The Critical Role of Data Fabric in Modern Business
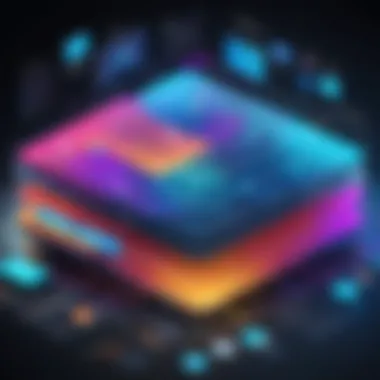
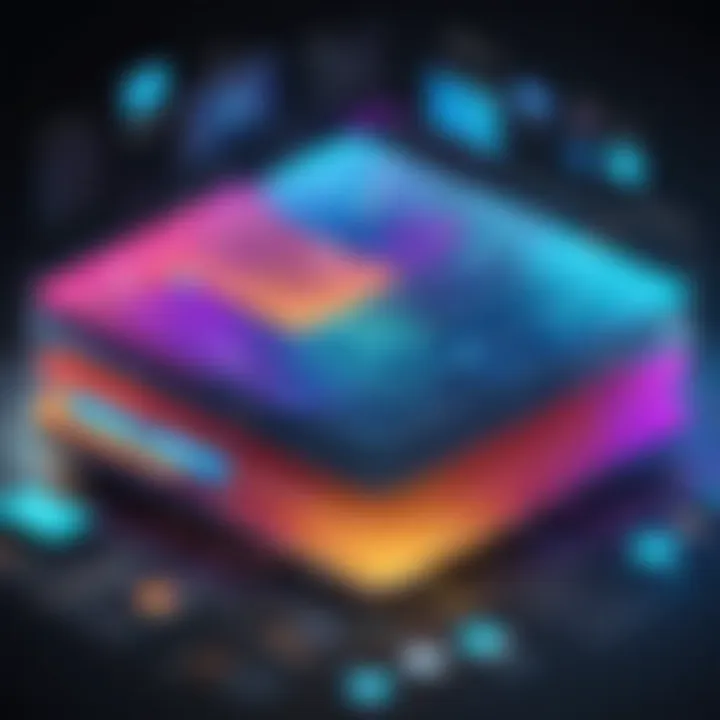
Intro
In today's digital economy, data is at the core of every operational process. Enterprises find themselves overwhelmed with the immense volume of data generated daily. The need for a comprehensive data management framework is clearer than ever, and this is where data fabric becomes essential. By providing a unified architecture for data management across an organization, data fabric offers improved accessibility and more efficient handling of data. Below, we will explore its significance in the current business landscape.
Technological Research Overview
Recent Technological Innovations
Innovations in data management technology have paved the way for data fabric to emerge as a viable solution. Modern businesses now leverage big data technologies like Hadoop and cloud storage options from providers such as Amazon and Google. These tools support seamless data integration from multiple sources. Furthermore, advancements in artificial intelligence allow for enhanced data analysis, creating patterns and spotting trends much more effectively than traditional methods.
Impact on Business Operations
Data fabric streamlines processes within organizations, allowing fast access and concise analysis of data. Many organizations report improved decision-making capabilities. By integrating disparate data sources, businesses develop a clear, unified view of their information landscape. This capability leads to improved internal communications and better coordination of projects. In summary, data fabric effectively transforms chaotic data into actionable intelligence closer to real-time.
Future Technological Trends
Looking forward, several trends are overtaking the technological landscape regarding data fabric. The arrival of edge computing emphasizes local data processing, reducing latency issues and empowering real-time analytics. Another significant trend is the increasing focus on data governance, ensuring compliance while maximizing value extraction from data assets. All these trends suggest data fabric's role will only become more critical for businesses aiming to compete in this fast-paced environment.
Data Analytics in Business
Importance of Data Analytics
As businesses continue to embrace digital transformation, data analytics stands out as a crucial factor for organizational success. It enables firms to make informed decisions, identify new opportunities, and improve operational efficiency. Companies that effectively use data analytics can gain deeper insights for better strategic planning.
