Unlocking Insights with Alteryx Sample Data
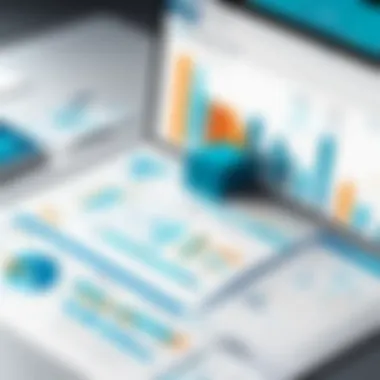
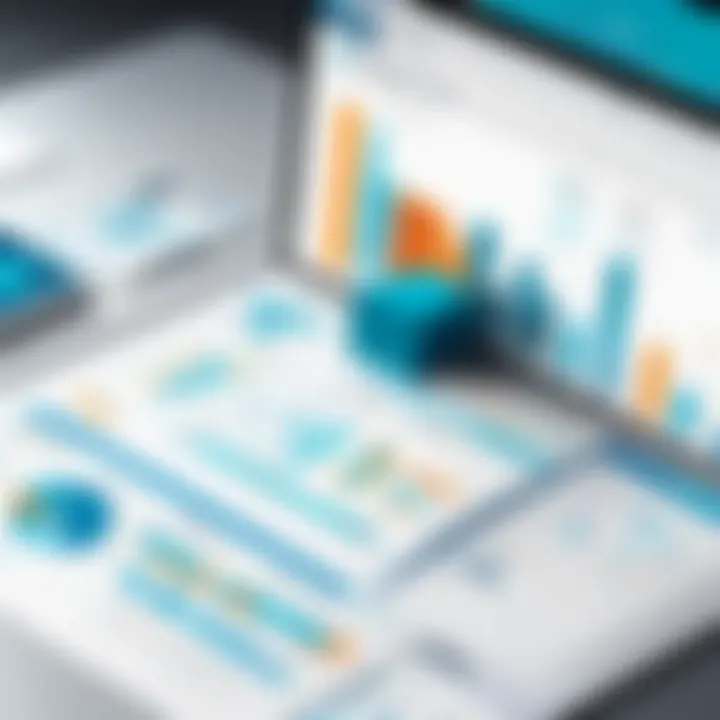
Intro
In an era where data-driven decision-making is paramount, the utilization of tools like Alteryx stands out. Alteryx streamlines data preparation, analysis, and visualization. This article delves into the concept of sample data within Alteryx, demonstrating its profound impact on analytics and business intelligence. By examining the structure, sources, and applications of sample data, readers will grasp how to leverage this resource effectively for enhanced analytical insight.
Technological Research Overview
Recent Technological Innovations
The landscape of data analytics has evolved significantly. Alteryx has introduced various tools that simplify complex data processes. For instance, automating data blending allows analysts to focus on interpreting insights rather than tediously preparing data.
Impact on Business Operations
Businesses that adopt Alteryx experience smoother operations. Efficient data handling translates to quicker decision-making processes. Companies no longer operate in silos, enabling cross-department collaborations that foster innovation and improved performance.
"The adoption of advanced analytic tools like Alteryx can be the differentiator in achieving operational excellence in today's competitive environment."
Future Technological Trends
Looking ahead, the integration of AI with data analytics is expected to rise. Alteryx is at the forefront, incorporating machine learning capabilities. This trend could reshape how organizations analyze data, making insights not only more accessible but also predictive.
Data Analytics in Business
Importance of Data Analytics
Data analytics is crucial for strategic planning and operational efficiency. Firms that leverage data effectively position themselves ahead of competitors. It allows businesses to identify trends and optimize resource allocation.
Tools for Data Analysis
Several tools complement Alteryxโs capabilities, enhancing the overall data analysis process. Solutions such as Tableau offer strong visualization features, while Microsoft Power BI provides comprehensive business intelligence functionalities. Integrating these tools with Alteryx can maximize analytical efforts.
Case Studies on Data-Driven Decisions
Real-world applications strengthen the argument for adopting analytics. Companies like Netflix use data insights to enhance user experience and content delivery. This data-centric approach not only boosts customer satisfaction but also drives revenues.
Cybersecurity Insights
Threat Landscape Analysis
Given the digital environment, cybersecurity cannot be overlooked. Data breaches are perpetually evolving threats, necessitating organizations to remain vigilant. Understanding these threats is essential for effective defense mechanisms.
