Top Data Integration Tools for Modern Businesses
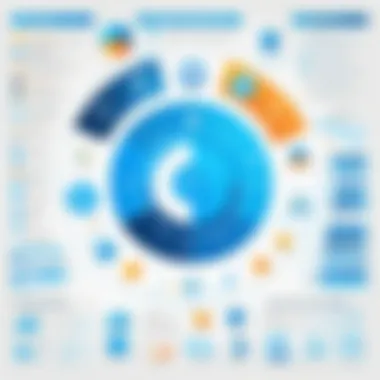
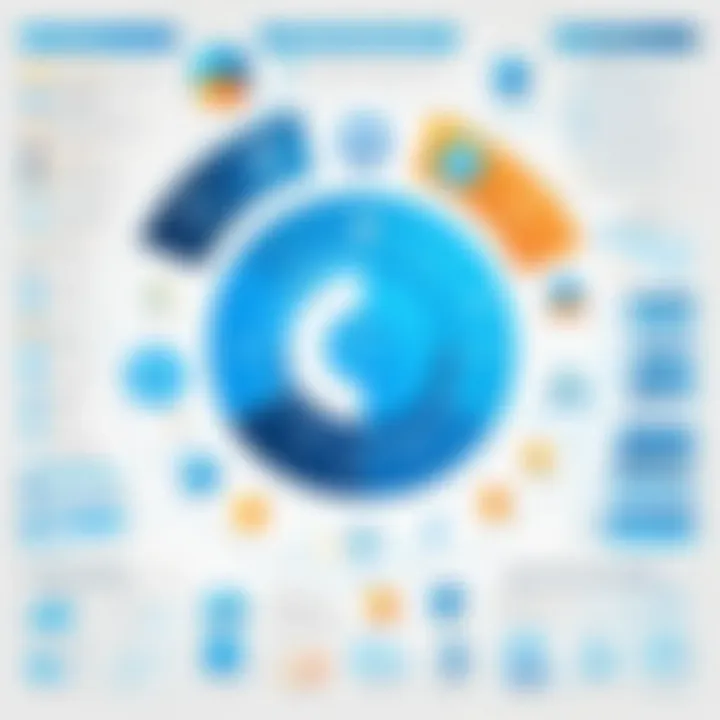
Intro
In the current landscape of modern business, data integration tools have become indispensable. Organizations generate vast amounts of data from various sources every day. The need to synthesize this data into meaningful insights is becoming more pronounced. This article focuses on key data integration tools that facilitate the processing and analysis of data, tailoring solutions to meet the specific requirements of businesses. By examining each tool's functionality, advantages, and application, businesses can make more informed decisions and improve their data management strategies.
Technological Research Overview
Recent Technological Innovations
Data integration has grown over the years, driven by rapid technological advancements. Cloud computing, for instance, has made data more accessible, allowing businesses to store and share data effortlessly. Technologies like application programming interfaces (APIs) enable seamless communication between different systems, further enhancing integration processes. Innovations in machine learning also contribute to data integration. With algorithms capable of analyzing complex datasets, businesses can derive insights with unprecedented accuracy.
Impact on Business Operations
The integration of data can lead to improved decision-making and greater operational efficiency. Organizations are not only able to access real-time data but also glean insights that guide strategic planning. This reduction in data silos fosters collaboration across departments, ensuring everyone works with the same information. Furthermore, businesses that implement effective data integration tools report higher productivity and lower operational costs.
Future Technological Trends
Looking ahead, businesses might expect the rise of more advanced data integration solutions. Trends such as automation and increased use of artificial intelligence in data management will likely dominate the industry. As businesses strive for agility and responsiveness, integrated solutions that reduce manual tasks will be critical. It's essential for organizations to stay abreast of these trends to remain competitively positioned.
Data Analytics in Business
Importance of Data Analytics
Data analytics plays a crucial role in guiding business strategies. By analyzing trends and patterns within data, organizations can make more accurate predictions. This data-driven approach is essential for minimizing risks and maximizing opportunities. It’s not just about handling data; it’s about deriving actionable insights from it.
Tools for Data Analysis
Several data analytics tools are available to businesses today. Some popular options include Tableau, Microsoft Power BI, and Apache Spark. Each tool offers unique features targeted at specific needs, such as visualization or real-time streaming analytics. Businesses must assess their requirements to choose the right tool that complements their data integration approach.
