Unleashing the Potential of Data Analytics in Business: A Comprehensive Exploration

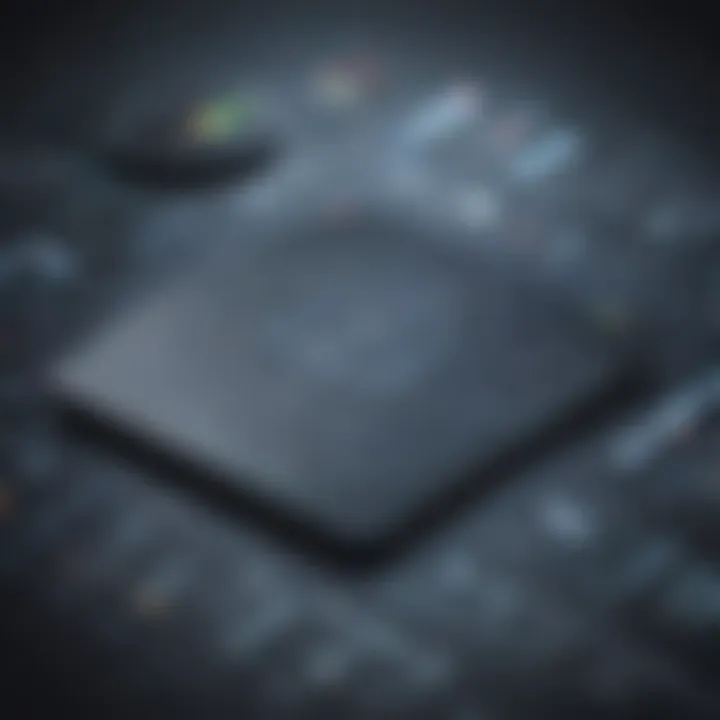
Technological Research Overview
Data analytics play a pivotal role in modern business operations, driving strategic decisions and improving overall performance. Recent technological innovations have revolutionized the landscape by introducing advanced analytics tools that offer unparalleled insights. These innovations have significantly impacted various aspects of business operations, from marketing strategies to supply chain management, reshaping how organizations approach decision-making processes. Future technological trends in data analytics indicate a continued evolution towards more sophisticated algorithms and automation, presenting new opportunities and challenges for businesses seeking to harness the power of data.
Data Analytics in Business
The importance of data analytics in business cannot be overstated. By utilizing tools for data analysis, companies can extract valuable insights from large datasets, enabling them to make informed decisions and gain a competitive edge in the market. Case studies showcasing data-driven decisions serve as concrete examples of how businesses have leveraged analytics to optimize strategies, improve operational efficiency, and drive growth. These real-world examples offer invaluable lessons for professionals looking to enhance their own data analytics capabilities and incorporate data-driven approaches into their decision-making processes.
Cybersecurity Insights
In an increasingly digitized world, cybersecurity has become a paramount concern for businesses across industries. Conducting a threat landscape analysis is crucial for understanding and mitigating potential risks to data security. Implementing best practices for cybersecurity, such as encryption protocols and regular vulnerability assessments, is essential for safeguarding sensitive information and maintaining the trust of stakeholders. Moreover, complying with regulatory requirements in cybersecurity is not only a legal necessity but also a strategic imperative to protect against data breaches and cyber threats.
Artificial Intelligence Applications
The integration of artificial intelligence (AI) in business automation has paved the way for enhanced operational efficiency and innovation. AI algorithms and applications have streamlined processes, from customer service interactions to predictive analytics, driving productivity and cost savings for organizations. However, ethical considerations in AI implementation are critical to ensure responsible and transparent use of these technologies. Addressing concerns such as bias in AI algorithms and data privacy issues is imperative for building trust with consumers and maintaining ethical standards in AI-driven decision-making.
