Selective Data Transition: Future of Data Management
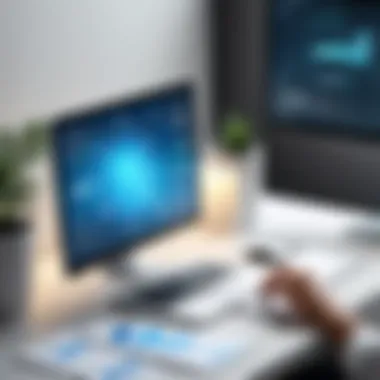
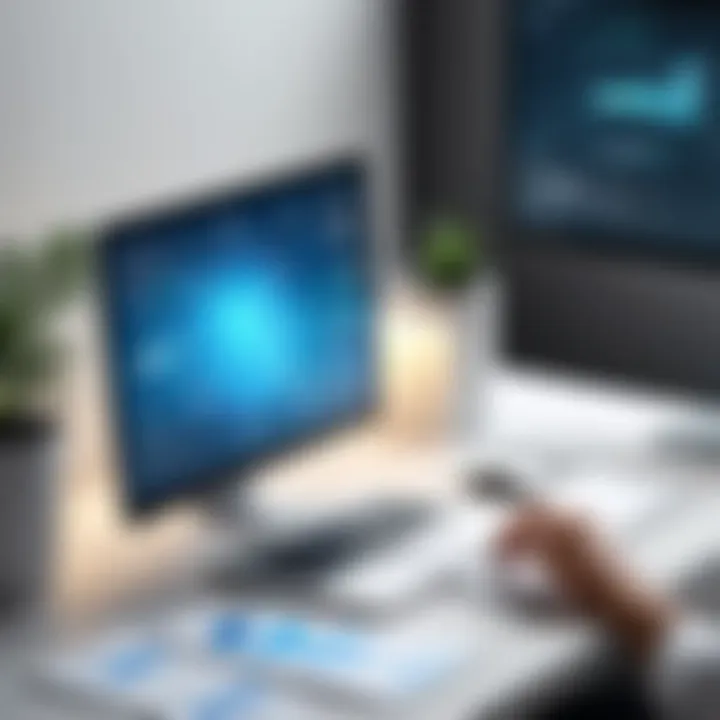
Intro
In the realm of data management, the landscape is shifting under our very feet. As the digital age continues to blossom, each tick of the clock sends more bytes and bits soaring through cyberspace, challenging businesses to adapt swiftly. In comes the concept of selective data transitionāa strategy thatās both timely and transformative. This approach promises not just to optimize how data is handled but also to enhance the decision-making process, allowing organizations to remain agile in a market thatās forever in flux.
Selective data transition refers to the careful process of sorting and filtering relevant information while leaving behind anything that doesn't serve a purpose. In an era when "less is more" often rings true, understanding how to navigate this process can make a significant difference in operational efficiency and strategic foresight. By embracing selective data methods, organizations can sift through their massive data reserves, retaining only whatās vital and actionable.
The significance of this practice can't be overstated, especially as data-related challenges escalate. From ensuring compliance with regulations to protecting data from cybersecurity threats, businesses must be judicious about what data they transition and how they do so. As we delve deeper into this article, we shall explore key areas including the technological innovations that facilitate this transition, the impact on business operations, and emerging trends that will shape the future of data management.
Understanding Selective Data Transition
Data management is an intricate tapestry, and selective data transition is one of its most critical threads. In a world awash with data, the ability to sift through, retain, and effectively utilize valuable information is becoming a defining factor for success in various industries. This section lays the groundwork for understanding selective data transition, dissecting its definition and historic evolution.
Definition and Importance
Selective data transition refers to the process of filtering and moving only relevant data from one system to another, rather than attempting to transfer entire datasets. This concept is vital because it serves as a countermeasure to data overload, a common plight in today's data-driven world. Businesses today deal with vast amounts of information, and sifting through it all can resemble finding a needle in a haystack.
The importance of selective data transition can be boiled down to several key benefits:
- Cost Efficiency: Transferring only the necessary data saves time and reduces costs associated with data storage and processing.
- Enhanced Decision-Making: By focusing on relevant data, decision-makers can access critical insights rapidly, leading to informed choices.
- Improved Data Quality: It allows organizations to maintain high-quality datasets by discarding outdated or irrelevant information.
- Regulatory Compliance: With data protection regulations becoming stricter, selectively transitioning data helps ensure compliance by only retaining what's necessary.
In essence, understanding selective data transition equips professionals with the knowledge to streamline their data processes while maximizing value extraction from their datasets.
