Metadata Management Automation in Data Governance
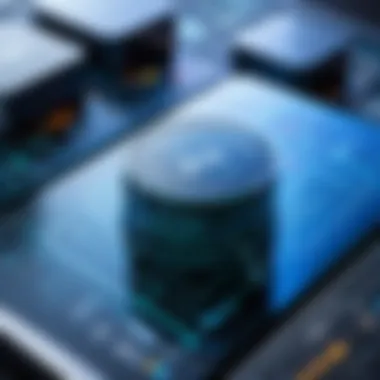
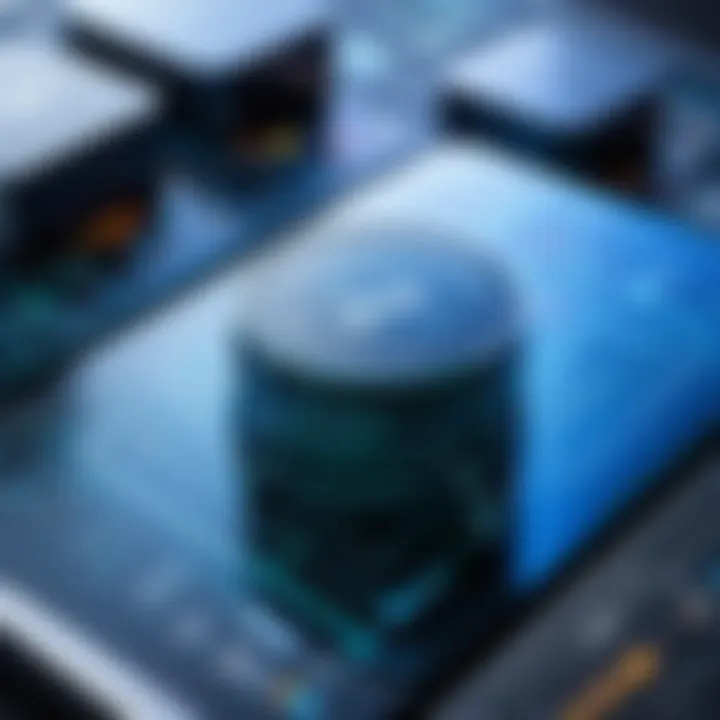
Intro
In today’s fast-paced digital age, businesses face an overwhelming influx of data, which necessitates effective management strategies. Metadata management automation emerges as a vital tool in navigating this complex landscape, acting as the backbone of robust data governance frameworks. This article takes a closer look at how automating metadata processes can not only enhance operational efficiency but also uphold compliance standards essential for contemporary organizations. As we journey through this topic, we will highlight critical insights, practical approaches, and emerging trends that are reshaping the future of data management.
Technological Research Overview
Recent Technological Innovations
The rise of cloud computing and big data analytics has reshaped how companies handle their metadata. An array of innovative tools and technologies is now available, suggesting a shift from traditional, manual systems to automated solutions that promise accuracy and efficiency. Software like Alation and Informatica Enterprise Data Catalog are leading the charge, allowing organizations to curate their data effectively while maintaining a strong grasp on compliance and governance aspects.
Impact on Business Operations
The impact of these technological advancements goes beyond just optimizing data management. Automation of metadata processes means that businesses can make quicker, smarter decisions, based on enriched insights. For example, a retail company implementing automated metadata tagging might streamline their inventory management, leading to quicker turnaround times and better customer satisfaction.
"Automation isn't just about replacing the workforce, it's about empowering it by reducing mundane tasks and increasing creativity and strategic thinking."
Future Technological Trends
With trends like artificial intelligence and machine learning shaping the metadata landscape, we will likely see a continued focus on predictive analytics and advanced data visualization tools. These technologies are set to simplify complex data relationships and provide deeper insights into the validity of the data being managed.
Data Analytics in Business
Importance of Data Analytics
Data analytics forms the cornerstone of effective metadata management, offering the means to convert raw data into actionable insights. By employing advanced analytics, organizations can glean patterns, identify anomalies, and improve future decision-making processes.
Tools for Data Analysis
Various tools can facilitate data analysis, such as Power BI or Tableau. These platforms allow users to visualize trends in data easily and generate reports that inform strategic actions across departments.
Case Studies on Data-Driven Decisions
To illustrate the impact of data-driven decisions, consider a financial institution leveraging advanced analytics. By integrating automated metadata management with their data analytics platform, they achieved a significant reduction in compliance breaches, thus saving substantial costs in penalties.
