively to market changes.
Conversely, Data Warehousing supports data-driven decision-making by providing a comprehensive view of historical trends. Businesses can generate reports and analytics that inform strategic planning, marketing campaigns, and financial forecasting. The synergy between these approaches cultivates an environment where data drives innovation and competitiveness.
Future Technological Trends
As technology continues to advance, it is crucial to consider emerging trends that may shape the future of data management. For instance, artificial intelligence (AI) is poised to play a significant role in both MDM and Data Warehousing. AI can enhance data quality by automating the cleansing process and ensuring consistency across various datasets. Moreover, predictive analytics facilitated by AI can unlock valuable insights, allowing organizations to anticipate future trends and make proactive decisions.
Additionally, the adoption of decentralized technologies, such as blockchain, may influence Data Warehousing and MDM strategies. By providing enhanced transparency and security, blockchain can reinforce trust in data integrity, which is critical for effective governance.
"Understanding the unique functionalities of MDM and Data Warehousing is fundamental to achieving effective data management in contemporary business environments."
Data Analytics in Business
Importance of Data Analytics
Data analytics has become an indispensable aspect of modern business. It enables organizations to harness the power of data to gain insights into customer behavior, market trends, and operational efficiency. By integrating robust data analytics capabilities with MDM and Data Warehousing, companies can position themselves for sustained growth.
Tools for Data Analysis
A variety of tools are available for data analysis, ranging from traditional business intelligence platforms like Tableau and Microsoft Power BI to advanced analytics solutions like DataRobot and Alteryx. These tools can process data stored in Data Warehouses while also observing rules set forth by MDM practices, ensuring consistent and reliable results.
Case Studies on Data-Driven Decisions
In practical scenarios, businesses that invest in data analytics have witnessed remarkable outcomes. For instance, a retail company employing MDM practices alongside a well-structured Data Warehouse improved its inventory management by analyzing sales patterns and reducing excess stock. This resulted in cost savings and enhanced customer satisfaction.
Cybersecurity Insights
Threat Landscape Analysis
As organizations embrace digital transformation, the threat landscape for data security has become increasingly complex. Cyberattacks can compromise the integrity of both MDM and Data Warehousing systems, posing risks to sensitive business information.
Best Practices for Cybersecurity
To mitigate these threats, implementing best practices is essential. Organizations should adopt multi-factor authentication, encrypt sensitive data, and regularly conduct security audits. Ensuring that both MDM and Data Warehousing systems are secured prevents unauthorized access and preserves data integrity.
Regulatory Compliance in Cybersecurity
Regulatory compliance is another vital aspect of data management. Organizations must adhere to regulations such as GDPR and HIPAA to ensure data privacy. Aligning MDM and Data Warehousing strategies with these compliance requirements safeguards businesses from potential legal ramifications.
Artificial Intelligence Applications
AI in Business Automation
The integration of artificial intelligence in data management processes has proven beneficial. AI can automate routine tasks such as data entry and cleansing, reducing human error. As a result, MDM systems can maintain high-quality data, while Data Warehousing can efficiently manage queries.
AI Algorithms and Applications
AI algorithms, particularly in machine learning, facilitate advanced analytics capabilities. By analyzing patterns in historical data stored in Data Warehouses, these algorithms help identify trends and support predictive analytics initiatives.
Ethical Considerations in AI
While the benefits of AI in data management are significant, ethical considerations must be addressed. Ensuring fairness in algorithms and safeguarding against data bias is crucial. Implementing transparent AI practices will build trust with stakeholders and maintain compliance with ethical standards.
Industry-Specific Research
Tech Research in Finance Sector
In the finance sector, MDM and Data Warehousing play critical roles in risk management and regulatory reporting. Financial institutions utilize MDM to maintain accurate customer and transaction records, while Data Warehousing supports comprehensive analysis of financial data.
Healthcare Technological Advancements
Healthcare organizations depend on accurate data for patient care. MDM helps ensure that patient records are consistent across systems, while Data Warehousing enables the analysis of vast amounts of healthcare data, ultimately improving patient outcomes.
Retail Industry Tech Solutions
In retail, the application of MDM and Data Warehousing enhances supply chain management and customer relationship management. Retailers can analyze sales trends and customer preferences for better inventory decisions and personalized marketing strategies.