Historical Context
To truly grasp the importance of selective data transition, one should reflect on its historical underpinnings. Data management has evolved significantly over the past few decades. In the early days, data was mainly stored in physical formats or in rudimentary databases. It was pretty much a simpler time, but as businesses adopted technology, the volume of data skyrocketed.
With the advent of the internet and the explosion of mobile technology, the landscape changed drastically. Organizations began collecting data at an unprecedented rate. The challenge, however, was that not all data collected was pertinent. Companies soon found themselves drowning in information, much of it irrelevant to their core operations.
As a response to this growing concern, selective data transition emerged as a strategy to mitigate data overload. In the mid-2000s, with the rise of big data analytics, businesses recognized that managing data resourcefully, rather than uncritically archiving every piece of information, was essential to remain competitive in the market.
This historical trajectory illustrates that selective data transition is not merely a trend; it is a necessary evolution in data management, rooted in the need for efficiency, relevance, and adaptability in a hyper-connected world. Understanding this context is essential for professionals aiming to optimize their data strategies moving forward.
Rationale Behind Selective Data Transition
In today's data-driven landscape, the rationale behind selective data transition has never been more critical. As organizations accumulate vast amounts of information, the challenge of effectively managing this data becomes paramount. Selective data transition is not just about moving data from one storage location to another; it embodies a strategic approach that aims to optimize data utility while minimizing unnecessary clutter. With the exponential growth of data, sifting through it to identify relevant parts ensures businesses can focus on what truly matters, making vital decisions based on precise insights.
Data Overload and its Consequences
The phrase "data overload" has become common in conversations on data management. But what does it really mean? Simply put, it refers to the overwhelming amount of data that companies face today. Think of a librarian who is supposed to find a specific book in a library filled to the brim with thousands of unread titles. When faced with such a deluge, the chance of retrieving the right information dwindles.
A few key consequences of data overload include:
- Reduced Efficiency: Employees may find themselves wasting time on irrelevant data, which can be particularly frustrating. Imagine trying to navigate a treacherous maze, only to find the exit blocked by heaps of paperwork.
- Poor Decision-Making: When decision-makers are inundated with excess data, extracting actionable insights becomes like finding a needle in a haystack. This can lead to choices that lack solid grounding.
- Increased Costs: Data storage and management solutions can become prohibitively expensive as organizations seek to store everything without a clear understanding of what is necessary. Think of it as paying for a second house simply to store old newspapers.
To combat these issues, selective data transition acts as a beacon guiding companies toward efficiency, clarity, and purpose in their data handling practices.
Strategic Advantages
Embracing selective data transition offers several strategic advantages that can significantly shape an organizationās operational framework. Here are some insights worth noting:
- Enhanced Focus on Core Objectives: By identifying and retaining only essential data, businesses can laser-focus on their objectives. This process enables teams to get results faster without wandering off track.
- Cost-Effectiveness: Reducing the amount of data stored can lead to substantial savings in storage costs, as companies avoid paying for unnecessary resources. Itās similar to trimming the fat off a budget; the leaner it is, the better it performs.
- Improved Data Quality: Beyond just reducing volume, selective data transition often enhances the quality of the data retained. With only relevant information in hand, organizations increase their chances of making informed decisions.
- Streamlined Compliance and Governance: Data regulations require businesses to manage sensitive information carefully. Selective data transition helps organizations maintain compliance by simplifying data governance. Consider it a clean-up crew that ensures everything is in order and accounted for.
In summary, the rationale behind selective data transition is grounded in the necessity to manage data strategically amidst challenges like overload and inefficiencies. Adaptability in the face of growing data volumes presents opportunities that savvy organizations can seize for long-term benefits.
Challenges in Implementing Selective Data Transition
Implementing selective data transition isnāt just a walk in the park. It's like steering a ship through stormy seas. There are several challenges that organizations face, which can significantly hinder the smooth transition of data management processes. Understanding these hurdles is crucial for businesses aiming to enhance their data handling strategies while reaping the benefits of efficiency and improved decision-making.
Data Integrity Issues
One of the primary challenges in selective data transition revolves around maintaining data integrity. Data integrity refers to the accuracy, consistency, and reliability of data throughout its lifecycle. When businesses filter out certain data for transition, the risk of losing valuable context or creating inconsistencies in the remaining data increases. If incorrect data slips through the cracks, it can lead to erroneous conclusions that misguide decision-making efforts.
To mitigate these data integrity issues, organizations are advised to implement rigorous validation checks during the transition process. This often involves:
- Regular audits of the data set to ensure rules are followed.
- Implementing checks and balances to track data changes.
- Employing data cleansing techniques to ensure only high-quality data remains post-transition.