Tools for Data Analysis
Numerous tools exist for analyzing data effectively. Popular options include Tableau for visual analytics, SAS for advanced analytical processes, and Google Analytics for digital marketing insights. These tools facilitate real-time access to business intelligence, enabling organizations to react swiftly to market changes.
Case Studies on Data-Driven Decisions
Case studies have demonstrated how data analytics can vastly improve decision-making.
- Coca-Cola: Their use of customer data helped unanimous optiomization of their marketing strategies, resulting in higher engagement.
- Netflix: Personalization through data analysis has significantly boosted customer satisfaction and retention rates.
Examining such real-life implementations illustrates data analysis's transformative effects on businesses.
Cybersecurity Insights
Threat Landscape Analysis
With an increase in cyber threats, businesses must stay secure while managing data. The threat landscape currently features sophisticated attacks, including ransomware, phishing, and data breaches. Awareness and preparedness are vital for surviving these challenges.
Best Practices for Cybersecurity
Organizations should implement robust cybersecurity practices. Effective practices include regular security audits, employee training, and multi-factor authentication. Monitoring data access actively and pemitting timely incident response plans should also be part of a comprehensive cybersecurity strategy.
Regulatory Compliance in Cybersecurity
Businesses increasingly face scrutiny regarding their data security measures. Compliance with regualtions such as GDPR and HIPAA guarantees that user data is appropriately protected. Ensuring compliance also reduces legal risks while fostering consumer trust.
Artificial Intelligence Applications
AI in Business Automation
Artificial Intelligence is becoming intergral to automating business processes. This reshapes workflows, allowing companies to better allocate human resources to high-impact tasks. Applications vary from chatbots for customer support to predictive analytics for sales management.
AI Algorithms and Applications
A variety of algorithms are in the forefront, ranging from basic linear regressions to sophisticated neural networks. Each serves purpose which helps enhance product offerings and operational efficiency. Conversely, an understanding of their limitations and challenges captures a well-rounded perspective of AI in neocompanie's arsenal.
Ethical Considerations in AI
The rise of AI brings forth urgent ethical considerations, including bias in algorithms and accountability for decision-making processes. Such challenges present brewing issues that urge organizations to adopt ethical frameworks and ensure fairness in AI applications.
Industry-Specific Research
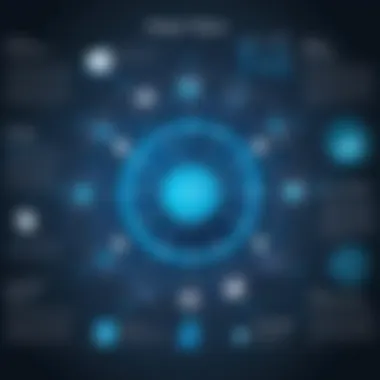
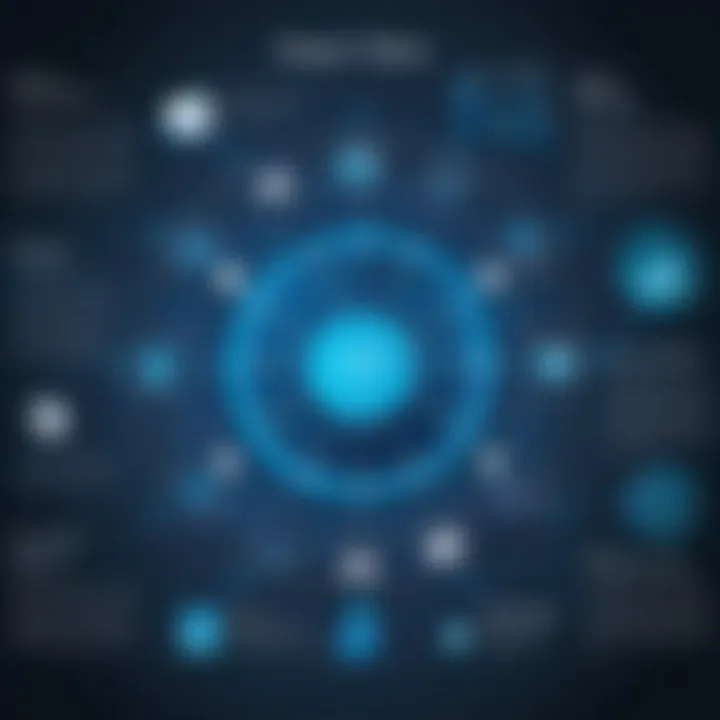
Tech Research in Finance Sector
The finance industry has witnessed substantial changes through technology, particularly with Artificial Intelligence and blockchain optimization. Data fabric optimizes access to data across direputable domains aiding meticulous decision-making making this area global competitive.