Industry-Specific Research
Different industries exhibit unique trends in technological research, each leveraging technology to address specific challenges and opportunities. In the finance sector, tech research focuses on enhancing transaction processing speed, improving risk management techniques, and enabling more efficient financial services. Healthcare technological advancements aim to streamline patient care, enhance diagnostic capabilities, and facilitate data-driven treatment decisions. In the retail industry, tech solutions are geared towards enhancing customer experiences, optimizing supply chain management, and implementing personalized marketing strategies. Industry-specific research highlights the tailored applications of technology in diverse sectors, offering insights into how different industries are harnessing innovation to drive growth and achieve strategic objectives.
Introduction to Data Analytics
In this expansive guide to 'Unlocking the Power of Data Analytics in Business,' the crucial role of data analytics in modern business landscapes unfolds. The understanding of data analytics is not merely a trend but a necessity for businesses striving to remain competitive. This section provides a detailed exploration of various facets, from fundamental concepts to cutting-edge techniques, essential for professionals navigating the data-driven world of today. Understanding the backbone of data analytics sets the stage for unlocking its full potential in shaping business strategies and decision-making processes.
Defining Data Analytics
The Role of Data in Business
Delving into 'The Role of Data in Business,' one encounters the essence of information as the backbone of operational intelligence. Data, in its various forms, powers every aspect of modern enterprises, driving informed decision-making and strategy formulation. The unique characteristic of data lies in its ability to unveil patterns, trends, and insights that shape business outcomes significantly. Its distinct advantage in this guide is its ability to transform raw data into actionable insights that propel business growth and efficiency.
Types of Data Analytics
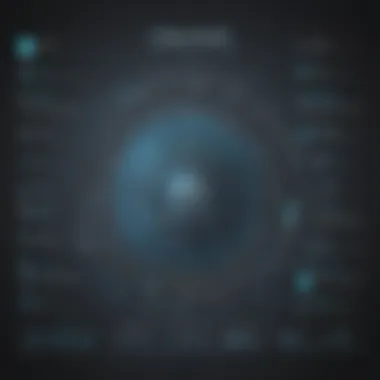
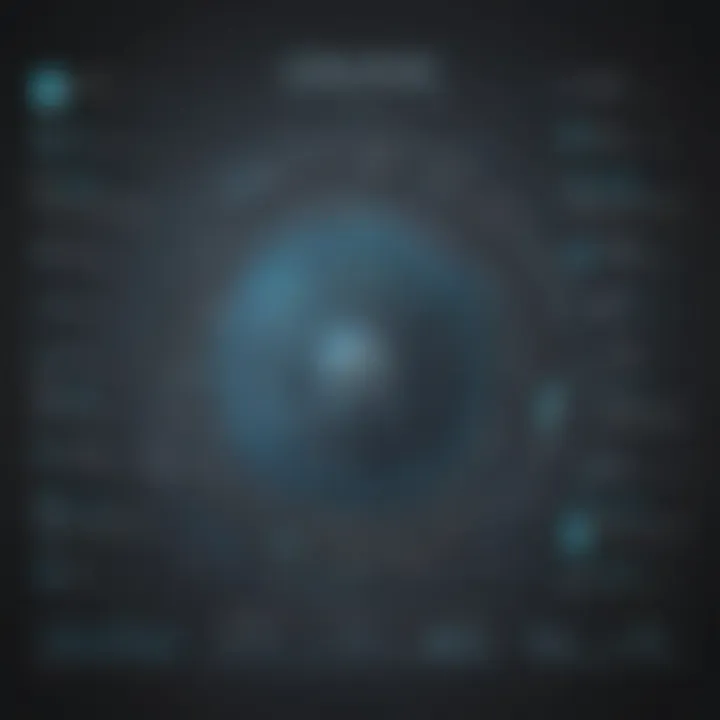
As we probe the realm of 'Types of Data Analytics,' we unearth the diversity embedded in analytical methodologies. Each type - be it descriptive, diagnostic, predictive, or prescriptive analytics - offers distinct advantages catered to specific business needs. Delving into these types enriches the reader's understanding of analysis tools and techniques, providing a holistic view of how data can be harnessed effectively. The significance of these types in this article is their capability to offer tailored solutions for varying business challenges, albeit with certain limitations that must be navigated adeptly.