Understanding the distinct roles of MDM and Data Warehousing helps organizations navigate the complexities of data management effectively. With informed decisions, businesses can harness the power of their data to drive success.
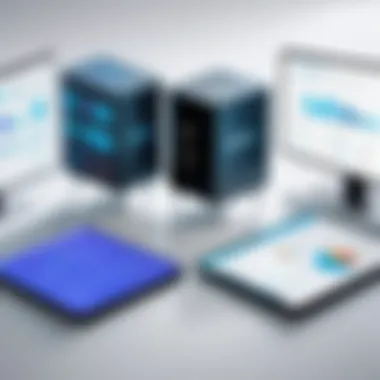
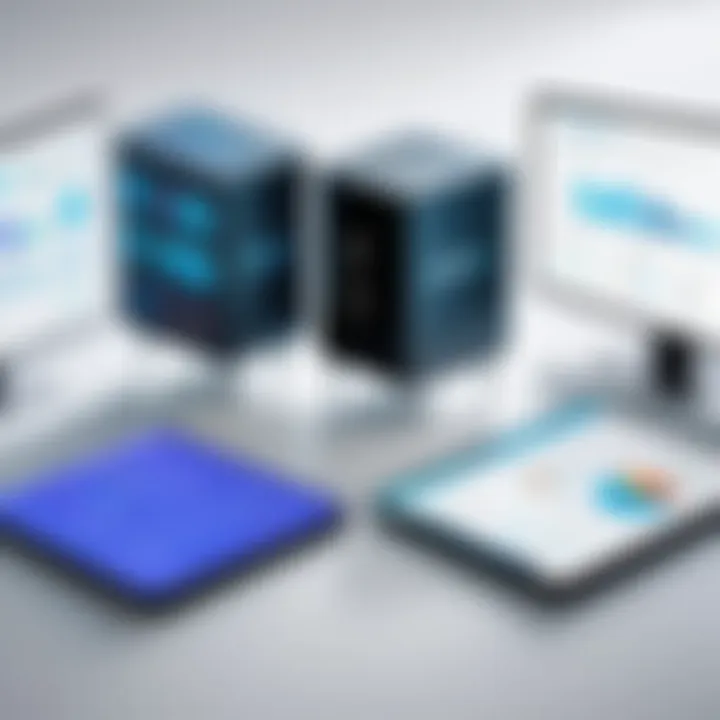
Foreword to Master Data Management and Data Warehousing
In today's fast-paced business environment, managing data effectively is crucial for gaining competitive advantages. Master Data Management (MDM) and Data Warehousing are two integral components of this landscape. Understanding their roles and functionalities can lead to improved decision-making, streamlined operations, and better data governance.
Master Data Management is focused on ensuring the consistency and correctness of a company's critical data assets. This includes data about customers, products, and suppliers, which are crucial for business operations. MDM enhances data quality, reduces redundancy, and fosters shared understanding among various systems and users.
On the other hand, Data Warehousing serves as a central repository for data gathered from various sources. It allows for complex queries and analysis, providing insights that inform strategic decisions. Data Warehousing can consolidate historical data to track performance over time, thus facilitating long-term analysis and planning.
By diving into the definitions and purposes of these systems, we set the foundation for understanding their benefits and the interplay between them. Both MDM and Data Warehousing play distinct yet complementary roles in a robust data management strategy.
Core Principles of Master Data Management
Master Data Management (MDM) is essential for organizations aiming to maintain consistency in data across various systems. Understanding its core principles enhances the effectiveness of data management strategies. MDM provides a structured approach to data governance, ensuring data integrity, usability, and compliance. This article examines its key components that drive successful MDM programs.
Data Ownership and Stewardship
In MDM, data ownership refers to the accountability for data within an organization. It's crucial because it establishes who is responsible for the quality and maintenance of data assets. Stewards play a vital role, acting as intermediaries between data producers and consumers.
Benefits of Data Ownership and Stewardship:
- Clear Responsibilities: Knowing who owns the data helps prevent confusion and overlaps in duties.
- Enhanced Data Quality: Owners are incentivized to maintain high standards, ensuring reliable data for decision-making.
- Facilitated Compliance: With clear stewardship, organizations can better adhere to regulations governing data management.