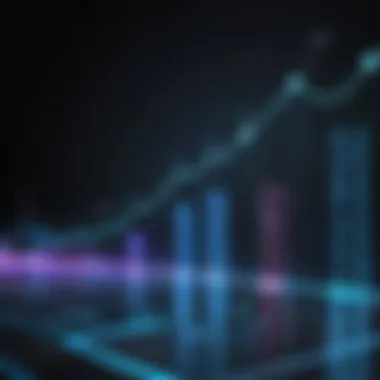
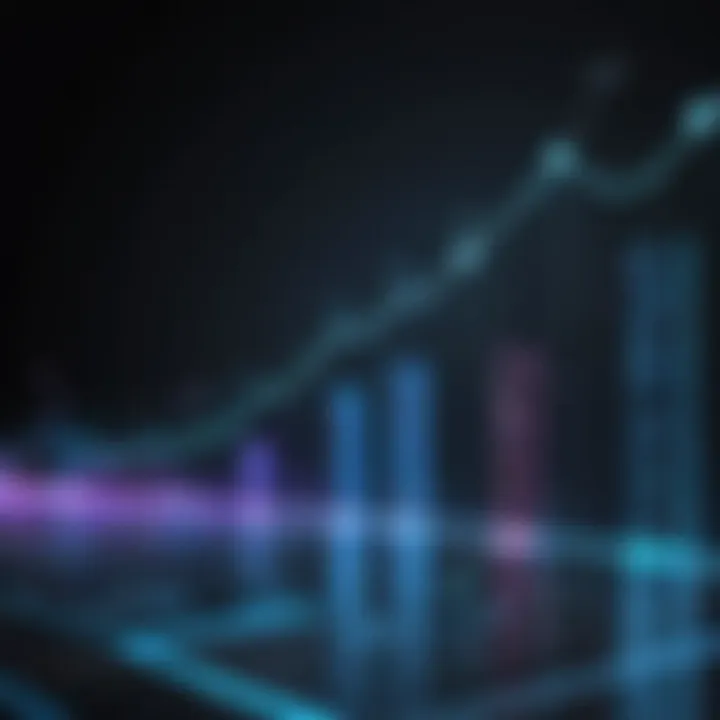
Addressing these integrity challenges is not just a quality matter; itās critical for compliance purposes as well.
Resistance from Stakeholders
Another significant hurdle lies with resistance from stakeholders. Transitioning to a selective data management system means change, and as the saying goes, people donāt generally embrace change with open arms. Employees or decision-makers may fear that selective data transition will lead to redundancy or job losses, or they might simply feel overwhelmed by the new processes and technologies.
It's essential to engage stakeholders early in the transition process. This ensures that they are informed and their concerns are taken into account. Strategies to reduce resistance include:
- Conducting training sessions that demonstrate the benefits of new systems.
- Encouraging open communication for stakeholders to voice their worries.
- Involving them in the planning stages to foster a sense of ownership.
Cultivating a culture of understanding and adaptability will help bridge the gap, making the transition smoother.
Technological Limitations
Lastly, technological limitations pose significant challenges in executing a selective data transition. Not every organization has the luxury of advanced data management tools or a robust IT infrastructure in place. Legacy systems can be clunky and not designed to handle selective data practices, leading to technical hiccups and delays.
Organizations need to evaluate their existing technology and identify gaps that may impede the transition. Often, the resolution involves:
- Investing in scalable data management solutions that can grow with your needs.
- Collaborating with IT teams to assess the compatibility of existing systems with new tools.
- Considering gradual rollout strategies that allow for testing and adjustment without disrupting daily operations.
By keeping an eye on technology limitations and preparing accordingly, businesses can better equip themselves to navigate the complexities of selective data transitions successfully.
"Transitioning does not mean leaving everything behind. It means leveraging lessons from the past for a smoother path forward."
Methodologies for Effective Selective Data Transition
Navigating the waters of data management necessitates a toolbox of methodologies that facilitate a seamless transition. Effectively implementing selective data transition is not just a technical requirement; it hinges on a well-structured approach that ensures the right data is identified, categorized, and automated. The stakes are particularly high; organizations are tasked with transforming chaos into a streamlined operation while safeguarding integrity and compliance.
Identifying Relevant Data
The journey begins with the critical task of identifying relevant data. This phase is akin to panning for gold: amidst the vast river of information, one must sift through the rock and debris to uncover valuable nuggets. Organizations often find themselves inundated with data, much of it redundant or outdated. To overcome this challenge, businesses should deploy analytical tools and frameworks designed to evaluate data relevance.