Cybersecurity Insights
Threat Landscape Analysis
As more organizations turn to automation, the cybersecurity landscape has grown more intricate. Today’s challenges include sophisticated cyber-attacks aimed at exploiting metadata vulnerabilities. Companies must remain vigilant and aware of potential risks to guard against data breaches effectively.
Best Practices for Cybersecurity
Implementing best practices, such as regular audits of metadata processes and robust access controls, can help mitigate potential threats. Companies should also conduct employee training programs to educate staff about the importance of data protection and potential cyber risks.
Regulatory Compliance in Cybersecurity
Compliance with regulations such as GDPR and CCPA requires that organizations demonstrate accountability in their metadata practices. Ensuring that data processing is transparent and that personal information is protected is not just best practice—it's a legal necessity.
Artificial Intelligence Applications
AI in Business Automation
AI’s role in metadata management should not be underestimated. Its capabilities can revolutionize how businesses automate their processes, leading to enhanced reliability and accuracy. Machine learning algorithms can sift through data sets to not only extract relevant metadata but also predict trends.
AI Algorithms and Applications
Consider an e-commerce company using AI-infused tools to enhance customer personalization strategies. By automating metadata tagging and organization, the company can easily segment users based on behavior, preferences, and purchase history.
Ethical Considerations in AI
However, the ambition to adopt AI comes with ethical responsibilities. Organizations must navigate the thin line between leveraging personal data for targeted strategies and respecting privacy rights. Developing transparent AI practices is essential in maintaining trust with customers.
Industry-Specific Research
Tech Research in Finance Sector
The finance sector stands at the forefront of implementing metadata management automation. With stringent regulations and the need for meticulous data tracking, automation can greatly enhance operational agility and ensure compliance.
Healthcare Technological Advancements
In healthcare, automated metadata systems can assist in managing sensitive patient information, ensuring confidentiality while improving patient care through timely access to data.
Retail Industry Tech Solutions
For retailers, metadata management automation assists in streamlining inventory and customer interactions. By using advanced systems to track product information and customer behavior, retailers can enhance their marketing strategies and optimize stock management.
In summary, the adoption of metadata management automation stands to benefit various industries significantly. By focusing on best practices, emerging technologies, and the implications of data-driven decisions, businesses can set themselves on a path toward unrivaled efficiency in data governance.
Understanding Metadata Management
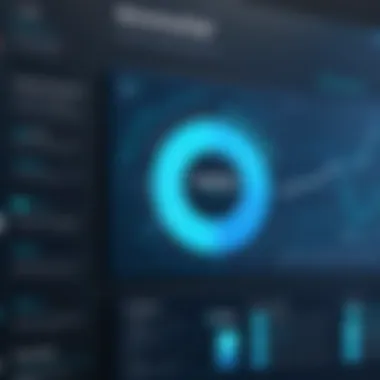
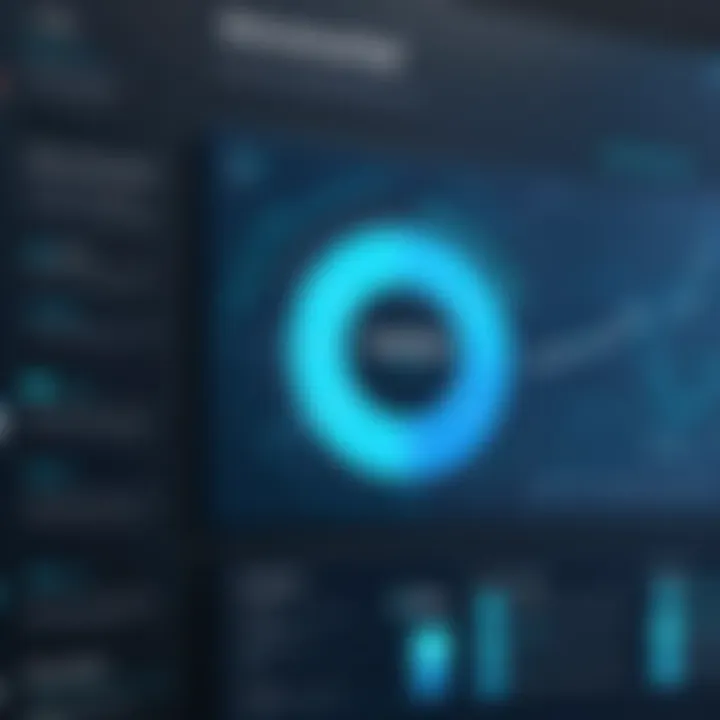
Metadata management is like the unsung hero in the world of data governance, yet it's crucial for every data strategy. Understanding metadata management helps organizations categorize, find, and utilize their data more effectively. By managing metadata, businesses can have clearer visibility into their data assets, minimizing the risk of duplication or misuse. For professionals looking to streamline processes, metadata management involves specific benefits like improved data discovery and enhance compliance with regulations.
