Optimizing Data Analytics Team Structure for Success
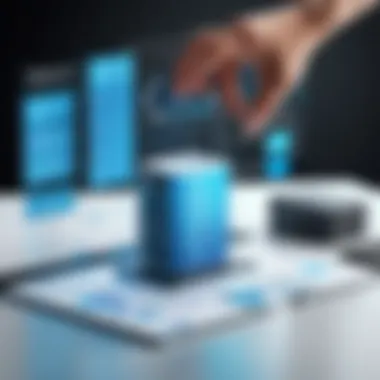
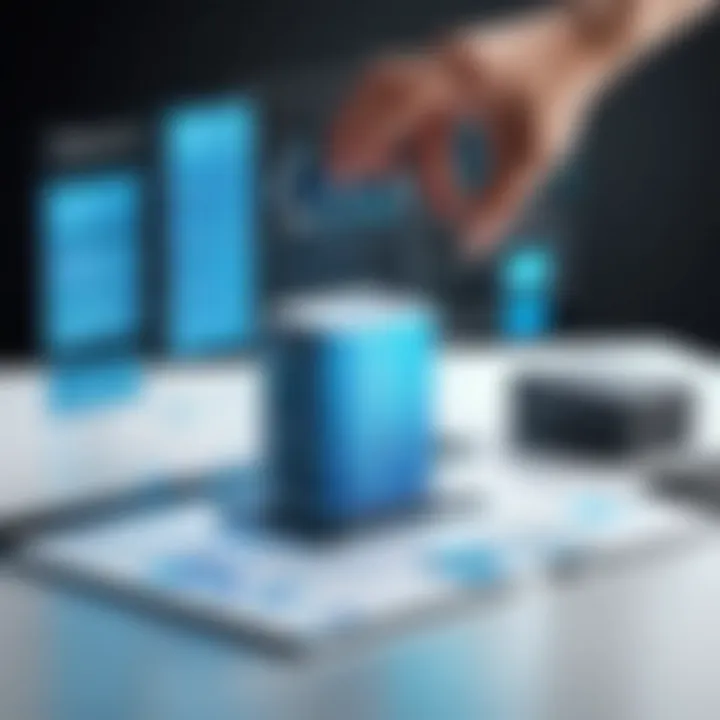
Intro
Data analytics teams are fundamental to modern businesses. They convert raw data into actionable insights, which influence strategic decisions. To achieve this, a coherent team structure is vital, supporting collaboration and efficiency among diverse skill sets. Understanding how to optimize these structures empowers organizations to harness analytics effectively and augment their competitive advantage.
In this article, we will explore the composition, roles, and necessary collaboration strategies within data analytics teams. We will also investigate the technological advancements and skill diversification that enhance these functions.
Technological Research Overview
Recent Technological Innovations
The data landscape is constantly evolving. Emerging technologies like machine learning, big data platforms, and cloud computing have changed how businesses analyze data. Tools such as Tableau, Power BI, and Apache Hadoop have made data visualization and processing more efficient. The integration of these technologies allows teams to work with larger datasets and facilitates more sophisticated analysis.
Impact on Business Operations
Data analytics is no longer a luxury; it is crucial for operational excellence. Real-time analytics helps businesses make prompt decisions. For instance, retail giants like Amazon utilize analytics not just for sales forecasting but also for optimizing supply chain logistics. This approach has led to smoother operations and elevated customer satisfaction.
Future Technological Trends
Looking ahead, companies must prepare for advances in AI and automation. As algorithms become more complex and capable, they will drive predictive analytics even further. Furthermore, organizations will increasingly integrate blockchain to ensure data integrity. Embracing these trends can offer significant advantages.
Data Analytics in Business
Importance of Data Analytics
Data analytics is crucial in today's data-driven landscape. It allows businesses to turn trends into insights, enhancing strategic decisions. Companies can pinpoint target markets and improve customer engagement, thereby increasing retention rates.
Tools for Data Analysis
Numerous tools support data analytics endeavors:
- Excel: A staple for any analyst, offering basic analysis capabilities.
- Python: A versatile programming language that enables complex data manipulation and visualization.
- R: A language crucial for statistical analysis, favored among data scientists for its power in predictive modeling.
- Tableau: Exceptional in data visualization, simplifying the communication of insights.
Each tool serves a purpose, and selecting the right mix can directly influence the effectiveness of analytics teams.
Case Studies on Data-Driven Decisions
Organizations like Netflix and Spotify have mastered data-driven decision-making. They analyze user behavior to recommend content, making their services highly personalized. This practice has resulted in heightened user satisfaction and loyalty, showcasing the power of leveraging data effectively.
Cybersecurity Insights
Threat Landscape Analysis
With increased focus on data analytics comes the necessity for robust cybersecurity measures. Data breaches can undermine trust and incur significant financial costs. Teams must be aware of new threats, such as ransomware and phishing attacks, as these can directly affect data integrity.
Best Practices for Cybersecurity
Establishing a culture of cybersecurity is essential. Here are some best practices:
- Regular training on security protocols for all team members.
- Implementing strong authentication measures.
- Regularly updating software and systems to patch vulnerabilities.
