Building a Strong Analytics Strategy Framework
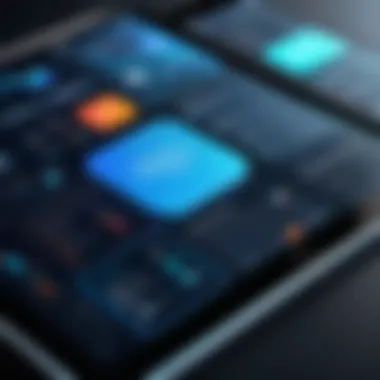
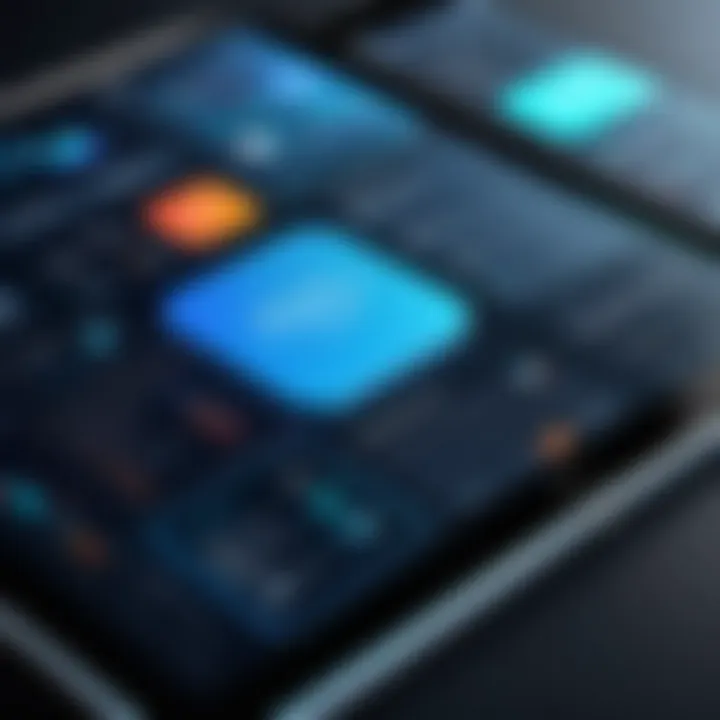
Intro
In today's intensely competitive business landscape, leveraging data effectively is akin to having a secret weapon. Organizations that can synthesize data into actionable insights are the ones that not only survive but thrive. A well-designed analytics strategy acts as a roadmap for navigating this complex terrain, giving businesses the ability to make informed decisions that align with their core objectives.
The framework we discuss here captures all the elements crucial to establishing an effective analytics strategy. From understanding technological innovations to data governance and continuous evaluation, each part of the framework plays a significant role in harnessing the power of data.
Technological Research Overview
Recent Technological Innovations
Technology evolves at warp speed, and recent innovations are shaping how businesses gather, analyze, and act on data. Cloud computing, for instance, has revolutionized data storage and accessibility, enabling companies to handle large datasets with efficiency. Alongside that, improvements in artificial intelligence, particularly machine learning algorithms, have enabled predictive analytics that is far more accurate than ever before.
Impact on Business Operations
These innovations don't just alter operations; they redefine them. With tools that can predict market trends and customer behaviors, businesses can make proactive moves rather than reactive ones. Studies show organizations adopting data-driven strategies see a notable boost in operational efficiency and decision-making speed.
"Ignoring data analytics is like throwing darts blindfolded, hoping to hit the bullseye. Without data, youâre shooting in the dark."
Future Technological Trends
Looking ahead, businesses should keep a keen eye on developments in areas like quantum computing and enhanced data privacy technologies. These advancements promise to offer unprecedented capabilities in data processing and security. Companies prepared to adapt to these technologies will be better equipped to navigate future challenges.
Data Analytics in Business
Importance of Data Analytics
In the grand schema of business strategies, data analytics stands out as a linchpin. The ability to analyze trends, consumer behaviors, and operational efficiencies is vital for informed decision-making. Companies that shy away from using data often find themselves lagging.
Tools for Data Analysis
The right tools can mean the difference between a successful analytics strategy and one that falters. Some of the leading analytics tools include Tableau for data visualization, Google Analytics for web insights, and SAS for advanced analytics. Understanding which tools align with business needs is crucial.
Case Studies on Data-Driven Decisions
Consider Netflix, which uses data analytics to curate content recommendations, enhancing customer satisfaction. Another example is Target, which successfully predicted customer shopping patterns through analytics, leading to improved inventory management and marketing strategies.
Cybersecurity Insights
Threat Landscape Analysis
As data becomes more valuable, the threat landscape intensifies. Cyberattacks have grown in frequency and sophistication, affecting organizations of all sizes. Understanding these threats is imperative for any analytics strategy, as a breach can negate all efforts undertaken to gather and analyze data.
