Mastering Data Analysis: A Comprehensive Guide for Businesses
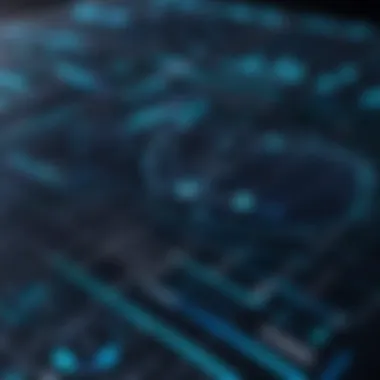
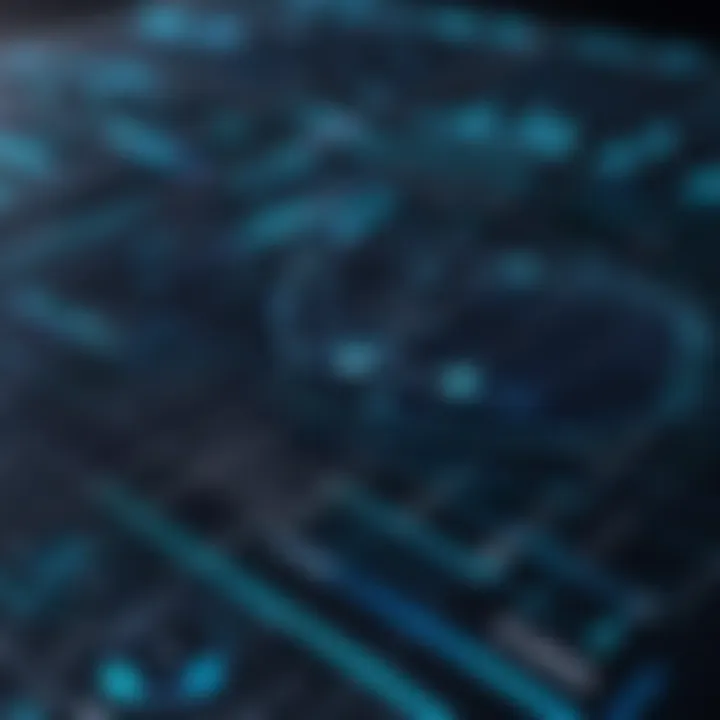
Technological Research Overview
In this section, we delve into recent technological innovations that are transforming the landscape of businesses globally. Understanding the impact of these innovations on business operations is crucial for staying ahead in today's competitive market. Looking towards the future, we analyze emerging technological trends that are set to redefine the way businesses operate, emphasizing the need for continuous adaptation and innovation.
Data Analytics in Business
The importance of data analytics for businesses cannot be overstated. We explore the significance of harnessing data for insights that drive strategic decision-making. Discussing various tools available for data analysis, we guide businesses on selecting the most appropriate solutions to suit their unique needs. Through case studies highlighting successful data-driven decisions, we demonstrate the real-world impact of effective data analytics in shaping business outcomes.
Cybersecurity Insights
Within the realm of cybersecurity, we conduct a comprehensive threat landscape analysis to identify potential risks and vulnerabilities facing businesses today. By emphasizing best practices in cybersecurity, we equip organizations with the knowledge needed to mitigate threats effectively. Exploring regulatory compliance requirements in cybersecurity, we navigate the intricate regulatory landscape to ensure businesses adhere to necessary protocols and standards.
Artificial Intelligence Applications
Artificial Intelligence (AI) is revolutionizing business automation, enhancing operational efficiency, and optimizing processes. We dive into the realm of AI algorithms and applications, shedding light on how businesses can leverage this technology for sustainable growth. Moreover, we touch upon the ethical considerations surrounding AI, emphasizing the need for responsible and transparent AI deployment in business settings.
Industry-Specific Research
Delving into industry-specific research, we explore technological advancements within the finance sector. By examining how technology is reshaping financial services, we offer insights into the future of finance. Transitioning to healthcare, we uncover the latest technological solutions driving advancements in the healthcare industry. Additionally, we analyze tech solutions tailored for the retail sector, outlining innovative approaches that businesses can adopt to enhance customer experiences and drive growth.
Introduction
In the fast-paced landscape of modern business operations, data analysis stands out as a pivotal tool for informed decision-making. This article delves deep into the intricate process of data analysis planning and execution within the business realm. By providing a detailed overview of the strategic steps involved in formulating an effective data analysis plan, it equips enterprises with the necessary knowledge and tools to leverage data effectively in today's digital-centric world.