- Establish Clear Criteria: Organizations must determine what constitutes relevant data. This involves defining parameters based on business objectives, user needs, and regulatory requirements. A checklist style evaluation can reduce ambiguity and streamline selection.
- Conduct Stakeholder Interviews: Conversations with team members from different departments provide diverse perspectives on what data is deemed useful. This collaborative approach can surface insights that formal analysis might miss.
- Utilize Data Profiling Tools: Tools that automate the profiling of unsolicited data can help pinpoint value, reliability, and applicability. This technology reduces manual workload and minimizes the risk of overlooking critical information.
"In the age of information overload, knowing what to keep and what to discard is an artāand a science."
Data Categorization Techniques
Once relevant data is identified, the next step is categorization. Think of it as organizing your closet; when clothes are grouped by type, color, or occasion, it's easier to access what you need. Similarly, categorizing data enhances accessibility and usability.
- Tagging and Metadata: Adding tags or metadata to data sets streamlines searchability. For instance, a simple tagging system could include labels such as "sales data," "customer feedback," or "inventory," aiding quick retrieval.
- Hierarchical Structures: Implementing a structured hierarchy can be especially useful for larger datasets. By creating folders and subfolders based on categories like project type or department, navigating complex datasets becomes manageable.
- Visualization Techniques: Data visualization tools can graphically represent categories. Heatmaps or tree maps provide intuitive ways for stakeholders to interpret data composition, thus aiding decision-making.
Automation in Data Transition
As organizations strive to keep pace with accelerating data growth, automation emerges as a corner stone of effective selective data transition. It reduces the manual burden and minimizes potential errors.
- Automated Workflows: By designing workflows that automate data transition stepsālike data extraction, transformation, and loading (ETL)āorganizations can streamline operations. For example, routine data migrations can be scheduled, ensuring timely updates without the need for constant human intervention.
- Integration with Existing Tools: It's advantageous for businesses to leverage existing software solutions that offer automated data integration capabilities. Tools like Zapier or Microsoft Power Automate, for instance, can connect different platforms, making data flow smoother.
- Continuous Monitoring: Automation shouldn't be a set-it-and-forget-it scenario. Implementing monitoring tools to continuously assess data quality ensures that issues are identified early, maintaining high data integrity throughout the transition process.
In sum, adopting methodologies for effective selective data transition is essential for any organization aiming to thrive in the digital age. By meticulously identifying relevant data, employing thoughtful categorization techniques, and embracing automation, businesses position themselves to navigate the complexities of data management more effectively.
Technology and Tools for Selective Data Transition
Selective data transition is not just a strategic choice; itās a necessity in the modern landscape of data management. The convergence of advancements in technology and the rising tide of data complexity necessitates robust solutions that can streamline processes while ensuring relevance and security. This section delves into the specific technologies and tools that support effective selective data transition, underscoring their relevance and inherent benefits for organizations aiming to thrive in a data-saturated environment.
Role of AI and Machine Learning
Artificial Intelligence (AI) and machine learning are at the forefront of enhancing selective data transition. These technologies play a pivotal role in data categorization and decision-making processes. With their ability to analyze massive datasets quickly, AI algorithms can identify patterns and trends that humans might overlook.
For instance, predictive analytics can help businesses determine which subsets of data are most pertinent to their objectives. When deployed correctly, these technologies can lead to significant savings in time and resources, allowing organizations to focus only on the most relevant information.
- Benefits of AI in Data Transition:
- Enhanced accuracy in data selection.
- Reduction in manual effort and human error.
- Scalability as data grows.
However, one must consider possible drawbacks too. Relying heavily on automated systems can sometimes lead to overlooking critical context that human insight would otherwise provide. Hence, a balanced approach is crucial. Incorporating human oversight into AI-driven processes ensures a more nuanced understanding of data relevance.
