Analyzing Databricks Costs on AWS: Key Insights

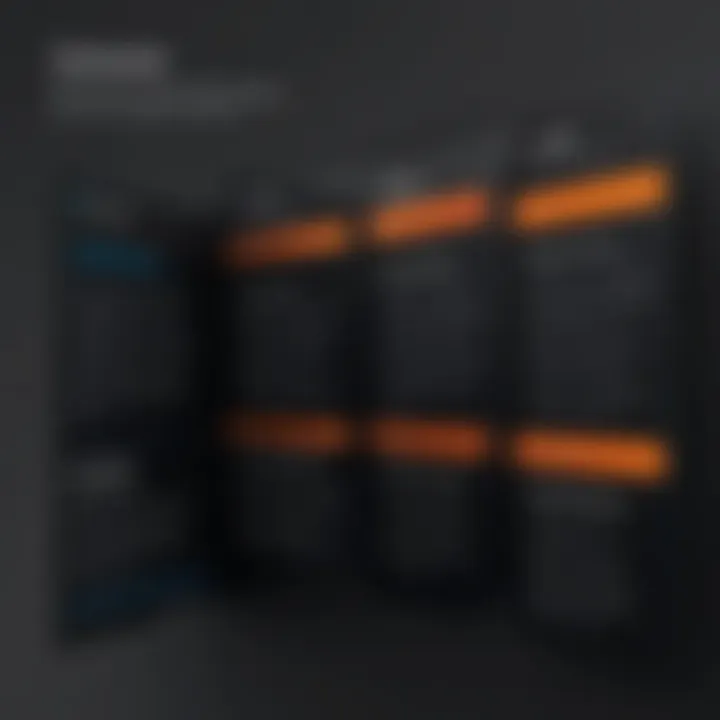
Intro
As businesses continue to dive deeper into the digital age, the role of data analytics has become increasingly pivotal. Organizations are increasingly dependent on data to make informed decisions, optimize operations, and drive innovation. At the heart of this journey lies Databricks, a powerful analytics platform that operates seamlessly on the Amazon Web Services (AWS) cloud infrastructure. Despite its advantages, the costs associated with utilizing Databricks on AWS can be complex and dynamic. This piece aims to provide a lucid exploration of those costs, helping you disentangle the intricate web of pricing models, resource allocation, and effective cost management strategies.
Technological Research Overview
Recent Technological Innovations
Databricks represents a significant advancement in the landscape of data processing and storage. By combining Data Lake architecture with scalable compute resources, it facilitates vast amounts of data analysis at lightning speeds. Innovations like Delta Lake have enhanced data reliability and performance, allowing for real-time data processing. Moreover, with integrated machine learning capabilities, users can transition from data gathering to actionable insights more swiftly than ever before.
Impact on Business Operations
The integration of Databricks into AWS environments has had a marked impact on operational efficiency. Companies can now harness vast datasets to derive predictive analytics, supporting data-driven decisions. This capability leads to smarter resource allocation and can significantly lower operational costs. However, understanding the cost implications of such integrations is essential for ensuring ROI.
Future Technological Trends
Looking towards the horizon, the trend seems to be leaning towards greater automation within cloud analytics platforms. Concepts such as AutoML and increased AI capabilities are likely to become more prevalent, further transforming how businesses engage with data. Keeping abreast of these developments could unlock new opportunities for cost-effective data processing.
Understanding Databricks Pricing Models
Databricks offers several pricing structures based on consumption, which can vary widely depending on specific business needs.
- Standard Pricing: Charges based on compute and storage usage, allowing flexibility for businesses to scale resources up or down.
- Pay-As-You-Go: Ideal for enterprises looking for a straightforward method to manage costs without long-term commitments.
- Reserved Instances: For businesses that anticipate a stable workload, this model can provide significant savings compared to on-demand pricing.
Understanding the nuances of these pricing models is crucial for optimizing cloud expenses. Consider how each option aligns with your organization's data strategy and anticipated usage.