Case Studies on Data-Driven Decisions
There are numerous examples of businesses leveraging data analytics for improved decision-making. For instance, Netflix employs sophisticated algorithms to analyze user preferences and recommend content effectively. Similarly, Target uses data analytics to understand shopping behaviors, allowing for targeted marketing strategies. Both instances demonstrate that when integrated effectively, data can significantly enhance business operations and customer satisfaction.
Cybersecurity Insights
Threat Landscape Analysis
As businesses become more data-centric, they also become targets for cyber threats. Understanding the threat landscape is vital. Cyber attacks can lead to data breaches, financial loss, and reputational damage. Organizations must be vigilant about the potential risks that may arise from data integration efforts.
Best Practices for Cybersecurity
To mitigate these risks, businesses should adopt best practices for cybersecurity. This includes implementing strong access controls, routinely updating software, and using encryption methods to protect sensitive data. Regular security audits can identify weaknesses in systems, allowing for timely repairs.
Regulatory Compliance in Cybersecurity
With increased scrutiny on data privacy, businesses must navigate a complex regulatory environment. Compliance with regulations like GDPR is critical. Organizations must ensure that their data handling practices align with relevant laws and regulations to avoid potential penalties.
Artificial Intelligence Applications
AI in Business Automation
Artificial intelligence can revolutionize data integration processes. Through automation, AI can significantly expedite data collection and analysis, freeing up employees to focus on strategic tasks. This not only increases efficiency but also reduces the likelihood of human error.
AI Algorithms and Applications
Various AI algorithms are being applied in business settings, such as natural language processing and predictive analytics. These technologies allow organizations to process large volumes of data quickly, providing valuable insights within a shorter timeframe. The ongoing development of AI capabilities suggests that its role in data integration will only continue to grow.
Ethical Considerations in AI
As businesses increasingly rely on AI, ethical considerations cannot be overlooked. Issues surrounding data privacy and algorithmic bias must be addressed. Organizations should develop clear policies that guide the ethical use of AI to protect their customers and uphold public trust.
Industry-Specific Research
Tech Research in Finance Sector
In the finance sector, data integration tools play a crucial role in risk management and regulatory compliance. Financial institutions are leveraging these tools to analyze portfolio performance and monitor market trends effectively.
Healthcare Technological Advancements
In healthcare, data integration tools improve patient care by consolidating patient data from various sources. This integrated approach allows for a more comprehensive understanding of patient histories, leading to better diagnosis and treatment options.
Retail Industry Tech Solutions
The retail industry utilizes data integration tools to understand consumer behavior. By integrating data from in-store transactions and online purchases, retailers can tailor their marketing efforts and optimize inventory management. This results in a more personalized shopping experience and improved sales outcomes.
"Understanding the right tools for data integration is essential for modern businesses aiming for growth and efficiency."
Prelude to Data Integration
Data integration has become an essential component for firms navigating today's data-driven landscape. It entails the process of combining data from different sources to provide a unified view, helping businesses streamline operations and enhance decision-making capabilities. Organizations generate immense amounts of data, and without effective integration techniques, valuable insights may remain buried. This article delves into the significance of data integration and its impact on modern businesses.
Definition of Data Integration
Data integration refers to the technique of combining data from various heterogeneous sources into one coherent system. This process combines data from databases, cloud services, files, and more into a single format for easier analysis and usage. The aim is to create a consolidated dataset that is accessible and understandable for various stakeholders within an organization.
The essence of data integration lies in its ability to enable a seamless flow of information among the disparate systems an organization might use, ensuring that users can make informed decisions based on the latest and most accurate data. The integration can be structured, as seen with database management systems, or unstructured, as encountered with document collections.