Healthcare Technological Advancements
Data fabric plays a vital role in the healthcare sector where patient data must seamlessly flow between systems while remaining compliant with regulations. Integrated data results in improved patient care and operational management, and illustrates potential predictive analytics efficiency.
Retail Industry Tech Solutions
In retail, firms utilize data fabric to fine-tune inventory management, study customer behavioral trends, and enhance the user shopping experience. Emphasizing real-time analysis leads retail organizations to become increasingly nimble and responsive to their clients' evolving preferences.
By establishing a data fabric, businesses can pursue opportunities for refinement, efficiency, and growth GP beyond today's competitive challenges.
The above sections are foundational to grasping the complexities surrounding data fabric and its place in our increasingly data-centric world.
Prolusion to Data Fabric
Understanding data fabric is crucial in today's business environment. Companies are bombarded with vast amounts of information from multiple sources. The need for a cohesive structure to manage and interpret this data efficiently has never been more significant. Data fabric provides such a framework.
This concept goes beyond mere data storage. It enables organizations to establish a comprehensive view of their data landscape. This abstraction allows data to flow between systems and departments seamlessly, improving collaboration and speeding up decision-making processes. Furthermore, data fabric supports diverse environments, including on-premise, cloud, and hybrid solutions, making it adaptable to various organizational needs.
In exploring this topic, we shall define what data fabric is, track its historical evolution, and examine its current significance in facilitating effective data management across industries. By understanding these elements, businesses can better leverage data fabric for enhanced productivity and competitive advantage.
Defining Data Fabric
Data fabric refers to a unified architecture that allows the integration, management, and sharing of data across different environments and sources. Its core principle revolves around enabling data accessibility regardless of the location or format it resides in. In simpler terms, it is a strategy that eliminates data silos, connecting disparate systems and datasets. This approach empowers companies to have a holistic view of their data assets.
Data fabric incorporates various technologies like data lakes, data virtualization, and API management to facilitate seamless data integration and accessibility. This capability is vital as businesses increasingly rely on analytics to derive insights from data. With data fabric, large enterprises can make more informed decisions, reducing the reliance on dependent legacy systems, which often hinder flexibility and resilience.
Historical Context and Evolution
The concept of data fabric can trace its roots back tow and possibly thee decades ago, as businesses began to realize the limitations of traditional data management systems. Initial approaches primarily focused on centralizing data in large databases, which were often expensive and lacked scalability. These methods were less adaptable to new challenges presented by technological advancements.
With the rise of cloud computing and big data, the paradigm shifted towards more flexible and decentralized structures. Organizations required solutions that could consolidate data from varied origins, enhance analytics capabilities, and support activities in real-time. As a result, data fabric has evolved to address integration issues and accommodate the growing complexities associated with large-scale data environments. Today, data fabric is not only a reaction to these challenges but also a proactive strategy that companies adopt to stay competitive in rapidly changing marketplaces.
The Architecture of Data Fabric
The architecture of data fabric stands as a vital framework central to integrated data management in modern organizations. This architecture underpins the how and why of data access, enabling diverse data sources to communicate effortlessly. Understanding the architecture is essential because businesses face ever-increasing complexity in their data environments. Some organizations might have data stored in cloud services, local servers, or even in edge devices. Therefore, a clear architectural overview aids in comprehending how bits and pieces connect together deterministically.
Key benefits arise from a solid data fabric architecture, allowing organizations to pull, integrate, and analyze data without barriers. It is a foundational aspect that accommodates both current requirements and future scalability. In this evolving landscape, the architecture also supports agility, fostering rapid responses to changing market conditions.