Best Practices for Cybersecurity
Implementing robust cybersecurity measures is non-negotiable. Companies should:
- Adopt multi-factor authentication.
- Regularly update software and systems.
- Train employees on security awareness.
Regulatory Compliance in Cybersecurity
Staying compliant with regulations like GDPR is critical. Non-compliance can lead to penalizations and loss of customer trust. Organizations must incorporate compliance into their cybersecurity strategy.
Artificial Intelligence Applications
AI in Business Automation
AI is progressively reshaping business processes. Automation of routine tasks frees employees to focus on more strategic activities. This shift not only enhances productivity but also drives innovation.
AI Algorithms and Applications
Utilizing algorithms such as neural networks can elevate analytical capabilities. Alteryx supports several AI functionalities which, when mastered, can yield valuable insights from immense datasets.
Ethical Considerations in AI
As AI adoption surges, ethical concerns become prominent. Issues surrounding data privacy and algorithm transparency must be addressed. Businesses must navigate these challenges to foster trust and integrity.
Industry-Specific Research
Tech Research in Finance Sector
The finance sector is a prime arena for analytics applications. By utilizing Alteryx, organizations can enhance predictive modeling to handle market volatility and consumer behaviors effectively.
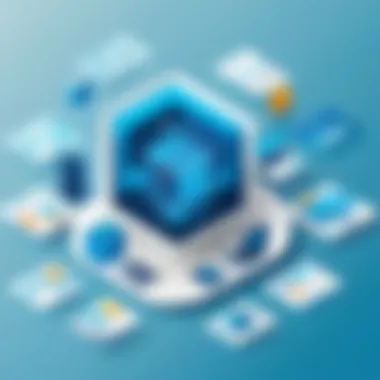
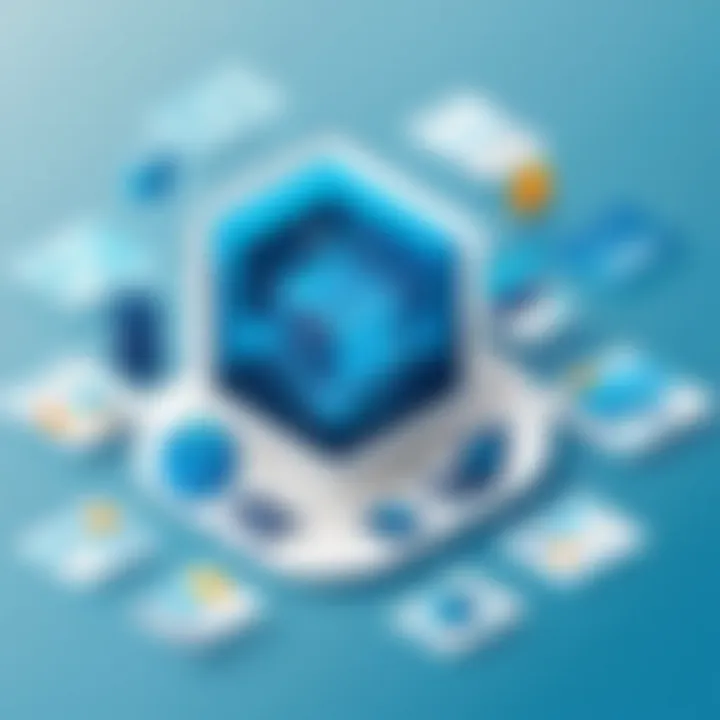
Healthcare Technological Advancements
In healthcare, data analytics can unlock efficiencies in patient care. Tools such as Alteryx support predictive analytics aimed at improving treatment outcomes and managing patient data responsibly.
Retail Industry Tech Solutions
In retail, understanding customer preferences through analytics directly impacts sales strategies. Utilizing Alteryx for data analysis allows businesses to adapt dynamically to consumer needs.
By understanding the nuances of Alteryx sample data, companies can harness analytics for impactful decision-making. In a world driven by data, proficiency in these tools is essential.
Understanding Alteryx
Understanding Alteryx is crucial when exploring sample data for effective analytics and data management. Alteryx is a powerful analytics platform that streamlines the data preparation and analysis processes. When users grasp the functionality and context of Alteryx, they can utilize sample data to make informed business decisions. This understanding allows professionals to leverage the software effectively, ensuring the analytical capabilities are maximized to derive actionable insights.
What is Alteryx?