Regulatory Compliance in Cybersecurity
Vigilance in regulatory compliance is also required for businesses. Laws like GDPR impose strict requirements on data handling. Non-compliance can lead to hefty fines and damage reputation. A dedicated approach is necessary to ensure all data practices meet legal standards.
Artificial Intelligence Applications
AI in Business Automation
Adoption of AI in business processes offers significant efficiencies. Automating repetitive tasks allows teams to focus on analysis and insights rather than menial data entry. AI-driven analytics tools can highlight trends and anomalies, supporting faster decision-making.
AI Algorithms and Applications
The rise of deep learning and neural networks has enriched the analytical capabilities available to businesses. These technologies enable more accurate predictions and classifications. Companies can leverage them to streamline operations or refine marketing strategies.
Ethical Considerations in AI
However, ethical implications of AI use must be acknowledged. Concerns around data privacy and bias in algorithms are critical. Teams must ensure that their approaches uphold ethical integrity while leveraging AI for insights.
Industry-Specific Research
Tech Research in Finance Sector
In finance, data analytics enhances risk management. For example, investment firms utilize analytics to forecast market trends, which is essential for strategic positioning.
Healthcare Technological Advancements
The healthcare sector thrives on data analytics for patient outcomes. Predictive analytics identifies at-risk populations, informing preventive care measures.
Retail Industry Tech Solutions
Retailers apply analytics to enhance product placements and improve inventory management. Efficient data utilization in this sector can mean the difference between success and failure.
Innovating team structures and roles allows organizations to tap into the full potential of their data analytics capabilities, driving growth and performance.
Understanding Data Analytics Team Structure
Understanding the structure of data analytics teams is vital for organizations aiming to harness the power of data for decision-making and performance optimization. In a landscape where data pervades various aspects of business, having a well-defined team structure not only enhances efficiency but also aligns analytics efforts with organizational goals. This section delves into the significance of recognizing how teams are organized and the roles that each member plays.
Definition and Importance
Data analytics team structure refers to the arrangement of roles, responsibilities, and hierarchies within an organization that focuses on data analysis. It acts as the framework that dictates how data-related tasks are executed, from data collection to analysis and reporting.
The importance of a thoughtful team structure lies in several factors:
- Enhanced Collaboration: With clear roles and lines of communication, team members can collaborate effectively. This minimizes misunderstandings and promotes a shared vision.
- Efficiency in Execution: When everyone knows their responsibilities, project completion becomes more streamlined. There's less chance of duplication of efforts, ensuring that resources are used wisely.
- Adaptability to Change: A well-structured team can quickly adapt to market changes or technological advancements. This agility is essential in todayโs rapidly evolving business environment.
Core Objectives of a Data Analytics Team
Establishing a data analytics team comes with distinct objectives that guide its operations:
- Data-Driven Decision-Making: The primary goal is to provide insights that inform business strategies. Analytics teams support executives by transforming raw data into actionable insights.
- Performance Monitoring: Teams assess the effectiveness of strategies through key performance indicators (KPIs). These metrics enable continuous performance evaluation and improvement.
- Fostering a Data-Centric Culture: A data analytics team promotes a culture where data-informed decisions take precedence over intuition-based decisions. This shift enhances accountability and transparency across the organization.
- Cross-Disciplinary Collaboration: Effective data analytics teams collaborate with various departments such as marketing, finance, and operations. This ensures that insights are relevant and aligned with departmental goals.
- Staying Ahead of Trends: By continuously analyzing data trends, analytics teams play a crucial role in identifying opportunities for innovation and competitive advantage.
Understanding these core objectives helps organizations shape their analytics focus, ultimately driving better performance and outcomes.
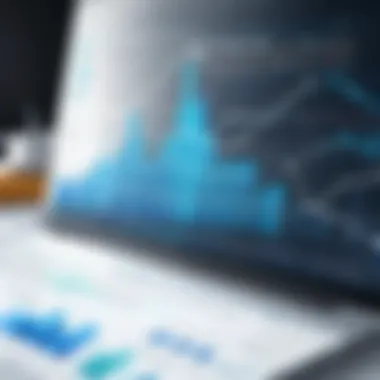
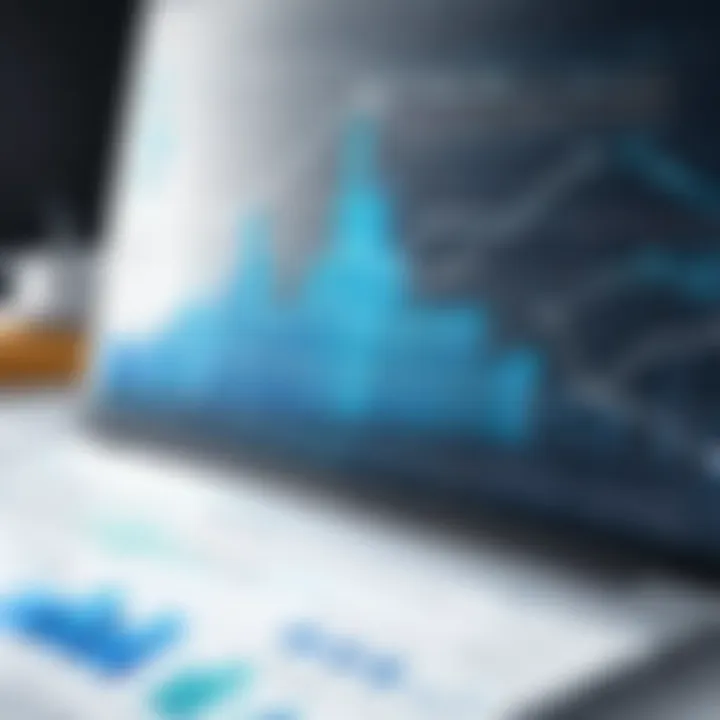
Key Roles Within a Data Analytics Team
Understanding the key roles within a data analytics team is vital for driving effective decision-making and optimizing performance. Each role contributes uniquely to the overall functionality and success of the team. The integration of these roles allows for a thorough analysis of data, ensuring that strategic decisions are backed by accurate insights.