Importance for Businesses
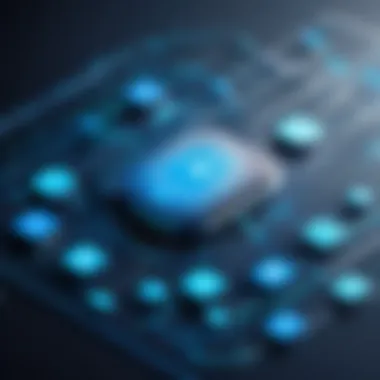
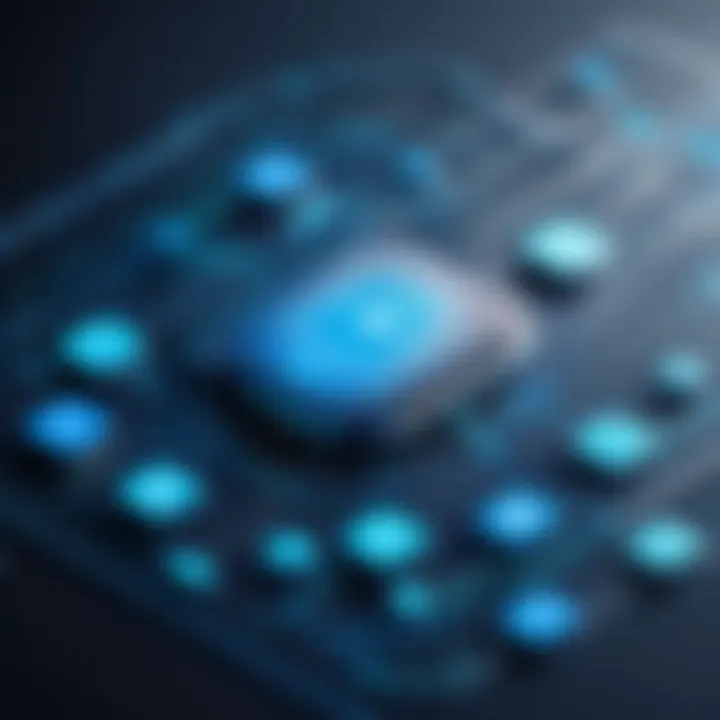
Understanding the importance of data integration is key for businesses aiming to stay competitive. Here are several reasons why it is crucial:
- Improved Decision-Making: When data is integrated, decision-makers have access to comprehensive information. This enhanced visibility allows for more informed decisions that align with current trends and operations.
- Increased Efficiency: Data integration reduces manual processes and the duplication of efforts. With a unified dataset, employees can focus more on analyzing data rather than collecting it.
- Data Quality: Integrated data often displays higher quality due to its synchronization from multiple sources. Enhancing data accuracy can lead to more reliable insights.
- Cost Reduction: By streamlining processes, data integration can minimize costs related to data management and potentially reduce operational expenses.
- Scalability: As businesses grow, their data needs expand. A solid data integration strategy allows organizations to scale their operations without significant challenges.
"Data integration serves as the foundation of effective analytics, enabling organizations to uncover valuable insights that drive strategic decisions."
As modern businesses grapple with ever-increasing data volumes, the significance of mastering data integration techniques cannot be overstated. The next sections will delve into key considerations and a review of top tools that facilitate these processes.
Key Considerations in Selecting Data Integration Tools
Selecting the right data integration tool is crucial for any modern business. This decision influences not only the efficiency of data management processes but also impacts overall operational effectiveness. Understanding the key considerations in this selection process can lead to informed decision-making. Key factors to consider include scalability and flexibility, ease of use and integration, as well as cost efficiency. Each of these elements plays a vital role in ensuring that the chosen tool aligns with business goals and adapts to evolving data landscapes.
Scalability and Flexibility
Scalability and flexibility are significant for choosing a data integration tool. Modern businesses often experience fluctuating data volumes. Thus, it is critical that the selected tool can handle increasing amounts of data without loss of performance. A scalable tool allows organizations to adjust resources in accordance with their needs.
Flexibility also can not be understated. As business requirements evolve, tools must adapt to various integration scenarios. This includes capabilities to connect with different data sources, both cloud-based and on-premises. If a tool lacks flexibility, businesses may face challenges in leveraging new data or integrating with emerging technologies.
A tool like Apache NiFi exemplifies strong scalability—allowing for dynamic data flows and real-time processing, which is essential for companies experiencing rapid data growth.
Ease of Use and Integration
The usability of data integration tools is another core consideration. These tools must be user-friendly to ensure that team members, whether technical or non-technical, can operate them effectively. A complicated interface not only raises training costs but can also lead to underutilization of the tool's features.
Moreover, ease of integration with existing systems is vital. A tool that does not easily connect with current software environments can slow down data processes. For instance, Talend offers a graphical interface which enables users to manage integration processes without extensive coding knowledge, making it accessible for various users.
Cost Efficiency
Cost efficiency is often a decisive factor when selecting a data integration tool. Organizations must evaluate both initial investment costs and ongoing operational expenses. Tools that offer robust features may come with a higher price tag. However, it is essential to assess long-term value rather than focusing solely on upfront costs.
Additionally, consider potential hidden costs, such as maintenance fees, training, and additional licensing. A tool like Microsoft Azure Data Factory not only provides competitive pricing but also integrates seamlessly with existing Microsoft resources, providing significant savings as a result.
