Key Trends Shaping Data Analytics for Businesses
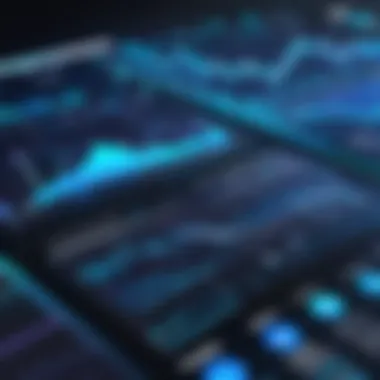
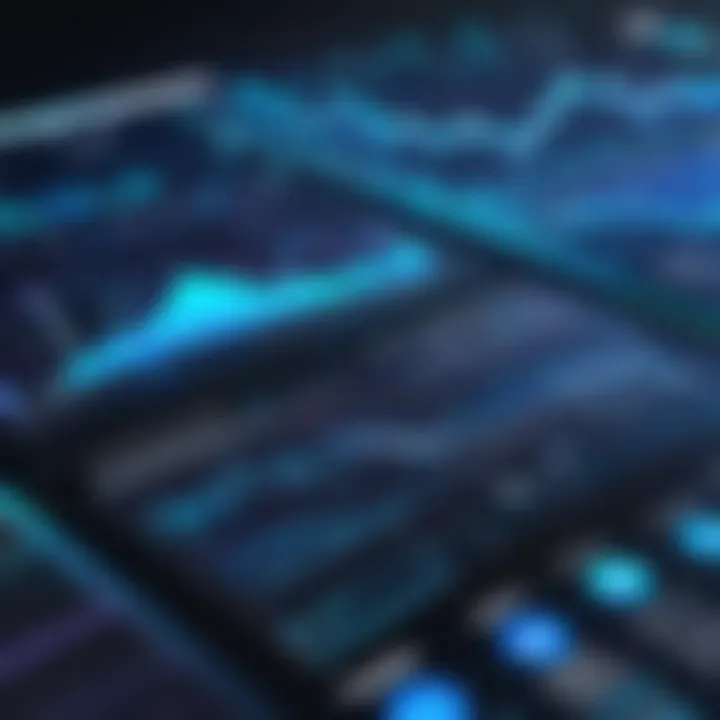
Intro
Data analytics has reached a pivotal point where the convergence of innovative technologies and the ever-growing demand for data-driven insights are shaping the very fabric of how businesses operate. It's not just about crunching numbers anymore; it's about deriving meaningful narratives from vast amounts of data. Understanding the maze of emerging trends in this realm is crucial for anyone keen on maintaining a competitive edge in today's fast-paced environment.
As organizations grapple with the increased complexity of their data landscapes, staying abreast of recent advancements becomes imperative. From machine learning to data visualization, these advancements promise to unlock new potential across various sectors. Professionals, entrepreneurs, decision-makers, and tech enthusiasts must grasp the nuances of these trends to harness their potential fully.
To effectively navigate this domain, we need a structured exploration that will inform and enlighten. Each section will dive into the trends and technologies shaping data analytics today, unpacking their characteristics and implications for the future. This strategic overview will serve as a compass, guiding readers through the fog of information to clear insights that drive innovation and informed decision-making.
Preface to Data Analytics Trends
In today's world, every decision made within a business is often backed by data. The topic of data analytics transcends mere number-crunching; it’s about tapping into the vast ocean of information that surrounds us. With the consistent growth of technology, spotting trends in data analytics isn't just a whim for some but a strategic necessity that can set companies apart from their competitors.
When we talk about the importance of data analytics trends, we dig into how companies can better predict outcomes, tailor services, and optimize operations. Understanding these trends enables organizations to stay one step ahead, capitalizing on emerging opportunities while sidestepping potential pitfalls. For decision-makers, being aware of the latest developments provides a competitive edge, something coveted more than gold in today’s digital landscape.
Definition and Scope of Data Analytics
Data analytics relates to examining data sets to draw conclusions about the information they contain. It includes various techniques and processes that can transform raw data into actionable insights. The scope of data analytics ranges from
- Descriptive analytics: Understanding past trends and patterns.
- Predictive analytics: Anticipating future outcomes based on historical data.