Importance of Data Analytics
Enhancing Decision-Making
Unveiling the realm of 'Enhancing Decision-Making,' we grasp the transformative impact of data analytics on the decision-making process. Empowering decision-makers with real-time insights and predictive capabilities, data analytics revolutionizes the traditional approach to strategic planning. The crux lies in its ability to mitigate risks, identify opportunities, and optimize outcomes, making it a quintessential tool for navigating today's dynamic business landscape.
Driving Business Growth
Exploring 'Driving Business Growth' uncovers the strategic leverage data analytics offers in fostering organizational expansion. By harnessing the power of data to spot growth opportunities, optimize processes, and enhance customer experiences, businesses can scale effectively. The prominence of this aspect in the article stems from its role in propelling sustainable growth, yet it necessitates a nuanced approach to deployment to maximize its advantages while mitigating potential drawbacks.
Fundamentals of Data Analysis
In the vast landscape of data analytics, understanding the fundamentals is akin to laying a sturdy foundation for a successful data-driven journey. The section on Fundamentals of Data Analysis serves as a cornerstone in this comprehensive guide, shedding light on the crucial elements that shape how businesses harness the power of data. This segment delves deep into the intricacies of data collection and preparation, emphasizing the pivotal role it plays in driving informed decision-making and shaping strategic initiatives. By grasping the fundamentals, professionals can navigate the complexities of data analysis with precision and agility, enabling them to extract meaningful insights efficiently. Notably, the exploration of data sources and cleaning techniques underscores the significance of ensuring data integrity and relevance in analytical processes. By mastering these fundamental aspects, businesses can elevate their analytical capabilities and gain a competitive edge in the dynamic market environment.
Data Collection and Preparation
Data Sources:
Data sources form the bedrock of any data analytics endeavor, serving as the reservoir from which valuable insights are extracted. In this section, the spotlight is on elucidating the diverse sources of data that fuel analytical processes, ranging from structured databases to unstructured sources such as social media and IoT devices. The significance of selecting appropriate data sources aligns with the overarching goal of this guide - to equip professionals with the knowledge to make strategic data-driven decisions. Emphasizing the reliability and relevance of data sources underscores their pivotal role in ensuring the accuracy and effectiveness of analytical outcomes. Understanding the unique features and characteristics of various data sources empowers businesses to curate datasets that align with their specific analytical objectives, thereby enhancing the efficacy of data-driven initiatives.
Data Cleaning Techniques:
Data cleaning techniques represent a critical step in the data analysis process, aimed at enhancing the quality and usability of data for analytical purposes. Within this segment, the focus is on exploring the methodologies and tools employed to rectify inconsistencies, errors, and redundancies within datasets. By weaving a narrative around the significance of data cleaning, this guide illuminates the transformative impact of ensuring data accuracy and completeness. Discussing the advantages and disadvantages of different data cleaning techniques offers professionals a holistic view of how to optimize data quality while mitigating potential challenges. By delving into the nuances of data cleaning, businesses can streamline their analytical workflows and derive more meaningful insights from their datasets.
Exploratory Data Analysis
Statistical Methods:
Statistical methods serve as the analytical compass that guides professionals through the vast expanse of data, enabling them to uncover patterns, correlations, and trends. Within this section, the emphasis lies on demystifying the various statistical techniques utilized in exploratory data analysis, ranging from descriptive statistics to inferential methods. By elucidating the role of statistical methods in deriving actionable insights, this guide equips professionals with the tools to discern meaningful patterns within complex datasets. Highlighting the advantages of statistical methods in enhancing data comprehension and decision-making underscores their instrumental value in the analytics landscape. By unraveling the intricacies of statistical analysis, businesses can transform raw data into actionable intelligence, driving operational efficiencies and strategic growth.