Best Practices for Cybersecurity
Incorporating solid cybersecurity practices into an analytics strategy cannot be overstated. Regular vulnerability assessments, data encryption, and employee training on phishing scams are fundamental to maintaining security.
Regulatory Compliance in Cybersecurity
Organizations must also be aware of regulations like GDPR and CCPA when handling data. Compliance not only avoids potential charges but also builds consumer trust. Ignoring these can lead to not just financial penalties but reputational damage as well.
Artificial Intelligence Applications
AI in Business Automation
Artificial intelligence is a game-changer when integrated into business processes. It automates mundane tasks, allowing human resources to focus on strategic planning. Businesses using AI tools report increased efficiency and reduced operational costs.
AI Algorithms and Applications
Various algorithms, like decision trees and neural networks, play pivotal roles in transforming data into strategic insights. By customizing these algorithms to meet specific business needs, organizations can enhance their analytics outcomes significantly.
Ethical Considerations in AI
As AI becomes more prevalent, ethical concerns also arise. Issues surrounding bias in algorithms, data privacy, and transparency in machine decision-making processes must be addressed. Companies should prioritize ethical practices to build credibility in their analytics strategies.
Industry-Specific Research
Tech Research in Finance Sector
The finance industry utilizes analytics to predict market shifts, assess risks, and enhance regulatory compliance. Machine learning models are increasingly being adopted for fraud detection, proving their worth in safeguarding financial interests.
Healthcare Technological Advancements
In healthcare, data analytics is making significant strides in improving patient care and operational efficiency. Predictive analytics are being used to foresee patient needs, streamline emergency response times, and support clinical decision-making.
Retail Industry Tech Solutions
For retailers, understanding consumer behavior through analytics leads to better inventory management and personalized marketing efforts. Data insights allow businesses to tailor promotions based on shopping trends.
By weaving together these elements and concepts, organizations can create a comprehensive analytics strategy that not only meets current needs but is also formidable for future challenges.
Understanding Analytics Strategy
An analytics strategy is a pivotal element in the modern data-driven world. Businesses today are deluged with an overwhelming amount of data. But data alone doesn't equate to value; itâs what you do with that data that counts. Understanding an analytics strategy provides organizations with a roadmap to harness their data effectively. It serves as a framework for interpreting information, guiding decision-making processes that are grounded in real insights rather than mere guesswork.
Analytics strategies mark the difference between organizations that flounder and those that flourish in competitive landscapes. When properly implemented, this framework allows for more informed choices, better customer experiences, and optimized operations.
Definition and Importance
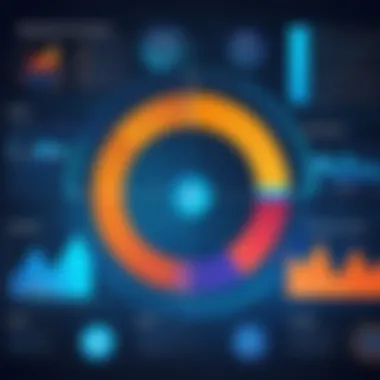
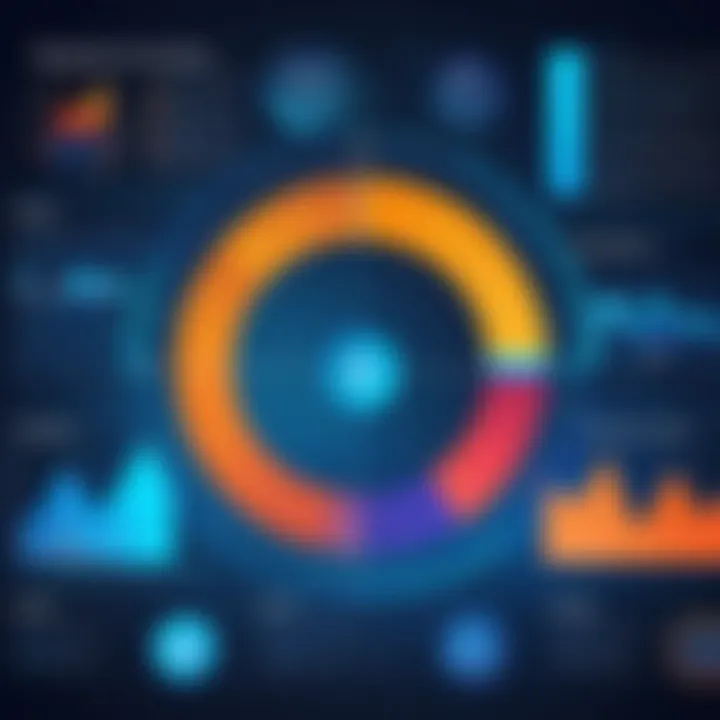
At its core, an analytics strategy is a comprehensive plan that outlines how an organization will collect, analyze, and leverage data to achieve its business goals. This approach is not merely about technology or tools; it encompasses a holistic view of processes, cultural attitudes, and alignment with organizational objectives.