- Prescriptive analytics: Recommending actions based on data insights.
These dimensions showcase the diverse application of data analytics in numerous sectors. By honing in on specific analytics subsets, organizations can develop tailored strategies that meet their unique needs.
Historical Context and Development
Data analytics has come a long way from its roots in simple reporting. Back in the day, businesses relied heavily on spreadsheets, calculating numbers manually which often led to errors and inefficiencies. But the advent of the internet and advanced computing drastically altered this landscape.
The development of software tools in the early 2000s marked a significant turning point. Businesses could now analyze vast data sets with high degrees of accuracy and speed. Over the years:
- Tools like SQL gained prominence for managing databases.
- The rise of big data analytics introduced the concept of structured and unstructured data being analyzed simultaneously.
- Platforms like Tableau revolutionized data visualization, making the insights more accessible to stakeholders.
Fast-forward to today, we see the integration of artificial intelligence and machine learning algorithms, making predictions more precise than ever before. This evolution not only challenges the status quo but pushes businesses to continuously adapt or risk being left behind. Overall, grasping the historical context of data analytics helps professionals appreciate its transformative power and anticipate future advancements.
Current Trends in Data Analytics
In today’s landscape, understanding current trends in data analytics is paramount for any organization looking to stay competitive. The world is flooded with data streams, and the challenge lies in distinguishing valuable insights from the noise. Companies are increasingly realizing that, by aligning their strategies with the latest trends, they can harness the power of data to drive decision-making, tailor offerings, and enhance customer experiences. These trends not only reflect the evolution of analytics but also highlight the ongoing shift in how businesses engage with their audiences.
Increased Adoption of Artificial Intelligence
Artificial Intelligence (AI) is transforming the data analytics arena. AI technologies enable faster processing of data and provide insights that were previously unimaginable. For many organizations, the adoption of AI is not just a luxury; it has become a necessity. From automating mundane tasks to predicting future trends, AI is enhancing efficiency and effectiveness in decision-making.
- Automation of Insights: AI-driven analytics platforms can automatically detect patterns in data, allowing businesses to react swiftly to changes in their environment.
- Enhanced Predictive Capabilities: With machine-learning algorithms, predictive analytics has come a long way. Companies can now forecast customer behavior, sales trajectories, and operational needs with increased accuracy.
The importance of AI is not confined to large enterprises. Small and medium businesses are also leveraging these technologies, democratizing access to insights. As AI continues to evolve, it’s evident that businesses must incorporate it into their analytics strategies to remain relevant.
Real-Time Data Processing
The demand for real-time data processing has surged, driven by an increasingly impatient consumer base. In a world where immediate feedback is expected, organizations are racing to implement systems that allow them to analyze and respond to data as it flows in.
Real-time data processing offers several advantages:
- Faster Decision-Making: Companies can react to market changes or consumer behaviors almost instantaneously, improving their agility and responsiveness.
- Improved Customer Experiences: By analyzing customer interactions as they happen, organizations can tailor experiences in real-time, creating a more personalized approach that can significantly boost customer satisfaction.
- Risk Management: Identifying potential issues or threats at the moment they arise allows businesses to mitigate risks more effectively.
This trend is pushing industries like finance and retail to invest heavily in technologies that support real-time analytics, such as in-memory databases and complex event processing.
Cloud-Based Analytics Solutions
The transition to cloud-based analytics solutions is a game-changer for many businesses. Cloud technology provides unprecedented access to analytical tools and resources without the hefty investments in infrastructure.
Here are some of the key benefits of cloud-based analytics:
- Scalability: As data volumes grow, organizations can easily scale their analytics capabilities without significant upfront costs.
- Collaboration: Cloud platforms allow for better collaboration among teams since data can be accessed and analyzed from anywhere, fostering a culture of data-driven decision-making.
- Cost Efficiency: Moving to the cloud often translates to reduced IT maintenance costs and operational overhead, freeing up funds for other critical initiatives.