Visualization Tools:
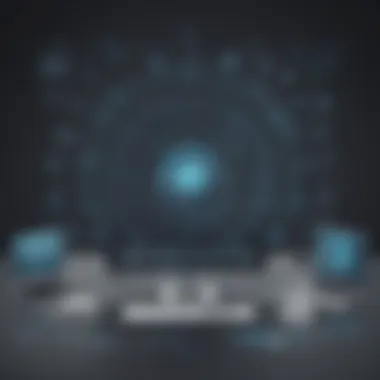
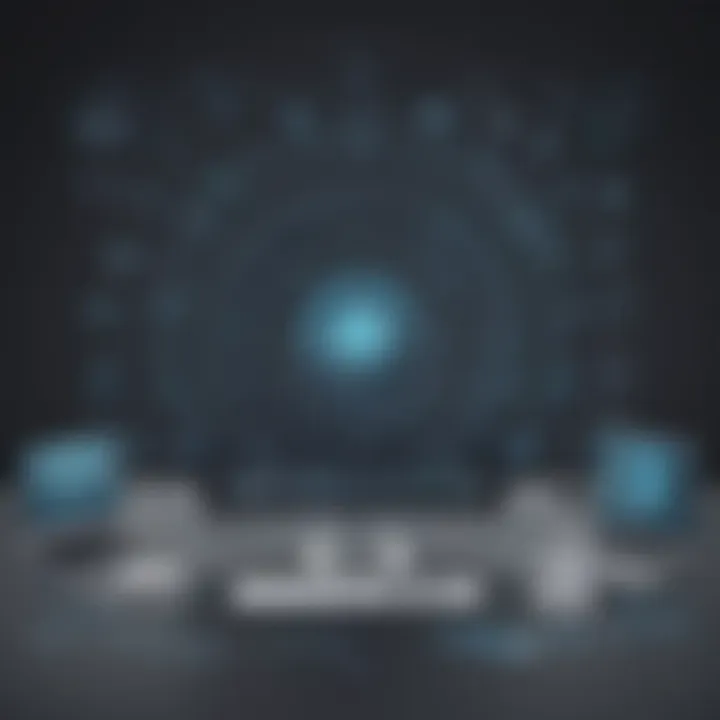
Visualization tools offer a visual narrative that complements statistical analyses, enabling stakeholders to grasp complex data dynamics effortlessly. In this segment, the focus is on exploring the diverse visualization techniques and platforms that facilitate data exploration and interpretation. By elucidating the role of visualization tools in transforming data into compelling visuals, this guide underscores their significance in enhancing data communication and comprehension. Discussing the advantages and disadvantages of different visualization tools empowers professionals to select the most appropriate tools for conveying insights effectively. By immersing in the realm of data visualization, businesses can communicate their findings persuasively, fostering data-driven decision-making and strategic innovation.
Advanced Data Analytics Techniques
In this section of the comprehensive guide on unlocking the power of data analytics in business, we delve into the critical aspects of advanced data analytics techniques. These techniques play a pivotal role in empowering organizations to extract valuable insights from data, driving informed decision-making and fostering business growth. By harnessing advanced data analytics, businesses can gain a competitive edge in today's dynamic market landscape. Emphasizing the significance of advanced data analytics techniques in this guide showcases their relevance in transforming raw data into actionable intelligence.
Predictive Analytics
- Machine Learning Algorithms: Machine learning algorithms are at the forefront of predictive analytics, enabling systems to learn from data patterns and make predictions without explicit programming. The versatility of machine learning algorithms allows businesses to uncover hidden trends and correlations within vast datasets, facilitating accurate forecasting and strategic planning. Their adaptability and scalability make them a go-to choice for organizations looking to leverage predictive analytics effectively in decision-making processes. Despite their complexity, machine learning algorithms offer unparalleled precision and efficiency in deriving meaningful insights from data.