The importance of having a sound analytics strategy canât be overstated:
- Enhanced Decision-Making: Businesses can avoid knee-jerk reactions and make well-informed choices based on data trends and insights.
- Resource Optimization: By identifying what data to collect and how to analyze it, organizations can allocate resources more wiselyâthus increasing efficiency.
- Competitive Advantage: Companies that understand their data landscape are better positioned to adapt and innovate, staying ahead of rivals.
In todayâs business environment, failure to adopt a proactive analytics strategy can lead to stagnation.
The Role of Data in Business Strategy
Data now sits at the heart of effective business strategies. Imagine steering a ship in turbulent waters without a compass. Thatâs how businesses operate without leveraging their data. Data provides the direction needed to navigate the complexities of the market. More importantly, it creates a clearer picture of consumer desires, market trends, and operational efficiencies.
Furthermore, integrating data analytics into the core of business strategy is not a one-off project but rather an evolving journey. Organizations must continuously gather and analyze data to keep pace with their dynamic environments. For example, retail businesses can track shopper behavior, adapting their marketing strategies in real time to cater to shifting preferences. On the other hand, companies in finance might utilize data to forecast economic downturns, allowing them to safeguard their interests and those of their clients.
In summary, data is not just an afterthoughtâitâs an integral part of strategic planning that informs every aspect of business operations. Those who grasp this fact and weave data into the very fabric of their strategies can expect to see significant returns on their investment.
Key Components of an Analytics Strategy Framework
In today's fast-paced business environment, a well-structured analytics strategy is more than just a luxury; it's a necessity. The overarching framework of analytics serves as a roadmap, guiding organizations through complex data landscapes and helping them leverage insights for strategic advantage. Understanding the essential components that make up this framework is crucial for developing effective data-driven solutions.
The importance of these key components extends far beyond mere data collection. Each element plays an integral role in ensuring that analytics efforts align with business goals, enhance decision-making, and ultimately drive performance. When put together thoughtfully, these components not only streamline the process of data analysis but also amplify its impact across the organization.
Setting Clear Objectives
The starting point for any analytics strategy is the establishment of clear objectives. These objectives should reflect the organizational mission and aspirations, serving as the guiding star for all analytics initiatives. Without defined goals, teams might find themselves wandering into unproductive territory, wasting time and resources on endeavors that do not contribute to the company's vision.
For instance, if a retail company aims to increase customer retention by 20% over the next year, the analytics strategy should focus on understanding customer behavior and preferences through targeted data collection and analysis. By funneling efforts toward such specific outcomes, organizations can ensure their analytics capabilities contribute directly to strategic aims and measurable results.
Data Governance and Management
Data Quality
Data quality underpins the entire analytics ecosystem. It refers to the accuracy, completeness, and reliability of data collected. Low-quality data can lead to misguided insights, ultimately affecting key business decisions. Therefore, ensuring high data quality is paramount.
A robust data quality framework involves establishing standards and processes for data entry and validation. High-quality data is a trusted resource that aids organizations in making sound decisions based on factual and comprehensive information. Poor data quality, on the other hand, can decimate the efficacy of even the best analytics tools, leading to flawed conclusions.
Data Accessibility
Accessibility of data is another vital aspect. This means that relevant stakeholders can easily access and utilize the data needed for decision-making. Itâs not just about making the data available; it's about ensuring that the right people can find and interpret it seamlessly.
A strong data accessibility framework enhances collaboration across departments and enables quick responses to market changes. However, organizations must balance accessibility with necessary restrictions to protect sensitive information.
Data Security
Data security is the assurance of protecting data from unauthorized access and corruption. With increasing regulations around data privacy, it's a hot topic for organizations handling customer information. A sound approach to data security involves creating protocols and technologies to safeguard assets effectively.
While ensuring data security might seem like a hurdle on the path to accessible insights, it's necessary for maintaining trust with customers and stakeholders. Organizations that neglect this aspect can face dire consequences, from hefty fines to reputational damage.
Choosing Analytical Techniques
Selecting the right analytical techniques is crucial for converting raw data into actionable insights. Depending on the specific goals, companies can choose from various methodologies, such as:
Descriptive Analytics
Descriptive analytics focuses on summarizing historical data to glean insights into past events. This technique can help organizations understand trends and patterns. For example, a company analyzing sales data from previous years can identify seasonal fluctuations in customer interest, allowing for better inventory management moving forward.
This approach is highly beneficial for establishing baselines and performance metrics, but organizations must be careful not to rely solely on historical data for future predictions, as that could limit innovation.