Data Management Platforms
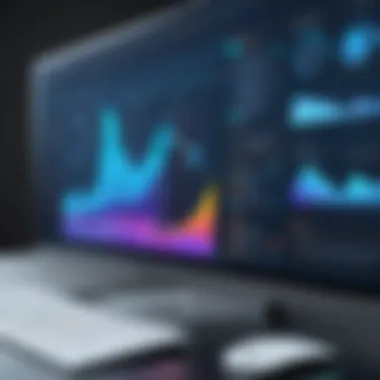
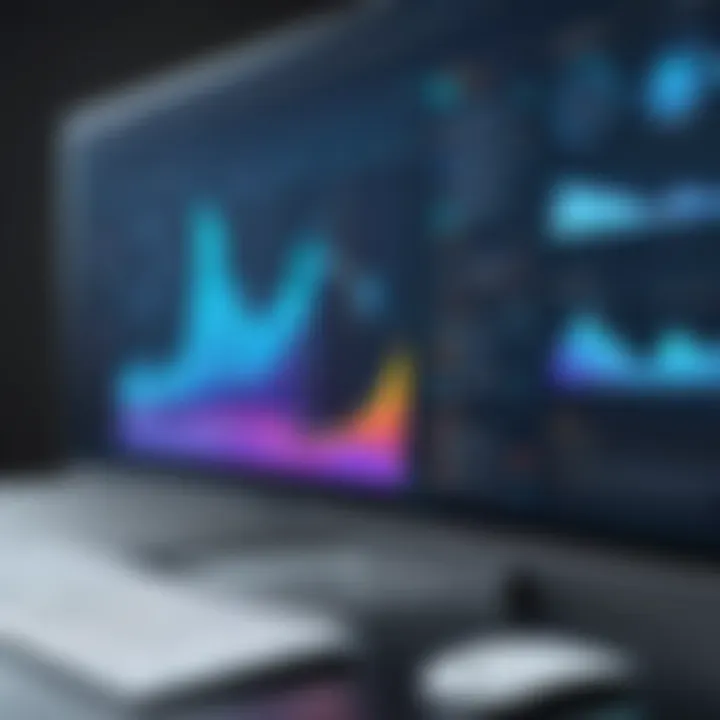
Data management platforms serve as the backbone for effective selective data transition, providing comprehensive tools and frameworks that aid in organizing, storing, and retrieving data efficiently. When organizations implement these platforms, they gain several advantages.
Some of the key features include:
- Centralized Data Repository: Ensures that all data is stored in one accessible location, simplifying data management.
- User-Friendly Interfaces: Facilitates ease of use, enabling users to interact with data without extensive technical training.
- Integration Capabilities: Allows linking with existing software and applications, ensuring seamless data flows.
Modern solutions, such as Salesforce or Microsoft Azure, offer functionalities that not only bolster data transition but also support compliance regulations and enhance data governance. Nevertheless, selecting the right platform requires careful evaluation of the specific needs of the organization, as well as the scalability of the solution chosen.
Cloud Solutions and Their Efficacy
In the realm of data transition, cloud solutions have emerged as a game-changer. They provide flexible, scalable options for businesses dealing with fluctuating data demands. The effectiveness of cloud-based tools lies in their accessibility and collaborative features. Teams can access data from virtually anywhere, which is incredibly advantageous for remote work environments.
Some notable benefits of utilizing cloud solutions include:
- Cost Efficiency: Many cloud solutions operate on a pay-as-you-go basis, which can significantly reduce infrastructure costs.
- Automatic Updates: Providers regularly update their services, ensuring that users always have the latest tools without the hassle of manual upgrades.
- Disaster Recovery Solutions: Most cloud providers include data backup and recovery options that enhance data security and availability.
However, businesses should remain vigilant regarding potential security risks associated with cloud storage. It is imperative to weigh the benefits against potential vulnerabilities, ensuring that robust security measures are in place to protect sensitive information.
In summary, the integration of advanced technologies and tools is not merely supportive; it is integral to the success of selective data transition. Embracing these solutions allows organizations not only to survive but to thrive amidst the complexities of modern data management.
Impact of Selective Data Transition on Business Operations
Selective data transition carries weighty implications for how businesses operate today. In a world where data is often equated with gold, companies find themselves sifting through mountains of information to pinpoint what truly matters. This particularly holds true as organizations strive for efficiency, elevated decision-making, and long-term advantages that can make or break their standing in competitive markets.
Through this lens, it's crucial to explore how selective data transition contributes to operational effectiveness. Businesses that embrace this approach not only streamline their processes but also position themselves better in understanding and responding to market dynamics. Thus, itās paramount to recognize its multifaceted impact on business operations.
Efficiency Gains
One of the first things that springs to mind when discussing selective data transition is the significant efficiency gains it fosters. When organizations utilize this technique, they essentially eliminate redundant and outdated data that clutters their systems. This not only helps in reducing data storage costs, but it also accelerates data retrieval processes. Time spent wading through irrelevant files can be cut down dramatically.
Consider, for instance, a mid-sized e-commerce firm struggling with slow load times due to excessive data. After a selective transition, the firm found that by managing data more effectively, not only did their system run smoother, but customers experienced faster web pages, leading to improved sales conversions.
"Streamlining data processes can lead to sizeable reductions in operational costs and time, creating a win-win scenario for businesses."
Enhanced Decision-Making
The benefits don't stop at mere efficiency. Selective data transition actively enhances decision-making capabilities. By concentrating on the most pertinent data, leaders and their teams can make informed choices grounded in high-quality information. In real-time scenarios, when a company has immediate access to relevant insights, it can pivot strategies swiftly as market conditions change.