- Predictive Modeling: Predictive modeling is a cornerstone of predictive analytics, encompassing statistical techniques to forecast future outcomes based on historical data. By constructing predictive models, businesses can anticipate trends, mitigate risks, and optimize resources with data-driven strategies. The flexibility and interpretability of predictive modeling make it a valuable asset for organizations seeking predictive analytics solutions. While predictive modeling empowers businesses with foresight and accuracy, it also necessitates careful model selection and validation to ensure robust and reliable predictions.
Prescriptive Analytics
- Optimization Techniques: Optimization techniques form the core of prescriptive analytics, guiding decision-makers on the best courses of action to achieve desired outcomes. By employing optimization algorithms, businesses can streamline processes, improve operational efficiency, and maximize performance metrics. The iterative nature of optimization techniques allows organizations to refine strategies continuously and adapt to changing business environments effectively. Despite their computational intensity, optimization techniques deliver actionable insights for enhancing business operations and strategic planning.
- Decision Support Systems: Decision support systems serve as decision-making tools that leverage data analytics to provide recommendations and insights to users. By integrating advanced algorithms and visualization capabilities, decision support systems enable stakeholders to assess complex scenarios, simulate outcomes, and evaluate alternative courses of action. The interactive interfaces and real-time functionality of decision support systems enhance decision-making processes, fostering agility and competitiveness in the business landscape. While decision support systems bolster decision-making processes, their efficacy hinges on data accuracy and user proficiency in interpreting analytics outputs.
Implementing Data Analytics in Business
Implementing Data Analytics in Business is a crucial aspect discussed in this article, emphasizing its pivotal role in driving business success through data utilization. By integrating data analytics into operations, businesses gain insights that inform strategic decision-making, optimize processes, and enhance overall performance. This section explores the specific elements of Implementing Data Analytics in Business, highlighting its benefits and considerations within the context of leveraging data effectively in competitive markets.
Data-Driven Decision Making
Data-Driven Decision Making is an essential component of implementing data analytics in business, underscoring the shift towards data-centric strategies for informed choices. In this article, the Cultural Shift towards Data is a key focus, illustrating how organizations are transitioning to data-driven cultures to stay abreast of market dynamics and consumer preferences. This shift involves embracing data as a primary driver of decisions and fostering a mindset that values data-backed insights. The Cultural Shift towards Data is fundamentally beneficial in facilitating faster and more accurate decision-making, enabling businesses to adapt proactively to changing environments. However, one challenge of this shift is overcoming resistance to change and ensuring that data interpretations align with organizational objectives.
Building Analytical Capabilities
Building Analytical Capabilities plays a vital role in empowering organizations to harness the full potential of data analytics. Highlighted in this article is the significance of developing the skills, tools, and infrastructure necessary to facilitate robust analytics processes. Organizations enhancing their analytical capabilities can delve deeper into data insights, derive meaningful patterns, and translate them into actionable strategies. The unique feature of Building Analytical Capabilities lies in its ability to foster a data-driven culture by upskilling personnel, adopting advanced analytics tools, and establishing data governance frameworks. While building analytical capabilities is advantageous for companies seeking competitive advantages through data analytics, it requires significant investments in training, technology, and governance structures.
Challenges and Best Practices
Exploring the Challenges and Best Practices in data analytics implementation sheds light on the critical considerations that organizations must address to ensure successful outcomes. Data Privacy Concerns surface as a prominent challenge, highlighting the importance of safeguarding sensitive information and complying with data protection regulations. This article delves into the key characteristic of Data Privacy Concerns, stressing the need for robust data security measures, ethical data handling practices, and transparency in data processing. While prioritizing data privacy is essential for maintaining trust with customers and mitigating legal risks, organizations face the challenge of balancing data protection with data utility.