Understanding the Importance of Data Analysis
Benefits of Data Analysis
Data analysis plays a significant role as it allows businesses to extract valuable insights from the vast pool of data at their disposal. The ability to identify trends, patterns, and anomalies empowers organizations to make data-driven decisions, leading to operational efficiencies and strategic advancements. Through data analysis, businesses can enhance their understanding of consumer behavior patterns, optimize marketing strategies, and tailor products and services to meet customer needs effectively. The comprehensive nature of data analysis ensures that businesses gain a competitive edge in the market by leveraging actionable insights to drive growth and innovation.
Impact on Business Growth
The impact of data analysis on business growth cannot be overstated. By harnessing the power of data analysis, companies can uncover hidden opportunities, mitigate risks, and capitalize on market trends. Data-driven decision-making not only enhances operational efficiency but also fosters a culture of innovation and adaptability within organizations. Through insightful data analysis, enterprises can optimize their processes, identify new revenue streams, and enhance customer experiences. However, it is essential to note that while data analysis offers a plethora of benefits, it also challenges organizations to effectively manage and interpret the vast amount of data at their disposal.
Scope of the Article
Overview of Key Sections
This article offers a detailed exploration of the key components essential for mastering data analysis in a business context. From defining key objectives to identifying data sources, and from data collection strategies to data preparation phases, each section provides crucial insights into the strategic steps involved. By examining data analysis strategies, interpreting data insights, and drawing actionable conclusions, this guide aims to equip professionals, entrepreneurs, decision-makers, and tech enthusiasts with a robust understanding of data analysis and its significance in driving business success.
Foundation of Data Analysis
In this segment of our comprehensive guide on data analysis for businesses, we delve into the foundational aspects that form the backbone of effective data analysis practices. Understanding the importance of establishing a strong foundation sets the stage for successful data-driven decision-making processes. Delving into the bedrock of data analysis not only provides a solid framework but also ensures that businesses can extract meaningful insights from their datasets efficiently. By defining key objectives, businesses can lay the groundwork for a structured and purposeful data analysis approach.
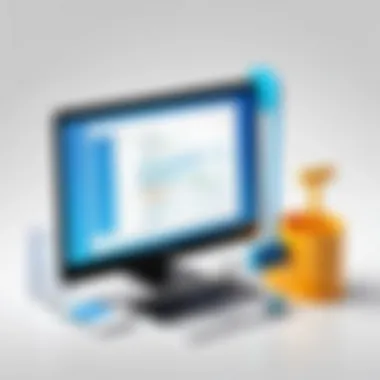
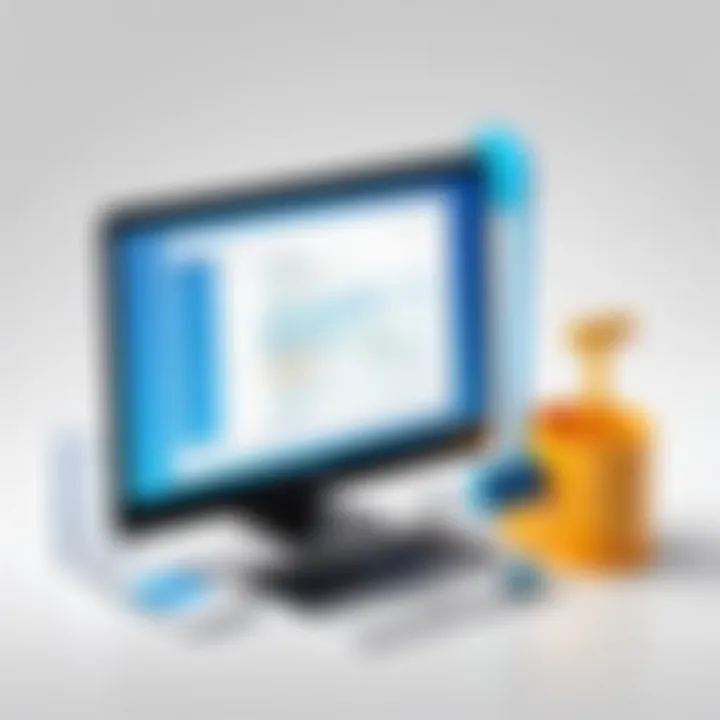
Defining Key Objectives
Setting Clear Goals
When it comes to setting clear goals in data analysis, fine-tuning the objectives of the analytical process is crucial for overall success. It involves outlining specific targets that need to be achieved through data analysis efforts. By setting clear goals, businesses can focus their resources and efforts on areas that align with their strategic priorities and desired outcomes. The key characteristic of setting clear goals lies in providing a roadmap for data analysis, guiding analysts on where to direct their attention and efforts. This approach is a popular choice in this article as it emphasizes the significance of clarity and precision in the data analysis process, enhancing the likelihood of actionable insights and informed decision-making. The unique feature of setting clear goals is its ability to align analytical efforts with organizational objectives, ensuring that data analysis outputs directly contribute to business goals. While setting clear goals offers numerous benefits such as improved focus and measurable results, it may also carry the disadvantage of overlooking potentially valuable insights that may not align directly with pre-defined goals.