Core Components of Data Fabric
Core components are the pillars upon which data fabric stands. They mold the flow of information, ensuring seamless transitions between systems. These components typically include data sources, connectors, and orchestration layers. Each of them serves a designated purpose, creating an interconnected system that enhances data accessibility.
- Data sources are where the data lives, such as databases, applications, or third-party services. Identifying these sources accurately is paramount.
- Connectors are tools that enable access to these data sources. They standardize the communication across different formats and protocols.
- Orchestration layers manage the flow and execution of data processes. They orchestrate when, how, and where data moves, ensuring operational efficiency.
Ensuring this cohesive interaction within the architecture means businesses experience improved operational function and user accessibility, along with enhanced data visibility throughout.
Data Integration Layers
The data integration layer is another cardinal structure in the data fabric architecture. This layer takes on the essential function of integrating disparate data into a harmonized output that businesses can utilize effectively. Lacking an efficient integration layer results in siloed data, which impairs decision-making and responsiveness.
Multiple strategies are utilized in data integration, among them ETL (Extract, Transform, Load) and ELT (Extract, Load, Transform). Here’s what differentiates them:
- ETL involves extracting data, transforming it for analysis, then loading it into the storage system. This is a more traditional approach characteristic of data warehousing
- ELT skips initial transformation, loading raw data into the target system, with transformation occurring afterwards. This strategy is favorable in cloud-based systems, allowing deeper, more flexible insights.
The ultimate goal of these integration layers is to create a unified consequently usable dataset. Decisions made based on integrated data are typically more informed and reliable, aligning with modern businesses' imperative of data-driven decision-making.
Integrating data from multiple sources allows companies to gain comprehensive insights and enhance overall operational efficiencies.
The combined efforts of the core components and the integration layers embed data fabric into organizations effectively, shaping its architectural backbone and fortifying its potential for superior data management.
Key Drivers for Adopting Data Fabric
Businesses today grapple with how to harness vast quantities of data. The central feature driving organizations toward implementing data fabric is multifaceted. Companies are increasingly recognizing that a data fabric can provide vital solutions. These include addressing data volume, variety, and the necessity for immediacy in access. Below, the two primary drivers that signify why adopting data fabric is no longer optional are unpacked.
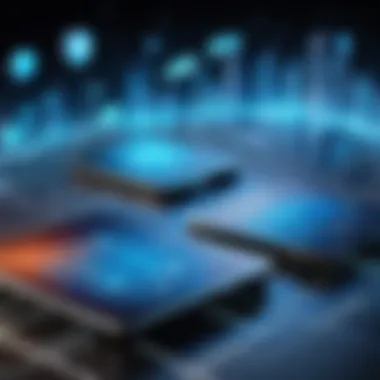
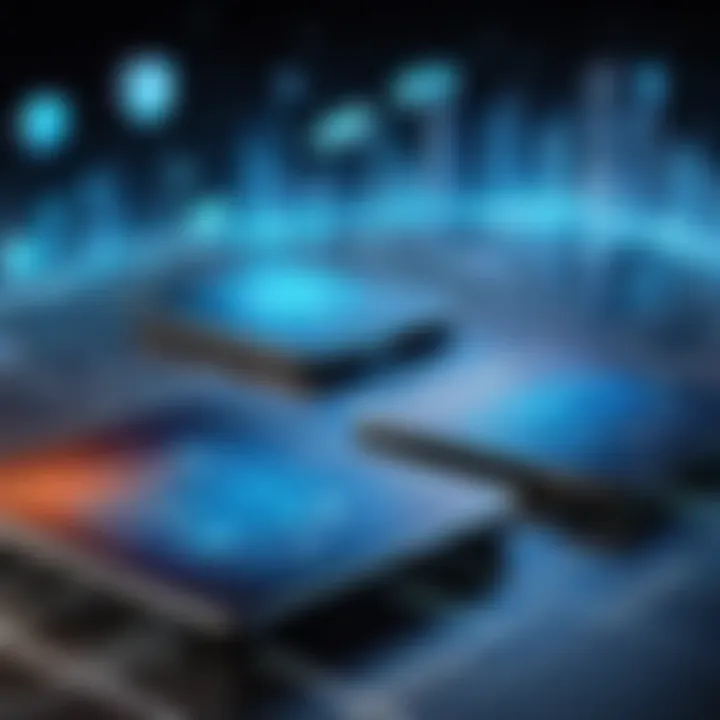
Increasing Data Volume and Complexity
The digital realm unleashes an unpredictable tide of data. Every interaction, decision, and operation generates more information. People create over 2.5 quintillion bytes of data daily. Emerging technologies additionally enhance this trend as they proliferate data sources. From social media posts to IoT devices, the complexity is staggering.