Ensuring Data Quality
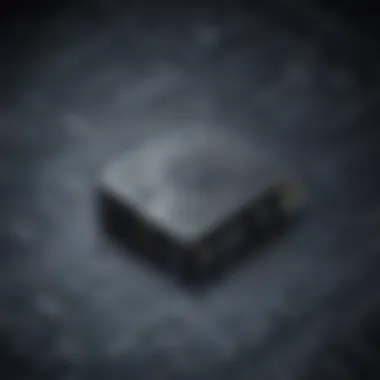
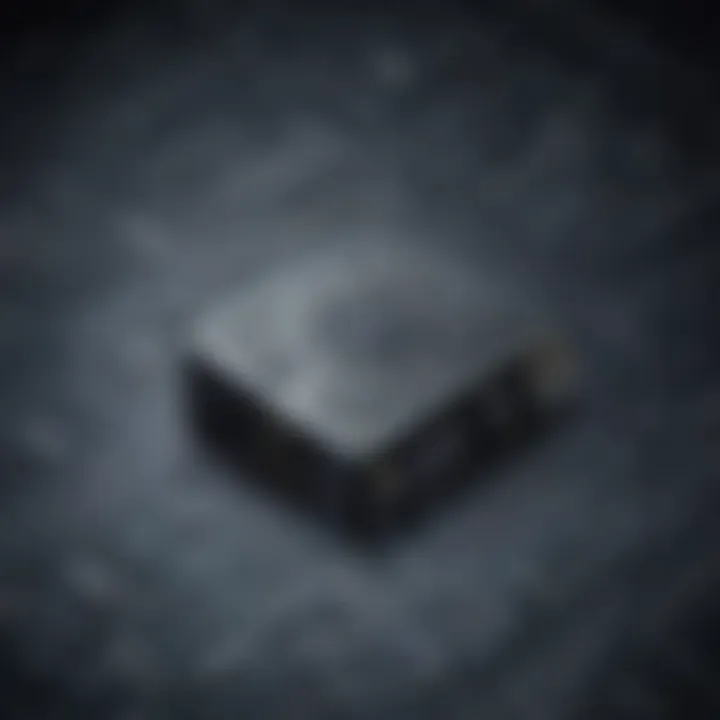
Ensuring Data Quality emerges as a best practice in data analytics implementation, emphasizing the need for accurate, reliable data inputs to drive insightful analyses. This section discusses the key characteristic of Ensuring Data Quality, which involves implementing data validation processes, eliminating inconsistencies, and maintaining data integrity throughout the analytics lifecycle. The unique feature of Ensuring Data Quality lies in its ability to enhance decision-making precision, improve predictive modeling accuracy, and fuel data-driven innovation. While prioritizing data quality is advantageous for cultivating data-driven cultures and enhancing business performance, organizations must allocate resources to data cleansing, validation, and quality assurance processes to ensure data integrity and validity.
Case Studies and Examples
In the realm of data analytics, Case Studies and Examples play a pivotal role in solidifying theoretical concepts into practical applications. These real-world instances provide professionals with tangible scenarios to understand how data analytics can be leveraged effectively. By delving into Case Studies and Examples, readers gain insight into the challenges, strategies, and outcomes of applying data analytics in diverse business settings. Through detailed analysis of successful implementations and case-specific methodologies, professionals can draw inspiration and valuable lessons to enhance their own data analytics practices.
Optimizing Marketing Strategies
Segmentation Analysis
Segmentation Analysis, a crucial component of optimizing marketing strategies, entails dividing a target market into distinct groups based on specific criteria such as demographics, behavior, or preferences. This segmentation approach allows businesses to tailor their marketing campaigns, product offerings, and communication strategies to cater to the unique needs and characteristics of each segment. The primary advantage of Segmentation Analysis lies in its ability to personalize marketing efforts, thus increasing relevance and effectiveness. However, challenges such as data accuracy and segment overlap require careful consideration to ensure the success of segmentation initiatives in this context.