Establishing Metrics for Success
In the realm of data analysis, establishing metrics for success is paramount for evaluating the effectiveness and efficiency of analytical processes. Metrics serve as quantifiable measures that indicate progress towards predefined goals and objectives. By defining specific metrics for success, businesses can track their performance and determine the impact of data analysis activities on overall outcomes. The key characteristic of establishing metrics for success is its role in providing tangible indicators of progress and success in data analysis initiatives. This approach is a popular choice in this article as it underscores the importance of measuring the value and impact of data-driven insights. The unique feature of establishing metrics for success is its ability to foster a data-driven culture within organizations, emphasizing the importance of accountability and results-oriented practices. While this approach offers advantages such as enhanced accountability and performance tracking, it may also present challenges in determining the most relevant metrics for complex analytical processes.
Identifying Data Sources
Internal Data
Internal data plays a significant role in influencing strategic decisions within businesses. This section explores the specific contributions of internal data to the overarching goal of data analysis. The key characteristic of internal data lies in its direct relevance and accessibility to organizations, offering a rich source of information derived from internal systems and processes. This popular choice in our article emphasizes the value of leveraging existing internal data repositories for analytical purposes, enabling businesses to extract valuable insights and drive informed decision-making. The unique feature of internal data is its inherent familiarity to organizations, allowing for deep insights into operational trends and patterns. While internal data offers advantages such as detailed insights and contextual understanding, it may present challenges in terms of data quality and integration complexities.
External Data
On the other hand, external data brings supplementary perspectives and insights that complement internal data sources in the data analysis process. This section explores the unique contributions of external data in enhancing the breadth and depth of analytical outcomes. The key characteristic of external data is its ability to provide diverse and novel information sources from external environments, enriching the analytical landscape for businesses. This popular choice in our article highlights the importance of considering external data sources to gain a holistic view of market trends, customer behavior, and competitive landscapes. The unique feature of external data lies in its capacity to unveil external market dynamics and emerging trends that may impact strategic decision-making. While external data offers advantages such as broadening analytical horizons and enhancing predictive capabilities, it may pose challenges related to data privacy regulations and reliability issues.
Data Collection Strategies
Automation Techniques
Automation techniques revolutionize the data collection process by introducing efficiency and scalability to data analysis endeavors. This section investigates the specific role of automation techniques in streamlining data gathering processes. The key characteristic of automation techniques is their ability to reduce manual intervention and accelerate data collection tasks, leading to increased productivity and accuracy. This popular choice in our article underscores the benefits of harnessing automation technologies to handle large volumes of data swiftly and systematically. The unique feature of automation techniques lies in their capacity to standardize data collection procedures and minimize errors associated with manual processes. While automation techniques offer advantages such as time savings and consistency in data acquisition, they may present challenges in adapting to evolving data sources and dynamic data formats.
Manual Collection Methods
Despite the advancements in automation, manual collection methods retain their significance in certain data analysis contexts. This part explores the nuanced contributions of manual collection methods to the overall data acquisition strategy. The key characteristic of manual collection methods is their flexibility and adaptability in gathering data from diverse sources, especially those that require human interpretation or qualitative assessment. This popular choice in our article highlights the relevance of manual collection methods in scenarios where human judgment and contextual understanding are paramount. The unique feature of manual collection methods is their suitability for capturing nuanced data points that may be overlooked by automated processes, enriching the depth of analysis and insights. While manual collection methods offer advantages such as personalized data extraction and nuanced insights, they may pose challenges in terms of time-consuming processes and potential biases introduced by human involvement.