Data Analysts
Data analysts are central to any data analytics team. They gather, process, and analyze data to uncover trends and insights. This role demands proficiency in statistical tools and analytical methods. Their work allows organizations to make informed decisions based on evidence and facts.
Data analysts typically focus on:
- Data Collection: Collecting relevant data from various sources.
- Data Cleaning: Ensuring the data is accurate and free from errors.
- Analysis: Interpreting data to identify trends, patterns, and anomalies.
- Reporting: Presenting findings in a clear and concise manner, often through dashboards or reports.
The importance of data analysts cannot be overstated. They transform raw data into actionable insights, driving business strategies.
Data Scientists
Data scientists take data analysis a step further. Their expertise lies in applying advanced statistical methods, machine learning, and predictive analysis. They interpret complex data and extract insights that can drive innovation and foster competitive advantage.
Key responsibilities of data scientists include:
- Model Development: Creating algorithms to analyze data predictions.
- Experimentation: Testing hypotheses through data-driven experiments.
- Visualization: Using sophisticated tools to present data findings effectively.
- Collaboration: Working closely with data engineers and analysts to ensure data integrity and relevance.
Data scientists are crucial for organizations aiming to harness the full potential of data, thus shaping strategic planning.
Data Engineers
Data engineers build and maintain the systems and architecture that allow data to be collected and analyzed effectively. Their role covers both the operational and infrastructural aspects of data management.
They are responsible for:
- Data Pipeline Architecture: Designing data pipelines for efficient data flow.
- Database Management: Overseeing the storage and retrieval of data.
- Quality Assurance: Ensuring data consistency and reliability.
Without data engineers, the analytical processes would struggle, as they provide the necessary environment for data analysts and scientists to perform their work.
Analytics Managers
Analytics managers oversee the entire analytics function within a team. They coordinate efforts between data analysts, scientists, and engineers, ensuring that projects align with business objectives.
Key duties of analytics managers include:
- Project Management: Leading analytics initiatives and managing timelines.
- Team Development: Mentoring team members and facilitating skill development.
- Strategic Alignment: Ensuring that analytics strategies align with organizational goals.
Analytics managers bridge the gap between technical teams and business stakeholders, enhancing the overall impact of analytics work.
Business Intelligence Analysts
Business intelligence analysts specialize in analyzing data to inform business strategy and operations. They focus on market trends, customer behavior, and performance metrics to provide insights that drive efficiency and profitability.
Their role includes:
- Data Interpretation: Analyzing data to derive actionable insights about business operations.
- Reporting: Creating reports that summarize findings for business stakeholders.
- Trend Analysis: Identifying patterns that affect business performance.
Business intelligence analysts play a critical role in guiding decision-making processes, ensuring that strategies are both data-driven and agile.
Organizational Structures for Analytics Teams
The organization of data analytics teams plays a crucial role in determining their efficiency and effectiveness. A well-defined structure allows for clarity in roles, enhances communication, and aligns analytics efforts with overall business objectives. The way analytics teams are organized can significantly influence their performance and outcomes. Understanding the different structures can help organizations choose the one that best fits their needs, leading to more informed decision-making and improved business performance.
In approaching the topic of organizational structures, it is essential to consider several key elements:
- Alignment with business strategy: The structure should support the organization's strategic goals.
- Flexibility: Teams must adapt to changes in technology and business environments.
- Scalability: The chosen structure should accommodate growth as the organization expands its analytical capabilities.
Each of these considerations can lead to distinct benefits, including better resource allocation, enhanced collaboration among team members, and improved quality of analytics outputs. The following sections will explore the three main types of organizational structures for analytics teams: centralized, decentralized, and hybrid.
Centralized Structure
In a centralized structure, analytics functions are grouped under a single team or department. This model typically provides uniformity in processes and methodologies across the organization. By centralizing analytics, companies can leverage shared resources and expertise more effectively.
Advantages of this structure include:
- Consistency in analytics: All teams work under the same guidelines, making it easier to maintain quality.
- Shared knowledge: Centralized teams can maintain a pool of knowledge, aiding in the dissemination of best practices.
- Cost efficiency: By pooling resources, organizations may reduce duplication of efforts.
However, this model may lead to challenges as well. There could be a disconnect between centralized teams and individual business units. This can result in analytics solutions that do not fully address specific departmental needs, reducing the overall impact of analytics on the business.
Decentralized Structure
A decentralized structure gives individual business units autonomy over their analytics functions. In this model, each team operates somewhat independently, focusing on their specific area of the business. This enables tailored analytics solutions that align closely with the immediate needs of departments, fostering innovation and agility.