Data Quality Management
Data quality management focuses on maintaining high standards for data collected and utilized by organizations. Poor data quality can lead to misguided business decisions, misallocation of resources, and compliance issues. Therefore, establishing rigorous data quality metrics is fundamental.
Key Elements of Data Quality Management:
- Accuracy: Data must accurately represent reality.
- Completeness: All necessary data should be present and accounted for.
- Consistency: Data should remain consistent across different systems and platforms.
- Timeliness: Data must be up-to-date to be useful in decision-making.
Employing tools for monitoring data quality can vastly improve an organization's ability to address issues promptly.
Data Integration Strategies
Effective data integration strategies are at the heart of MDM. They involve consolidating data from different sources to create a unified view. Integration enables organizations to break down data silos and fosters better overall data management.
Common Data Integration Approaches:
- ETL (Extract, Transform, Load): This traditional method remains popular for batch processing of data.
- Real-time Integration: Leveraging APIs allows for immediate updates, reflecting real-time changes.
- Data Virtualization: This approach enables access to data without moving it, providing flexibility in data usage.
Incorporating a mix of these strategies can help organizations utilize their data more effectively, leading to enhanced analysis and reporting capabilities.
"The success of MDM relies heavily on the principles of data stewardship, quality, and effective integration strategies, fostering an environment of reliability and trust in data."
By focusing on these core areas, organizations can strengthen their MDM initiatives. Addressing data ownership and stewardship leads to improved accountability, while rigorous data quality management and strategic integration pave the way for a data-driven culture.
Core Principles of Data Warehousing
Understanding the core principles of data warehousing is crucial for any organization looking to harness the power of its data assets. Data warehousing serves as a central repository of integrated data from multiple sources, and it enables users to conduct analysis and reporting. The architecture, processes, and adjunct capabilities influence how effectively data can be utilized for decision-making.
ETL Processes Explained
ETL stands for Extract, Transform, Load. This is a critical process in data warehousing. It involves three key stages that are essential for populating the data warehouse.
- Extract: The first step involves extracting data from various sources. These sources can range from databases, logs, external applications, and more. The challenge lies in ensuring that the extraction process is efficient and does not hinder the performance of the source systems.
- Transform: Once the data is extracted, it undergoes a transformation process. This conversion includes cleaning, filtering, and organizing the data to ensure uniformity and relevance. Transformation also addresses discrepancies in data formats and eliminates redundancies.
- Load: Finally, the transformed data is loaded into the data warehouse. This process must be carefully managed to maintain the integrity of the warehouse and ensure it functions optimally. The timing and method of loading can significantly impact user access and query performance.
In summary, the ETL processes establish the groundwork for effective data usage in analytical operations.
Data Storage Architecture
The architecture of a data warehouse is vital as it affects retrieval speed and storage efficiency. A traditional architecture typically consists of staging, data integration, and presentation layers.
- Staging Layer: This is where the raw data from various sources is collected following the extract phase. The staging area acts as a temporary holding place for the data before transformation.
- Data Integration Layer: At this layer, after the ETL process, the data is organized and stored in a structured way. This usually follows a star or snowflake schema that defines how the data entities relate to each other. This structure supports efficient querying and reporting.
- Presentation Layer: This is the user-facing side of the data warehouse where reports and dashboards can access and present data. Data in this layer is typically summarized for faster access.
The right architectural design can help organizations achieve scalability and performance while accommodating future data growth appropriately.
Analytic Processing Types
Analytic processing is a core function of data warehouses, enabling organizations to make informed decisions based on reported data. There are several types of processing, each suitable for different analytical needs.
- Online Analytical Processing (OLAP): This type allows users to perform multidimensional analysis of business data, providing the capability to analyze different perspectives (like sales by region, product line, etc.) in a time-efficient manner.
- Data Mining: This involves examining large datasets to uncover patterns and relationships. Data mining tools help organizations predict outcomes and trends, essential for strategic planning.
- Reporting Services: These services enable users to create reports that summarize data findings. Reports can articulate insights that inform operational and strategic decisions.
Ultimately, understanding the types of analytic processing aids in aligning data strategies with business objectives. This alignment enhances data-driven decision-making capability in organizations.
"The effectiveness of data warehousing hinges on the strategic execution of its core principles."