Data Preparation Phase
In the realm of data analysis within businesses, the Data Preparation Phase stands as a crucial stage in the process. This phase is where raw data is refined and shaped into a format ready for analysis. Data preparation ensures that the data collected is accurate, complete, and relevant to the analysis objectives at hand. It involves various steps like data cleaning, transformation, and feature selection to ensure the integrity and quality of the data being used for analysis. By focusing on the Data Preparation Phase, businesses can guarantee that their subsequent analysis is based on a solid foundation of reliable and well-prepared data.
Data Cleaning Process
Handling Missing Values
Handling Missing Values is a fundamental aspect of the Data Cleaning Process. When dealing with real-world data, missing values are a common occurrence that can impact the accuracy and reliability of analysis results. By addressing missing values effectively, businesses can avoid skewed insights and inaccurate conclusions. The key characteristic of Handling Missing Values lies in its ability to impute or remove missing data points, thereby ensuring that the dataset remains complete and usable for analysis. This approach is beneficial for businesses as it helps maintain data integrity and reliability in the overall analysis process.
Removing Outliers
Another crucial step in the Data Cleaning Process is Removing Outliers. Outliers are data points that significantly differ from other observations in a dataset, potentially skewing analysis results. Removing outliers helps in ensuring that the analysis is based on typical data patterns, leading to more accurate and reliable insights. The key characteristic of Removing Outliers is its impact on the distribution and statistical measures within the dataset. This approach is beneficial for businesses as it contributes to the robustness and accuracy of the data used for analysis.
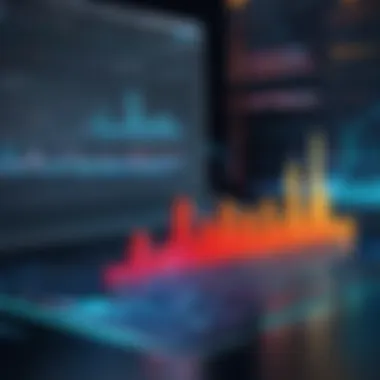
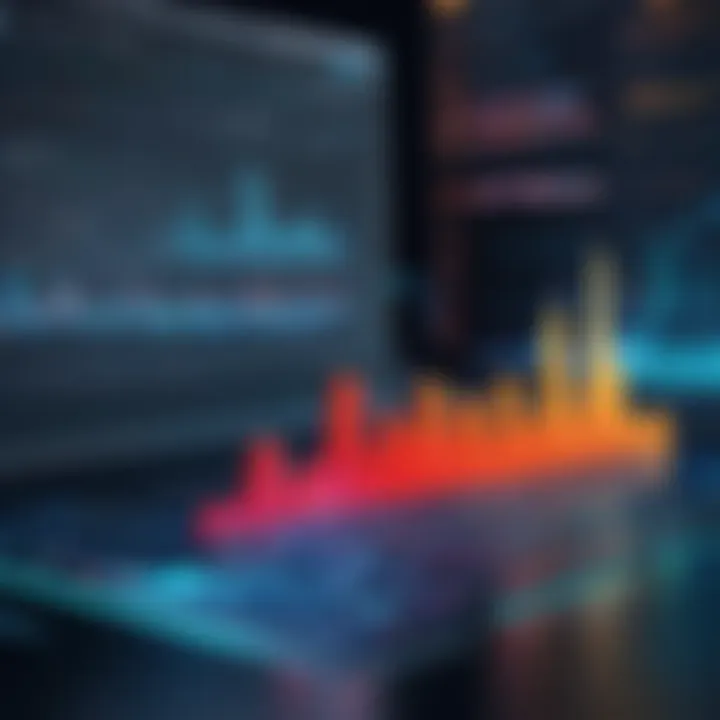
Data Transformation Techniques
Normalization
Normalization is a vital aspect of data transformation that plays a significant role in standardizing the scale and distribution of data. By normalizing data, businesses can remove biases introduced by varying scales and facilitate better comparison and analysis across different features. The key characteristic of normalization lies in its ability to bring consistency to data, making it easier to interpret and analyze. This technique is beneficial for businesses as it enhances the accuracy and efficiency of statistical models and machine learning algorithms.