Notable benefits of this approach include:
- Tailored solutions: Business units can develop analytics strategies that are specific to their operations.
- Faster decision-making: Independent teams can respond quickly to local needs and changes in the market.
- Enhanced local expertise: Teams develop specialized knowledge that caters directly to their unit's dynamics.
However, decentralization can lead to certain inefficiencies, such as:
- Siloed knowledge: Information may not flow freely between teams, leading to duplicated efforts and missed opportunities for collaboration.
- Inconsistent quality: Varying levels of expertise among teams can affect the quality of analyses and outputs.
Hybrid Model
The hybrid model combines elements of both centralized and decentralized structures, aiming to optimize their respective advantages. In this approach, core analytics functions are centralized while still allowing individual departments the flexibility to develop their analytics teams.
This model offers:
- Balance: Core competencies can be maintained at a central level, while specific needs are still addressed regionally.
- Collaborative innovation: Teams share insights and resources, enabling both innovation and best practices.
- Resource efficiency: Shared resources help with specialized analytical tasks while allowing for tailored strategies at the local level.
Despite its strengths, a hybrid model may face challenges in coordination. Ensuring effective communication between central and local teams is vital to leverage the full potential of this structure. Without proper management, this model can potentially lead to confusion about roles and responsibilities.
Key takeaway: The choice of organizational structure for analytics teams is not merely a matter of preference; it is strategic and should align with the organization's goals and resources.
Skills Required for Data Analytics Teams
In the realm of data analytics, possessing the right skills is crucial for team effectiveness and success. Technical proficiency underpins the ability to interpret and manipulate data while soft skills facilitate collaboration and effective communication within teams. Mastery of specific skills enables data analytics professionals to derive meaningful insights and, ultimately, drive informed decision-making in organizations.
Technical Skills
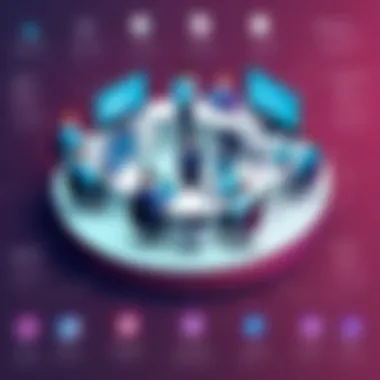
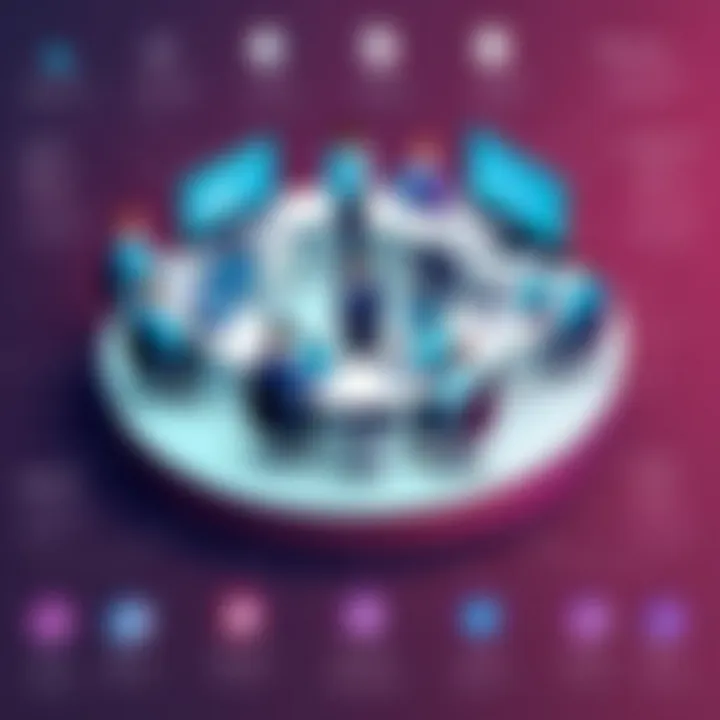
Statistical Analysis
Statistical analysis forms the backbone of data interpretation. It enables analysts to draw conclusions from data sets through descriptive and inferential techniques. A key characteristic of statistical analysis is its reliance on quantitative data, allowing teams to validate hypotheses and measure variability.
This method is widely recognized as a beneficial choice for analytics teams due to its capability to provide reliable evidence for strategic decisions. A unique feature of statistical analysis is its versatility; it can be adapted to any dataset to unearth patterns and correlations. The advantage lies in its scientific approach to problem-solving, although it can be limited by the need for accurate data and careful implementation of methods, which may require substantial statistical knowledge.
Programming Languages
Programming languages, such as Python and R, are essential tools for data manipulation and analysis. The key characteristic of these languages is their ability to handle large volumes of data efficiently, allowing for sophisticated analytics processes.
Python stands out for its libraries tailored to data science, making it a popular choice among data professionals. Moreover, R excels in statistical analysis and visualization, positioning it as a strong contender for analytics tasks. The unique feature of programming languages is their flexibility and integration capabilities with other tools. However, their disadvantage can sometimes be a steep learning curve for team members who are not technologically proficient.