With such a plethora of unstructured and structured data, businesses face challenges in managing and utilizing information. Data fabric provides a solution. It enables organizations to streamline data integration and management across their system. By offering a cohesive structure, data fabric assists in transforming diverse data sources into unified insights.
Data analytics, driven by data fabric architecture, not only assists in processing extensive data but also enhances the quality of outcomes. By using sophisticated algorithms, businesses view data context clearer. They can adjust their operational strategies to meet market demands effectively.
Utilizing data fabric allows organizations to redefine their data strategy, turning previously untouchable data into actionable insights.
Demand for Real-Time Access
Today's market demands rapid decisions. Companies that leverage real-time analytics tend to gain a competitive edge. Conventional methods, often involving meta-layers of software, lead to delays in data processing and impeding timely decision-making. Fast-paced environments benefit from instant access to data—the crux of operational success.
Data fabric provides the infrastructure for this requirement. It lying at the intersection of speed and efficiency. Integration of advanced analytics into data fabric allows each user voice, in real-time, to respond to navigational changes in dynamics. Immediate acess to actionable insights can dictate the competitive advantage of an organization in a fast-moving market.
The skills to leverage data insights come at both human and technological dimensions and rely on firm dynamics. A framework supported by a data fabric not only grants access to real-time insights but also ensures the validity and integrity of that data, leading to informed decisions.
Organizations pivot with agility in trade leadership when data access aligns directly with data analysis actions. Embracing data fabric naturally eases strategic goals allowing innovative thinking to align perfectly with execution.
Benefits of Data Fabric for Businesses
The adoption of data fabric is reshaping how businesses manage and leverage their data. In the realms of information technology, data access has become a pivotal factor influencing operational effectiveness and strategic outcomes. Understanding the benefits inherent to data fabric provides businesses with the insight to streamline operations, enhance productivity, and capitalize on opportunities.
Enhanced Data Accessibility
Accessibility of data is a cornerstone for businesses striving to maintain competitive advantages in their sectors. Data fabric provides a unified framework for managing data across multiple platforms. This integrated approach allows organizations to break down silos, making data available to all stakeholders who need it. This democratization of data reduces redundancies, facilitates faster decision-making, and empowers teams.
Key aspects of enhanced data accessibility include:
- Real-Time Access: Users can obtain real-time insights, allowing for more agile responses to changing business conditions.
- Interoperability: Diverse data systems can communicate effectively, fostering innovation in business processes.
- Self-Service Capabilities: Employees are empowered to extract relevant data without needing extensive IT intervention, thereby increasing efficiency.
As data accessibility improves, organizations can drive engagement and collaboration among teams, significantly improving performance metrics.
"Harnessing enhanced data accessibility can create strategic benefits including operational efficiency and improved innovation cycles."
Improved Decision-Making Processes
Effective decision-making processes rest on solid data foundations. Data fabric supports intelligence-driven decisions by organizing and structuring data seamlessly. By converging disparate data streams into a single, searchable repository, companies obtain a clear view of their operational landscape. This holistic perspective enables informed decisions backed by solid analytics.
Elements that contribute to improved decision-making processes include:
- Data Consistency: A unified view ensures consistency in data interpretation, minimizing errors and misunderstandings.
- Predictive Analytics: The integration of advanced analytics technologies, like AI and machine learning, aids businesses in anticipating market trends and customer preferences.
- Collaborative Insights: Teams have access to a shared data environment, promoting collaborative decision-making and extensive knowledge sharing.