Encoding Categorical Variables
Encoding Categorical Variables is another essential data transformation technique that involves representing categorical data in a numerical format. This transformation is crucial as many machine learning algorithms require numerical input. The key characteristic of encoding categorical variables is its conversion of qualitative data into a quantitative form, making it ready for analysis. This technique is advantageous for businesses as it enables the inclusion of categorical data in predictive models and statistical analysis, leading to more comprehensive insights.
Feature Selection Methods
Filter Methods
Filter Methods are critical in the feature selection process, where irrelevant or redundant features are filtered out before model training. These methods assess feature importance based on statistical measures like correlation or mutual information, aiding in selecting the most informative features for analysis. The key characteristic of filter methods is their simplicity and computational efficiency in handling high-dimensional data. This approach benefits businesses by improving model performance and interpretability by focusing on the most relevant features.
Wrapper Methods
Wrapper Methods are another set of feature selection techniques that involve selecting features based on model performance. These methods evaluate feature subsets using a chosen model, iterating through combinations to identify the most predictive set. The key characteristic of wrapper methods is their ability to consider feature interactions and nonlinear relationships, leading to more accurate feature selection. This technique is advantageous for businesses as it optimizes feature subsets for specific modeling algorithms, enhancing the accuracy and robustness of predictive models.
Data Analysis Strategies
Data Analysis Strategies are fundamental in the realm of data analysis within businesses. In this article, we emphasize the critical role that Data Analysis Strategies play in deriving meaningful insights and facilitating informed decision-making. By employing effective Data Analysis Strategies, businesses can streamline operations, enhance efficiency, and gain a competitive edge in today's data-driven landscape. This section delves into the specific elements, benefits, and considerations that characterize successful Data Analysis Strategies, guiding professionals on leveraging data effectively to drive actionable outcomes and strategic growth.
Exploratory Data Analysis
Descriptive Statistics
Descriptive Statistics form a pivotal aspect of Exploratory Data Analysis, offering essential insights into the distribution, central tendency, and dispersion of data. In the context of this article, Descriptive Statistics serve as a foundational tool for summarizing and interpreting complex datasets, allowing analysts to extract key patterns and trends. The significance of Descriptive Statistics lies in its ability to condense large volumes of data into comprehensible summaries, aiding decision-makers in drawing meaningful conclusions with statistical significance. While Descriptive Statistics present a structured approach to data summarization, their limitations may include oversimplification of intricate data relationships and an over-reliance on aggregate measures, necessitating cautious interpretation within the broader data analysis framework.
Data Visualization Techniques
Data Visualization Techniques play a vital role in transforming raw data into visually engaging representations that facilitate intuitive understanding and insightful analysis. Within this article, Data Visualization Techniques are highlighted for their capacity to elucidate complex patterns, relationships, and anomalies hidden within datasets, empowering users to make data-driven decisions with clarity and precision. The key characteristic of Data Visualization Techniques lies in their ability to convey information effectively through graphical elements, enhancing cognition and interpretation while maximizing data value. However, challenges such as potential misinterpretation of visualizations and information overload underscore the importance of selecting appropriate visualization methods aligned with the specific analytical objectives, ensuring accurate and actionable insights are derived.
Statistical Modeling
Hypothesis Testing
Hypothesis Testing serves as a cornerstone of Statistical Modeling, enabling analysts to evaluate and validate hypotheses based on sample data to make informed inferences. In the context of this article, Hypothesis Testing plays a crucial role in substantiating analytical findings, drawing conclusions, and supporting decision-making processes with statistical rigor. The key characteristic of Hypothesis Testing lies in its ability to assess the significance of observed results, guiding practitioners in accepting or rejecting null hypotheses to navigate uncertainty and derive conclusive insights from data. While Hypothesis Testing offers a robust framework for statistical inference, its limitations may include sensitivity to sample size, assumptions of data normality, and potential errors in interpretation, necessitating careful consideration and validation of results within the analytical context.
Regression Analysis
Regression Analysis serves as a powerful tool in Statistical Modeling, allowing analysts to explore relationships between variables, predict outcomes, and uncover causal mechanisms within datasets. Within this article, Regression Analysis is underscored for its capacity to model complex phenomena, optimize predictions, and inform decision-making processes in various business domains. The key characteristic of Regression Analysis lies in its ability to quantify relationships, identify significant factors, and assess the impact of predictor variables on the outcome of interest, fostering data-driven insights with practical implications. However, challenges such as model overfitting, multicollinearity, and assumptions of linear relationships pose considerations for analysts, necessitating careful model selection and validation to ensure robust and reliable results within the analytical framework.