Predictive Analytics
Predictive analytics, on the other hand, leverages statistical models and machine learning to forecast future outcomes based on historical data. This approach embraces uncertainty and provides a competitive edge by anticipating market trends, customer behavior, and potential risks. For instance, an insurance company might use predictive analytics to assess the likelihood of claims among its policyholders.
The unique feature here is its capacity to forecast, which can significantly aid in strategic planning. However, organizations must understand the limitations of predictive analytics, as predictions are never foolproof and require continuous adjustments based on new data.
Prescriptive Analytics
In contrast to the previous techniques, prescriptive analytics goes one step further by recommending actions based on predicted outcomes. It combines historical and predictive data with optimization algorithms. This advanced category can help decision-makers choose the best course of action.
For example, a logistics firm may use prescriptive models to decide the optimal route for deliveries based on predictions of traffic patterns. While undeniably valuable, its complexity requires robust analytical tools and expertise, which can be a barrier for smaller firms.
Technology and Tools
Data Visualization Tools
Data visualization tools transform complex data into easy-to-understand graphical representations. These visual aids help teams identify trends and outliers quickly without sifting through piles of data. Tools such as Tableau and Power BI are popular in making data accessible and comprehensible for stakeholders at all levels.
A unique feature of these tools is their ability to foster collaboration; visual data narratives are more engaging than spreadsheets. However, over-simplifying data can sometimes lead to critical insights being overlooked, making it essential to provide context alongside visuals.
Statistical Software
Statistical software provides capabilities for advanced analyses using techniques like regression, correlation, and hypothesis testing. Software like R and SAS enables organizations to perform in-depth analysis and interpretation of their data.
This software can be quite powerful, but it often requires statistical expertise to utilize effectively. If teams lack this skill set, they might struggle to unlock the full potential of their data.
Machine Learning Platforms
Machine learning platforms use algorithms to identify patterns in data and automate decision-making processes. This technology can offer solutions in numerous domains, from market analysis to fraud detection. Platforms like TensorFlow and Azure Machine Learning facilitate organizations in developing customized solutions tailored to their needs.
The unique advantage of machine learning is its ability to improve over time with experience and new data. However, deploying such platforms may come with challenges, including the need for significant data quality and proper handling of ethical considerations in decision-making.
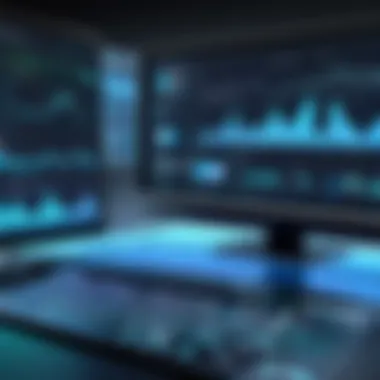
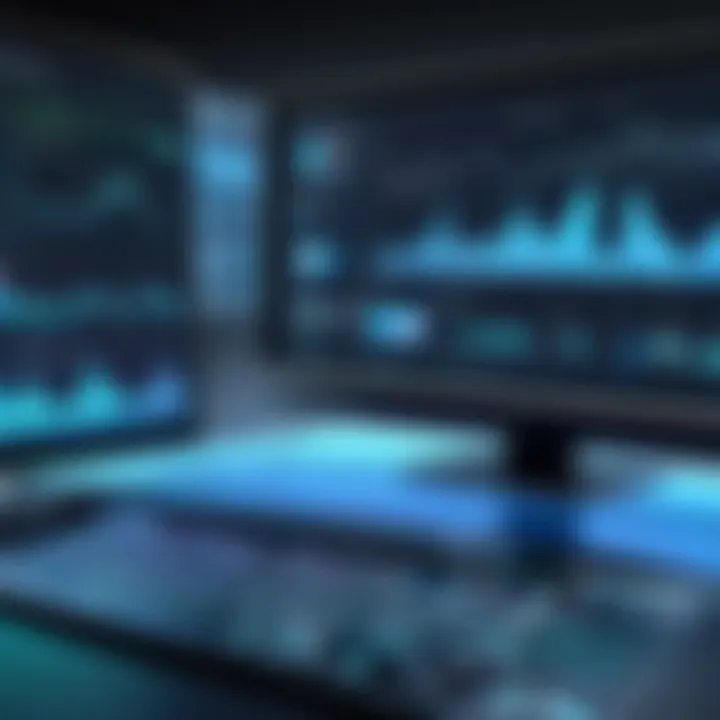
Understanding these components of an analytics strategy framework allows organizations to construct a cohesive and efficient system that leads to better, more informed decision-making.
Implementation of the Framework
Implementing an analytics strategy framework isnât just about checking boxes or following a one-size-fits-all approach. It's about creating a well-oiled machine that aligns data insights with business objectives. When organizations put this process into play, they're not just stacking up numbers and reports. They are laying down a foundation that allows them to make informed, precise decisions that can significantly impact their success. A structured implementation offers several key benefits:
- Clarity and Direction: An implementation roadmap provides clear guidance and defines the specifications needed to turn strategies into actions.