Data Visualization Tools
Data visualization tools like Tableau and Power BI play a significant role in simplifying complex data findings. They enhance data interpretation through visual representation, which makes insights more accessible and understandable. A notable characteristic is their user-friendly interfaces, allowing both technical and non-technical users to create compelling visual reports.
These tools are beneficial as they transform data into visuals that highlight trends and anomalies. A unique feature is the ability to customize dashboards and reports according to specific business needs. Nevertheless, the effectiveness of these tools can diminish if the underlying data is flawed or poorly structured.
Soft Skills
Critical Thinking
Critical thinking is indispensable within data analytics teams. It allows professionals to analyze problems from multiple angles, ensuring a comprehensive understanding of data and its implications. The key characteristic of critical thinking is its emphasis on logic and reasoning, which drives teams toward objective conclusions.
This skill is vital for navigating the complexities of data interpretation and ensuring robust analyses. An important aspect of critical thinking is the ability to question assumptions and recognize biases, which can lead to better decision-making. However, a disadvantage may arise if team members lack confidence or experience, potentially stifling innovative approaches.
Communication Skills
Effective communication skills are necessary for sharing insights derived from data analyses. Analysts must articulate their findings clearly to various stakeholders, regardless of their technical expertise. The prominent characteristic of these skills is the ability to explain complex ideas simply and concisely.
Good communication fosters collaboration between team members and ensures that insights are recognized and valued by decision-makers. Unique to communication skills is their ability to bridge the gap between data and business strategies. A disadvantage, however, can occur when there are misunderstandings, which might impede action on critical insights.
Team Collaboration
Collaboration is vital for teams engaged in data analytics, emphasizing collective intelligence. Team collaboration skills enhance brainstorming and idea sharing, leading to innovative solutions. A key characteristic is the respect and leverage of diverse viewpoints within the team.
These skills encourage a supportive environment and promote efficient workflow. A unique aspect of team collaboration is the synergy achieved when members share their expertise. Nevertheless, a challenge can be the potential for conflict or differences in opinion, which may disrupt team harmony and productivity.
Collaboration Tools and Technologies
Collaboration tools and technologies play a critical role in the structure and efficiency of data analytics teams. As these teams are often multidisciplinary, effective collaboration is essential to achieving key objectives. Proper tools facilitate communication, enhance productivity, and ensure that data is accessible to team members when needed. The integration of technology into collaboration supports a cohesive unit, even when members work remotely or from various locations.
Project Management Software
Project management software serves as the backbone of an analytics teamโs organization and workflow. Tools like Trello, Asana, or Jira help teams to set clear objectives, assign tasks, and track progress. By clearly defining roles and timelines, teams can avoid overlaps and ensure accountability among members.
Moreover, project management software provides visibility into the status of various tasks. This transparency is invaluable for decision-makers, allowing them to manage resources effectively and make informed choices based on real-time data. The ability to prioritize work and manage deadlines enhances the overall efficiency of the analytics process. Without these tools, many teams face challenges in maintaining organization and achieving their objectives effectively.
Data Sharing Platforms
Data sharing platforms are critical in promoting collaboration among members of data analytics teams. Platforms like Google Drive, SharePoint, and Dropbox enable users to store and share files securely. This is especially important as data analytics work often requires shared access to datasets, visualizations, and reports.
These platforms not only support access to information but also enable version control, which is essential for maintaining the integrity of data. Team members can track changes, review updates, and avoid potential conflicts that arise from multiple versions of the same file. Additionally, by ensuring that relevant data is centralized, data sharing platforms help to minimize the time spent searching for necessary information, allowing teams to focus on analysis and insights.
Communication Tools
Effective communication is vital in any analytics team. Tools like Slack, Microsoft Teams, or Zoom facilitate real-time communication and collaboration. These platforms allow team members to discuss projects, share insight, and resolve issues immediately. The ability to quickly exchange ideas is crucial in an environment where timely decisions often dictate success.
By using these tools, teams can maintain a steady flow of communication across different channels and formats. Team discussions can be documented, allowing for future reference and accountability. Moreover, many of these tools have integrations with project management and data sharing platforms, creating a seamless workflow that enhances overall productivity.
"Utilizing the right tools can truly transform the performance of analytics teams, promoting a culture of collaboration and efficiency."
In summary, collaboration tools and technologies are not merely supportive elements; they are foundational to the operations of data analytics teams. The strategic use of project management software, data sharing platforms, and communication tools can lead to enhanced outcomes, ensuring that analytics teams not only function smoothly but also achieve their organizational goals.
The Impact of Technology on Team Structure
In today's fast-paced business environment, technology plays a crucial role in shaping the structure of data analytics teams. As organizations increasingly rely on data-driven insights for decision-making, the integration of technology within these teams becomes paramount. Understanding the impact of technology on team structure helps organizations to enhance their operational efficiency, facilitate better collaboration, and improve the overall performance of their analytics functions.
The key elements of this impact can be examined through the lens of emerging technologies and automated analytics tools. Both of these factors provide significant benefits that can optimize analytics teams, streamline processes, and drive better outcomes for organizations.
Emerging Technologies
Emerging technologies are revolutionizing how data analytics teams operate. Innovations such as artificial intelligence (AI), machine learning, and big data processing have altered traditional analytics practices. By leveraging these technologies, teams can analyze vast amounts of data at unprecedented speeds, leading to faster and more accurate insights.