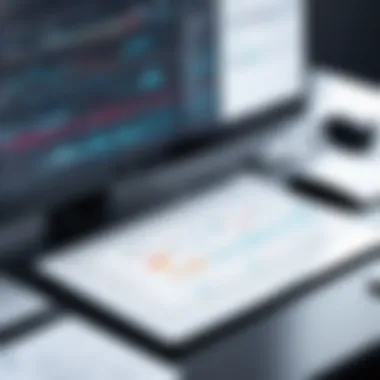
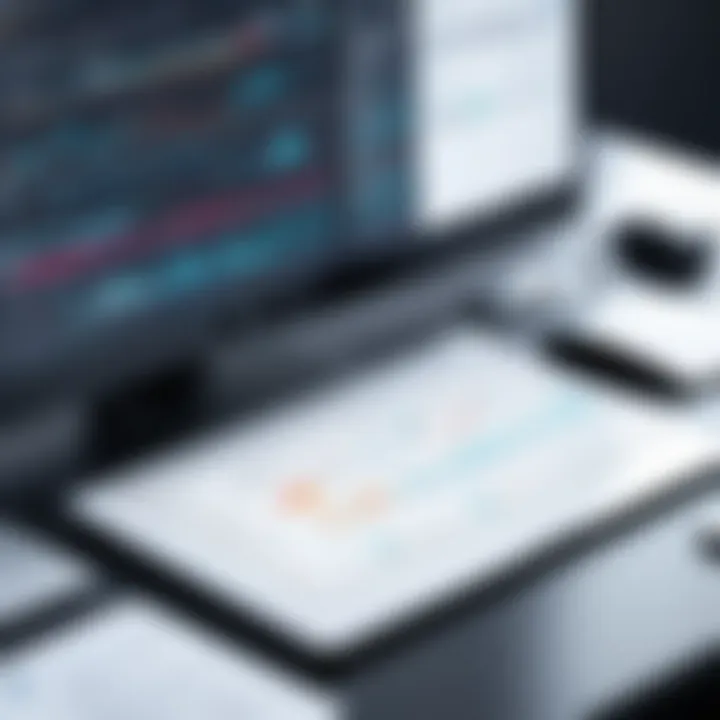
Machine Learning Applications
Classification Algorithms
Classification Algorithms play a pivotal role in Machine Learning Applications, enabling automated categorization of data into distinct classes or groups based on input features and patterns. In the context of this article, Classification Algorithms are highlighted for their ability to predict outcomes, classify entities, and optimize decision-making processes through algorithmic learning and pattern recognition. The key characteristic of Classification Algorithms lies in their versatility and adaptability to varying datasets and classification tasks, providing scalable solutions for data classification and predictive modeling. However, considerations such as algorithm bias, class imbalance, and interpretability challenges underscore the importance of robust model evaluation and validation techniques to ensure the reliability and fairness of classification outcomes within the analytical framework.
Clustering Techniques
Clustering Techniques serve as essential tools in Machine Learning Applications, facilitating unsupervised learning and pattern discovery by grouping data points based on similarity and proximity metrics. Within this article, Clustering Techniques are emphasized for their capacity to reveal hidden patterns, segment data intuitively, and uncover latent structures within datasets, enabling analysts to explore data relationships and identify meaningful clusters for further analysis. The key characteristic of Clustering Techniques lies in their flexibility and scalability across diverse data types and clustering algorithms, offering insights into data organization and segmentation for exploratory analysis and decision support. However, challenges such as cluster validation, selection of optimal clustering algorithms, and interpretability of cluster results present considerations for analysts, necessitating a systematic approach to clustering implementation and validation to enhance the robustness and interpretability of clustering outcomes within the analytical context.
Predictive Analytics
Time Series Forecasting
Time Series Forecasting plays a significant role in Predictive Analytics, guiding analysts in predicting future trends, patterns, and behaviors based on historical time series data. In the context of this article, Time Series Forecasting is highlighted for its utility in forecasting future values, detecting seasonality and trends, and optimizing predictive models for informed decision-making. The key characteristic of Time Series Forecasting lies in its ability to capture temporal dependencies, model time-varying patterns, and generate reliable forecasts, enabling organizations to anticipate future outcomes and mitigate risks effectively. However, challenges such as data stationarity, model overfitting, and forecast uncertainty underscore the importance of robust time series analysis, model selection, and performance evaluation to ensure accurate and actionable forecasting outcomes within the predictive analytics framework.