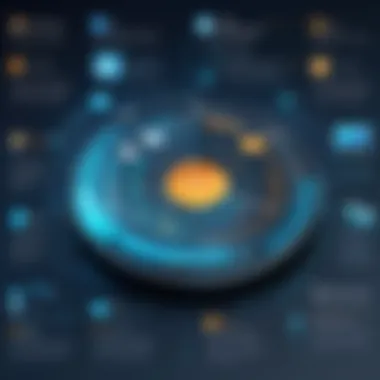
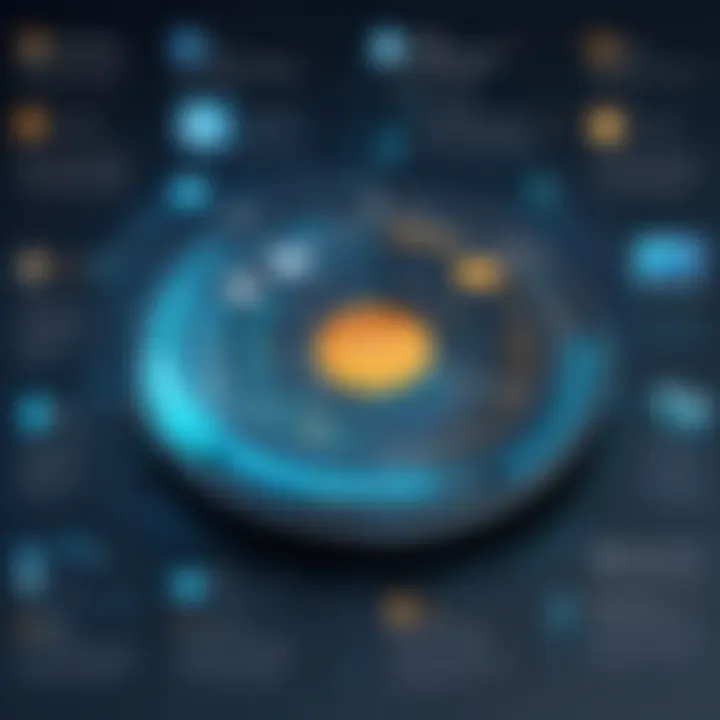
Adopting cloud solutions is no longer an option but a strategic move that aligns with the fast-paced nature of today’s business environment.
The convergence of AI, real-time processing, and cloud technology represents a pivotal moment for data analytics, shaping the future of how organizations leverage insights for strategic advantage.
The Role of Big Data in Analytics
Understanding the role of big data is paramount in grasping how organizations can tap into its potential for enhanced insights and decision-making. In an age where information burgeons at an unprecedented rate, big data not only refers to vast volumes of data but also encompasses the complexities involved in collecting, processing, and analyzing this information. Companies that master big data find themselves at a distinct advantage, driving smarter strategies and fostering innovation.
Understanding Big Data Concepts
Big data is characterized by the three Vs: volume, velocity, and variety.
- Volume refers to the enormous amounts of data generated every second. Think of social media, transaction records, sensor data from IoT devices, and much more; the simply staggering figures are dizzying.
- Velocity highlights the rapid pace at which this data is created and processed. The ability to analyze streams of data in real time allows businesses to react promptly to market shifts, customer behavior changes, or operational efficiencies.
- Variety encompasses the different types of data generated: structured, semi-structured, and unstructured. From traditional databases to social media posts and videos, organizations now must extract value from an ever-expanding array of formats.
To illustrate, consider a retail company analyzing customer interactions. They may collect data from point-of-sale systems, social media feedback, and website traffic analytics. By harnessing big data concepts effectively, the company can derive insights about customer preferences and trends, ultimately enhancing targeted marketing strategies and driving sales.
Moreover, big data analytics the playfield for advanced technologies like Machine Learning and Artificial Intelligence. As these technologies evolve, the capacity to make predictions and glean actionable insights from big data becomes more achievable.
Data Sources and Integrations
Data sources are as diverse as they are plentiful. Organizations can tap into:
- Transactional data: Data generated from sales transactions, including purchase history and inventory levels.
- Social media: Platforms like Facebook and Twitter provide valuable insights into customer sentiment, trends, and engagement.
- IoT devices: Smart devices generate continuous streams of data regarding user behavior and product performance.
- Surveys and feedback: Direct customer input can yield qualitative data on user experience and satisfaction, enhancing the quantitative data collected.