Selecting the right tool must be a balance of capabilities and financial sustainability.
By weighing these considerations, businesses can select data integration tools that not only support their current needs but also scale with their growth ambitions. It's important to invest time in this evaluation process to ensure the foundation for effective data management is solid.
Overview of Top Ten Data Integration Tools
Data integration tools play a pivotal role in contemporary data management strategies. They facilitate the seamless convergence of data from disparate sources, enabling businesses to make data-driven decisions efficiently. In this article, we will provide a comprehensive overview of ten leading data integration tools, each bringing unique features and capabilities to the table.
The importance of understanding these tools cannot be overstated. By selecting the right integration solution, organizations can enhance their processes, improve data quality, and ultimately drive better outcomes.
Tool One: Apache NiFi
Features
Apache NiFi is recognized for its sophisticated data flow management. Its key characteristic is its user-friendly interface, which allows users to visually design and manage data flows. This feature ensures that businesses can quickly adapt their integration processes to meet changing demands. A unique aspect of NiFi is its ability to handle data provenance, giving users insights into data movement and transformation history. However, the complexity of its advanced features may pose a challenge for inexperienced users.
Use Cases
The versatility of Apache NiFi makes it suitable for various applications. It is widely used in real-time data streaming, IoT data ingestion, and ETL processes. This popularity stems from its capability to process large volumes of data with low latency. The ability to create data-driven workflows ensures efficient data processing across organizational departments. The advantage of using NiFi lies in its capacity for handling diverse data types, but it may require considerable resources for optimal performance.
Limitations
Despite its strengths, Apache NiFi has limitations. Its configuration can be complex, leading to a steep learning curve for new users. Moreover, its performance can degrade with overly complex workflows. The need for robust hardware can also restrict its deployment in resource-limited environments. Nonetheless, for organizations with adequate technical expertise, NyFi presents a powerful option.
Tool Two: Talend
Features
Talend stands out due to its open-source nature and extensive integration capabilities. The key characteristic of Talend is its component-based architecture, which allows for easy customization and scalability. This makes it an appealing choice for organizations looking to tailor their data solutions. A notable feature is its built-in support for cloud integration, enhancing flexibility. However, relying heavily on open-source components may lead to potential support challenges.
Use Cases
Talend caters to various integration scenarios, including data migration and real-time analytics. Its beneficial aspect includes compatibility with a wide range of data sources, from databases to cloud services. This flexibility in use cases allows businesses to streamline their data operations effectively. However, organizations must assess their needs against Talend's complexity to maximize its potential.
Limitations
While Talend offers robust functionalities, it can also present challenges. The learning curve associated with mastering the platform can be significant, especially for smaller teams. Furthermore, the reliance on community support for the open-source version may not suffice for organizations requiring immediate assistance. Thus, while Talend is powerful, businesses must be prepared for a commitment to learning.
Tool Three: Informatica PowerCenter
Features
Informatica PowerCenter is notable for its enterprise-grade data integration capabilities. The key strength of this tool lies in its metadata management features, which help maintain data integrity. Additionally, PowerCenter offers a wide range of connectors for various data systems, promoting compatibility. A noteworthy aspect is its advanced security features, which ensure data protection during the integration process. However, these extensive features come with a licensing cost.
Use Cases
PowerCenter is primarily utilized in large organizations for complex data integration tasks. It is ideal for enterprise-level ETL operations and offers extensive support for batch processing. Its popularity in large-scale implementations underscores its ability to manage high-volume data loads efficiently. Nevertheless, smaller organizations may find the extensive capabilities to be more than they need.
Limitations
The licensing costs associated with Informatica PowerCenter may pose a financial hurdle for many businesses. Furthermore, the complexity of its interface can lead to a prolonged onboarding process for new users. While its features are undoubtedly powerful, the resource investment can be a consideration for budget-conscious organizations.
Tool Four: Microsoft Azure Data Factory
Features
Microsoft Azure Data Factory is designed for cloud data integration. A primary characteristic of this tool is its ability to create data-driven workflows across Azure services and on-premises data sources. Its unique feature—integration with Azure services—offers businesses a cohesive data ecosystem. The platform provides scalability and robustness but may require cloud familiarity from users.