The Importance of Resource Allocation
A critical area affecting costs is how resources are allocated within Databricks on AWS. In the cloud environment, the principle of "right-sizing" your resources can make a significant dent in overall expenses. Here are factors to consider:
- Cluster Configuration: Optimizing the configuration of your clusters can lead to cost reductions.
- Job Scheduling: Effective scheduling of jobs can help manage compute costs by utilizing cheaper resources during off-peak hours.
- Monitoring and Analytics: Utilize built-in tools to monitor resource usage for further optimization. The ability to understand where and how resources are consumed can provide insight into potential savings.
Cost Management Strategies
To navigate the costs effectively, several strategies can be employed:
- Budgeting and Forecasting: Keeping abreast of your usage will enable more accurate budgeting and forecasting for data analytics expenses.
- Automated Scaling: Leveraging automated scaling features can adjust resources based on workload in real-time, ensuring you're only paying for what you use.
- Utilizing Free Tiers: AWS often provides free tiers for initial usage which can serve as a testing ground before committing significant investments.
Through the prudent application of these strategies, organizations can harness the full potential of Databricks on AWS while minimizing unnecessary expenditures.
Prelims to Databricks and AWS
When talking about modern data solutions, the role of Databricks on AWS cannot be overstated. The synergy between these two powerhouses offers a robust platform for data engineering, analytics, and machine learning. Understanding how these entities operate together is crucial for businesses that aim to leverage data effectively.
Overview of Databricks
Databricks is a cloud-based platform that simplifies big data and machine learning processes. It enables users to create and share interactive notebooks, collaborate on data projects, and harness Apache Spark’s speed and flexibility. This platform supports multiple languages such as Python, R, and SQL, making it appealing to teams with diverse skillsets.
The significant ease of use is one of its hallmark traits; users often refer to it as a "one-stop shop" for data applications. Databricks eliminates much of the setup friction that typically accompanies data management and analysis—leading to increased productivity. With its managed Spark service, organizations can dive directly into the analytics without the hassles of infrastructure management.
In addition to that, the integrated machine learning capabilities are a game-changer. It allows for seamless transitions from data preparation to training models, ultimately shorting the time from idea to implementation.
The Role of AWS in Data Engineering
AWS plays a pivotal part in the landscape of data engineering, providing the foundation that powers Databricks. With its expansive ecosystem of services—like Amazon S3 for storage, EC2 for compute power, and IAM for security—AWS can accommodate the complex needs of data workloads.
Organizations using Databricks on AWS enjoy the flexibility of the cloud without the significant overhead traditionally associated with on-premises solutions. The AWS infrastructure supports auto-scaling, enabling companies to adjust resources on the fly according to their workload demands. This elasticity is key in managing costs effectively and ensuring that resources are not left idle.
Further, AWS's robust security framework complements the data capabilities of Databricks. Providing advanced features like data encryption and compliance certifications, organizations can trust their sensitive data is safeguarded. In a world where data breaches loom large, this feature alone brings peace of mind to decision-makers.
"The combination of Databricks and AWS is not just about performance; it’s about unlocking the full potential of data-driven decision-making."