By investing in a robust data fabric architecture, businesses can expect notable improvements in execution, aligning operational strategies with their overarching business goals. Overall, the significance of data fabric often extends beyond foundational benefits, fundamentally changing how organizations interpret and interact with their data.
Challenges in Implementing Data Fabric
The implementation of a data fabric architecture does not come without its share of challenges. Organizations need to approach these difficulties with a clear understanding, as they can hinder the expected benefits of enhanced data integration and accessibility. In this section, we will explore two significant challenges: integration with legacy systems and data governance and compliance issues.
Integration with Legacy Systems
Many organizations rely on legacy systems, which often impede the transition to a data fabric model. These older systems may contain valuable historical data that is difficult to integrate into modern data architecture. Compatibility issues can arise due to outdated technologies or structures that do not align with contemporary data integration strategies.
Organizations must consider the following factors:
- Cost and Resources: Upgrading or replacing legacy systems can be an expensive undertaking. This includes investment in new technology, potential downtime, and training staff.
- Data Migration: Migrating data from older systems to a more modern data fabric architecture poses risks, such as data loss or corruption. Extensive planning is necessary to mitigate these risks.
- Resistance to Change: Employees familiar with legacy systems might resist transitioning to new technologies. Change management is crucial in guiding staff through this process.
Ultimately, addressing these integration challenges can mean balancing modernization while ensuring that valuable data is not lost or compromised. Smooth integration is key to a successful data fabric implementation.
Data Governance and Compliance Issues
Data governance is fundamental when implementing data fabric, especially in regulated sectors such as finance and healthcare. Ensuring compliance with data protection laws can become complex when integrating dispersed data sources.
Key challenges often include:
- Data Privacy Regulations: Companies must navigate complex legal frameworks such as the General Data Protection Regulation (GDPR) or the Health Insurance Portability and Accountability Act (HIPAA). Organizations need processes for data access, usage, and consent.
- Data Quality: Insight from a data fabric is only as good as the data it uses. Poor data quality can lead to misleading interpretations. Governance structures must be put in place to ensure data remains consistent, accurate, and accessible across the organization.
- Stakeholder Alignment: The involvement of various stakeholders in decision-making can also complicate compliance. Clear policies and communication are needed to ensure consensus.
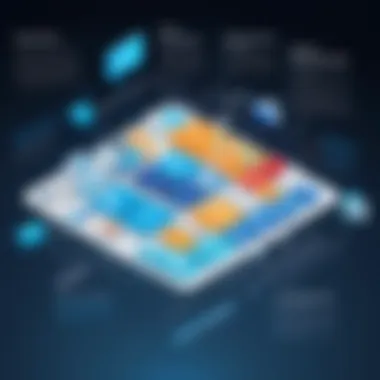
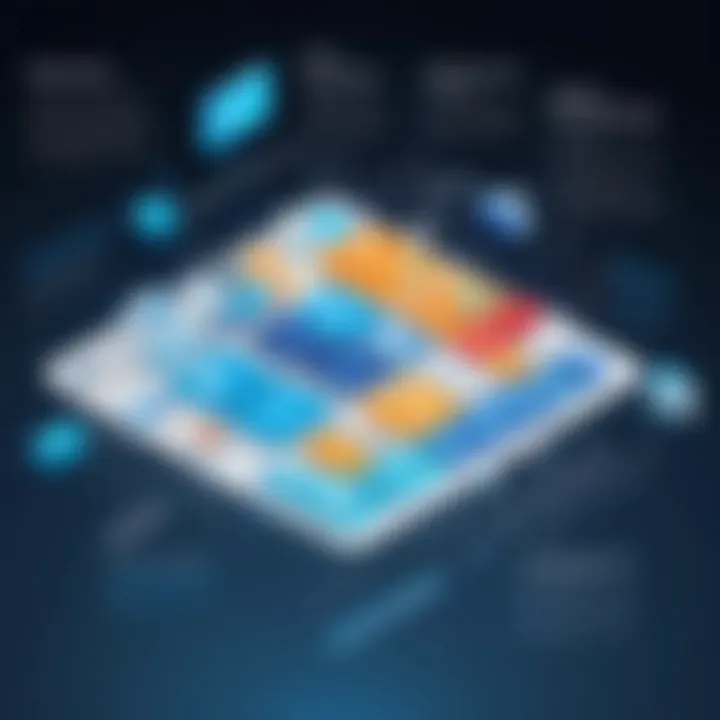
Effective data governance promotes trust and can improve the overall decision-making processes within an organization.