Comparative Analysis of Objectives
The Comparative Analysis of Objectives section serves as a pivotal part of this article. Understanding the differing aims of Master Data Management (MDM) and Data Warehousing is essential for professionals looking to enhance their data strategies. By clarifying their goals, organizations can make informed decisions about which approach to adopt based on their unique needs and contexts.
Goals of Master Data Management
Master Data Management aims primarily at ensuring data consistency and accuracy across the enterprise. This involves the creation and maintenance of a single, authoritative source of critical business data, which helps in reducing redundancy and discrepancies. Here are some core objectives of MDM:
- Data Consolidation: MDM integrates data from multiple sources, creating a unified view of essential entities like customers and products.
- Data Quality: The focus is on improving data quality through validation processes, which ensures that users access accurate and reliable information.
- Data Governance: Establishing stewardship roles and procedures, MDM supports regulatory compliance and enhances accountability for data management practices.
- Customer Insights: By providing a 360-degree view of customers, MDM improves the ability to analyze customer behavior and preferences, driving targeted marketing and enhanced service delivery.
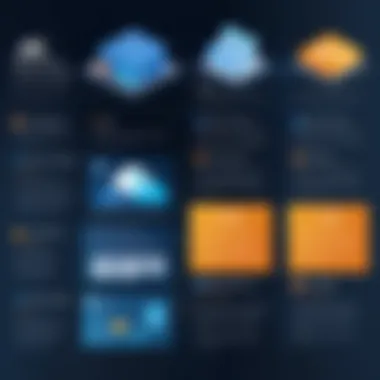
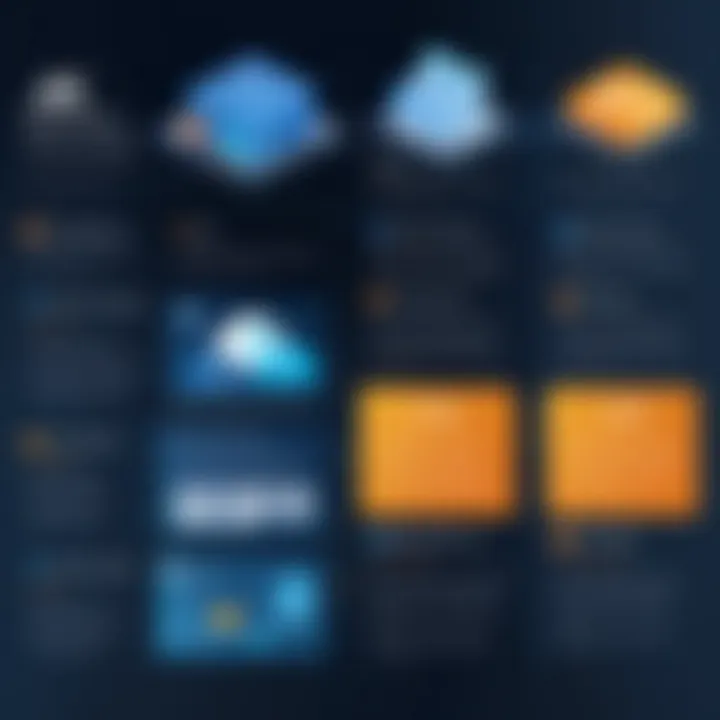
In many organizations, these goals lead to better decision-making and increased operational efficiency.
Goals of Data Warehousing
Data Warehousing, on the other hand, primarily focuses on the effective storage and retrieval of data for analytic purposes. Its objectives differ significantly from those of MDM:
- Historical Analysis: Data Warehouses are designed to store large volumes of historical data for trend analysis and reporting, which helps in strategic planning and forecasting.
- Support Business Intelligence: They enable complex queries and analytics, supporting tools like Tableau and Microsoft Power BI for insightful dashboards and reports.
- Data Aggregation: Aggregating data from various operational sources, Data Warehousing provides a coherent view for analysis, enhancing business insights.
- Performance Optimization: With a structure designed for quick querying, Data Warehousing improves the performance of data retrieval processes, which is crucial for timely decision-making in fast-paced environments.
Taken together, these goals illustrate the unique contributions of MDM and Data Warehousing in shaping effective data strategies.