Alteryx is a data analytics platform that facilitates the preparation, blending, and analysis of data. It is designed to empower users, regardless of their technical expertise, to analyze data effectively and autonomously. Unlike traditional data processing tools, Alteryx provides a user-friendly interface that employs a visual workflow approach. This allows users to perform complex data tasks without needing extensive programming skills. The software integrates various data sources seamlessly, making it a versatile tool for analysts and decision-makers.
Key Features of Alteryx
Alteryx stands out for its comprehensive features that enhance the data analytics workflow. Key features include:
- Data Preparation: Alteryx simplifies data cleaning and preparation with tools that automate tasks and reduce errors.
- Data Blending: Users can connect and combine data from multiple sources effortlessly. This capability is essential for creating a unified data view.
- Advanced Analytics: Alteryx offers predictive analytics, spatial analytics, and machine learning capabilities. These tools empower users to uncover insights that drive strategic decisions.
- Collaboration Tools: The platform includes features that enable team collaboration. This ensures that stakeholders can share insights and work together on analytics projects efficiently.
- User-Friendly Interface: The drag-and-drop interface makes it easy for users to create workflows without writing code, enhancing accessibility for users at all skill levels.
The Role of Alteryx in Data Analytics
Alteryx plays a pivotal role in modern data analytics by facilitating quick and reliable decision-making. It bridges the gap between data integration and actionable insights. Alteryx enables users to create visual data workflows, significantly reducing the time spent on data preparation.
Furthermore, its capabilities extend beyond mere data handling. Alteryx allows users to apply complex analytics models with speed and precision. This results in a more agile and responsive approach to data-driven decision-making within organizations. In the competitive landscape of business intelligence, leveraging Alteryx effectively can enhance operational efficiency and lead to better results.
Preface to Sample Data
Understanding sample data is vital in the realm of analytics and data management. Sample data serves as a foundational element in the process of extracting insights from complex datasets. It provides a simplified, yet representative subset of information that can help analysts and decision-makers visualize potential outcomes without the need for processing entire datasets, which can be cumbersome and time-consuming. The introduction of sample data into analytics frameworks like Alteryx enables users to prototype, validate, and iterate on their analysis swiftly.
Defining Sample Data in Analytics
Sample data refers to a smaller, manageable portion of larger datasets that can be analyzed to detect trends or patterns. It acts as a miniature version of the original dataset, allowing for exploratory analysis without exhausting resources. In analytics, effective sample data carries statistical significance; its selection must be deliberate to ensure that the insights derived are reflective of the broader dataset.
Common methods of defining sample data include:
- Random Sampling: In this method, samples are chosen randomly from the larger dataset, ensuring each member has an equal chance of selection.
- Stratified Sampling: This involves dividing the dataset into subgroups and sampling from each subgroup, which is particularly useful when certain characteristics need to be represented.
- Systematic Sampling: Here, samples are taken at regular intervals from a sorted dataset. For example, every tenth record might be selected.
Using these techniques ensures that sample data is both representative and robust, supporting sound decisions in analytics practices.
Importance of Sample Data in Business Intelligence
Sample data plays a crucial role in business intelligence (BI). It empowers organizations to glean insights efficiently without overwhelming the analytics infrastructure. Here are some significant benefits of using sample data in BI:
- Cost Efficiency: Analyzing smaller datasets reduces computational costs and speeds up the processing time. This allows businesses to focus on insights without extensive resource allocation.
- Faster Decision-Making: With sample data, teams can conduct preliminary analyses quickly and derive actionable insights to inform decision-making. This rapid feedback loop is essential in dynamic market environments.
- Risk Mitigation: Working with sample data can minimize risks when testing hypotheses or validating models. If decisions are based on smaller, controlled datasets, the potential for adverse effects on the entire operation is reduced.
- Enhanced Data Quality: By focusing on specific aspects of a dataset, organizations can identify and rectify data quality issues in a more manageable context.
"Effective use of sample data can be a game changer for organizations looking to leverage analytics for strategic advantage."
In summary, sample data in analytics is not merely a convenience; it is a critical component that facilitates enhanced data management and analytics. By strategically employing sample data, organizations can streamline their BI efforts, ultimately leading to more informed and timely decisions.
Types of Alteryx Sample Data
Understanding the types of Alteryx sample data is crucial for professionals in analytics and data management. This understanding helps in optimizing the use of Alteryx as a powerful tool. Sample data can guide users in testing various analytical methods, ensuring better decision-making based on simulated outcomes. Additionally, knowing the types of data available can lead to efficiency in workflows and a clearer understanding of problem-solving through data.