Addressing governance issues during the implementation phase can streamline future activities by reducing the time spent on compliance audits and adjustments. Organizations should view these challenges not merely as obstacles but as opportunities to tighten control over their data environments.
Use Cases of Data Fabric in Various Industries
Data fabric is increasingly crucial in managing data across different sectors. By streamlining data integration and ensuring accessibility, organizations can strategically leverage their data resources. Understanding the specific use cases of data fabric helps businesses identify potential benefits and consider applications in their contexts. This not only drives operational efficiency but also supports innovation across diverse industries.
Healthcare Sector Applications
In the healthcare industry, data fabric facilitates the integration of varied data sources. Hospital systems, laboratories, and insurance providers generate data in siloed formats. Data fabric allows healthcare providers to break down these siloes, enabling a more unified data view. This is important for improving patient outcomes and operational efficiencies.
A few key aspects include:
- Interoperability: Data fabric enhances communication and data sharing among disparate systems often used by providers, pharmacies, and labs. This capability boosts collaborative care efforts.
- Real-Time Data Access: Clinicians need quick access to patient information. A data fabric architecture can streamline access to electronic health records and up-to-date lab results, allowing healthcare professionals to make informed decisions rapidly.
- Data Analytics: With vast amounts of data generated, robust analytics become essential. Data fabric helps in creating comprehensive datasets that improve population health management strategies and enhance predictive analytics for disease outbreaks.
Healthcare organizations increasingly recognize that implementing a data fabric approach can be a transformative step that advances clinical research and patient care. Maintaining compliance with healthcare regulations also benefits from structured data management across platforms.
The adoption of data fabric can significantly enhance patient care, operational efficiency, and data governance in the healthcare sector.
Financial Services Innovations
The financial services sector has its own distinct requirements for data management. Data fabric provides a framework that modernizes legacy systems while ensuring robust data governance. As regulations and customer expectations evolve, financial institutions increasingly benefit from flexible data architectures.
Key innovations include:
- Risk Management: Financial decisions rely heavily on accurate data. Data fabric structures multiple sources for more accurate assessments, thus improving risk management.
- Personalized Services: Customers expect tailored financial products. Through data fabric, companies can analyze customer behaviors and preferences to better customize services.
- Fraud Detection: The ability to quickly process and analyze transaction data can enhance an organization’s response to fraudulent activity. Data fabric architectures allow for real-time analysis that improves overall security measures.
As customer sensitivity increases regarding data security and privacy, a data fabric can equip financial services with the tools to meet these demands effectively while adhering to compliance requirements.
The ability to synthesize information from numerous sources through a data fabric approach paves the way for significant competitive advantages in the financial services landscape.
Future Trends in Data Fabric Development
The relevance of future trends in data fabric development is vital in today's highly digitized business environment. As organizations generate vast volumes of data, the need for dynamic integration solutions becomes increasingly paramount. Data fabric is evolving to ensure businesses can manage this complexity efficiently. With innovations at play, it's essential for decision-makers to understand how these trends affect operational strategies and data accessibility.
Artificial Intelligence and Machine Learning Integration
Integrating artificial intelligence (AI) and machine learning (ML) into data fabric is revolutionizing how businesses interpret large datasets. This fusion cannot be overlooked. It enables automated data classification, improves predictive analytics, and fosters smarter insights. Organizations can leverage these technologies to uncover patterns that traditional methods would miss. Such capabilities not only enhance data accuracy but speed up decision-making processes significantly.
- Predictive Maintenance: Industries can reduce operational costs by using AI to analyze the data and predict failures before they occur.
- Customer Insights: Companies can utilize ML models to better understand customer preferences and tailor experiences accordingly.