Understanding Databricks Pricing Structure
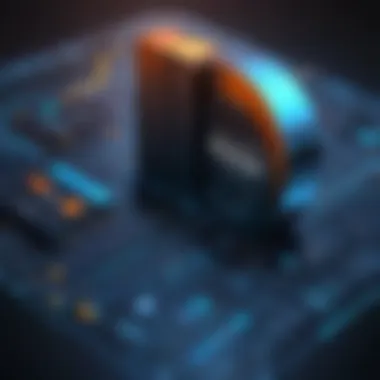
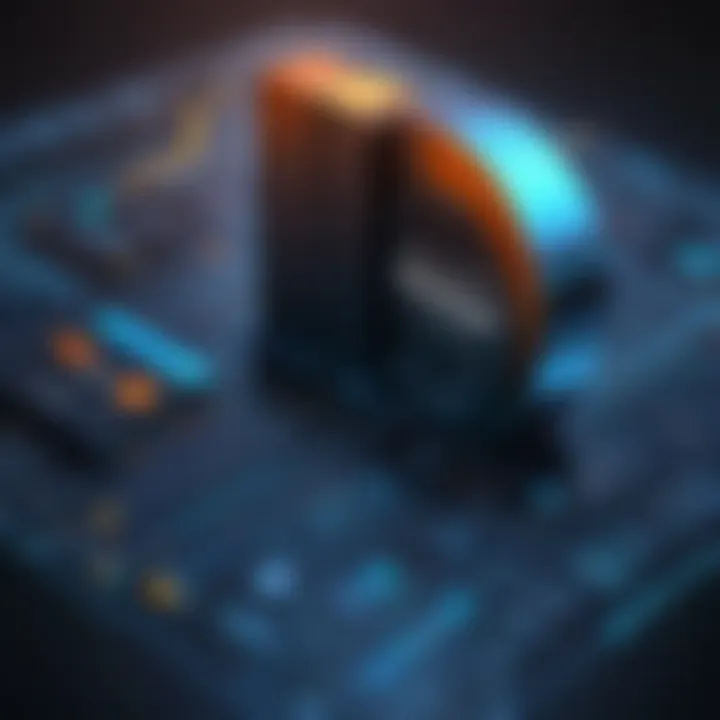
Understanding the pricing structure of Databricks is vital for any organization that is looking to harness the power of big data analytics on the AWS platform. The intricacies of costs can catch many off guard if they’re not thoroughly examined.
For businesses, knowing how expenses accumulate is essential to balance efficiency with effectiveness. The Databricks pricing model isn’t just about raw numbers or spreadsheets; it’s a lens into how resources are allocated and used in the cloud. When analyzing costs, organizations can identify areas that may lead to wasted funds and discover potential for optimization. Thus, a deep understanding here directly ties into better budgeting and forecasting.
Several elements come into play when discussing Databricks pricing. This includes workspace charges, compute costs, and storage fees. Each element combines to create the total cost of ownership.
Workspace Charges
Workspace charges are basically the entry point for using Databricks. In layman's terms, you could think of it as the membership fee to access the platform’s features and capabilities. Having a workspace means you can collaborate on notebooks, run queries, and manage clusters easily — but it does come with a price attached.
Different types of workspaces can incur different charges. For instance, standard workspaces may have a lower flat fee, while premium workspaces provide enhanced functionalities at a higher cost.
Organizations should carefully assess their needs. It might be tempting to opt for the premium services right away, thinking they might need that functionality down the line. However, if the current projects don't warrant premium tools, sticking to the standard offering can save quite a bit.
Some key takeaways regarding workspace charges:
- Subscription-based charges: Monthly fees may vary based on usage and tier.
- Scaling needs: More team members mean possibly needing more seats, affecting the overall charge.
- Feature access: Some features might only be available in higher-tier workspaces, nudging companies to choose wisely.
"Choosing the right workspace model can often dictate the entire budgeting process. A small misjudgment can lead to cost overruns."
Compute Costs Explained
Compute costs represent one of the most significant portions of any bill when using Databricks. Unlike fixed costs associated with workspaces, compute costs can fluctuate based on a variety of factors, notably usage spikes and job types. This is particularly relevant for businesses running data pipelines that vary in workload.
Databricks operates on a pay-as-you-go model for compute resources, meaning that the more you use, the more you pay. This flexibility can be beneficial — during off-peak hours, lesser processing power means reduced costs. However, it also requires organizations to monitor their workload closely to avoid any unexpected spikes in charges.
Key considerations for managing compute costs include:
- Cluster types: Different types of clusters cater to different needs (e.g., interactive vs. job clusters).
- Job sizes: Some jobs require more power than others. Planning can help optimize costs.
- Use of spot instances: Using spare computing power can significantly decrease costs but comes with its own risks of interruption.
Delving into these compute costs isn't just accounting; it's about forecasting and planning for future expenses.
Storage Costs Overview
When we talk about storage costs, we’re stepping into the territory of data retention and management. Databricks on AWS uses a variety of storage options, such as Amazon S3, to house data. Each option comes with its own pricing model that must be factored in.