The use of AI algorithms can uncover hidden patterns in data that may not be obvious to human analysts. This capability allows teams to focus on higher-level strategy and interpretation rather than merely data computation. Additionally, machine learning can enhance predictive analytics, helping organizations to anticipate market trends and customer behaviors.
Consider the following points when implementing emerging technologies into your data analytics team:
- Skill Requirements: Teams need members trained in new technologies to utilize their full potential effectively.
- Technology Integration: It is vital to integrate these technologies into existing workflows to avoid disruption.
- Cost Considerations: Investing in new technology can be significant. Organizations must weigh the costs against the potential benefits.
Automated Analytics Tools
Automated analytics tools are essential in optimizing team structure. These tools simplify complex data analysis tasks, reducing the time it takes to derive meaningful insights. By automating repetitive tasks, data analysts can devote their time to more strategic activities that drive business value.
Automation also minimizes human error, providing more reliable data analysis results. Tools like Tableau and Power BI enhance data visualization, making insights more accessible to stakeholders.
When considering automated analytics tools, organizations should account for:
- User-Friendliness: Tools should be intuitive, enabling all team members to utilize them regardless of tech expertise.
- Customization: The ability to tailor tools to specific business needs can ensure better alignment with organizational goals.
- Collaboration Features: Automated tools that facilitate collaboration among team members can enhance communication and cohesion.
"Integrating technology effectively into a data analytics team not only streamlines processes but also enhances the decision-making capabilities of the organization as a whole."
Analytics Team Leadership
Effective leadership within data analytics teams is a fundamental aspect of driving performance and achieving positive outcomes. Analytics team leadership encompasses a variety of skills and approaches necessary to guide team members and establish a productive environment. The ability to make decisions based on data, foster collaboration, and cultivate a culture of learning is crucial. As organizations rely more on data for strategic decisions, strong leadership in analytics teams plays an essential role in bridging the gap between data insights and actionable business strategies.
A key element of effective analytics leadership is promoting clarity in objectives. Leaders must clearly define goals and how each team member contributes to these goals. This alignment ensures that everyone is working toward a common purpose. Additionally, analytics leaders need to maintain transparency in decision-making processes. When teams understand the rationale behind decisions, they are more likely to feel engaged and invested in the outcomes.
Moreover, good leadership encourages continuous learning and skill development. The analytics landscape is dynamic, with new tools and methodologies emerging regularly. Leaders should prioritize training initiatives, enabling team members to stay current with industry trends and technological advancements. This approach not only enhances the team's capabilities but also fosters a spirit of innovation.
"The strength of data analytics teams lies in the synergy between leadership and the diverse skills of the team members."
In summary, the importance of analytics team leadership cannot be overstated. A strong leader inspires, aligns, and motivates the team, ultimately driving greater efficiency and effective outcomes in data analytics initiatives.
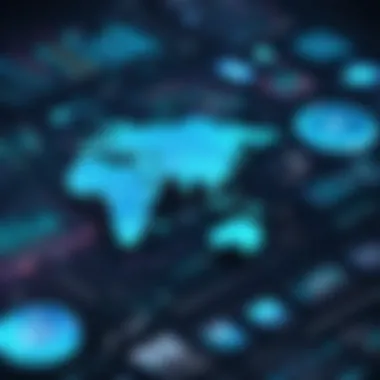
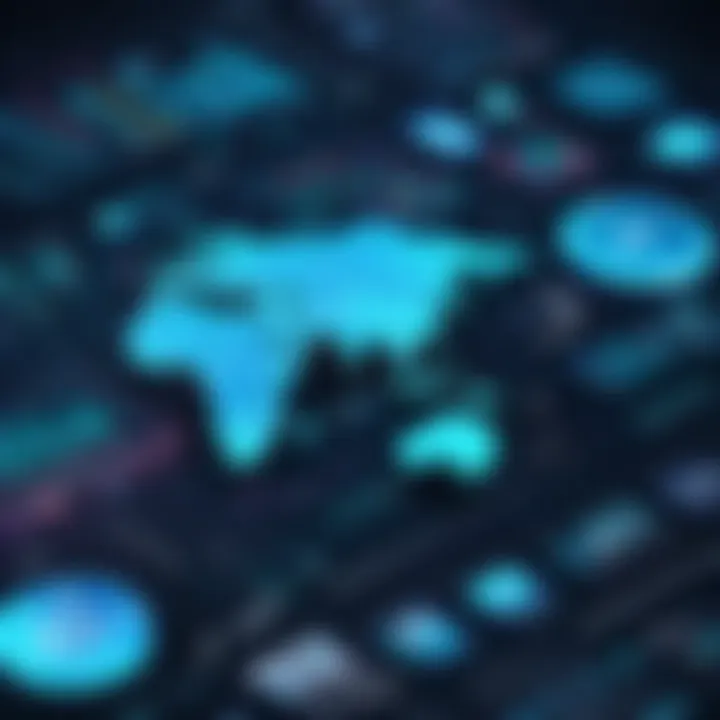
Leadership Skills for Analytics Managers
Analytical managers should possess a varied skill set to effectively lead their teams. Firstly, strong communication skills are vital. Leaders must articulate complex data findings in a manner that is understandable to non-technical stakeholders. This ensures that insights can be acted upon at all levels of the organization.