Defining Metadata
Metadata can be simplified as "data about data." It encapsulates the information that describes various attributes of data, making it easier to classify, understand, and manage it. Think of it as a book's index or table of contents – it guides you to exactly what you need. For instance, consider a dataset containing customer details. The metadata would provide context about what each field represents, such as name, age, or purchase history, along with guidelines for how the data can be used.
Types of Metadata
Understanding the types of metadata is critical in enhancing data management workflows. Here’s a closer look:
Descriptive Metadata
Descriptive metadata is essentially the searchable details of a dataset. It lets users query the database effectively. A striking feature is its ability to facilitate data discovery through clear and concise descriptions. For instance, in a university's database, descriptive metadata might include course titles, instructor names, and semester offerings. This makes fetching information more efficient and user-friendly.
However, it comes with a catch—accuracy in this metadata type is crucial; incorrect descriptors can lead to confusion and mismanagement of data. Thus, one needs to ensure that the descriptive data consistently aligns with its corresponding content.
Structural Metadata
Structural metadata defines how different pieces of data relate to one another and the organization of the dataset. Think of it as the blueprint for a building. It outlines how data elements fit together, enhancing understanding of complex datasets. For instance, a digital library might have structural metadata specifying the relationship between books, authors, and genres. This organization helps in efficient navigation and retrieval of data.
While structural metadata is beneficial for enabling data interoperability, it requires adequate governance structures to prevent chaos, especially in large datasets where relationships can quickly become convoluted.
Administrative Metadata
Administrative metadata provides information on how to manage data, including its provenance, rights, and lifecycle. Consider it as someone's user manual for a product. In libraries, administrative metadata may track who has access to what data, retention schedules, and audit trails. This type of metadata ensures compliance with legal regulations, which is essential in today’s data governance landscape.
Yet, there’s a downside; if not regularly updated, it may become obsolete, potentially leading to data mismanagement. Therefore, organizations need to establish consistent processes for maintaining administrative metadata without becoming cumbersome.
Importance of Metadata in Data Management
In the realm of data management, the relevance of metadata cannot be overstated. Metadata not only facilitates easier access and retrieval but also aids organizations in making informed decisions based on reliable data. It helps in complying with regulatory standards by offering detailed transparency of the data lineage and usage. Good metadata practices enhance the overall quality of information, ultimately boosting operational efficiency.
"Without metadata, data loses context, leading to diminished value and missed opportunities."
In summary, metadata management acts as the backbone of effective data governance, enabling organizations to streamline operations and make better strategic decisions.
Metadata Management Overview
In the rapidly evolving landscape of data governance, proper metadata management stands at the forefront. It serves as a cornerstone for organizations aiming to organize, access, and utilize their data efficiently. With the surge in data volumes and diversity, understanding how metadata management operates is essential. Organizations are now embracing automation to streamline these processes, which not only heightens efficiency but also fortifies compliance and enhances decision-making capabilities.
The Role of Metadata in Organizations
Metadata acts as data about data, offering context that clarifies the meaning and usage of information. It plays a vital role in organizations by enabling better data discovery and utilisation, thus supporting decision-makers in their strategic endeavors. When metadata is well managed, stakeholders find it easier to understand data lineage, access appropriate datasets, and ensure proper data governance practices. Essentially, metadata transforms raw data into a usable asset.
Key Components of Metadata Management
The landscape of metadata management is multifaceted, comprising several key components that together enhance the efficiency and effectiveness of data governance initiatives. Three of the most critical components are the Metadata Repository, Data Catalogs, and Metadata Standards. Each of these elements bears significant weight in how organizations approach metadata management.
Metadata Repository
The Metadata Repository centralizes critical information about organizational data assets. Think of it as a well-organized library where every book (data asset) is cataloged with detailed annotations (metadata). This centralization simplifies searching and accessing data, reducing the time spent poring over numerous sources. The key characteristic of a metadata repository is its ability to integrate information from various sources and formats into one cohesive system. This is particularly beneficial in environments overflowing with disparate data—which is nearly every organization today.