The nature of the data stored can impact overall costs. For instance, if you’re working with large datasets that must be retained indefinitely, it’s wise to choose a more economical storage option that fits this need.
Key factors including:
- Data lifecycle management: Archiving older data can lower costs significantly.
- Data access frequency: Different storage classes can save money based on retrieval needs (e.g., frequent vs. infrequent access).
- Integration with other services: Making use of integrated services within AWS can also streamline costs and drive efficiencies.
Ultimately, understanding storage cost implications is integral for holistic cost management strategies within Databricks on AWS.
Factors Affecting Databricks Costs on AWS
Understanding the factors impacting Databricks costs on AWS is crucial for organizations aiming to navigate their cloud expenditure effectively. This section dives into various elements that can influence these costs, including resource allocation, job scheduling, and scaling strategies. Each of these components plays a pivotal role in shaping the financial landscape for businesses leveraging Databricks within AWS.
Resource Allocation and Usage
At its core, resource allocation and usage dictate how efficiently an organization can operate within the Databricks environment. When resources are allocated wisely, it leads to cost savings and optimized performance. Conversely, mismanagement can quickly drain budgets without yielding significant benefits.
A few key points to consider include:
- Instance Selection: The choice of AWS instances directly affects both performance and cost. Different instance types have distinct pricing models and performance characteristics. Organizations should evaluate their workload needs and choose wisely to balance cost and efficiency.
- Idle Resources: Keeping resources running that are not in active use leads to unnecessary costs. Implementing measures to shut down inactive clusters can lead to savings that positively impact the bottom line.
- Utilization Metrics: Monitoring utilization is essential. If the compute power is underused, costs can be higher than necessary. Utilizing Databricks’ built-in tools can provide insights into resource usage patterns, enabling targeted adjustments.
Making informed decisions in resource allocation can lead to not only reduced costs but also improved performance across data-intensive tasks.
Job Scheduling and Optimization
Job scheduling is another fundamental aspect affecting costs in Databricks. Properly scheduling jobs according to peak usage times can significantly cut down on expenses. Think of it like planning a road trip: if you start your journey at off-peak travel times, you save time and fuel.
Consider the following:
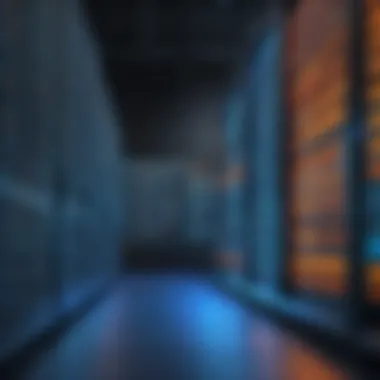
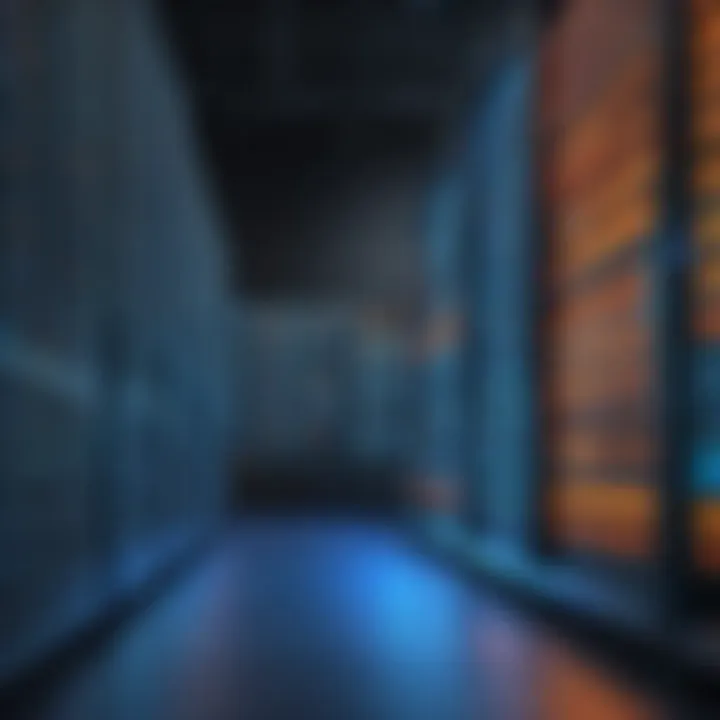
- Batch vs. Real-Time: Understanding the difference between batch processing and real-time processing is key. For some workloads, batch processing, which allows for more flexibility in timing, can be more cost-effective.