However, the efficacy of these sources hinges on how well they are integrated into an analytics strategy. Disparate data silos can render insights less impactful. Thus, a cohesive approach that unites various sources into a single analytical framework is crucial.
Data integration tools such as Apache Kafka or Talend can facilitate this process, ensuring that businesses draw from comprehensive datasets rather than isolated snippets of information.
In summary, as data volumes swell and its nature becomes more complex, professionals and decision-makers must understand the role of big data in analytics. It is the bedrock upon which informed, strategic decisions are built, guiding businesses to not just survive but thrive in the competitive landscape.
"In the data-driven age, understanding big data isn't just an option; it’s a necessity." - Data Expert
By addressing both the fundamental concepts and integration strategies of big data, organizations can pave the way for more informed policies and practices, ultimately leading to better business outcomes.
Data Privacy and Compliance Considerations
Understanding the realm of data privacy and compliance in analytics has become increasingly paramount in today’s highly digitized world. With the growing amounts of data generated and processed, having a rigorous framework that safeguards user information isn’t just good practice – it’s a necessity. Organizations tapping into data analytics without addressing these critical aspects can face significant legal repercussions and lose the trust of their customers.
Key Regulations Impacting Data Analytics
The landscape of data privacy is shaped by numerous regulations, which have evolved in response to increasing concerns about how personal information is handled. Key regulations that impact data analytics include:
- General Data Protection Regulation (GDPR): Introduced by the European Union, GDPR sets stringent rules regarding how data is collected, stored, and processed. It emphasizes the rights of individuals to retain control over their personal data.
- California Consumer Privacy Act (CCPA): This U.S. legislation provides California residents with rights related to their personal information. It gives consumers the ability to know what personal data is being collected and the purposes for which it's used.
- Health Insurance Portability and Accountability Act (HIPAA): For organizations dealing with healthcare data, HIPAA mandates strict regulations on patient information. Compliance is not just necessary but crucial to protect sensitive health data.
- Payment Card Industry Data Security Standard (PCI DSS): This standard applies to organizations that handle credit card information. It ensures that companies take the necessary steps to protect cardholder data.
Violating these regulations can not only lead to heavy fines but also damage an organization's reputation considerably. Staying compliant requires constant vigilance and an understanding of the changing regulatory landscape.
Best Practices for Data Governance
Implementing effective data governance practices allows organizations to navigate the intricate web of data privacy regulations confidently. Some recommended practices include:
- Data Classification: Understand and categorize the types of data your organization collects. This helps identify what data requires protection.
- Access Controls: Limit access to sensitive data. Only those who need specific data for their roles should have permission.
- Regular Audits: Conduct frequent audits of data practices to ensure compliance with policies and regulations. Audits reveal gaps in data handling processes.
- Employee Training: Educate staff about compliance requirements and the importance of data privacy. A well-informed team can be the first line of defense in protecting sensitive information.
- Incident Response Planning: Develop a robust incident response plan that outlines steps to take in case of a data breach. Swift action can mitigate damage.
"Failing to prepare is preparing to fail."
By threading these practices into the very fabric of your organization’s culture, businesses not only protect themselves from external legal threats but also foster a sense of trust among their clients. In a landscape increasingly influenced by data, this trust is invaluable.
One misstep can lead to perceptions of negligence and result in losing customer loyalty and potential revenue. Organizations that prioritize data privacy and compliance not only safeguard their operations but place themselves ahead in the competitive digital marketplace.
Emerging Technologies Influencing Data Analytics
The field of data analytics is not stagnant; it thrives on innovation, particularly through emerging technologies. These advancements play a pivotal role in shaping how organizations harness data to drive insight and efficiency. By integrating methodologies from various tech realms, businesses can not only enhance their analytics capabilities but also reap substantial benefits like improved decision-making and enhanced operational efficiency. The increasing interdependence of data analytics with new technologies cannot be overstated. It is fundamentally changing the landscape for professionals across industries.