A unique feature of a metadata repository lies in its integration capabilities. It enables organizations to track data lineage, which illustrates how data flows through different systems, methodologies, and transformations. This visibility not only aids in compliance but also supports data integrity. However, while this structured approach to data storage is advantageous, it requires ongoing maintenance to keep everything current and relevant.
Data Catalogs
When it comes to Data Catalogs, think of them as a user-friendly interface that allows users to easily navigate through the vast sea of data available to them. They serve as a dynamic inventory of data assets accompanied by rich context about their purpose and how to access them. The key characteristic of a data catalog is its focus on enhancing data discoverability and usability.
By implementing data catalogs, organizations can foster a data-driven culture. Users can better understand available datasets, which leads to more informed decision-making. The unique feature of data catalogs is often their capacity to allow user-generated content, enabling colleagues to enrich catalog entries through feedback or descriptions. However, organizations need to be wary of discrepancies in contributions that could lead to confusion or misinformation.
Metadata Standards
Metadata Standards encompass the guidelines and conventions employed to create and manage metadata consistently across an organization. It’s the blueprint for how metadata should be structured, which not only facilitates clarity but also promotes interoperability across systems. These standards are essential as they establish a common language that ensures that metadata can be shared and understood across various platforms and teams.
A prominent advantage of having metadata standards is the reduction of ambiguity in data interpretation and use. Establishing these standards can mitigate risks related to compliance and data governance. One unique feature of metadata standards is their role in fostering collaboration, as they enable different teams to communicate effectively and make use of the same data sets without confusion. Yet, it’s important for organizations to guard against rigidity; overly strict standards may stifle innovation or adaptability when new data needs arise.
Proper metadata management requires a blend of structure and flexibility—too much of either can hinder your organization.
Through a strategic focus on these components, organizations are poised to harness the full potential of their data, turning information into a strategic asset. In this age where decision-making can mean the difference between staying ahead or falling behind, understanding the role and structure of metadata management couldn’t be more crucial.
The Necessity of Automation in Metadata Management
In today’s fast-paced data landscape, the need for automation in metadata management has become increasingly significant. Organizations are wrestling with large amounts of data, often spread across various platforms. This complexity often leads to numerous challenges that impede effective governance. Automation can streamline processes which in turn enhances efficiency, reduces errors, and fortifies data integrity.
Challenges of Manual Metadata Management
Inconsistencies
One of the most glaring issues in manual metadata management is the inconsistencies that frequently arise. When data is handled by human hands, the possibility of error expands rapidly. For instance, if different teams are inputting metadata for the same dataset, variations can easily occur. This leads to a mishmash of duplicate information: one team may name a field "EmployeeID" while another might take liberties and call it "Emp_ID". These discrepancies make it far more difficult to pull accurate data insights.
The key characteristic of inconsistencies is their pervasive nature; they can exist at various levels of metadata. The detrimental effects of such inconsistencies on overall data governance are profound, as they can severely compromise data quality and hinder trust in the system. Automation offers a consistent methodology for metadata entry which not only reduces the chances of human error but also solidifies data integrity across all departments. This is a beneficial twist for organizations needing precise datasets to support decision-making.
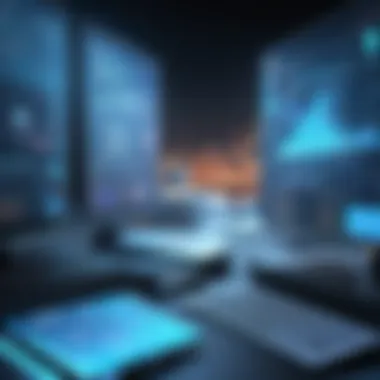
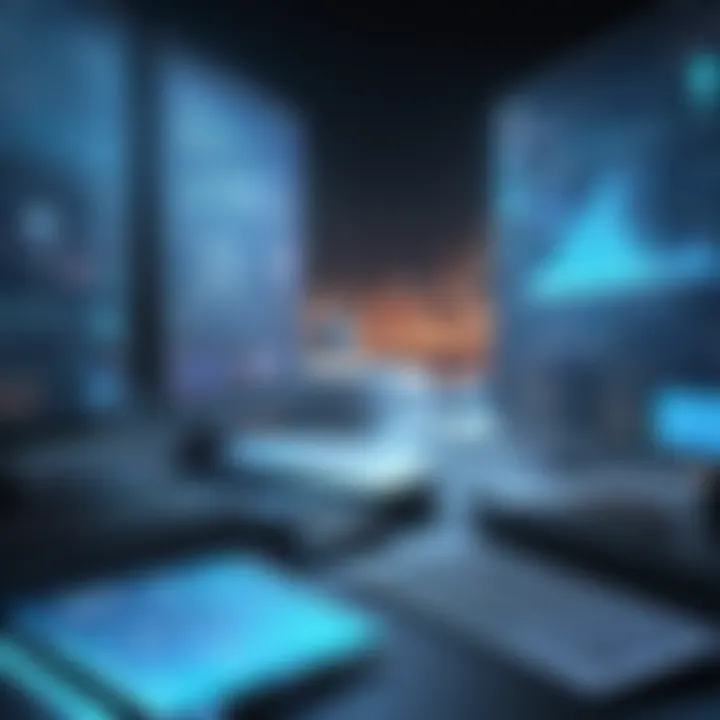
Data Governance Risks
Another thorny issue tied to manual metadata management is the data governance risks it engenders. Poorly defined metadata procedures can lead to data misuse or breaches. Consider a healthcare organization that fails to document patient data correctly. Here, the repercussions could extend not only to compliance violations but also to breaches of trust with patients. Such risks illuminate the necessity for a standardized approach to metadata management.