Pre-Loaded Sample Datasets
Pre-loaded sample datasets in Alteryx serve as foundational tools for users exploring data analysis without needing prior datasets. These datasets cover various domains such as retail, finance, and healthcare, allowing for comprehensive analysis within a familiar environment. They provide a controlled setting to practice skills and test methodologies. Using these datasets, individuals can learn how to manipulate and transform data effectively. Each dataset has distinct characteristics and scenarios that highlight specific features of Alteryx.
Moreover, these datasets can offer insights into common data patterns and anomalies. This exposure is beneficial for new users who may not yet have access to their own data. It provides a platform for learning without the stress of data cleansing or transformation. Users can simply load the dataset and begin analyzing immediately.
Custom Sample Data Creation
Custom sample data creation allows analysts to generate datasets tailored to specific scenarios, which enhances the analytical experience significantly. This capacity is especially relevant for organizations that possess unique datasets or industry-specific needs. Users can create datasets that reflect realistic situations, thus making models more applicable to actual business conditions.
Creating custom data typically involves several steps. First, analysts must identify the variables relevant to their analysis. Then, they can use Alteryx tools to generate synthetic data that mirrors those variables. This customization ensures that the analysis is relevant and contextually appropriate.
Moreover, custom sample data is beneficial for testing new models or algorithms. Analysts can simulate different outcomes based on varying parameters. This not only explores different analytics strategies, but it can also provide critical insights into forecasting and trend analysis.
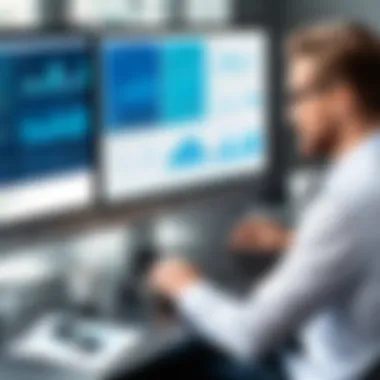
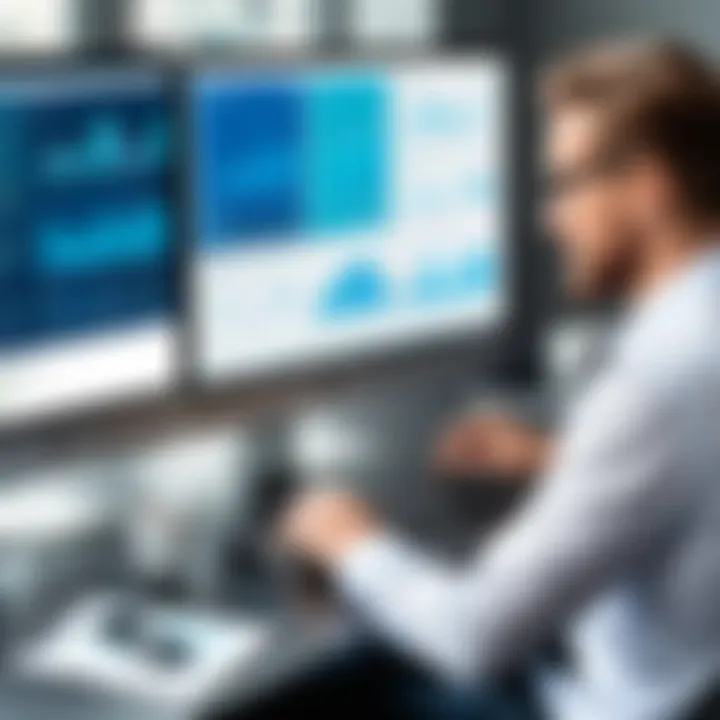
The ability to create and manipulate custom datasets emphasizes the versatility of Alteryx in addressing specific analytical challenges. It enhances the overall analytical process, allowing professionals to explore possibilities in ways that pre-loaded datasets may not facilitate.
Accessing Sample Data in Alteryx
Accessing sample data in Alteryx is a critical process for anyone looking to leverage the platform's capabilities. Sample data serves as a foundation for analysis and can help users understand how to manipulate and transform information effectively. Understanding the methods and interface for accessing this data can significantly enhance one's analytical workflow.
Navigating the Alteryx Interface
The Alteryx interface is designed for user-friendliness while also providing advanced functionalities. It typically consists of several key components, including the Tool Palette, Canvas, and Configuration Window. Users should familiarize themselves with these elements to navigate effectively.