For example, a financial services company that adopted selective data practices was able to harness analytics far more effectively. This allowed them to predict trends and customer behaviors accurately. The insights gleaned enabled their marketing team to tailor campaigns specifically to customer needsāleading to an increased client retention rate.
Long-Term Strategic Benefits
Looking beyond immediate efficiency and decision-making, the long-term strategic benefits are perhaps the most profound. Companies utilizing selective data transition are not just reacting to trends but are setting themselves up as industry leaders. This foresight allows for proactive planning, ensuring that organizations can align their goals with evolving market demands.
For instance, a tech startup that regularly applies selective data transition can anticipate what innovations its customers may demand in a yearās time. By analyzing selective datasets, they are able to innovate their product development roadmaps, ultimately leading to sustainable growth. As the marketplace evolves, those firms equipped with the right information stand to thrive while othersāclinging to outdated dataārisk stagnation.
In summary, the impact of selective data transition on business operations is both comprehensive and significant. It unlocks efficiency gains, enhances decision-making abilities, and paves the way for long-term strategic benefits that ensure an organization remains competitive in the ever-evolving landscape of modern business.
Case Studies in Selective Data Transition
In the world of data management, the ability to learn from both successes and setbacks can provide invaluable insights. Case studies serve as powerful tools, offering real-world examples that illuminate the path forward. They showcase how various organizations have approached selective data transition, highlighting methods that yielded positive results while also revealing pitfalls to avoid. The analysis of these experiences not only paints a richer picture of the capabilities of selective data transition but also equips professionals with actionable strategies and a deeper understanding of how to navigate the complexities of the data landscape.
Successful Implementations
Many companies have embraced selective data transition with splendid results. One notable example is Toyota Motor Corporation, which has integrated selective data practices to streamline its supply chain processes. By focusing on key performance indicators and relevant data sets, Toyota effectively minimized waste and improved production speed. This targeted approach enabled the company to adjust its operational strategies in real-time, significantly increasing efficiency without a total overhaul of its existing systems.
Another impressive case can be seen in Netflix. Their selective data transition strategies have empowered them to leverage viewer habits to drive content creation. By sifting through enormous amounts of user data, Netflix identifies trends and preferences, allowing it to craft shows that resonate with audiences. This not only enhances viewer satisfaction but also boosts engagement and subscriptions, illustrating how aligned data decisions can transform business outputs.
- Toyota Examples:
- Netflix Examples:
- Efficient production adjustments
- Minimization of waste
- Real-time updates
- Data-driven content creation
- Increased viewer satisfaction
- Enhanced engagement through targeted strategy
These case studies depict a compelling narrative on how organizations can drive meaningful changes through selective data application. When done right, selective data transition empowers companies to align their operations with market demands and consumer preferences, creating a robust business model.
Lessons Learned from Failures
On the flip side, studying failures offers critical lessons in what not to do. The case of Target provides a glaring example. Aimed at refining its marketing strategies through selective data usage, the retailer faced a substantial backlash when it inaccurately targeted customers based on sensitive data. This tactic not only raised privacy concerns but also alienated customers, illustrating that miscalibrated data transition can breach trust and lead to brand damage.
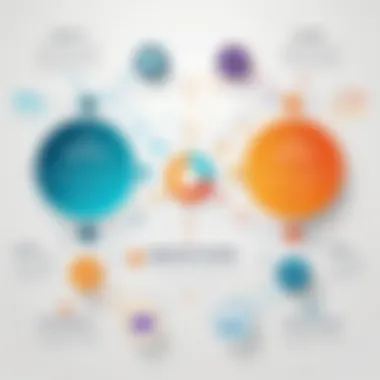
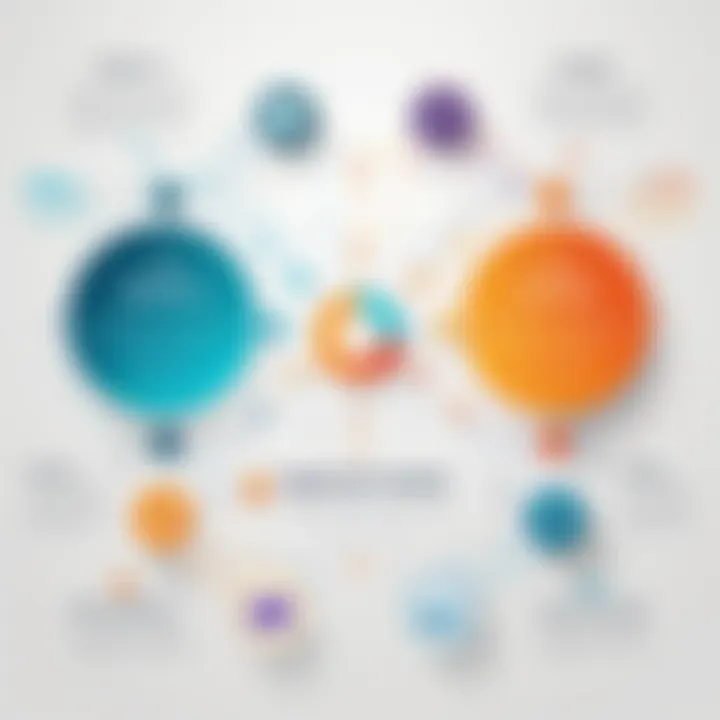
Similarly, Yahoo! struggled with its approach to selective data management during the early 2000s. The company collected vast amounts of user data but failed to effectively prioritize what was meaningful. As a result, their data strategies became cumbersome and led to invaluable oversight, contributing to the decline of their relevance in the competitive digital marketplace.