The Rise of Machine Learning Techniques
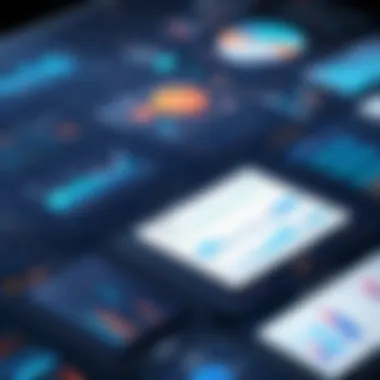
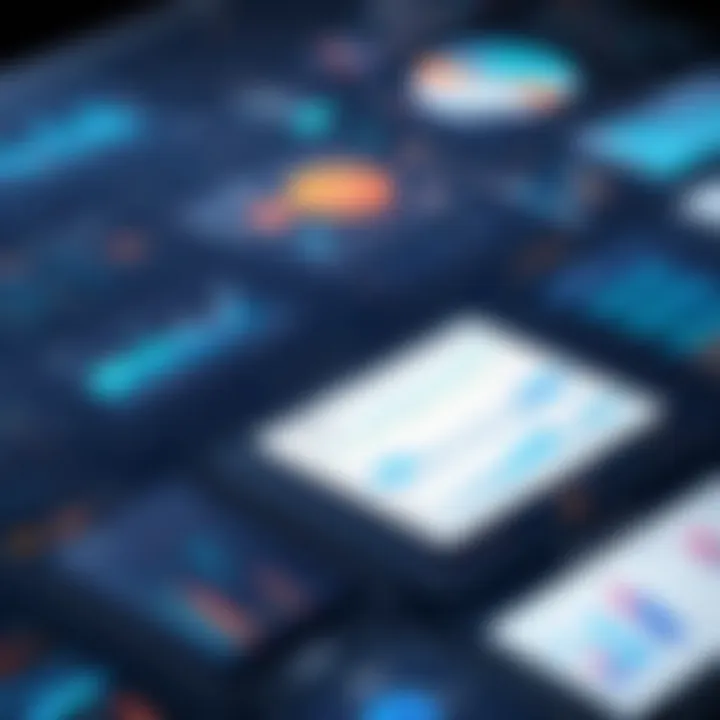
Machine learning has surged into the spotlight in recent years, becoming a cornerstone of modern data analytics. This technique enables systems to learn from data patterns and make decisions with minimal human intervention. From predictive modeling to anomaly detection, machine learning techniques offer powerful tools to interpretation of complex datasets.
- Enhanced Predictions: Machine learning algorithms can analyze vast amounts of data quickly. They excel at identifying trends and forecasting future outcomes, allowing companies to be a step ahead in their strategy planning.
- Automation of Processes: With the capability to adjust algorithms as new data flows in, businesses can automate many decision-making processes that were once manual. This enhancement not only saves time but also reduces errors, leading to better outcomes.
- Personalization: Firms leverage machine learning to personalize customer experiences, which is increasingly vital in the age of information overload. Companies like Netflix use these techniques to recommend shows based on viewing history, exemplifying how analytics can elevate customer satisfaction.
- Cost-Effective Operations: By optimizing processes through machine learning, organizations often find substantial cost reductions. This efficiency is a direct result of leveraging algorithms that automate time-consuming tasks.
Blockchain and Data Integrity
Blockchain technology introduces a new dimension to data integrity and security in analytics. Its decentralized nature ensures that data is not only more secure but also verifiable across various stakeholders without relying on a single point of failure. This is highly relevant as organizations grapple with the challenges of cybersecurity and data fraud in today’s digital age.
Benefits of incorporating Blockchain with Analytics:
- Increased Transparency: Blockchain creates an immutable record of all actions taken on data. This aspect promotes trust among stakeholders who can navigate the data trail with confidence.
- Enhanced Security: By encrypting data on a blockchain, the risk of data breaches is significantly reduced. Hackers face substantial hurdles when trying to alter data registered across multiple nodes.
- Improved Compliance: Many industries face strict regulations regarding data handling. With blockchain, organizations can maintain auditable records that are crucial for compliance with laws such as GDPR.
- Speedy Transactions: Smart contracts on a blockchain can automate verification processes, leading to faster transaction processing times, which is invaluable, especially in finance.
"By integrating machine learning and blockchain with data analytics, companies position themselves not just to survive, but to thrive in an increasingly data-driven world."