- Tool Palette: This is where you find all available tools, categorized logically to streamline your workflow. You will see options like Data Input, Data Preparation, and Analysis tools. Familiarizing yourself with these sections is essential for efficient data access.
- Canvas: This central workspace allows users to visually layout their workflows. You can drag and drop tools from the Tool Palette onto the Canvas. Connecting these tools properly is key to creating an effective data flow.
- Configuration Window: This area provides settings and configurations for your selected tool. When loading sample data, understanding the options available here can impact data manipulation and analysis.
The overall design emphasizes a logical flow of data processing, making it easier for users to access and interact with sample datasets.
Importing Sample Data from External Sources
Importing sample data into Alteryx from external sources is a straightforward process, yet it offers flexibility that can cater to diverse analytics needs. Here are the common methods available:
- Direct Import: Users can directly import data from files such as CSV, Excel, or SQL databases. The Input Data tool allows users to select files from their local system.
- Database Connections: Alteryx enables connections to various databases such as Microsoft SQL Server, Oracle, or cloud databases. Setting up these connections often requires authentication details and connection strings. This capability is significant for users handling large datasets.
- APIs and Web Services: For advanced users, Alteryx supports importing data through APIs. This method enables integration with web services, providing real-time data access for analysis.
By mastering these import methods, users can enhance their workflows, tailoring the source data to fit their unique analytical requirements. The ability to access diverse types of data broadens the scope for analysis and reporting.
Takeaway: Mastery of the Alteryx interface and familiarity with data import methods set the stage for effective analysis, allowing users to fully leverage the power of sample data in their projects.
Analyzing Sample Data
Analyzing sample data is a crucial aspect of extracting insights and value from the information available in Alteryx. This process not only allows for better decision-making but also enhances overall data management capabilities. Proper analysis of sample data involves several components that contribute to generating meaningful analytics. The aim here is to not only understand how to work with data but also recognize its potential impacts on business outcomes.
When analyzing sample data, the focus should be on accuracy, relevance, and timely integration of data sources. Beyond merely handling raw data, effective analysis encompasses a systematic approach to data preparation and visualization. This ensures that the insights derived are trustworthy and actionable.
Data Preparation Techniques
Data preparation is the initial step in the analysis pipeline. It involves cleaning, transforming, and organizing data to ensure it is ready for analysis. The importance of rigorous data preparation cannot be overstated. It determines the quality of insights that will follow.
To prepare data effectively in Alteryx, consider the following techniques:
- Data Cleaning: Remove duplicates or erroneous entries. These include blanks or inconsistencies that can skew analysis results. Tools within Alteryx can automate much of this process.
- Data Transformation: Alter the structure or format of the data. This may involve normalizing data ranges or converting data types to ensure compatibility with analytical tools.
- Data Matching and Merging: Use Alteryx tools to join different datasets. This can reveal relationships that are not apparent in isolated data sets and enhances the breadth of analysis.
- Data Enrichment: Integrate external data sources to provide additional context or information. This can improve the robustness and accuracy of insights derived from the data.
By applying these techniques, one sets the foundation for more effective analysis and ensures that the results are based on reliable data.
Visualization Best Practices
Visualization plays a critical role in data analysis by converting complex data into easily digestible formats. Effective visualizations can help stakeholders grasp insights quickly, which is essential in data-driven decision-making. In Alteryx, best practices for visualization include:
- Know Your Audience: Customize visuals according to the audience's needs. Different stakeholders may require different levels of detail and complexity.
- Use Appropriate Chart Types: Different types of data require different visual representations. For instance, bar graphs are useful for categorical data, while line graphs are better for showing trends over time.
- Maintain Simplicity: Avoid clutter. Overly complex visuals can confuse rather than inform. Aim for clarity and focus on the key message you want to communicate.
- Interactive Elements: Utilize Alteryxโs tools that allow for interactivity. This can enhance user engagement and exploration of the data.
- Consistent Formatting: Use a uniform color scheme and layout to maintain coherence across multiple visualizations. This helps in building a narrative around the data.
In summary, analyzing sample data with robust preparation and effective visualization techniques can lead to significant improvements in data management and analytics. This systematic approach ensures that organizations can leverage data intelligently and make informed decisions based on sound insights.