Data governance risks highlight a crucial characteristic of automation: it establishes a protocol that eliminates ambiguity. Automated systems can enforce rules and ensure consistency, thus enhancing compliance with governmental and industry regulations. This mitigates the likelihood of penalties and fosters a culture of accountability. The unique feature of automating data management is its ability to provide real-time updates and alerts, minimizing risks before they spiral out of control.
Scalability Issues
Manual systems also struggle significantly with scalability issues. As businesses grow, their data multiplies exponentially. A manual approach quickly becomes untenable, leading to bottlenecks in processes and response times. For example, what might work well for a small startup with minimal data can quickly become a quagmire for a corporation managing terabytes of information. When faced with sudden peaks in data volume, manual processes falter.
The key to this scalability problem is hidden in the limitations of human capacity to manage ever-increasing datasets. Here lies the beauty of automation: it can easily adjust and scale to accommodate growth without the growing pains typically faced during manual transitions. The inherent capacity for managing vast amounts of data efficiently is a significant advantage that companies cannot afford to overlook.
The Need for Automation
Given the challenges highlighted above, it becomes patently clear that automation is not merely an option but a necessity. The insights gleaned from previous sections illustrate how automation enables organizations to overcome inconsistencies, minimize risks, and manage scalability effectively. Automating metadata processes can result in actionable data insights that drive better business decisions.
In a world where the decisions can either make or break a company, automated metadata management stands as a pillar of support. Organizations must harness these technological advancements to establish a solid data governance framework that not only meets current needs but also prepares for future challenges and complexities.
Benefits of Metadata Management Automation
Metadata management automation stands at the crossroads of efficiency and reliability within data governance frameworks. In an era where data proliferates at an explosive rate, the ability to automate metadata management is not just a luxury; it has become a necessity. This section elucidates significant benefits that come with embracing automation in metadata management practices, illustrating how it positions organizations favorably within their competitive landscapes.
Enhanced Efficiency
One of the standout benefits of automating metadata management lies in the efficiency it brings to the table. Manual processes, often cluttered with time-consuming tasks, hinder the agility needed in today’s fast-paced business environments. With automation, organizations can seamlessly streamline workflows, enabling teams to focus on strategic initiatives rather than getting bogged down by repetitive tasks.
For instance, think about a financial services company struggling to catalog thousands of datasets scattered across departments. By employing automated tools, they can systematically extract and organize metadata, dramatically reducing the time spent on data discovery. This not only frees up personnel resources but also permits quicker adaptations to business needs.
Improved Data Quality
Data quality is non-negotiable; poor data can lead organizations to unfavorable decisions that ripple across their operations. Automated metadata management improves data quality by enforcing consistency and accuracy. Automation tools can set clear standards for data entry and processing, minimizing human error which is often the Achilles’ heel of manual systems.
Consider a healthcare institution that manages patient records. An automated metadata system ensures uniformity in how data is recorded and cross-referenced, thereby increasing accuracy in patient outcomes and compliance with regulatory requirements. Furthermore, it allows for near real-time monitoring of data quality issues, so teams can swiftly act to rectify them.
Facilitated Compliance
Regulatory landscapes are ever-evolving, demanding that organizations stay alert and compliant. Automating metadata management not only simplifies compliance processes but also ensures organizations can swiftly meet audit requirements. Automated systems can provide detailed logs and lineage of data, showcasing provenance and allowing regulatory bodies to trace the data journey easily.