- Enhanced Collaboration: By delineating roles and responsibilities, teams work in sync. This collaboration fosters innovation and makes problem-solving more efficient.
- Measurable Results: With a framework in place, organizations can set benchmarks and KPIs that allow for ongoing assessment of both short-term victories and long-term goals.
While these points showcase the advantages, one must also consider the nuances of the implementation process. Challenges such as changing company culture or misalignment with existing processes may arise. However, with careful planning and consideration of the elements involved, these obstacles often can be navigated successfully.
Creating an Implementation Roadmap
An implementation roadmap is like a GPS for your analytics strategy; it guides every move you make. Without this roadmap, itâs easy to veer off course, especially when dealing with the complexities of data analytics. A well-crafted roadmap lays out the steps required to operationalize your strategy, from inception to execution.
Here are several elements to consider while crafting your roadmap:
- Timeline: Define deadlines for each phase, ensuring the strategy rolls out in a timely manner.
- Resource Allocation: Identify the tools and budget necessary for each step.
- Risk Assessment: Understand potential drawbacks and prepare contingency plans, making room for flexibility.
"An effective roadmap not only provides a clear path forward but also allows you to adapt when the road gets bumpy."
Team Structure and Roles
Roles within the analytics framework need to be established thoughtfully. A well-defined team structure ensures each member knows what is expected of them and how their role correlates with the organizationâs strategy. Three pivotal roles stand out: Data Analysts, Business Intelligence Professionals, and Data Scientists. Each plays a key part in driving the analytics effort forward.
Data Analysts
Data Analysts are at the heart of processing and interpreting the data. They sift through raw information, spotting trends and providing actionable insights. The key characteristic of a Data Analyst is their analytical mindset. They possess the knack of translating data into stories that stakeholders can comprehend. This role is highly beneficial as it serves as a bridge between technical data and strategic decisions.
However, one unique feature of Data Analysts is their reliance on established methodologies and tools, which may limit their creativity when presenting insights. Still, their contribution to clarifying complex data sets can be the difference between success and failure.
Business Intelligence Professionals
Business Intelligence Professionals take it a step further by focusing on the overall performance of the company. They look beyond the raw numbers and ask the important questions like, "How can this data inform our business decisions?" Their key characteristic is a strong understanding of the business landscape: they blend analytics with industry knowledge. They hold a vital role in discerning what data matters most for decision-making processes.
One unique feature of this profession is their ability to utilize advanced analytical tools to create comprehensive dashboards and reports. This can be both a strength and a weaknessâthe widespread use of such tools means that some critical insights might be overlooked unless any additional interpretation is performed.
Data Scientists
Data Scientists are often viewed as the tech wizards of the analytics world. Equipped with machine learning capabilities and vast statistical knowledge, they can predict future trends based on historical data. Their key characteristic is versatility; they marry coding with analytical skills, enabling them to navigate through different programming languages and frameworks.
This versatility is a game changer, allowing them to customize models that can deliver deep analytical insights. However, the complexity of the tools and techniques they employ can sometimes alienate business users who are not as technically versed, posing a challenge that needs addressing.
By delineating these roles clearly, organizations can enhance their analytical strategy. Each position contributes a unique piece to the puzzle, making it imperative to implement a collaboration framework that promotes synergy and shared vision across the team.
Challenges in Developing an Analytics Strategy
In the realm of analytics, the pathway to effective implementation is often fraught with hurdles. Understanding these challenges is crucial because they can significantly influence the success of your analytics strategy. When organizations set out to harness the full potential of their data, they may stumble upon various roadblocks that can derail the entire initiative. Addressing these challenges proactively helps in cultivating a robust plan and ensures that the analytics strategy aligns with business objectives.
Cultural Resistance and Change Management
Cultural resistance is one of the primary obstacles that organizations face. Employees may see the introduction of an analytics framework as a threat to their existing roles or routines. Change is often met with skepticism; individuals may wonder if their jobs are at risk or if their current processes will be rendered obsolete. To overcome this, itâs essential to foster an environment that promotes openness and adaptability.
Organizations should consider the following strategies to ease cultural resistance:
- Communication is Key: Keeping the lines of communication open helps in dispelling myths and fears. Information sessions detailing the benefits of analytics can be pivotal.
- Involving Employees: Get employees involved in the planning and implementation process. Their insights can provide valuable context and might ease the transition.
- Training and Support: Offering comprehensive training programs can equip teams with the necessary skills, alleviating fears about job security and competence in new technologies.
Anticipating resistance and formulating an action plan not only aids successful implementation but also builds necessary buy-in from all stakeholders.