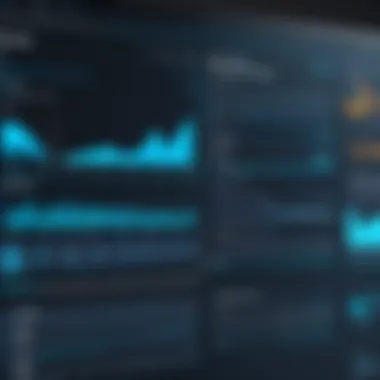
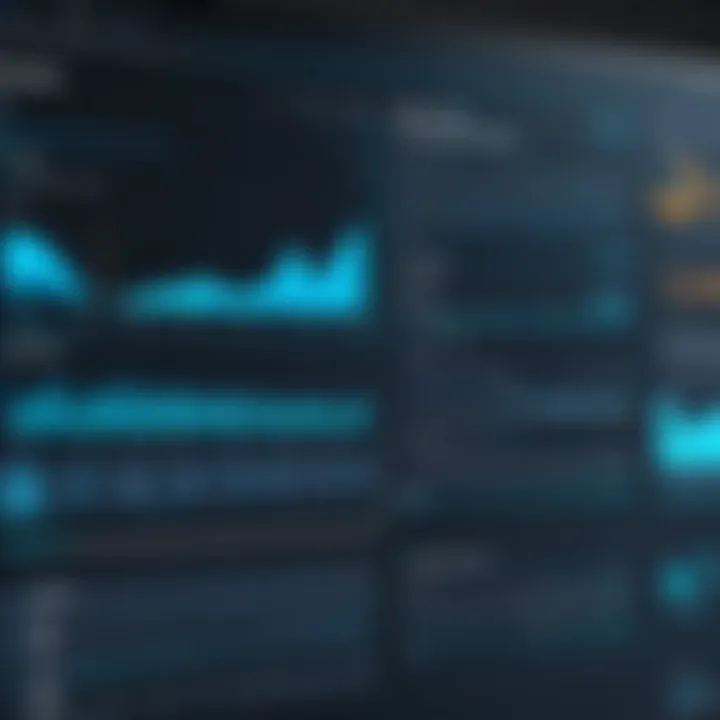
Use Cases
Azure Data Factory is primarily used for ETL processes in cloud environments. It supports hybrid data integration, allowing organizations to connect on-premises data with cloud resources seamlessly. The advantage here lies in leveraging Microsoft’s ecosystem for data analytics and management. However, companies unfamiliar with the Azure environment may face initial integration challenges.
Limitations
While Azure Data Factory offers multiple benefits, reliance on the cloud can limit access for organizations with strict data regulations. Additionally, costs can accumulate based on resource usage, potentially leading to unexpected expenses. Although it presents a flexible solution, monitoring usage is crucial for budget control.
Tool Five: MuleSoft Anypoint
Features
MuleSoft Anypoint is designed for application integration. Its key aspect is its API-led connectivity, enabling businesses to create reusable APIs for integration. This facilitates rapid data exchange across various applications. A notable feature is its Anypoint Studio, allowing for easy development and management of integrations. However, the complexity could be a barrier for teams lacking programming expertise.
Use Cases
Organizations employ MuleSoft for integration projects ranging from CRM systems to ERP applications. Its advantage comes from the ability to streamline data flow between applications, enhancing operational efficiency. While effective, the initial setup can be time-intensive, which companies need to consider.
Limitations
MuleSoft's cost is a concern for many organizations, particularly small businesses. The need for technical expertise to fully utilize its features can also restrict usage. Additionally, while powerful, it may not address every specific integration need, requiring supplementary solutions.
Tool Six: Fivetran
Features
Fivetran specializes in automated data pipelines. Its key characteristic is its ease of use, providing plug-and-play functionality for data connectors. This accessibility makes it a popular choice for businesses seeking simplicity. A unique attribute is its focus on schema management, ensuring that data remains consistent during integration. However, its automated approach may reduce customization flexibility.
Use Cases
Fivetran is commonly used for analytics and data warehousing projects. The benefit here is the reduction in manual workload and faster time to insights. Companies focusing on data analytics can significantly enhance their operational efficiency. Yet, its automation means that businesses must be comfortable with less hands-on control over the integration process.
Limitations
Despite its convenience, Fivetran's pricing structure can become expensive over time, especially for organizations with large datasets. Customization options are also limited, possibly leading to difficulties in meeting specific integration requirements. Therefore, businesses need to weigh the balance between ease of use and necessary customizability.
Tool Seven: Boomi
Features
Boomi is known for its low-code integration capabilities. The key strength of this platform is its visual interface, which simplifies the integration process. This enables non-technical users to contribute effectively. A notable feature includes its extensive library of connectors, allowing for fast deployment across applications. However, while it is user-friendly, performance may vary under heavy loads.