- Time-Window Scheduling: Implementing a job scheduling strategy that leverages known time windows can effectively align resource usage with lower-cost periods. This often means scheduling non-time-critical jobs for when demand is low.
- Dynamic Job Management: Utilization of advanced features such as job dependency management and automated retries can optimize workflows and reduce cost overhead by minimizing unnecessary retries and keeping resources in check.
Job scheduling, when executed adeptly, allows organizations to maximize savings while ensuring timely data processing.
Scaling Strategies
Scaling is a double-edged sword in the cloud world. It’s easy to get caught in a cycle of over- or under-provisioning resources, which can wreak havoc on budgets. A sound scaling strategy can help businesses maintain the delicate balance of performance and expense.
Key strategies include:
- Autoscaling: Automating the scaling process can lead to efficient resource usage. With Databricks' autoscaling feature, clusters can automatically expand or contract based on workload demand, ensuring that resources are aligned with current needs.
- Preemptive Scaling: Instead of waiting until peak usage hits, consider using historical data to predict upcoming requirements. This proactive approach to scaling can prepare your systems for heavy loads without the last-minute scramble.
- Cost Monitoring and Alerts: Set up systems to monitor costs actively and trigger alerts at certain thresholds. This ensures that scaling decisions are informed and timely, avoiding unexpected spikes in expenditures.
Scaling strategies, when implemented effectively, can transform cost management and allow organizations to be agile in their data operations.
"The art of managing costs in cloud environments like AWS is about leveraging the tools available to strike the right balance between performance and spending." - Source
Cost Management Strategies for Databricks on AWS
Managing expenses effectively is crucial for organizations utilizing Databricks on AWS. Without a solid grasp of cost management, even a small oversight can organically lead to ballooning expenses that catch businesses off guard. Understanding how to navigate the intricacies of cost structures ensures that companies can focus on their analytical needs without feeling the financial strain. This section dives into specific elements, benefits, and considerations surrounding effective cost management.
Implementing Autoscaling Features
Autoscaling features are a game changer when it comes to managing costs. They allow businesses to automatically adjust resources based on current workloads. Think of it this way: it’s akin to the thermostat in your home adjusting heat or air conditioning in response to temperature. When usage spikes, Databricks appears to increase resources seamlessly; when workloads decrease, it pulls back. This not only guarantees efficiency but also ensures that you’re not paying for unused capacity.
However, implementing autoscaling requires careful configuration. You need to determine the optimal thresholds for scaling up or down. Too sensitive adjustments can lead to frequent resource changes, which might paradoxically inflate costs. Companies ought to conduct thorough testing and analytics to find the sweet spot that balances performance while keeping costs manageable.
Using Spot Instances Effectively
Key strategies for leveraging spot instances include:
- Plan Ahead: Understand the workflow to predict the demand accurately. Jobs that can be scheduled flexibly work best here.
- Job Resilience: Utilize managed services that allow jobs to resume if they’re interrupted, which is a common case with spot instances.
- Cost Monitoring: Keep abreast of the current spot prices. Sometimes, it makes sense to mix standard instance types with spot instances to ensure that critical tasks don’tget disrupted if prices rise unexpectedly.
Monitoring and Analyzing Costs
Ongoing monitoring of expenses tied to Databricks is just as critical as the initial planning stage. To keep expenses in check, organizations should leverage AWS’s cost management tools to get insights into where their money is going. Dashboards that track usage can become invaluable, providing a clear picture of which resources are running up the bill.
Some effective steps include:
- Establish Clear Budgets: Setting a budget allows for easier identification of anomalies. If costs spike unexpectedly, it warrants immediate investigation.