Managing Data Privacy and Compliance
With the ever-growing focus on data privacy, managing compliance is a critical challenge that cannot be overlooked. As laws and regulations around data usage become more stringent, organizations must ensure they are adhering to relevant standards.
The following considerations can aid in navigating the complexities of data privacy:
- Regular Audits: Conducting regular audits of data practices ensures that your organization remains compliant with local laws and policies.
- Data Minimization Principle: This principle emphasizes collecting only the data that is necessary for analysis, reducing the risk exposure.
- Training and Awareness: Awareness campaigns that educate employees about data privacy norms can create a compliant culture that prioritizes safe data handling practices.
- Choose Reliable Vendors: If your organization relies on third-party services, ensure they adhere to compliance and data privacy guidelines, mitigating risks of breaches.
"In todayâs data-driven world, neglecting data privacy can lead to severe repercussions, including financial penalties and loss of trust."
By prioritizing data privacy and compliance, organizations can protect against potential legal and reputational risks while cultivating responsible data-handling practices.
Navigating these challenges demands creativity and commitment. However, by addressing cultural resistance and focusing on data privacy and compliance, organizations can set a solid foundation for their analytics strategy.
Measuring Success and Performance Metrics
Establishing a solid analytics strategy is not merely about collecting and analyzing data; it's about understanding the outcomes of those analyses. This section delves into Measuring Success and Performance Metrics, laying bare the significance of tracking measurable objectives as organizations navigate their data journey. By measuring success effectively, businesses can ensure that their analytics initiatives not only align with strategic goals but also deliver tangible benefits.
The art of measurement sets the stage for learning and improvement. Organizations can detect patterns, validate assumptions, and pivot strategies if things don't pan out as expected. Without suitable metrics, a company risks drifting aimlessly, relying on hunches rather than data-driven decisions. The key here is to pin down what success looks like and to ensure that everyone is rowing in the same direction.
Key Performance Indicators (KPIs)
At the heart of measuring success are Key Performance Indicators (KPIs)âthe quantitative metrics that reflect how effectively an organization is achieving key business objectives. KPIs should be smartly defined, taking into consideration the specific goals of an analytics strategy.
- Types of KPIs to Consider:
- Financial KPIs: This includes revenue growth, net profit margin, or return on investment.
- Operational KPIs: This might cover customer satisfaction scores, time taken to convert leads, or inventory turnover rates.
- Customer-Centric KPIs: Focus on metrics like customer retention rates and net promoter scores (NPS).
Setting and regularly reviewing KPIs creates a feedback loop. Companies can identify what's working and what isn't. Moreover, it's essential to communicate these KPIs across teams, creating a shared understanding of targets and success. Don't overlook the importance of making KPIs adaptable; as the business environment shifts, so too should the measures of success.
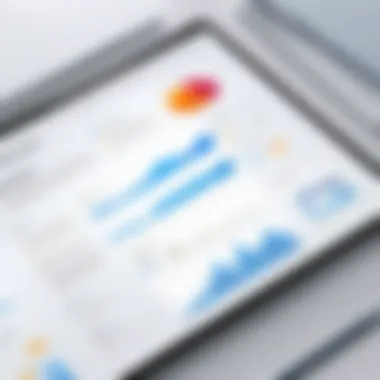
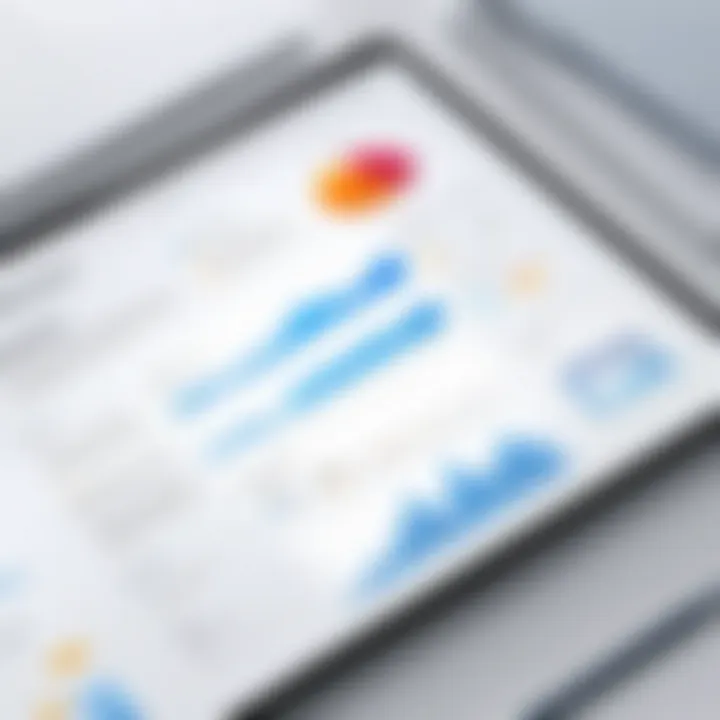
"What gets measured gets managed."