Use Cases
Organizations frequently use Boomi for quick integrations in hybrid and multi-cloud environments. Its strength lies in enabling rapid setup, which is crucial for businesses needing to adapt swiftly. This flexibility can lead to faster project turnaround times, but resource demands should be monitored.
Limitations
The limitations of Boomi include potential performance issues during peak usage times. Furthermore, subscription costs can escalate as usage grows, placing a strain on budgets. Organizations must evaluate their long-term needs against Boomi's operational demands.
Tool Eight: SAP Data Services
Features
SAP Data Services is recognized for its data quality and cleansing capabilities. The core feature is its ability to enhance data integrity by applying advanced validation algorithms. This ensures organizations maintain high-quality datasets. A key advantage is integration with other SAP solutions, which can streamline processes. However, its complexity may require substantial training and resources.
Use Cases
Companies typically use SAP Data Services in business intelligence and data warehousing scenarios. The benefit lies in the enhanced ability to manage large data volumes effectively. However, businesses may need to invest in training to fully leverage its functionalities, which can be a barrier for smaller organizations.
Limitations
SAP Data Services may be too complex for smaller companies, both in terms of features and costs. Integration with non-SAP systems can also present challenges, limiting flexibility. Organizations should carefully consider their existing infrastructure before adopting this tool.
Tool Nine: Oracle Data Integrator
Features
Oracle Data Integrator provides a unified platform for data integration. Its primary characteristic is its support for various data models and sources, which broadens its applicability. The tool's unique aspect is its high performance in processing large datasets, which appeals to enterprise-level organizations. However, this performance often comes with a steep learning curve for beginners.
Use Cases
Oracle Data Integrator is typically employed in data transformation and migration processes. Its beneficial aspect lies in the ability to handle complex ETL workflows efficiently. Nevertheless, organizations may find initial setup cumbersome due to its extensive functionalities.
Limitations
The reliance on Oracle environments can restrict flexibility for organizations leveraging multiple systems. Licensing costs may also be a barrier for some businesses. Companies should evaluate their integration needs thoroughly before committing.
Tool Ten: Stitch Data
Features
Stitch Data is recognized for its simplicity and straightforward approach to ETL. A key feature is its cloud-based architecture, which facilitates easy data replication. The advantage lies in its extensive connector library, enabling integration with many data sources. However, its minimalistic design can limit customizable integration flows.
Use Cases
Stitch Data is particularly useful for analytics and reporting use cases, especially in cloud environments. Its key characteristic is scalability, allowing businesses to grow without significant changes to their integration architecture. However, the need for specific customizations may prompt organizations to consider supplementary solutions.
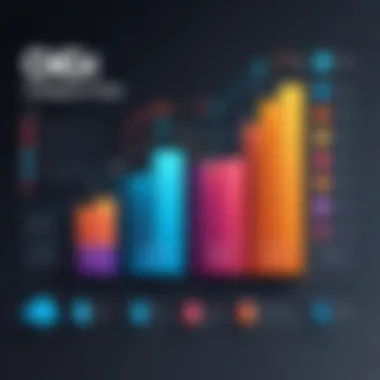
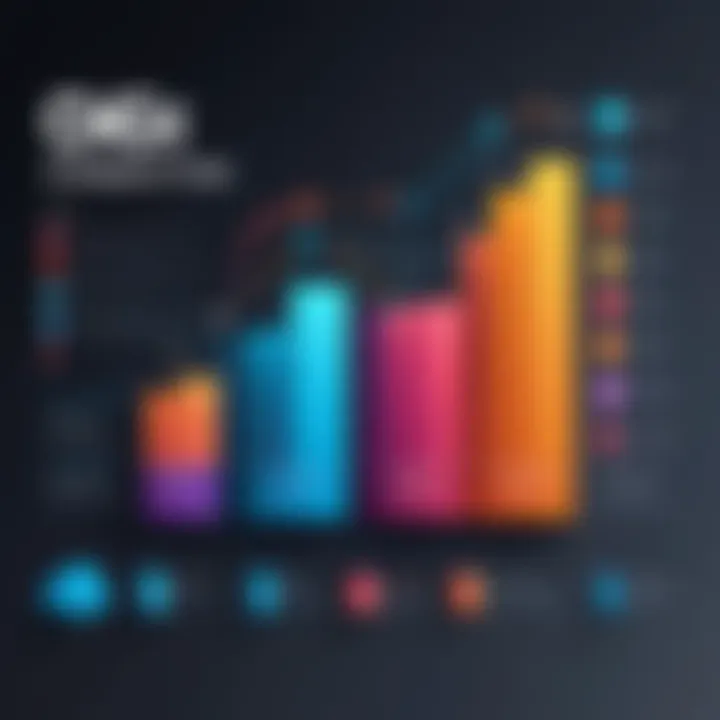
Limitations
While easy to set up, Stitch Data has limitations in terms of customization. This can pose challenges for organizations needing specific transformations during the data integration process. Additionally, as data volumes grow, subscription costs may increase significantly. Organizations must weigh ease of use against their unique data integration requirements.