Use Cases and Applications
The understanding and implementation of Master Data Management (MDM) and Data Warehousing are crucial for organizations striving to optimize their data strategies. Both these systems not only enhance data quality but also streamline decision-making processes. The use cases and applications of MDM and Data Warehousing span various industries, illustrating their benefits and importance in real-world scenarios.
Use Cases in Organizations
Master Data Management is pivotal in many organizational settings. It assures that the core data entities, such as customers, products, and suppliers, are accurate and consistent across the board. For instance:
- Customer Data Management: With a robust MDM solution, companies can create a single customer view, merging information from various sources. This leads to improved customer relationship management.
- Product Information Management: Organizations that rely on numerous data sources for product information can use MDM to maintain a unified repository. This ensures that sales channels reflect the same product data, thus avoiding discrepancies.
- Regulatory Compliance: In sectors like healthcare and finance, accurate master data is imperative for compliance with regulations. MDM can support businesses by providing correct and up-to-date data for audits and reporting.
- Merger and Acquisition Integration: During mergers, organizations face the challenge of integrating data from different entities. MDM helps to resolve duplicate records and standardize data definitions, enabling smoother transitions.
"MDM is not just a data management practice; it’s a strategic initiative that aligns data with business objectives."
By focusing on these use cases, it becomes clear that MDM plays a vital role in enhancing organizational efficiency and effectiveness.
Data Warehouse Applications in Business Intelligence
Data Warehousing serves as a backbone for Business Intelligence (BI), enabling organizations to convert raw data into actionable insights. Its applications are broad and impactful. Key areas include:
- Data Analysis and Reporting: A well-structured data warehouse allows for efficient analysis of large data sets. Business analysts can extract reports quickly, leading to timely decision-making.
- Historical Data Analysis: Organizations can retain historical data over long periods. This enables them to identify trends and patterns that are critical for forecasting and business strategy.
- Enhanced Decision Making: With comprehensive data stored in a warehouse, decision-makers have the analytics tools to evaluate various scenarios. This results in more informed decisions, which can significantly impact the organization’s performance.
- Resource Optimization: A data warehouse can consolidate data from various departments and external sources, eliminating redundancies. This helps organizations to optimize resources effectively and focus on their core competencies.
Overall, the use cases for both MDM and Data Warehousing demonstrate their essential role in modern data management strategies. By integrating these systems, organizations can achieve greater data governance, improved analytics capabilities, and a more profound insight into their operations.
Data Governance and Compliance Considerations
Data governance and compliance considerations play a crucial role in the landscape of Master Data Management (MDM) and data warehousing. These frameworks ensure that data is managed effectively, securely, and in adherence to legal and industry standards. Without proper governance, organizations face risks such as data breaches, legal penalties, and a loss of trust from stakeholders.
In terms of benefits, effective data governance fosters accountability and clarity. It establishes protocols for who controls data, how it is used, and who oversees its integrity. By having defined data policies, companies can improve their data quality, which is vital for informed decision-making. Moreover, compliance with relevant regulations helps organizations avoid costly fines and reputational damage.
Regulatory Frameworks Impacting
Regulatory frameworks impacting MDM include various international and local laws that dictate how data should be handled. One significant framework is the General Data Protection Regulation (GDPR) in Europe, which mandates strict data protection measures. Organizations must ensure that personal data is collected and processed lawfully, transparently, and fairly.
Another important regulation is the Health Insurance Portability and Accountability Act (HIPAA) in the United States. This act requires healthcare organizations to safeguard sensitive patient information. MDM systems must accommodate these regulations to ensure compliance.
Organizations need to stay updated on changes in legislation and trends in data governance. Failure to comply with these regulations can lead to severe penalties.
Compliance Standards for Data Warehousing
Data warehousing also faces several compliance standards that must be met to protect data integrity and privacy. Standards such as ISO/IEC 27001 focus on information security management, ensuring that organizations establish systems to manage confidential data securely.
Additionally, companies should adhere to standards related to data quality, such as the Data Management Association's (DAMA) Framework. This ensures that the data within the warehouse is accurate, consistent, and trustworthy.
In sum, the interplay between data governance, compliance, and both MDM and data warehousing considerably influences the overall success of data management initiatives. Organizations that prioritize these factors can enhance their operational integrity and build a solid foundation for achieving strategic objectives.