- Target Example:
- Yahoo Example:
- Targeted marketing gone wrong
- Customer trust compromised
- Privacy issues raised
- Data overload without prioritization
- Loss of competitive edge
- Missed opportunities for meaningful engagement
From these cautionary tales, it emerges that an unfocused approach to data transition not only complicates analytics but can jeopardize relationships with stakeholders and customers. Thus, it's paramount to strike a balance between the volume of data collected and the relevance of that information. Keeping an ear to the ground and being sensitive to the implications of data practices is crucial for sustainable success in selective data transition.
Future Trends in Selective Data Transition
As the landscape of data management morphs and evolves, the discussion around future trends in selective data transition becomes increasingly crucial. Organizations seek to remain agile and responsive in an environment that resembles a runaway freight train of information overload. Adapting to emerging trends is essential not only for survival but for enhancing operational efficacy and making informed decisions. Understanding these future trends equips businesses with tools that can shape strategies, streamline processes, and address evolving challenges.
Emerging Technologies
Technology is the wind in the sails of selective data transition, pushing it toward new horizons. Among the emerging technologies driving change, the role of artificial intelligence and machine learning stands out. These tools offer significant potential to automate the data selection and categorization processes, making transitions less burdensome and more efficient. For example, machine learning algorithms can identify patterns and anomalies in data that may not be immediately obvious. This capability allows organizations to filter through mountains of information and focus only on what truly matters.
Additionally, advancements in natural language processing (NLP) are making it easier for non-technical users to interact with complex datasets. Business professionals, regardless of their technical know-how, can now harness the power of data through intuitive interfaces. With tools like chatbots and voice search, teams can retrieve information without going down a digital rabbit hole.
Moreover, as the Internet of Things expands its reach, companies are presented with streams of real-time data that must be utilized effectively. Companies that can leverage this influx of data into actionable insights will gain a competitive edge.
"Data is the new oil; the ability to refine it is where true value lies."
Evolving Business Needs
The demands placed on businesses today are nothing short of Herculean, and the need for selective data transition reflects that. Modern enterprises operate in an era where speed and accuracy are paramount. Clients expect tailored services, and as a result, businesses must become nimble, often recalibrating their strategies on the fly. This is where selective data transition shinesāit allows organizations to adapt to shifting market dynamics and consumer preferences.
Furthermore, many companies are transitioning toward a more customer-centric approach. Understanding customer behavior, preferences, and feedback in real time can dramatically influence product development and marketing strategies. Employing selective data transition enables organizations to capture only the data that directly impacts this customer-centric model. This focused approach can lead to enhanced customer satisfaction and, counterintuitively, lower operational costs.
The rise of remote work and globalization also play vital roles in shaping future needs. Businesses must ensure seamless collaboration across dispersed teams, and selective data transition can facilitate secure and efficient access to the right data at the right time. Businesses that adapt to these evolving needs will not only improve their operational workflow but can also position themselves as industry leaders.
Ethical Considerations in Data Transition
As businesses embrace selective data transition, it becomes increasingly crucial to address ethical considerations. In the age of information, handling data is not merely a technical challenge; it's a moral obligation. To ensure that organizations maintain trust with their stakeholders, consideration of privacy and security risks must be at the forefront of their data management strategies. Ignoring these aspects can lead to reputational damage and legal repercussions. Navigating ethical dilemmas in data transition is essential for sustainability and compliance in modern business practices.