- Categorize Expenses: Dividing expenditures by team, project, or department helps pinpoint where costs might be climbing.
- Regular Reviews: Establish frequent check-ins on expense reports to ensure nothing slips through the cracks. It’s essential to find patterns and identify areas for potential savings.
"The key is to be proactive rather than reactive, adopting a continuous approach to managing costs rather than waiting for surprises on the monthly bill."
By effectively employing these strategies, companies can optimize their Databricks usage on AWS, minimizing costs while not sacrificing performance. Ultimately, being informed about the various components of cost management sets the stage for smarter, more sustainable business operations.
Real-World Case Studies of Databricks and AWS
When considering the implications of Databricks running on AWS, examining actual use cases serves as a reality check. These real-world case studies not only shed light on how companies are leveraging this powerful combination but also highlight the tangible benefits and challenges they've encountered. In doing so, they provide insights that guide other organizations in making informed decisions.
Successful Implementations
Successful implementations of Databricks on AWS come from diverse sectors, showcasing versatility and potential. For instance, a retail giant was able to enhance its supply chain management through robust data integration and real-time analytics. By employing Databricks, they unified multiple data sources, allowing data scientists to extract valuable insights rapidly. The outcome? A substantial reduction in inventory costs by over 15% and improved demand forecasting accuracy.
- Data Collaboration: With Databricks, teams across departments worked seamlessly. Utilizing notebooks for collaborative coding, they boosted productivity and innovation.
- Scalability: The organization effortlessly scaled infrastructure based on demand peaks, particularly during holiday seasons, thanks to AWS’s flexibility.
- Cost Efficiency: Effective use of spot instances resulted in reduced compute costs, maximizing ROI while keeping analytics time-sensitive.
Such successes are not just flukes. Other firms in financial services and healthcare spheres have experienced similar advantages. For example, a healthcare provider employed Databricks for analyzing patient data, resulting in better patient outcomes through data-driven decisions. Joining forces with AWS enabled them to securely manage sensitive health records while extracting insights at scale. The implementation led to a 25% increase in care efficiency, proving that thoughtful execution can yield remarkable results.
Lessons Learned from Failures
On the flip side, some implementations of Databricks on AWS didn't go quite as planned. Learning from these experiences is equally crucial. A notable tech startup attempted to use Databricks but overlooked the importance of data governance. The lack of structured data management resulted in significant compliance issues, leading to hefty fines and a tarnished reputation.
- Planning and Governance: It quickly became clear that without a robust plan for data governance and security, even the best technology could falter.
- Training and Skillsets: Another failing was underestimating the need for data literacy among staff. Users struggled to leverage the platform’s capabilities, leading to wasted resources and frustrated teams.
- Resource Over-Allocation: In one instance, a company recklessly allocated vast computational resources without evaluating performance needs. This over-provisioning led to soaring costs, overshadowing potential benefits.
The road to success is paved with failures; learning from these missteps can ultimately drive innovation and improvement, fostering a more resilient organization.