In summary, the integration of emerging technologies such as machine learning and blockchain into data analytics is not merely a trend—it's a fundamental shift that paves the way for more sophisticated and reliable data handling. Companies that embrace these technologies gain a competitive edge, better addressing customer needs and enhancing operational outcomes.
Industry Applications of Data Analytics
The practical use of data analytics has transcended mere categorization into trendy buzzwords; it is now fundamentally reshaping various industries. As organizations navigate a rapidly evolving marketplace, the application of data analytics offers not only insights but also actionable strategies for enhancing operational efficiency, boosting customer relations, and ultimately, driving profit. Businesses that can recognize and harness these applications position themselves ahead of the curve, benefiting from data-informed decision-making that mirrors the real-time dynamics of their industries. Let’s dive into how specific sectors leverage data analytics to create remarkable advancements.
Healthcare: Predictive Analytics for Patient Care
In healthcare, predictive analytics serves as a game changer for patient care management. Hospitals and clinics employ data derived from patient history, demographic information, and even genetics, allowing clinicians to predict potential health risks and outcomes more accurately. For example, an analysis of past hospitalization data can illuminate trends that lead to preventative measures being established before patients even display symptoms.
Healthcare providers can optimize resource allocation as well. When predictive models indicate increased likelihood of specific health conditions, hospitals can ensure that more staff is on hand or necessary supplies are stocked accordingly.
- Benefits of Predictive Analytics in Healthcare:
- Enhanced patient outcomes through early intervention
- Reduced readmission rates, saving costs
- Efficient use of hospital resources
By utilizing predictive analytics, healthcare professionals not only improve care but also manage costs effectively while ensuring compliance with regulations and guidelines.
Finance: Risk Management and Fraud Detection
The financial sector has always been at the forefront of data analytics adoption. From detecting fraudulent transactions in real-time to managing risk across diverse portfolios, analytical tools have transformed the landscape of finance.
Fraud detection systems analyze transaction patterns, using algorithms to flag any irregularities that could signal fraudulent activity. This isn’t just about cutting losses; it enables companies to build trust with customers by swiftly addressing potential breaches.
- Data Analytics in Finance:
- Real-time fraud detection
- Risk assessment for lending decisions
- Regulatory compliance monitoring
As companies face increasing regulatory pressures, data analytics provides the necessary tools to meet compliance requirements while securing their assets.
Retail: Personalization and Customer Insights
In the retail sphere, leveraging data analytics leads to highly personalized shopping experiences. Retailers can analyze customer buying habits, preferences, and feedback to tailor recommendations and marketing strategies that resonate with specific demographic segments. This targeted approach not only enhances customer satisfaction but also drives sales directly.
- Applications of Data Analytics in Retail:
- Personalized marketing campaigns based on user data
- Inventory management through sales forecasting
- Customer segmentation for nuanced targeting
Moreover, as consumers engage with brands across multiple platforms, integrating data can provide a unified view of the customer. This synergy allows retail brands to create a seamless shopping experience, fostering loyalty and repeat business.
"The power of data analytics is not just in the volume of information but in the insightful conclusions that organizations can draw from it."
Challenges in Data Analytics Implementation
Data analytics can seem like a golden ticket for organizations aiming to make sense of their data. Yet, the path to effective implementation is often strewn with challenges that can trip up even the most prepared teams. These hurdles can have a significant impact on the overall success of data-driven initiatives, influencing not just the technical aspects, but also the culture of decision-making within the whole organization.