Privacy and Security Concerns
When we talk about privacy and security, we touch upon the backbone of ethical data management. Today, organizations collect vast amounts of information, much of which is personal and sensitive. Mismanagement of this data can lead to identity theft, breaches, and loss of consumer confidence.
- User Consent: One of the cornerstones of ethical data handling is ensuring that users have authorized the collection and transition of their data. Organizations must be clear about what data is being collected and for what purpose. Consent should not be a vague box to check but an active agreement from the user.
- Data Minimization: Itās crucial to collect only the data that is necessary for specific objectives. This means companies should avoid hoarding unnecessary information, which can create additional risks.
- Secure Transition Methods: Implementing precise methodologies to protect data during transitions is vital. Companies should employ encryption and other security measures to safeguard data from unauthorized access.
- Regular Audits: Conducting frequent audits can help organizations recognize potential vulnerabilities in their data handling practices. This proactive approach is essential for addressing any privacy concerns before they escalate.
"In a world where data is currency, trust is the key to unlocking its value."
Transparency in Data Handling
Transparency in data handling entails being open about how data is collected, stored, and utilized. This includes providing clear and accessible information to users regarding key aspects of data management. Organizations that embrace transparency foster a culture of trust and accountability, which is paramount in today's data-driven ecosystem.
- Clear Policies: Organizations must craft clear data policies that are easily understood by all stakeholders. These should detail how data will be used and outline users' rights regarding their personal information.
- Communication with Stakeholders: Keeping stakeholders informed about any changes in data processing practices can help reduce misunderstandings. Regular updates and open channels for questions can enhance transparency.
- Engagement with Data Owners: Actively involving data owners in discussions about their data can enhance trust. Furthermore, involving them in decisions regarding data transitions can ensure their concerns are taken into account.
- Accountability Mechanisms: Establishing accountability mechanisms is critical. Organizations should be willing to accept consequences for failing to meet ethical data standards and demonstrate how they plan to rectify such situations.
In short, ethical considerations in data transition are not merely about compliance; they are fundamentally about doing right by the users. By prioritizing privacy and security, and maintaining transparency, businesses can navigate the complexities of data management while building stronger, more trust-based relationships with the individuals whose data they handle.
Ending and Recommendations
In the landscape of contemporary data management, concluding thoughts yield significant insights into selective data transition. Itās not just a passing trend; itās essential for organizations trying to keep their heads above the ever-rising tide of data. With the increasing demands for efficiency, strategic advantage, and accountability, understanding the fundamentals and implications of selective data transition is crucial. Businesses that prioritize this practice can avoid drowning in data overload while harnessing the benefits that come with targeted information retention and utilization.
Summarizing Key Insights
Reflecting on the discussions throughout this article, several key insights emerge regarding selective data transition:
- Critical for Navigation: Organizations must efficiently sift through extensive data landscapes to ensure they can identify what is truly relevant and useful. This requires a robust framework to categorize and manage data effectively.
- Challenges Exist: Bridging the gap between data overload and informed decision-making isn't without challenges. Issues such as data integrity, resistance from key stakeholders, and technological constraints pose obstacles that must be navigated.
- Future-Focused Strategies: Organizations looking to thrive should maintain a continuous focus on emerging technologies and trends in data management. This guarantees adaptability to the ever-changing landscape of data utilization and access.
"Selective data transition is not a luxury; itās a necessity in todayās data-driven world. Adapting to this wave can determine the future of your organization."
These insights serve as a foundation for developing actionable steps that organizations can undertake to implement selective data transition successfully.
Actionable Steps for Businesses
For businesses aiming to enhance their data management practices, the following steps are recommended for implementing selective data transition:
- Conduct an Assessment of Current Data: Before transitioning, businesses must understand what data they currently possess and its relevance to their operations. Identifying which datasets hold value and which can be discarded is the first crucial step.
- Develop a Data Governance Framework: Establish clear policies and procedures for managing data. This framework should outline how to categorize, review, and retain relevant data while ensuring compliance with relevant regulations.
- Engage Stakeholders: Involve key stakeholders from various departments early on. This collaborative approach helps mitigate resistance and ensures that the selected data aligns with broader organizational goals.
- Utilize Technology Solutions: Leverage tools and technologies like data management platforms that incorporate AI and machine learning to aid in data categorization and automate the transition process.
- Establish Metrics for Success: Implement metrics to evaluate the effect of selective data transition on decision-making and operational efficiency. Regularly review these metrics for improvements.
- Continual Training and Development: Keep staff updated on best practices in data management and emerging technologies. Ongoing education reinforces the importance of data management and keeps the organization adaptable.
By adhering to these actionable steps, businesses can not only navigate the complexities of selective data transition but also maximize the benefits it promises, fostering a more efficient data environment.