Feedback Mechanisms
Putting KPIs in place isn't the end of the story. Enter Feedback Mechanismsâthe subtle yet crucial elements that allow organizations to gather insights on performance and pivot strategies accordingly. These mechanisms act as the voice of the data, sharing reflections from the analytics efforts with the broader business context.
- Types of Feedback Mechanisms:
- Surveys and Questionnaires: Collecting direct customer feedback about their experiences can inform adjustments in service or product offerings.
- Analytics Dashboards: Visual presentations of data for continuous monitoring, offering real-time feedback on how well KPIs are being met.
- Regular Review Meetings: These can tie back to both KPIs and qualitative feedback, fostering open discussions among stakeholders and facilitating guided decision-making.
The true power of feedback mechanisms is their capacity to foster a culture of continuous improvement. When feedback is taken to heart, organizations can break free from stagnant practices and adapt swiftly to the changing market dynamics.
In summary, Measuring Success and Performance Metrics encompasses selecting relevant KPIs and actively implementing feedback mechanisms. This dynamic approach ensures that organizations can cultivate a responsive and informed analytics framework.
Continuous Improvement in Analytics Strategy
In the realm of analytics, the pursuit of continuous improvement isn't merely a luxury; it's a necessity. The landscape of data analytics is perpetually evolving, and organizations must adapt or risk falling behind. Continuous improvement in this context means regularly reviewing and refining analytical processes to maximize efficiency and relevance.
The iterative nature of analytics allows businesses to learn from past experiences. By establishing feedback loops, organizations can pinpoint what works, what doesnât, and subsequently enhance their strategies. Embracing this iterative process is vital to staying ahead of competitors. With data at the core, the goal is to transform raw insights into actionable information that informs decision-making.
Iterative Process of Evaluation
The iterative process of evaluation is all about getting into a rhythm of consistent reassessment. Organizations that actively analyze their analytics strategies can make adjustments in real time. Think of it as tuning a musical instrument; it requires ongoing tweaks to ensure that everything is in harmony.
Implementing a regular evaluation framework can yield substantial benefits:
- Identify Bottlenecks: Keeping a keen eye on data flow helps expose excessive delays or inaccuracies that can hinder performance.
- Enhance Data Quality: Through constant analysis, firms can enforce better data collection methods, reflecting on their effectiveness and making necessary changes.
- Increase User Engagement: Regularly evaluating how end-users interact with analytics tools can highlight areas for enhancement, thus improving satisfaction.
To effectively manage this iterative evaluation, organizations should adopt the following:
- Set Clear Timelines for Review: Decide how often evaluations should occur, whether that be quarterly or biannually.
- Engage Stakeholders: Ensure the inclusion of staff from different departments. Their insights can greatly enhance the understanding of analytics' impact.
- Leverage Technology: Utilize automation tools that offer performance analytics to facilitate seamless evaluations.
"Success is not final; failure is not fatal: It is the courage to continue that counts." - Winston S. Churchill
Adapting to Emerging Trends
In a fast-paced digital world, adaptability is king. Emerging trends in analyticsâlike artificial intelligence, machine learning, and real-time analyticsâpose both challenges and opportunities. Organizations that remain rigid in their approaches are likely to be overtaken by more flexible competitors who readily embrace new technologies.
When adopting new trends, consider these aspects:
- Continuous Learning Opportunities: Invest in training programs to ensure teams are well-versed in the latest tools and technologies. This increases overall organizational agility.
- Scouting Innovations: Make it a priority to monitor industry developments and evaluate how these innovations could fit into your existing strategy. Find ways to incorporate AI-predictive models to understand customer behaviors more acutely.
- Stay Aligned with Business Goals: Each emerging trend should be scrutinized for its alignment with the organization's overarching objectives. Not everything trendy will suit every business, so discern what really adds value.
Ultimately, embracing emerging trends while iteratively improving existing methods leads to a dynamic analytics strategy that is both relevant and robust. The secret lies in the ability to oscillate seamlessly between evaluating past methods and adapting to future innovations.
Case Studies and Best Practices
The discussion around case studies and best practices is pivotal in the realm of analytics strategy. These narratives not only bring to life the theoretical aspects of analytics but also ground them in the practical world. They present real-world examples of how various organizations have harnessed analytics effectively or encountered pitfalls along the way.
Incorporating case studies fosters a deeper understanding, as they illustrate the nuances of implementation that can often be overlooked in textbooks. They shed light on both triumphs and failures, enriching the knowledge base of those looking to build or refine their analytics strategies. More importantly, they inspire confidence among decision-makers, showcasing clearly defined paths to success and simultaneously serving as cautionary tales about obstacles and missteps.