Data Quality and Accuracy Issues
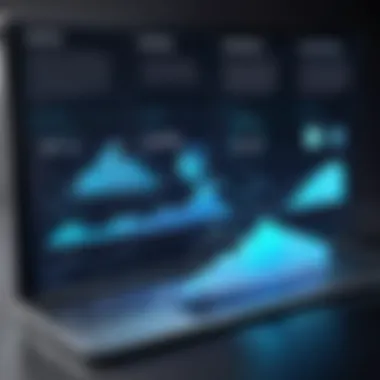
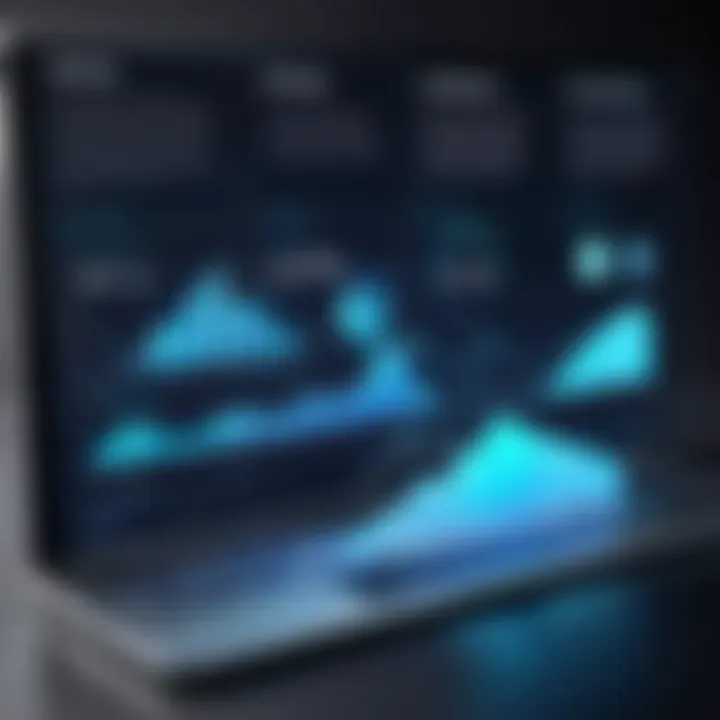
One of the first stumbling blocks in data analytics is the ever-present issue of data quality. You can have all the advanced software in the world, but if the data you feed into it is riddled with errors, the output will be flawed. This situation can arise from various sources, including human error during data entry, outdated information, or inconsistencies across different data systems.
- Consequences of Poor Data Quality:
- Misleading insights can lead decision-makers to travel down the wrong road.
- Financial losses could occur if untrustworthy data influences budgeting or resource allocation.
- The team’s trust in analytics might erode. When employees observe that data-driven conclusions frequently miss the mark, skepticism can take root.
Ensuring data accuracy is essential; organizations should adopt robust data validation processes. Regular audits, automated quality checks, and integrating data from reliable sources can enhance the integrity of their datasets. A culture that emphasizes accuracy over speed is crucial. Strong data governance practices can provide the necessary framework to manage these complexities effectively.
Skill Gap in Data Literacy
As organizations embrace data analytics, they often hit a wall due to a gap in data literacy among their staff. Not every employee is born with the ability to interpret complex datasets or generate insights from them. This limitation can be detrimental. It creates a bottleneck, where skilled data analysts or data scientists become overwhelmed by requests for analysis from various departments, resulting in delays and frustration.
- Factors Contributing to Skill Gaps:
- Rapid changes in technology outpacing training programs.
- The misconception that data analytics is only for specialized roles, rather than a fundamental skill for many positions.
- Companies often neglect continuous education and professional development in their analytics strategies.
To bridge this gap, organizations must prioritize data literacy training. Workshops and seminars focusing on practical applications of data can empower employees, from C-suite executives to entry-level staff. Moreover, encouraging a data-centric mindset across all levels can foster collaboration and innovation, leading to a rich pool of insights and better decision-making.
"The most important thing is not the data itself but how people interpret and use it."
– Anonymous
While challenges in data analytics are formidable, they are not insurmountable. By addressing data quality and investing in data literacy, companies can position themselves to leverage the full power of their data. Understanding these hurdles is the first step in overcoming them, allowing organizations to thrive in a data-driven world.
Future Directions in Data Analytics
The content landscape in data analytics is shifting at breakneck speeds, fueled by both advancements in technology and evolving business needs. Understanding future directions within this field is no longer optional; it's a strategic necessity for professionals navigating an increasingly data-centric world. This section will delve into two pivotal areas: the impact of quantum computing and how predictive analytics is reshaping decision-making processes for organizations.
The Impact of Quantum Computing
Quantum computing, while still in its infancy, holds immense potential to transform data analytics. Unlike traditional computers that process information using bits, quantum computers utilize qubits, enabling them to perform calculations at lightning speed.