Secondly, strategic thinking capabilities are essential. Analytics managers need to analyze trends, foresee potential challenges, and make informed decisions that align with broader business objectives. They should engage in problem-solving discussions that encompass perspectives from diverse team members.
In addition, emotional intelligence plays a significant role in leadership. Managers need to be attuned to the needs and feelings of their team members. By recognizing and addressing team dynamics and individual motivations, they can create a more cohesive and high-performing unit.
Finally, tech-savviness is a must. Managers should be well-versed in the analytical tools and technologies in use. This understanding allows them to guide their teams effectively and identify opportunities for improvement in workflows or tools.
Developing a Data-Driven Culture
Cultivating a data-driven culture is paramount for any organization seeking to maximize its analytical capabilities. A data-driven culture promotes using data in decision-making processes at all levels, fostering an environment where insights are valued and pursued. It encourages team members to rely on factual evidence rather than assumptions or intuition.
To establish a data-driven culture, organizations must start with leadership buy-in. Senior management should openly advocate for data utilization and lead by example in making data-informed decisions. This sets the tone for the organization and demonstrates the importance of analytics in achieving business goals.
Moreover, providing access to data tools and training is essential. Employees should be equipped with the necessary resources to analyze data and derive insights independently. This empowerment not only boosts morale but also enhances the overall analytical proficiency of the organization.
Additionally, celebrating data-driven decisions and successful implementations can reinforce this culture. When organizations acknowledge achievements that stem from insightful analytics, it motivates employees to engage more in data-driven practices.
Case Studies on Successful Data Analytics Teams
The examination of case studies focused on successful data analytics teams is critical in forging a deeper understanding of effective strategies and practices. These case studies provide invaluable insights into how various organizations have harnessed the power of data analytics to make informed decisions and drive tangible outcomes. They offer a practical perspective that complements theoretical knowledge, showcasing real-world applications of concepts discussed earlier in this article.
Companies that implement effective analytics frameworks often share common characteristics. These include clear objectives, cohesive team dynamics, and appropriate technologies. Each case serves as an example of how a structured data analytics team can contribute to business growth and efficiency. Analyzing these examples can help organizations identify potential challenges and solutions that may arise during their statistical endeavors.
Corporate Examples
One notable corporate example is Netflix, which employs a sophisticated data analytics team to enhance viewer experience and content strategy. By analyzing watching habits and preferences, they tailor recommendations that keep users engaged. This approach not only improves customer satisfaction but also drives retention rates. The outcome is impressive: a vast library of content curated based on robust data insights leads to high global subscriber counts.
Another example is Amazon, where data analytics empowers decision-making across diverse functions, from inventory management to personalized marketing. The real-time data processing capabilities of their analytics team enable quick responses to market trends. This has resulted in improved operational efficiency and boosted sales figures. These two examples illustrate the powerful role of data analytics in shaping corporate strategies.
Cross-Industry Comparisons
When comparing data analytics teams across various sectors, significant differences and similarities emerge. In the healthcare industry, Mayo Clinic utilizes data analytics to improve patient care. Through predictive analytics, they can anticipate patient needs, leading to better health outcomes. Conversely, the financial sector often emphasizes risk management analytics, where companies like Goldman Sachs leverage data to make informed investment decisions.
In tech, companies like Google focus on user behavior data, allowing for optimized services and targeted advertising. Each industry demonstrates unique challenges yet benefits from data analytics. While methodologies may vary, the core objective remains consistent: driving growth through informed decision-making.
"Data is the new oil; itโs valuable, but if unrefined, it cannot really be used."
โ Clive Humby
In summary, studying successful data analytics teams across industries reveals how proper structuring and strategic implementation can yield significant advantages. Such insights not only inform best practices but also highlight the versatility of data analytics in solving complex business problems.
Evaluating the Performance of Analytics Teams
Evaluating the performance of analytics teams is a critical aspect of ensuring organizational effectiveness in data-driven decision making. Performance evaluation not only assesses how well teams are achieving their objectives but also identifies areas for improvement and growth. Understanding the dynamics of analytics teams allows leaders to optimize their resources and align analytical output with corporate strategies.
A robust evaluation process integrates feedback mechanisms, performance metrics, and analytical insights, driving teams toward continuous enhancement. Furthermore, this fosters a culture of accountability and supports the development of skills necessary to adapt to evolving challenges in the field.
In this context, two main elements become pivotal: Key Performance Indicators (KPIs) and a systematic approach to feedback and continuous improvement. Both components work together to create an environment where teams can thrive and contribute meaningfully to organizational goals.
Key Performance Indicators
Key Performance Indicators serve as quantifiable measures that gauge the effectiveness of analytics teams. These metrics provide insights into how well teams are performing in relation to defined goals. The following KPIs are particularly relevant for evaluating analytics teams:
- Accuracy of Data Analysis: This measures the precision of the insights derived from data.
- Timeliness of Deliverables: Analyses must be completed within deadlines to ensure that decision-making is not delayed.
- Impact of Insights on Decisions: Assessing how insights have influenced strategic decisions can highlight the value provided by analytics teams.
- Collaboration Effectiveness: Evaluating how well team members work together can provide clues about team dynamics.