Methods for Leveraging Alteryx Sample Data
Leveraging Alteryx sample data effectively allows organizations to streamline their analytics processes and enhance data management capabilities. This section details best practices for data integration and explores the application of predictive analytics, both crucial for maximizing insights from data in a structured manner.
Best Practices for Data Integration
Data integration is a foundational skill in analytical environments. Effective data integration ensures that various data sources come together seamlessly, providing a comprehensive view for analysis. Working with Alteryx sample data requires careful planning and execution of data integration strategies:
- Understand the Sources: Knowing where your sample data originates is essential. This includes understanding data formats, structure, and any transformations that may be needed.
- Utilize Alteryx Tools: Alteryx offers numerous built-in tools for data blending and transformation. Tools such as the Join Tool, Union Tool, and Data Cleansing Tool can significantly enhance integration processes by automating data preparation steps.
- Ensure Data Quality: Always check for inaccuracies or inconsistencies in your data. Utilizing the Data Profiling tool within Alteryx can help identify issues before they affect your analysis.
- Document Integration Processes: Maintaining documentation of how data is integrated aids in accountability and reproducibility. This ensures that the processes can be repeated or modified in future projects without losing the context.
Implementing these best practices results in a more streamlined process, reduces errors, and offers a higher quality output.
Applying Predictive Analytics
Predictive analytics stands as a powerful functionality within Alteryx, allowing businesses to forecast trends and behaviors based on historical data. Focusing on sample data provides a concrete starting point for building predictive models:
- Select Appropriate Models: Choose models that fit the type of data and business objective. Alteryx provides a range of predictive tools including regression, clustering, and time series analysis.
- Evaluate Model Performance: Utilize the built-in tools to assess how well the model performs against known outcomes. Metrics such as accuracy, precision, and recall are important.
- Iterate and Improve: Predictive modeling is not a one-time effort. Continuously refine your models based on new data and insights.
- Communicate Insights Clearly: Visualization tools within Alteryx can effectively showcase your findings. Clear communication of predictive results to stakeholders is crucial for informed decision-making.
Incorporating predictive analytics into your approach allows a proactive stance, pushing businesses toward data-driven strategies. This not only maximizes the utility of Alteryx sample data but also enhances overall data management.
Real-World Applications of Alteryx Sample Data
The exploration of Alteryx sample data reveals practical applications that extend far beyond theoretical concepts. Organizations across various industries have harnessed the capabilities of Alteryx to streamline their data processes and improve decision-making. Understanding these real-world applications is pivotal for professionals looking to leverage analytics for competitive advantage. The benefits are numerous, including enhanced efficiency, increased accuracy of insights, and improved data-driven strategies.
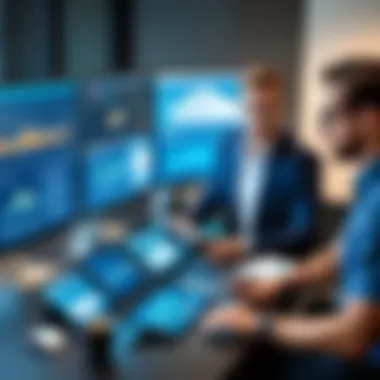
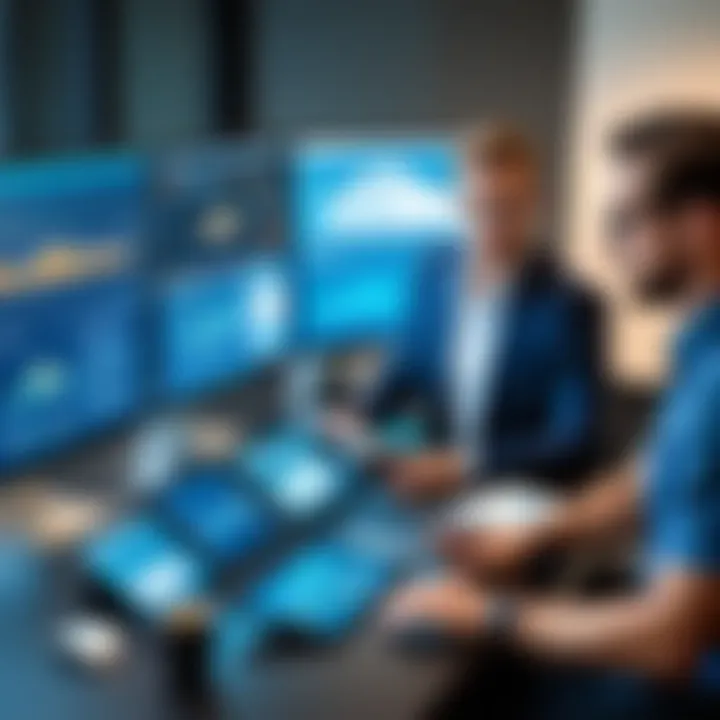
Case Studies from Different Industries
Real-world examples highlight the effectiveness of Alteryx in diverse sectors. In healthcare, for instance, a major hospital network utilized Alteryx sample data to analyze patient outcomes while integrating various datasets. They identified trends in readmission rates, allowing them to tailor interventions effectively. This not only improved patient care but also reduced operational costs.