- Why Quantum Matters: The implications of quantum computing in data analytics are profound. These systems can tackle data sets that would take current supercomputers years to process, facilitating real-time insights on an unprecedented scale. For instance, organizations that depend on modeling complex scenarios, like climate change or financial markets, could benefit from vastly improved speed and accuracy.
- Industry Changes: Areas such as pharmaceuticals are already exploring quantum algorithms to enhance drug discovery. Companies like IBM and Google are at the forefront, experimenting with quantum machine learning frameworks that could redefine the boundaries of data science.
"As quantum computing continues to evolve, businesses not yet considering its potential risks being left behind."
However, it’s crucial to be mindful of the challenges that accompany this technology. Security concerns and the need for specialized skills to manage quantum data are just some hurdles that organizations must navigate.
Predictive Analytics and Decision Making
Predictive analytics is one of the defining characteristics of modern data analytics. It is not just a buzzword but a game-changer for how decisions are made in the business arena. By employing statistical algorithms and machine learning techniques, businesses can analyze historical data to discover patterns and make forecasts about future events.
- Enhanced Decision-Making: Through predictive analytics, organizations gain clarity on customer behavior, market trends, and potential risks. This information can guide strategic decisions—whether it’s optimizing supply chains, launching targeted marketing campaigns, or assessing credit risk in finance. Take Netflix, for example; their recommendation engine utilizes predictive analytics to tailor content suggestions, significantly boosting user engagement.
- Example Use Cases: Here are some practical implementations of predictive analytics in diverse sectors:
- Healthcare: Predicting patient admissions and optimizing staffing in hospitals.
- Retail: Anticipating stock needs based on seasonal demand fluctuations.
- Manufacturing: Predicting machinery failures to minimize downtime.
In today’s competitive landscape, decision-makers who leverage predictive analytics effectively will have a distinct advantage. Data is plentiful, but insights derived from it—tailored to specific strategic needs—are what drive innovation and growth.
Epilogue
The conclusion of this article dives into the critical importance of understanding emerging trends in data analytics, especially as we navigate an increasingly complex digital landscape. As data continues to swell in volume, variety, and velocity, the insights from analytics not only become invaluable but also transformative for organizations across various sectors.
Summary of Key Insights
In synthesizing the information presented throughout this piece, several core insights stand out:
- Artificial Intelligence: The integration of AI is reshaping how businesses analyze data, shifting from traditional methods to predictive analytics that can forecast trends and inform strategy.
- Real-Time Processing: Organizations are moving towards real-time data analysis, enabling swift decision-making and fostering a proactive approach in competitive markets.
- Cloud Solutions: The adoption of cloud-based analytics platforms is growing, offering scalability and flexibility that on-premise solutions simply cannot match.
- Data Privacy: With new regulations emerging, businesses must prioritize data governance to build trust and reduce compliance risk.
- Emerging Tech: Technologies such as machine learning and blockchain are carving out new pathways in analytics that emphasize security and accuracy.
These insights underline the pressing need for organizations to not only adopt these trends but to actively embrace a culture of data-driven decision-making. The ability to leverage data effectively can mean the difference between leading the pack or falling behind.
Encouragement for Business Leaders
As we conclude, it's essential for business leaders to recognize the vast potential that data analytics has to offer. Investing in analytics capabilities isn't merely a competitive advantage; it's becoming a prerequisite for survival. Here are a few considerations for leaders:
- Foster a Data-Driven Culture: Encourage teams to view data as a crucial asset rather than just a byproduct of operations. Training programs and workshops can effectively bridge the skills gap in data literacy.
- Stay Ahead of Regulations: Compliance isn't a checkbox; it's an ongoing commitment. Regularly updating policies and procedures in line with evolving regulations can protect your organization from significant risks.
- Invest in Technology: Consider newer technologies that enhance data integrity and security, like blockchain. Adopting the latest innovations can place your organization at the forefront of the industry.
"The secret of success is to be ready when your opportunity comes." - Benjamin Disraeli
In summary, embracing these emerging trends allows business leaders not just to meet the current demands but to anticipate future challenges effectively. The path forward is clear—data analytics is not just for tech teams but should be integral to every decision made within an organization.
By holistically incorporating these strategies and insights, organizations can harness the full power of data analytics. This will not only foster innovation but lead to sustainable growth and success in the digital age.