Comparative Analysis of Tools
In the realm of data integration, a comparative analysis of tools is essential. This section provides a framework for assessing various integration tools based on critical factors that impact their performance and usability. By looking at functionality and user experience, businesses can make informed decisions, tailoring their choice to their specific needs.
Functionality Comparison
When evaluating data integration tools, functionality is paramount. Each tool offers distinct features that address different data integration requirements. Key functional aspects to consider include:
- Data Sources and Connectors: Does the tool connect easily with various data sources like databases, cloud applications, and on-premises systems? Some tools, like Talend, provide extensive support for various connectors, while others might be more limited.
- Real-Time Processing: The need for real-time data integration grows as businesses require timely information. Tools like Apache NiFi excel in this area, providing capabilities to process data on the go.
- Data Transformation and Cleansing: Many integration tasks involve data transformation. Tools such as Informatica PowerCenter offer robust features for cleansing and transforming data to ensure it meets the desired format for analysis.
- Automation and Scheduling: Tools which provide automation capabilities can significantly reduce manual workloads. Scheduling options allow businesses to set integration processes that run during off-peak hours, optimizing resource usage.
Ultimately, understanding the functionality of each tool helps businesses identify the ideal solution that aligns with their operational demands.
User Experience Evaluation
The user's experience with data integration tools greatly influences their effectiveness. A user-friendly interface can enhance productivity and decrease the learning curve. Consider these elements in user experience evaluation:
- Ease of Use: An intuitive user interface supports quicker adoption. For instance, Microsoft Azure Data Factory's drag-and-drop interface is straightforward, making it accessible for users with limited technical expertise.
- Documentation and Support: Comprehensive documentation and responsive support can facilitate appropiate integration. Tools that lack these resources can lead to frustration and slow down deployment.
- Community and Reviews: Engaging with user communities, such as on Reddit, can provide insight into real-world usability. Feedback from users often highlights common pain points and effective features that are not immediately evident.
In summary, a thorough user experience evaluation guides businesses to tools that not only meet technical requirements but also foster a smoother operational workflow.
"Selecting the right data integration tool enhances overall efficiency and decision-making capabilities in a data-driven world."
Implementation Challenges
Implementing data integration tools is not a straightforward task. Various challenges can arise during the process. Understanding these challenges is crucial for businesses looking to implement data integration effectively. Failure to address them can lead to inefficiencies and setbacks. Organizations must focus on specific elements for successful adoption. Moreover, recognizing the choice of tools plays a significant part in overcoming implementation obstacles. Here, two significant issues are outlined: data quality and complexity of integration processes.
Data Quality Issues
Data quality issues can severely hinder the effectiveness of data integration. Poor data quality leads to incorrect insights, which can affect decision-making. Data can become inconsistent, incomplete, or outdated. Businesses must ascertain that what they are integrating is reliable and relevant. This starts by auditing existing data sources. Organizations should focus on:
- Data validation: Ensure that the information being processed meets necessary standards.
- Data standardization: Establish uniform formats to enhance consistency across systems.
- Data cleansing: Remove duplicates and correct errors to improve overall data quality.
It's imperative to involve all stakeholders in the data quality journey. This promotes a culture of responsibility for data integrity across the organization. Doing so minimizes potential discrepancies that can emerge during integration.
Complexity of Integration Processes
The complexity of integration processes cannot be ignored. Data integration involves numerous systems, applications, and data silos, making it inherently complicated. Each tool has its own protocols and requirements, which can vary widely. Some factors to consider include:
- Diverse data sources: Organizations often pull from various platforms, each with different data formats. This increases integration challenges.
- Legacy systems: Older systems may not easily connect with modern tools. Upgrading legacy systems often requires significant investment.
- Real-time integration: Many organizations require real-time data processing, which adds another layer of complexity.