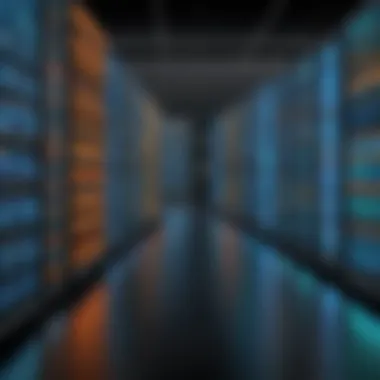
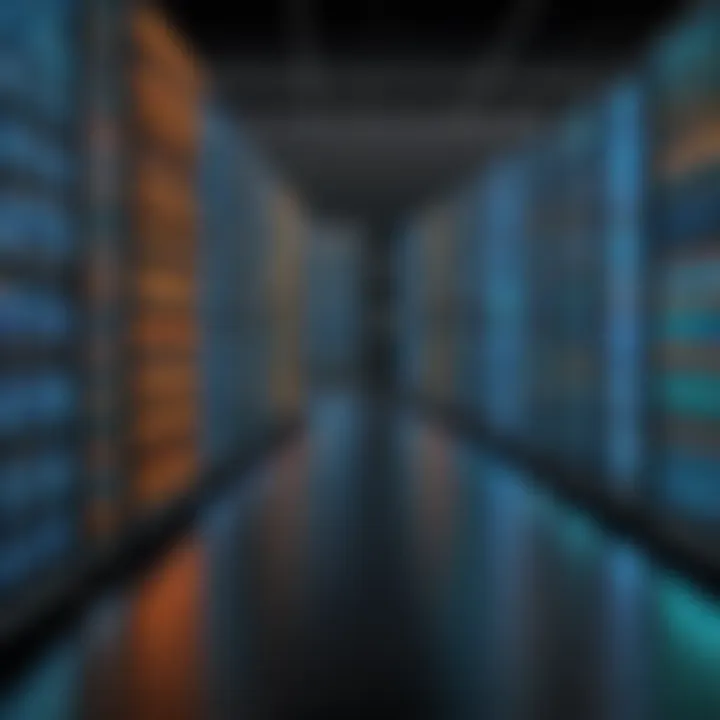
These cautionary tales underscore the necessity of preparation and adaptability when working within this ecosystem. By examining both successful implementations and lessons from failures, businesses can gain a rounded perspective. This understanding can steer them toward better decisions that ultimately enhance their data capabilities while keeping costs in check.
Comparative Analysis: Databricks on AWS vs. Other Platforms
Understanding the costs associated with Databricks is critical for any organization looking to leverage the power of cloud computing for data analytics. While AWS is a popular choice for running Databricks, it's worthwhile to compare how this platform stacks against alternatives like Google Cloud and Microsoft Azure. Decision-makers seeking effective data solutions must consider several crucial elements that impact not just initial investment but also long-term operational costs and performance efficiencies.
When analyzing different platforms, organizations often take into account the following aspects:
- Pricing Models: Each cloud provider has a distinct pricing structure that can significantly affect overall expenses.
- Feature Set: The capabilities offered by each platform can aid in selecting the right environment for unique data workloads.
- Integration: Some businesses may prefer specific cloud ecosystems for better integration with existing services.
- Performance Metrics: Key performance indicators, such as compute power and processing speed, can dictate which platform provides the best value for money.
This comparative analysis aims to shed light on both Databricks execution on AWS and its competitors, giving potential users a clearer picture of what to expect in terms of costs and benefits.
Databricks and Google Cloud
Databricks on Google Cloud presents an interesting alternative for organizations looking to utilize cloud data platforms. Google Cloud offers a competitive pricing model, often appealing for startups and smaller enterprises due to its flexible billing options. Its serverless option allows companies to pay only for the resources they actually utilize, which can lead to significant cost savings.
On the technical side, Google Cloud integrates well with tools like BigQuery, allowing for efficient data querying and analytics. However, it can also lead to complex data management tasks as datasets grow. Organizations must weigh the ease of use against potential overheads when integrating multiple systems.
Databricks and Microsoft Azure
Microsoft Azure is another prominent player in the cloud scene, providing robust support for Databricks applications. Azure combines the best of two worlds: the complete, scalable features of Databricks along with Microsoft’s enterprise-grade technology. This integration often results in a seamless experience for businesses already utilizing Microsoft products, particularly for those leveraging Office 365 and other Azure services.
Cost considerations also play a pivotal role here. Azure typically charges for Databricks based on the Databricks Unit (DBU), thus making understanding expenses a bit more complicated compared to straightforward compute pricing. Yet, its discount options for reserved capacity can drive down costs even further for long-term users, making it an attractive choice for organizations willing to commit to longer service contracts.
The choice of cloud platform is not merely about cost but about aligning the technology with business objectives.
Future Trends in Databricks Cost Structures
In the continuously evolving world of cloud computing, keeping an eye on future trends in Databricks cost structures isn't just prudent; it's crucial. As organizations increasingly turn to Databricks on AWS for their data analytics needs, understanding how the landscape of costs is changing can provide key insights for financial planning and operational efficiency. By analyzing emerging technologies and anticipating shifts in pricing models, businesses can prepare for more strategic decision-making and ensure optimal use of their budgets.