Challenges in Master Data Management
Master Data Management (MDM) presents numerous opportunities for organizations to enhance their data accuracy and accessibility. However, these benefits do not come without challenges. Understanding these challenges is crucial for effective implementation and long-term success in data strategy. Identifying issues within MDM allows organizations to mitigate risks, adopt better practices, and ultimately achieve their data management objectives. This discussion will cover two prominent challenges: data silos and integration issues, as well as resistance to change within organizations.
Data Silos and Integration Issues
Data silos arise when departments or systems hold onto data independently, resulting in fragmented views of critical information. This lack of integration can lead to inconsistencies and duplications that undermine the reliability of master data. The consequences of data silos extend beyond operational inefficiencies. They can impact regulatory compliance, data quality, and decision-making processes.
The challenge of data integration encompasses various aspects:
- Technological Compatibility: Different systems often utilize different technologies, making data transfer and synchronization difficult.
- Quality of Data: When data from silos is integrated, variances in data formats and standards may cause inconsistencies.
- Data Governance: Insufficient governance frameworks can lead to a lack of accountability for data accuracy and access rules.
Addressing these integration issues typically demands a multi-faceted approach. Establishing a robust data governance strategy can create a structured environment for data management. Additionally, investing in middleware solutions can help bridge the technological gaps between various systems, facilitating smoother data flows.
"Integrating data across silos is not merely a technical challenge but a strategic imperative for organizations aiming for coherent data management practices."
Resistance to Change in Organizations
The successful implementation of MDM relies heavily on the adaptability of an organization's culture. Resistance to change is a common obstacle in embarking upon MDM initiatives. Employees may fear that new systems will disrupt their workflow or that their roles will be diminished in the MDM process.
Factors contributing to resistance include:
- Lack of Awareness: Employees may not understand the full potential of MDM and how it can benefit their roles and the organization.
- Training Deficiencies: Inadequate training and support can leave employees feeling ill-equipped to navigate new systems, which can reinforce resistance.
- Perceived Threats: Some employees might view MDM as a threat to job security or an increase in workload.
Overcoming this resistance requires effective change management strategies, including:
- Communicating Benefits: Clearly outline how MDM will improve processes and job satisfaction, highlighting positive outcomes.
- Providing Adequate Training: Support staff with the necessary training resources, enabling them to feel confident in using new systems.
- Involving Stakeholders: Encourage participation from various departments in the MDM process to foster a sense of ownership and accountability.
Challenges in Data Warehousing
Data warehousing is an essential part of data management for many organizations. However, it presents various challenges that can impact its effectiveness. Understanding these challenges is crucial for professionals seeking to optimize their data warehouse solutions. Key areas of concern include scalability, performance, and data security.
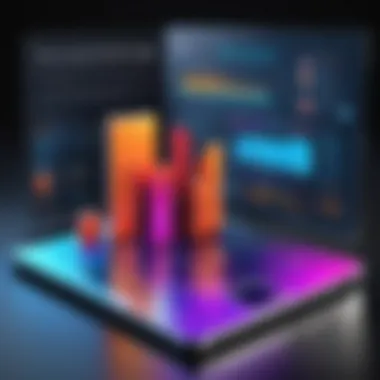
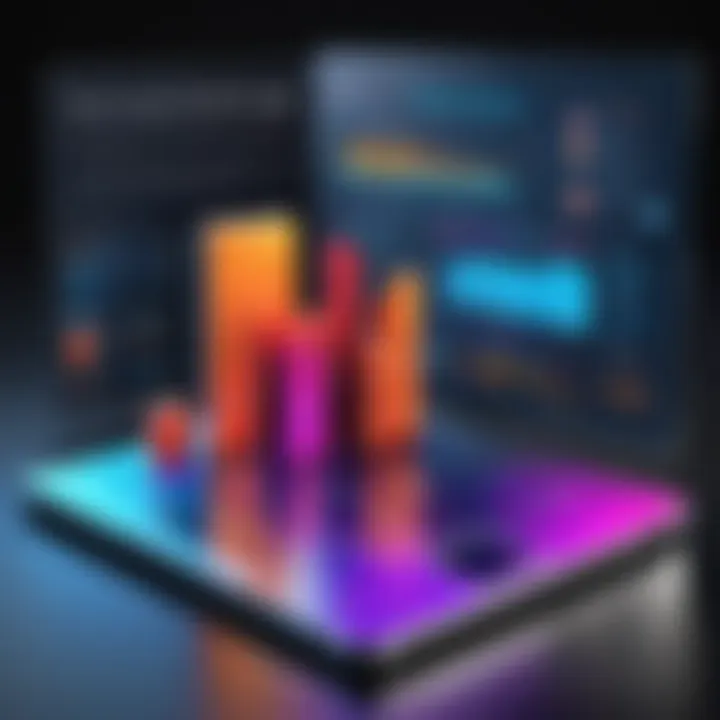
Scalability and Performance Concerns
One major challenge that organizations face with data warehousing is scalability. As businesses grow, their data needs expand, requiring the warehouse to handle increased volumes of information. This can lead to performance issues if the infrastructure is not designed to scale effectively. Performance concerns arise when systems struggle to process queries efficiently, especially during peak usage times.