- Enhanced Security: AI-driven monitoring helps fortify data security by identifying and responding to threats in real-time.
Tapping into AI and ML capabilities allows businesses to handle data more intelligently. The results can lead to streamlined operations, more robust data management, and informed strategic decisions.
Shift Towards Hybrid and Multi-Cloud Strategies
As businesses navigate the digital transformation era, a shift towards hybrid and multi-cloud strategies becomes increasingly apparent. Organizations are moving data between various cloud platforms seamlessly. This shift maximizes flexibility and operational agility.
“By adopting a hybrid approach, businesses can effectively optimize costs, enhance performance and ensure data redundancy.”
Engaging multiple cloud solutions allows for:
- Cost Efficiency: With various providers competing, companies can select options that offer the best value for their data storage and processing needs.
- Risk Management: Distributing data across multiple clouds mitigates risks associated with vendor lock-in.
- Regulatory Compliance: Organizations can choose where to store data, ensuring compliance with location-based regulations.
Therefore, implementing hybrid and multi-cloud strategies promotes not just flexibility but security and legal adherence. As these trends continue to evolve, they set a clear pathway for optimizing business operations through a resilient data fabric.
Culmination: The Strategic Role of Data Fabric
Data fabric emerges as a vital system in modern business environments, providing a seamless way to manage increasing data complexities. This article has emphasized data fabric’s significance, shedding light on its components, benefits, and challenges. It situates data fabric not just as a technical solution but as a key strategic asset for organizations navigating the intricate data-driven landscape today.
Summary of Main Points
Throughout this discussion, we investigated multiple facets of data fabric. Here are some essential points:
- Definition and Purpose: Data fabric is a cohesive architecture designed to manage diverse data sets across various platforms while ensuring accessibility and data security.
- Integration of AI: The role of artificial intelligence and machine learning is essential as they enable enhanced real-time data processing and insights generation.
- Adoption Drivers: Businesses face rising data volumes and complexity, often stemming from hybrid work environments—which drive the adoption of data fabric systems.
- Industry Applications: Our analysis highlighted practical applications across sectors, specifically in healthcare and financial services, where data integrity and accessibility are crucial.
- Future Outlook: Trends indicate a shift towards hybrid and multifaceted solutions as organizations continue to embrace cloud services, anticipating a long-term impact on data strategies.
Final Recommendations for Businesses
Ultimately, businesses aiming to thrive in this evolving data landscape should consider the following:
- Evaluate Current Systems: Assess existing data infrastructure and identify necessary upgrades that align with data fabric capabilities.
- Invest in Training: Ensure business units are educated on data fabric functionalities to harness its benefits fully, fostering a culture in data-driven decision-making.
- Implement Gradually: Begin the integration of data fabric methods in manageable phases to mitigate disruptions and align with business objectives steadily.
- Focus on Compliance: Pay attention to governance aspects to ensure that the adopted solutions respect and adhere to regulatory and compliance standards in your industry.
By embracing data fabric, organizations prepare themselves for a future where seamless access to reliable data is not just an advantage but a necessity in shaping strategic decisions and sustaining competitive advantages.
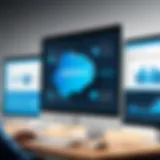
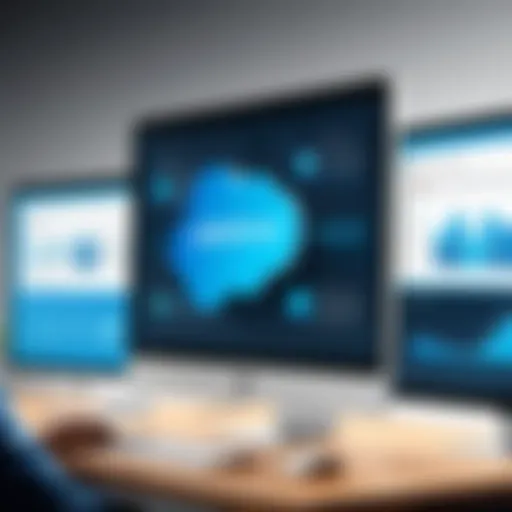