Predictive Modeling
Predictive Modeling forms a cornerstone of Predictive Analytics, enabling analysts to construct predictive models based on historical data to forecast future outcomes and trends. In this article, Predictive Modeling is underscored for its capacity to identify predictive patterns, optimize decision-making processes, and drive strategic insights through data-driven predictions. The key characteristic of Predictive Modeling lies in its ability to generalize from past data, predict future outcomes, and optimize model performance through iterative learning and model refinement, empowering businesses to anticipate trends, capture opportunities, and mitigate risks proactively. However, challenges such as model bias, data quality issues, and model interpretability pose considerations for analysts, necessitating rigorous model validation, interpretive techniques, and performance evaluation to enhance the reliability and accuracy of predictive models within the analytical framework.
Interpreting Data Insights
The subsection on Drawing Conclusions within the Interpreting Data Insights section further elaborates on the process of synthesizing information to extract valuable insights. Two key components are addressed within this subsection namely identifying patterns and inference and analysis. Each of these components plays a pivotal role in the overarching goal of making data-driven decisions to enhance business performance.
Drawing Conclusions
Identifying Patterns
The identification of patterns is a fundamental aspect of data analysis as it involves recognizing recurring trends or relationships within datasets. By discerning patterns, businesses can uncover key associations that may not be apparent on the surface. This section explores how identifying patterns contributes to the overall objective of extracting actionable insights from data. A detailed analysis of patterns enables businesses to make informed decisions based on historical trends and predictive patterns, thereby optimizing strategies and mitigating risks. The unique feature of identifying patterns lies in its ability to reveal hidden correlations and anomalies that can significantly impact business outcomes. However, it's essential to recognize the limitations of pattern identification, such as the potential for biased interpretations or oversimplification of complex data sets.
Inference and Analysis
Inference and analysis focus on the process of drawing conclusions and making informed predictions based on available data. This subsection highlights how inference and analysis contribute to the overall goal of leveraging data for strategic decision-making. By applying statistical inference techniques and robust analytical methods, businesses can uncover underlying insights that drive performance improvements and innovation. The unique feature of inference and analysis lies in its ability to provide a structured framework for synthesizing data points into actionable recommendations. However, it's vital to acknowledge the potential pitfalls of inference and analysis, such as the risk of overgeneralization or misinterpretation of statistical outcomes.
Decision-Making Process
Within the Decision-Making Process section, the focus shifts towards the critical steps involved in translating data insights into actionable strategies. Two key elements are explored in detail - actionable recommendations and strategic planning - both essential components in the decision-making continuum.
Conclusion
Key Takeaways
Significance of Data Analysis
The exploration of the "Significance of Data Analysis" within this comprehensive guide sheds light on the critical role data plays in shaping business landscapes. Highlighting the essence of extracting meaningful insights from raw data, this section underscores the transformative power data analysis holds for driving informed decision-making. By harnessing the potential of data, businesses gain a competitive edge, enabling them to anticipate market trends, optimize operational efficiencies, and enhance customer experiences. The key characteristic of thorough data analysis lies in its ability to transform unprocessed information into actionable strategies, propelling organizational success in the digital era. While data analysis presents immense advantages in enhancing business intelligence, it also requires a keen eye for detail and a robust analytical framework to avoid misinterpretation of results, ensuring data accuracy and relevance throughout the decision-making process.
Future Trends
Delving into the realm of "Future Trends" offers a glimpse into the evolving landscape of data analysis and its implications for businesses. Emphasizing the ongoing advancements in machine learning, artificial intelligence, and predictive analytics, this section underscores the importance of staying abreast of emerging technologies to maintain competitive relevance in the market. The key characteristic of future trends lies in their potential to revolutionize data analysis methodologies, offering enhanced predictive capabilities, real-time insights, and personalized recommendations tailored to individual business needs. While embracing future trends can unlock endless opportunities for innovation and growth, it also necessitates a proactive approach to adapting to changing data landscapes, fostering a culture of continuous learning and adaptation to leverage the full potential of evolving technologies.