To address scalability issues, organizations often need to invest in advanced architectures or cloud-based solutions. These options can be more flexible and better suited for handling sudden increases in data. Moreover, companies must carefully monitor their data queries to mitigate performance bottlenecks.
Considerations for scalability may include:
- Assessing current storage capabilities and forecasting future needs
- Implementing optimized data storage techniques
- Exploring hybrid cloud solutions
Data Security Challenges
Data security is another critical challenge in data warehousing. As organizations store sensitive and sometimes regulated data, maintaining security protocols becomes a priority. Data breaches can lead to significant financial and reputational damage, making it essential for companies to adopt comprehensive security measures. This includes safeguarding data both at rest and in transit.
Organizations must consider the following aspects of data security:
- Access Control: Ensuring that only authorized personnel can access sensitive data is vital.
- Encryption: Implementing encryption methods protects data from unauthorized access.
- Regular Audits: Conducting audits to identify vulnerabilities and ensure compliance with regulations, such as GDPR or HIPAA, is necessary.
By addressing these challenges, businesses can enhance their data warehousing strategies, leading to more effective data management.
In summary, tackling scalability and data security challenges is essential for maximizing the effectiveness of data warehousing efforts.
These elements are intertwined with the broader context of data management, emphasizing the need for strategic planning in the design and maintenance of data warehouses.
Tools and Technologies
The consideration of tools and technologies is fundamental when evaluating Master Data Management (MDM) and Data Warehousing. Selecting the right frameworks and solutions can significantly impact the efficiency and effectiveness of data governance practices. In this section, we will dissect the varied offerings in MDM and Data Warehousing, highlighting how each contributes to the goals and objectives of a data strategy.
Solutions and Software
MDM software plays a critical role in the orchestration of data across various business functions. These solutions aim to provide a unified view of critical data entities, such as customers, products, and suppliers. Effective MDM solutions enable organizations to streamline operations and ensure data consistency across systems. Some of the key elements of MDM software include:
- Data Integration: MDM solutions facilitate the integration of data from disparate sources, ensuring that a centralized repository is created. This is vital for maintaining accurate records across an organization.
- Data Governance: Implementing robust governance frameworks helps in managing data lifecycles, ensuring compliance with external regulations.
- Quality Assurance: High-quality data is paramount. MDM tools employ data cleansing, validation, and enrichment processes to enhance data quality.
Various MDM solutions are available in the market. Major players include Informatica, SAP Master Data Governance, and Oracle Master Data Management. Each of these software options offers unique features tailored to different industries and organizational needs.
"The implementation of MDM can effectively reduce the costs associated with data inaccuracies, ultimately enhancing customer satisfaction and operational efficiency."
Data Warehouse Platforms
Data Warehouse platforms serve as the backbone of business intelligence and analytics, providing organizations with the ability to analyze vast amounts of data for strategic decision-making. These platforms are specifically designed to facilitate complex queries, integrate data from various operational systems, and support reporting needs. Important factors to consider when evaluating data warehouse solutions include:
- Scalability: Organizations generate increasing volumes of data daily. A scalable data warehouse can accommodate growth without a decline in performance.
- Data Modeling: Proper data modeling capabilities ensure that data is organized logically. Solutions like Microsoft SQL Server and Amazon Redshift offer advanced modeling features that suit various analytic needs.