In retail, a well-known brand used Alteryx to optimize their supply chain management. By analyzing historical sales data alongside current inventory levels from sample datasets, they improved inventory forecasting. The result was minimized stock-outs and better product availability, leading to significant sales growth.
In the financial services sector, companies have implemented Alteryx to enhance risk assessment processes. By analyzing sample data that reflect market conditions and customer behavior, firms improved their predictive models. This led to better-informed lending decisions and reduced default rates.
Success Stories of Businesses Using Alteryx
Many organizations have shared their success stories after implementing Alteryx sample data into their operations. A global telecommunications company reported a remarkable improvement in their customer service metrics. By analyzing call data and customer interactions, they identified key factors affecting customer satisfaction. Implementing changes based on these insights resulted in a 20% increase in customer retention.
Another example involves a nonprofit organization that analyzed community engagement data with Alteryx. By utilizing various sample datasets, they recognized patterns in volunteer participation. This insight allowed them to better coordinate events, leading to a 30% rise in volunteer involvement.
These examples demonstrate the tangible benefits of Alteryx when properly applied to real-world scenarios. Companies that adopt these tools not only gain insight into their operations but also cultivate a culture of data-driven decision-making. As a result, they stand to benefit immensely in terms of efficiency and strategic growth.
Challenges in Using Sample Data
Using sample data in Alteryx can present several challenges that can impact analysis outcomes and decision-making. Understanding these challenges is essential for professionals seeking to harness the full potential of Alteryx sample data. By being aware of common pitfalls and strategies to overcome them, organizations can ensure superior data quality, reliability, and usability in their analytics endeavors.
Common Pitfalls to Avoid
- Inadequate Understanding of Sample Data: Many users dive into sample datasets without comprehensively understanding their structure or limitations. This may lead to misinterpretations during analysis or misguided conclusions based on erroneous data insights.
- Overlooking Data Relevance: Not all sample data is relevant for every analysis. Practitioners often use generic datasets without assessing their applicability to the specific business problem at hand. This can result in wasted resources and time spent on unproductive analysis.
- Ignoring Data Quality: Sample data may contain inaccuracies or outdated information. Users can fall into the trap of assuming that sample data is accurate because it is provided within the platform, neglecting to perform necessary data checks and cleansing processes.
- Failure to Document Changes: When users modify sample data for their specific analytical needs, they may fail to document these changes. This oversight can lead to inconsistencies over time, creating confusion during collaborative projects or audits.
- Inconsistent Use of Data: Different teams within an organization may use sample data inconsistently, resulting in varied interpretations and analyses. This can lead to discrepancies in reporting and misalignment in decision-making processes.
By recognizing these common pitfalls, an organization can encourage a more careful approach when engaging with sample data, ultimately driving better decisions and outcomes from data analyses.
Strategies for Overcoming Data Quality Issues
Improving data quality while utilizing sample datasets is crucial. Here are several strategies that professionals can implement to address potential data quality issues:
- Conduct Regular Data Audits: Regularly check sample datasets for integrity, accuracy, and relevance. This practice ensures that the data used in analytics is up to date and trustworthy.
- Utilize Data Profiling Tools: Employ data profiling applications to identify anomalies and discrepancies in sample data. Tools like Alteryx offer functionality to monitor data quality, making it easier to spot areas needing attention.
- Create Data Validation Procedures: Establish clear protocols for data review and validation before analysis. Involve data stewards or quality assurance teams to verify that any modifications to sample datasets maintain data integrity.
- Integrate Feedback Loop: Set up a systematic feedback loop where data users can report any issues they encounter with sample data. This collaborative problem-solving approach fosters a culture of continuous improvement and accountability.
- Train Users on Data Best Practices: Consider implementing training sessions that focus on best practices for working with sample data. Raising awareness about potential pitfalls will better equip employees to avoid mistakes and uphold data quality standards.