Using a combination of these metrics helps organizations understand both individual and collective performance. Monitoring these KPIs regularly allows for proactive adjustments rather than reactive measures.
Feedback and Continuous Improvement
A feedback mechanism forms the backbone of performance evaluation. Regular feedback can be facilitated through structured reviews, one-on-one meetings, and informal check-ins. This continuous dialogue encourages team members to share their views on processes and outcomes.
The emphasis on continuous improvement is equally crucial. Teams should cultivate habits that foster learning and adaptation. For example, conducting post-project reviews can uncover lessons learned, which can be applied to future projects. This practice benefits teams by enhancing their analytical capabilities over time and promoting a mindset of experimentation and growth.
Ultimately, as organizations navigate the complexities of data analytics, establishing a robust framework for evaluating team performance becomes indispensable. Continuous evaluation rooted in clear KPIs and persistent feedback mechanisms is key to realizing the full potential of analytics teams and ensuring they contribute effectively to organizational success.
"Performance evaluations that prioritize learning and feedback cultivate a culture of excellence within analytics teams."
For further insights into performance metrics, you can explore resources like Wikipedia or Britannica.
Future Trends in Data Analytics Team Structures
The landscape of data analytics is continuously evolving. Understanding the future trends in data analytics team structures is vital for organizations aiming to stay ahead. This section will discuss significant elements like the influence of AI and machine learning, as well as the rising demand for diverse skills within analytics teams. These factors are crucial for improving overall performance and achieving better outcomes.
Influence of AI and Machine Learning
Artificial Intelligence and Machine Learning are quickly reshaping the dynamics of data analytics teams. As these technologies become more sophisticated, they enable analytics teams to process larger data sets faster and with greater accuracy. This leads to deeper insights that inform strategic decisions.
Integration of AI tools can streamline routine tasks, allowing data analysts to focus on more complex analysis and interpretation. For instance, machine learning algorithms can automate data cleaning and processing, reducing the time spent on these tasks. This capability fosters agility within teams, enabling faster turnaround on projects and improving responsiveness to business needs.
Organizations will need to adapt their team structures to incorporate these technologies effectively. Training becomes essential, as team members must understand how to leverage AI tools. A blend of domain knowledge and technical skill in AI is increasingly important for team success. Therefore, analytics teams that embrace these capabilities stand to enhance their effectiveness significantly.
Increased Demand for Diverse Skills
The data analytics field is no longer confined to traditional roles. There is a growing demand for diverse skill sets within analytics teams. Companies seek individuals who are not only strong in statistics or programming but also possess skills in business acumen, data storytelling, and ethical consideration in data usage.
The following points illustrate the shift:
- Interdisciplinary Collaboration: Analytics teams frequently collaborate with marketing, finance, and operations to derive insights applicable to varying business functions. This requires a mix of expertise that transcends data alone.
- Soft Skills Importance: Communication and collaboration skills are vital. Analysts must explain complex data insights to non-technical stakeholders. Being able to convey value through storytelling aids in decision-making processes.
- Adaptability: The pace of technological advancement necessitates that analytics professionals stay versatile. Continuous learning and adapting to new tools, languages, and methods are essential for maintaining effectiveness.
The surge in demand for these broadened skill sets means that organizations must rethink their hiring and development strategies. Creating an environment that encourages upskilling and cross-training can boost team performance and improve outcomes.
"Analytics teams that adapt to evolving technologies and diversify their skill sets will lead the future of data-driven decision making."
Finale
The conclusion of this article serves as a critical reflection on how data analytics teams can enhance performance and outcomes within organizations. As we explored various facets of data analytics team structures, it became clear that the organization of these teams profoundly impacts their effectiveness in achieving business objectives. A well-defined structure ensures clarity in roles, allowing team members to leverage their strengths and skills appropriately.
Recap of Key Insights
Several key insights emerge from this exploration:
- The Importance of Clear Roles: Each role within the data analytics team brings unique expertise. Data scientists, analysts, and engineers must work cohesively, understanding their functions and how they contribute to overall project success.
- Collaborative Tools Are Essential: Using the right collaboration tools can bridge the gaps between different roles and enhance communication. Technologies such as project management software and data-sharing platforms play a significant role in this.
- Emphasis on Continuous Improvement: Performance evaluation is not merely a checklist but a continuous process. Feedback mechanisms and adaptive strategies enhance team function and project outcomes.
- Future Trends Indicate Evolution: Trends such as AI integration and the need for diverse skills highlight the shifting landscape of data analytics. Teams must adjust to these changes proactively to remain competitive.
Strategic Recommendations
To optimize the performance and outcomes of data analytics teams, consider the following recommendations:
- Foster a Data-Driven Culture: Encourage a culture within the organization that values data insights in decision-making, thus empowering analytics teams.
- Invest in Training and Development: Continuous skill development ensures team members are equipped to tackle emerging technologies and methodologies, promoting agility.
- Implement Agile Methodologies: Adopting agile practices allows teams to respond quickly to changes, focusing on iterative progress and flexibility.
- Encourage Cross-Functional Collaboration: Facilitating communication between analytics teams and other departments will promote a deeper understanding of business needs and improve data utilization.
- Utilize Performance Metrics Wisely: Establish clear key performance indicators (KPIs) that reflect both efficiency and impact, helping teams focus on outcomes rather than only activities.