- Integration with BI Tools: Effective data warehousing solutions should seamlessly integrate with business intelligence tools. This integration enhances reporting and visual data interpretation, allowing users to derive actionable insights.
Popular data warehouse platforms include Snowflake, IBM Db2 Warehouse, and Google BigQuery. Each platform comes with its distinct advantages, and the choice should align with your organization’s specific analytical requirements and technology landscape.
Future Trends in Data Management
As the landscape of data management transforms, future trends play a crucial role in how organizations approach Master Data Management (MDM) and Data Warehousing. Recognizing these trends offers businesses the opportunity to enhance their data capabilities, improve operational efficiency, and maintain a competitive edge. Keeping abreast of future trends is important because it allows decision-makers to strategically invest in technologies and methodologies suited for evolving requirements.
Integration of AI and Machine Learning
The integration of artificial intelligence (AI) and machine learning into data management is a significant trend that is reshaping how data is processed and analyzed. AI technologies enhance data quality by automating data cleansing and validation processes. This leads to higher accuracy in MDM efforts, as AI systems can identify patterns in data anomalies that may go unnoticed by humans.
Machine learning algorithms can also optimize data warehousing by predicting storage needs and improving performance. For instance, these algorithms can analyze usage patterns and suggest data models that enable faster query response times. Businesses leveraging such technologies can expect better insights and efficiencies.
- Machine learning aids in predictive analytics, allowing organizations to forecast trends based on historical data.
- AI can personalize data solutions, aligning them with specific business requirements.
- Enhanced automation reduces manual efforts, freeing up resources for more strategic initiatives.
"AI and machine learning not only enhance data quality but also enable smarter data-driven decision-making."
Cloud-based Data Solutions
The adoption of cloud-based data solutions marks another pivotal trend in the realm of data management. Organizations are increasingly migrating their data systems to the cloud to take advantage of flexibility, scalability, and cost-effectiveness. These solutions enable businesses to handle vast amounts of data without the need for extensive on-premises infrastructure.
Cloud platforms can integrate seamlessly with both MDM and data warehousing systems, facilitating real-time data access and collaboration across multiple departments. This agility is essential for organizations aiming to stay responsive to market changes and operational demands.
- Benefits of Cloud-based Solutions:
- Considerations for Implementation:
- Scalability: Easily adjust resources as data needs grow.
- Cost Savings: Reduce expenditure on hardware and maintenance.
- Data Security: Ensure robust security protocols are in place to protect sensitive information.
- Compliance: Adhere to regulations to avoid potential legal issues.
Organizations that embrace cloud strategies can achieve more streamlined workflows and enhanced collaboration, ultimately leading to improved data governance and analytics capabilities.
End
The conclusion serves a significant role in this article by synthesizing the insights presented in the prior sections. It enables readers to grasp the core distinctions between Master Data Management (MDM) and Data Warehousing. This understanding is not merely academic; it has real-world implications for professionals seeking informed strategies in data management.
Recap of Key Differences
Master Data Management and Data Warehousing, while interconnected, serve different purposes:
- Purpose: MDM focuses on maintaining accurate and consistent master data across an organization, while Data Warehousing is designed for storing vast volumes of historical data for analysis.
- Data Management: MDM emphasizes data quality and governance, ensuring that the data is reliable and up to date. Conversely, Data Warehousing prioritizes data retrieval, enabling users to analyze data efficiently.
- Technology: MDM may utilize various tools to manage data quality, such as Talend or Informatica. Data Warehousing often employs platforms like Snowflake or Amazon Redshift to handle large datasets and perform complex queries.
This differentiation is foundational in understanding how each component fits within an organization's overall data strategy.
Final Thoughts on Choosing Between and Data Warehousing
Deciding between MDM and Data Warehousing hinges on an organization’s specific needs. If an enterprise grapples with inconsistent data across departments, implementing MDM should be a priority. However, if the goal is to analyze historical data to inform business decisions, investing in a Data Warehouse is advisable.
Organizations may even benefit from using both. MDM can enhance the quality of data flowing into a Data Warehouse, resulting in more accurate reports and analytics.
In summary, understanding the roles and functionalities of MDM and Data Warehousing is critical for tech enthusiasts and decision-makers alike. By making informed choices, organizations can effectively govern their data and leverage it for strategic advantage.