To address these complexities, businesses should remain focused on planning and training. Proper planning involves mapping out current data flows and designing a strategy for integration. Training staff on both the tools and processes can also alleviate burdens experienced during implementation.
Thorough understanding of integration complexity ensures smoother transitions and minimizes risks.
Epilogue and Recommendations
Understanding the conclusion and recommendations section is vital for professionals navigating the landscape of data integration tools. This part of the article synthesizes the insights gathered from previous sections while offering actionable guidance for selecting and implementing the ideal tool for specific organizational needs. The dynamism of contemporary business environments necessitates continuous adaptation, making it imperative to choose a data integration solution that aligns with both current and future demands.
Summary of Insights
Throughout the exploration of various data integration tools, several key points emerge clearly:
- Functionality: Each tool brings distinct features to the table, ranging from real-time data processing to extensive support for various data formats.
- Use Cases: The appropriateness of a tool can hinge on the use case it is intended to address. Each business has unique data challenges, influenced by factors like industry, data volume, and integration requirements.
- User Experience: Ease of use is a crucial factor for many organizations. A platform with a steep learning curve may not be feasible for teams under time constraints.
- Cost: While budget considerations are important, they should not overshadow the long-term value a robust integration tool can bring.
The attention to these elements ensures that organizations can make well-informed decisions relevant to their specific contexts.
Choosing the Right Tool
Selecting the right data integration tool involves several considerations:
- Alignment with Business Needs: Businesses must evaluate their specific integration needs. What are the sources of data? How often is the data updated? Understanding these questions helps in narrowing down options.
- Scalability and Flexibility: The tool should grow alongside the organization. Scalability ensures that the system can handle increasing data loads without a hitch.
- Support and Community: Assess available support structures. A tool supported by a robust community can provide valuable resources and troubleshooting assistance.
- Trial and Feedback: Utilizing free trials or demo versions can provide insight into how the tool performs in practice. Collecting feedback from team members can also guide the final decision.
In summary, adequate due diligence and consideration of specific needs are paramount in selecting a tool that can fundamentally enhance data management strategies.
"Choosing the right data integration tool is not just about features; it's about understanding the unique narrative of your data journey."
By utilizing this structured approach to decision-making, organizations can position themselves effectively in the data-driven landscape.
Future of Data Integration Tools
As businesses increasingly rely on data for decision-making, the future of data integration tools is becoming critical. The landscape is shifting with innovations that address the evolving needs of organizations. These tools are not just facilitators of data sharing; they are becoming intelligent solutions that adapt to dynamic business environments. This section examines emerging trends and technological advancements that will redefine data integration practices in the coming years.
Emerging Trends
The data integration field is witnessing several trends that are reshaping its future. Here are a few notable ones:
- Cloud-based Solutions: More businesses are moving to cloud environments. This shift allows for greater scalability and flexibility. Tools that enable seamless integration with cloud platforms are becoming essential.
- Real-time Data Processing: Companies are moving towards real-time data analytics. This trend demands integration tools that can process data on-the-fly, ensuring timely insights for decision-makers.
- Data Fabric Architecture: This architecture enables a unified approach to data management. Integration tools that support data fabric will allow organizations to streamline access across various sources, thereby reducing silos.
- Self-service Integration: Non-technical users increasingly need to access and manipulate data. Tools that offer self-service capabilities empower business users. This capability reduces dependency on IT and expedites data availability.
The rise of these trends illustrates the importance of evolving data integration strategies. Businesses must keep an eye on these developments to maintain competitive advantage.
Technological Advancements
In addition to trends, various technological advancements are influencing the future of data integration tools. Consider the following:
- Artificial Intelligence and Machine Learning: AI and ML are being integrated into data tools. These technologies can automate data mapping and quality checks, improving accuracy and saving time.
- API Management: As businesses leverage APIs for data access, tools that streamline API management are essential. Proper management ensures secure and efficient data sharing across applications.
- Integration Platform as a Service (iPaaS): This model provides companies with a cloud-based environment to manage their integrations. iPaaS is growing in popularity due to its cost-effectiveness and ease of use.
"The integration tools of the future will be adaptive, delivering insights instantaneously and engineered for the complexities of modern data ecosystems."
- Data Governance Enhancements: As regulations around data continue to tighten, integration tools focusing on data governance will become increasingly vital. These tools will aid organizations in complying with laws by managing data privacy and security.
These technological advancements highlight the importance of staying current with integration tool offerings. By embracing these innovations, businesses can enhance their data integration capabilities and better meet their strategic goals.