The key benefits of employing case studies include:
- Contextual Learning: Helps professionals understand how theoretical concepts appear in practical scenarios.
- Benchmarking: Organizations can set their pace by looking at peers' successes and failures, thus avoiding common mistakes.
- Inspiration: Success stories can motivate teams, encouraging innovative thinking and proactive behaviors in addressing analytical challenges.
Ultimately, this section pulls together the collective wisdom gained across different sectors and companies, emphasizing that a thoughtful and informed analytics approach is fundamental for guiding business decisions effectively.
Successful Implementation Examples
Successful implementation examples illustrate how organizations have leveraged their analytics strategies to achieve tangible benefits. Companies like Starbucks and Netflix serve as shining beacons in this respect.
Starbucks employs advanced analytics to enhance customer experience and optimize its supply chain. Using data gathered from customer purchases, they tailor their promotions and product offerings to align with customer preferences. Notably, their My Starbucks Reward program pays dividends, translating data into actionable strategies that drive sales and foster loyalty. By analyzing customer behavior and purchasing trends, Starbucks continually refines its menu and marketing tactics, ultimately resulting in increased sales.
Netflix is another innovator in this domain. Utilizing user data, it personalizes viewing recommendations, boosting user retention. Its analytics approach doesn't only stop with recommendations; by examining viewer data, Netflix can determine which shows to produce. This data-driven decision-making contributed significantly to the success of original productions like House of Cards, shaping the company into a leading player in the entertainment industry.
Through these examples, we see the power of data in action and how a well-defined analytics strategy aligns with broader business objectives for success.
Lessons Learned from Failures
Despite the illuminating successes, the journey in analytics isn't without its share of missteps. There are valuable lessons to extract from failures too. Consider Target's infamous miscalculation of customer preferences, which led to a major public relations mishap when they mistakenly sent baby-related coupons to a teenage girl. This incident highlighted a significant gap in understanding customer data, raising questions about the ethics of data handling and analysis.
Another example is Kodak. Once a giant in the photography industry, Kodak did not adapt quickly enough to the digital revolution. Despite having the technology for digital cameras, internal analysis led to a failure in decision-making, as executives underestimated the shift in consumer preferences toward digital photography. This failure to leverage analytics in making strategic decisions culminated in a dramatic loss of market share and eventual bankruptcy.
These instances serve as reminders: while analytics equips organizations with the tools to improve and innovate, a misinterpretation, lack of ethical considerations, or poor decision-making can lead to downfall. Thus, a critical and reflective approach to analytics is essential.
"Success is not final, failure is not fatal: It is the courage to continue that counts."
â Winston Churchill
The End
Concluding this article on analytics strategy frameworks brings to light several crucial elements that cannot be overstated. An effective analytics strategy is not simply a collection of data points or fancy software; itâs a holistic approach that intertwines organizational goals with practical data-driven methodologies.
Recap of Key Insights
To summarize the discussion, here are the fundamental takeaways:
- Clear Objectives: Every analytics journey begins with distinctly defined goals. Without knowing the destination, navigating the vast sea of data can lead to a drifting aimlessly.
- Data Governance and Management: Effective governance ensures that data is not just plentiful but quality-driven, accessible, and secure. This is the backbone of trust within an organization.
- Analytical Techniques: The choice of analytical methodsâwhether descriptive, predictive, or prescriptiveâhighly determines the insights derived and their relevance to real-world applications.
- Technology Integration: Employing the right tools, whether they be visualization software or machine learning platforms, equips teams to turn raw data into actionable intelligence.
- Continuous Improvement: Analytics is not a one-time gig. Itâs a continual cycle of learning, adapting, and evolving. Organizations must be nimble in their strategies, ready to pivot as they encounter new challenges and opportunities.
Each of these points emphasizes that a robust analytics strategy is essential for leveraging data to make informed business decisions. Itâs not just beneficial but rather fundamental in maintaining a competitive edge.
Emphasizing the Importance of a Tailored Approach
We cannot forget that a one-size-fits-all mentality in analytics often leads to subpar results. Instead, an organization must craft its analytics strategy based on its unique contextâconsidering factors such as industry dynamics, company culture, and resource availability. Tailoring ensures that the analytics efforts resonate with the specific needs of the business, thereby increasing the likelihood of achieving desired outcomes.
In essence, fostering a personalized approach not only boosts engagement but also enhances the practical application of insights gleaned from data, making analytics drive meaningful change. Therefore, rather than hurriedly adopting trends, organizations should prioritize deliberate strategies that reflect their environments for sustainable success.
Ultimately, as businesses venture deeper into the realm of data, their approach to analytics should mature in parallelâcommitting to a roadmap that is not only structured but adaptable, ensuring they remain ahead in an ever-evolving landscape.