Emerging Technologies and Their Impact
Emerging technologies are reshaping how organizations interact with data, and they hold significant implications for cost structures within Databricks. For instance, advancements in artificial intelligence and machine learning are not just buzzwords; they are materially changing performance metrics and potential costs.
"The integration of AI tools into Databricks could potentially streamline workflows and reduce resource consumption, effectively lowering costs in the long run."
The impact of automation, for example, can’t be overstated. Automation tools can help in maintaining optimal resource allocation, effectively scaling usage based on real-time needs, and thus leading to savings. Moreover, developments in quantum computing, although still in early stages, could offer enhanced processing power at a fraction of the cost by executing complex computations faster than traditional methods.
Another crucial aspect is the rise of serverless computing options. These technologies enable organizations to pay only for the computing resources they actually use, eliminating the idle spending that often plagues traditional cloud services. With Databricks adopting serverless architectures, users might find themselves benefiting from more predictable cost structures, which aligns with their operational strategies while keeping budgets in check.
Anticipated Changes in Pricing Models
As the competitive landscape becomes more intense, pricing models associated with Databricks on AWS will likely evolve. Many organizations are already witnessing shifts toward more flexible subscription models designed to cater to specific use cases or user needs. This adaptability allows companies to scale their service accordingly, which can lead to significant cost savings over time.
The subscription models could introduce tiered pricing based on usage patterns, allowing organizations to choose plans that match their operational scale and budget. Such a model not only democratizes data analytics access but can also mitigate the cost spikes often associated with data processing and storage.
Moreover, we may see a transition to pay-as-you-go offerings that align pricing with actual usage. This dynamic billing could promote healthier spending habits as organizations become more aware of their resource consumption.
As technology advances and competition heats up, staying ahead of pricing changes will be essential for effective financial planning. Businesses that keep close tabs on these trends will be better positioned to leverage Databricks on AWS efficiently, ensuring they aren't caught off guard by unexpected costs or pricing adjustments.
In summary, the future of Databricks cost structures is poised for significant transformation driven by advancing technologies and shifting pricing models. By preparing for these changes, organizations can harness the full potential of Databricks while keeping a firm hold on their budgets.
Finale
In the realm of data analytics, understanding the costs that come with utilizing Databricks on AWS is not just advisable—it's essential. As organizations increasingly pivot towards cloud-based solutions to handle their vast arrays of data, finding a balance between performance and cost-effectiveness emerges as a paramount concern. This section encapsulates the key insights gathered throughout the analysis, underscoring the importance of continuous evaluation when it comes to managing expenses on such platforms.
Final Thoughts on Cost Optimization
Cost optimization can be likened to maintaining a well-tuned engine. If you’re not periodically checking the parts, you may end up with performance issues down the line. The same principle applies when working with Databricks on AWS. Every decision—be it in resource allocation, job scheduling, or even the choice of compute instances—can reverberate through your financial structure.
Strategies to Consider
- Embrace Autoscaling: By allowing your resources to scale automatically with demand, you prevent over-provisioning of resources that could otherwise rack up unnecessary costs.
- Monitor Usage Regularly: Keeping a watchful eye on resource consumption can provide insights into potential savings. Regular audits of your databricks instances often reveal areas where resources might be sitting idle.
- Leverage Spot Instances: Spot instances are often cheaper than standard on-demand pricing. Understanding when and how to use them can lead to significant savings.
- Implement Cost Monitoring Tools: Tools like AWS Cost Explorer can provide insights into spending patterns and highlight peaks in usage that might be optimized further.
In wrapping up, while Databricks offers powerful tools for data flavoring and analytics, it’s vital for organizations to cultivate a strong grasp of the financial implications of their cloud usage.
"Cost efficiency isn't just about reducing expenses; it’s about maximizing value from your investments."
As you head into cloud projects, keep these strategies and insights in mind. The road to cost optimization is continuous and evolving, but armed with the right knowledge, organizations can navigate it effectively, ensuring that their investments translate not just into operational capabilities, but also into financial prudence.