Customer Lifetime Value Modeling
Customer Lifetime Value Modeling is a strategic tool used to assess the long-term value a customer brings to a business throughout their relationship. By predicting the potential revenue generated by a customer over their entire engagement with the company, organizations can optimize their marketing strategies, retention efforts, and customer satisfaction initiatives. The key benefit of Customer Lifetime Value Modeling is its ability to prioritize high-value customers, allocate resources efficiently, and enhance overall customer lifetime profitability. Despite its advantages, this modeling approach necessitates accurate data inputs, proper interpretation, and continuous refinement to maximize its value in the context of this article.
Improving Operational Efficiency
Supply Chain Optimization
Supply Chain Optimization involves enhancing the efficiency and effectiveness of the supply chain processes to minimize costs, streamline operations, and meet customer demands promptly. By optimizing the flow of goods, reducing lead times, and improving inventory management, businesses can realize significant improvements in operational performance and customer service levels. The core advantage of Supply Chain Optimization lies in its capacity to create a responsive and agile supply chain that adapts to changing market dynamics and consumer preferences. However, factors such as global disruptions, technology integration, and sustainability considerations present challenges that must be addressed in implementing supply chain optimization strategies within this article.
Predictive Maintenance
Predictive Maintenance employs data analytics to predict when maintenance should be performed on equipment to prevent sudden breakdowns, enhance equipment reliability, and extend asset lifecycles. By analyzing historical data, monitoring equipment performance in real-time, and applying machine learning algorithms, organizations can schedule maintenance activities proactively, reduce downtime, and optimize maintenance costs. The primary advantage of Predictive Maintenance is its ability to transition maintenance practices from reactive to proactive, thereby improving operational efficiency and resource utilization. Nevertheless, issues related to data quality, algorithm accuracy, and implementation complexity require careful management when integrating predictive maintenance solutions into business operations discussed in this article.
Conclusion
In this culminating section of the detailed guide on unlocking the power of data analytics in business, we come to grasp the pivotal role of drawing conclusions based on data-driven insights. A key element that stands out is the critical importance of leveraging analytical findings to guide strategic decision-making processes within a business setting. By synthesizing and interpreting vast amounts of data, organizations can extract valuable nuggets of information that can steer them towards competitive advantages and sustainable growth. The ability to draw sound and actionable conclusions from complex datasets is a hallmark of successful enterprises, providing them with a pathway to optimizing operations, enhancing customer experiences, and fostering innovation. It is crucial for businesses to not only collect and analyze data but also to derive meaningful conclusions that can be translated into tangible business outcomes.
Harnessing the Power of Data
Key Takeaways
Exploring the realm of key takeaways in the context of data analytics unveils a treasure trove of actionable insights and strategic directions for decision-makers. These key takeaways serve as the distilled essence of the data analytics process, encapsulating the most crucial findings and implications derived from in-depth analysis. They play a pivotal role in guiding business strategies, enhancing operational efficiency, and empowering organizations to make informed decisions based on data-driven evidence. The beauty of key takeaways lies in their ability to simplify complex data patterns and trends into digestible pieces of information that can drive impactful decision-making processes. By focusing on key takeaways, businesses can prioritize their resources effectively, mitigate risks, and capitalize on emerging opportunities with confidence and precision.
Future Trends in Data Analytics
As we look towards the horizon of data analytics, the landscape is continuously evolving, ushering in a wave of future trends that promise to revolutionize the field. One such trend is the proliferation of artificial intelligence and machine learning algorithms, enabling businesses to unlock predictive and prescriptive analytics capabilities with unprecedented accuracy and speed. The advent of big data technologies and cloud computing solutions is reshaping the way data is collected, stored, and analyzed, offering scalability and flexibility to organizations of all sizes. Moreover, the emphasis on data privacy and security is driving the development of robust governance frameworks and compliance measures to safeguard sensitive information. Embracing these future trends in data analytics equips businesses with the tools and insights needed to stay ahead of the curve, adapt to changing market dynamics, and capitalize on data as a strategic asset in the digital age.