By proactively addressing these strategies, organizations can significantly enhance their data quality and reliability when working with Alteryx sample data, ultimately leading to more informed business decisions.
Future Trends in Data Analytics with Alteryx
As we look forward in the landscape of data analytics, understanding the evolving trends is crucial for leveraging tools like Alteryx effectively. Future trends will redefine how we analyze, interpret, and apply sample data in various business settings. The significance of this topic lies in its ability to guide organizations in making informed decisions and adapting to changes in technology and market demands.
- Adaptive Learning Techniques
As machine learning becomes more accessible, Alteryx is likely to incorporate adaptive learning capabilities. This means users can expect more intuitive processes that learn from data patterns over time, improving model accuracy and insight generation. - Integration of AI-driven Tools
The infusion of artificial intelligence into data analytics will enhance how users interact with Alteryx. Expect tools that can automate routine tasks and generate complex analyses with minimal human input. This shift frees data analysts to focus on strategic decision-making rather than mundane tasks. - Cloud-based Solutions
With the rise of remote work and collaboration, cloud-based analytics will take center stage. Alteryx will continue to enhance its capabilities in cloud environments, offering seamless integration and real-time data access for teams spread across multiple locations.
"The future of data analytics is not just about data processing but also about intelligent data storytelling."
Emerging Technologies and Their Impact
The continuous emergence of technologies reshaping data analytics is noteworthy. Here are some of the key technologies likely to influence Alteryx:
- Natural Language Processing (NLP): This technology enables users to query data using everyday language, making analytics more accessible to non-technical users.
- Augmented Analytics: Advanced systems can automate data preparation and insight generation, allowing users to dictate queries without needing deep technical knowledge.
- Internet of Things (IoT): The proliferation of IoT devices will provide vast amounts of data. Alteryx can help organizations harness this data stream for actionable insights.
These technologies will not only improve efficiency but also enhance the quality of insights drawn from sample data.
Predictions for the Future of Alteryx
Looking ahead, several predictions can be made about how Alteryx will evolve:
- Increased Focus on Data Governance
As data becomes a more significant asset, Alteryx will prioritize data governance. Strong governance frameworks can ensure data privacy and compliance, which is vital in todayโs regulatory environment. - Enhanced Collaboration Features
Alteryx will likely improve collaborative features, supporting team analytics and fostering sharing of insights across departments. This can also involve tighter integration with other collaboration tools. - Diversified Learning Resources
Training and skill development resources will expand. As the complexity of data grows, Alteryx will need to provide clear and accessible learning pathways for users.
These predictions signify a shift towards more user-driven, collaborative, and data-aware analytics practices, designed to keep pace with rapid technological changes.
Epilogue
The conclusion serves a vital role in synthesizing the major insights derived from the exploration of Alteryx sample data. This section aims to distill the essence of the discussed topics, highlighting the significance of Alteryx in enhancing analytics capabilities and data management practices. Understanding the structure and application of sample data not only empowers professionals but also fosters a data-driven culture within organizations.
Alteryx facilitates the integration of sample data into analytical workflows, enabling users to generate actionable insights. This process leads to better decision-making and strategic planning. As organizations increasingly rely on data for competitive advantage, the relevance of leveraging Alteryx becomes undeniably clear.
Summarizing Key Takeaways
- Alteryx is a powerful tool for data preparation, blending, and analysis.
- Sample data provides a platform for users to practice and master data manipulation techniques.
- Understanding the available sample datasets is crucial for effective data integration.
- Best practices in data preparation and visualization lead to more impactful analysis outcomes.
- Real-world case studies illustrate the successful application of Alteryx in various industries, reinforcing its effectiveness.
Encouraging Informed Data Practices
To promote effective data practices, professionals must prioritize understanding and leveraging Alteryx sample data. Here are several key considerations:
- Foster a culture of data literacy, ensuring that teams have the necessary skills to utilize tools like Alteryx.
- Regularly assess and refine the data integration processes to enhance accuracy and relevance of insights.
- Encourage collaboration among team members to share knowledge and strategies for better analytical outcomes.
- Stay updated with emerging trends and technologies in data analytics, adapting practices accordingly.
Adopting these strategies will empower organizations to navigate the complexities of data, ensuring they can maximize the benefits of using Alteryx for informed decision-making.
"The ability to analyze and act on data effectively transforms the role of organizations in a data-driven world."