For example, in sectors like finance or pharmaceuticals, where regulatory scrutiny is stringent, having an automated ledger of metadata signifies adherence to standards and enhances trust.
"In today’s data-centric world, compliance is not just about meeting regulations; it's about managing risk effectively."
Accelerated Decision-Making
Speed in decision-making can be the difference between seizing a market opportunity or losing it to competitors. By automating metadata management, organizations can enhance the speed at which they access and analyze relevant data. This agility allows decision-makers to respond to changes in the market or operational conditions more effectively.
For instance, in retail, having automated metadata management enables real-time access to inventory levels, customer preferences, and sales patterns. Decision-makers can derive insights almost instantaneously, leading to agile strategies that meet customer demands promptly.
Technologies Driving Automation
In today's fast-paced digital landscape, organizations increasingly rely on technology to streamline operations, especially in the realm of metadata management. This section delves into the various technologies that play pivotal roles in automating metadata management processes. With a clear understanding of these technologies, professionals and organizations can enhance their data governance frameworks, leading to more efficient operations and better decision-making.
Artificial Intelligence in Metadata Management
Artificial Intelligence (AI) is transforming the way organizations manage and utilize metadata. By employing advanced algorithms, AI significantly accelerates the process of metadata analysis and enhancement. One notable benefit is the ability for AI systems to learn from historical data patterns, thus improving accuracy in cataloging and presenting relevant information.
Consider a scenario where an organization has thousands of datasets, many of which may overlap. An AI-driven metadata management system can automatically identify redundancies and recommend consolidations, thereby improving the overall data quality. This reduces manual effort and allows data stewards to focus on more strategic initiatives.
Moreover, AI enhances search capabilities by enabling natural language processing (NLP). Users can interact with the metadata repository using everyday language, making it intuitive to locate specific datasets. Organizations like IBM Watson offer solutions that showcase these capabilities, streamlining access to critical data resources.
Machine Learning Applications
Alongside AI, machine learning (ML) plays a crucial role in automating metadata management. ML algorithms process large volumes of data to identify patterns and derive insights, which can be invaluable for metadata creation and maintenance. For instance, a ML model trained on past metadata can predict what attributes are essential for future datasets, thus aiding in the structuring and categorization process.
Machine learning's predictive abilities also extend to enhancing data governance protocols. By analyzing usage patterns, ML can identify potential compliance risks or areas requiring further scrutiny, allowing organizations to take proactive measures before issues arise.
In practical terms, platforms like Google Cloud AutoML empower businesses to harness machine learning without needing extensive coding knowledge, thus making this technology accessible to a broader range of users.
Cloud-Based Solutions
Cloud-based solutions have revolutionized metadata management by offering scalable, flexible, and cost-effective options for organizations. These solutions provide centralized repositories for metadata, enabling seamless access and collaboration among teams. This accessibility is vital as it ensures that all stakeholders can engage with the data governance framework without being tethered to specific locations or devices.
Additionally, cloud platforms inherently offer benefits such as automated updates and maintenance, reducing the workload on IT teams. For instance, using Microsoft's Azure Data Catalog, organizations can auto-discover and manage their data assets in real-time, ensuring that metadata remains up-to-date without manual intervention.
An essential consideration when adopting cloud solutions is ensuring data security. With robust encryption and security measures, cloud providers allow organizations to protect sensitive metadata while reaping the benefits of automation.
"In the world of metadata management, the choice of technology is not just about automation; it’s about enabling smarter data governance."
Implementing Metadata Management Automation
The successful implementation of metadata management automation is a cornerstone in establishing robust data governance practices. This process isn't just about deploying tools; it signifies a cultural shift within an organization, promoting an agile and informed approach to data utilization. Businesses today are bending over backwards to ensure they leverage their data assets effectively. As the complexity of data environments grows, so does the imperative to streamline and automate the management of metadata.
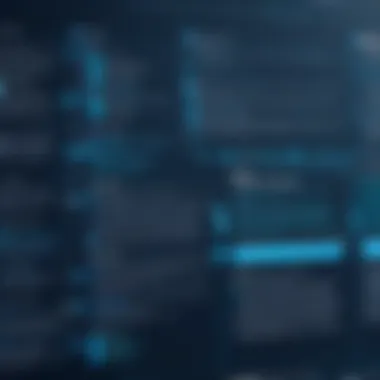
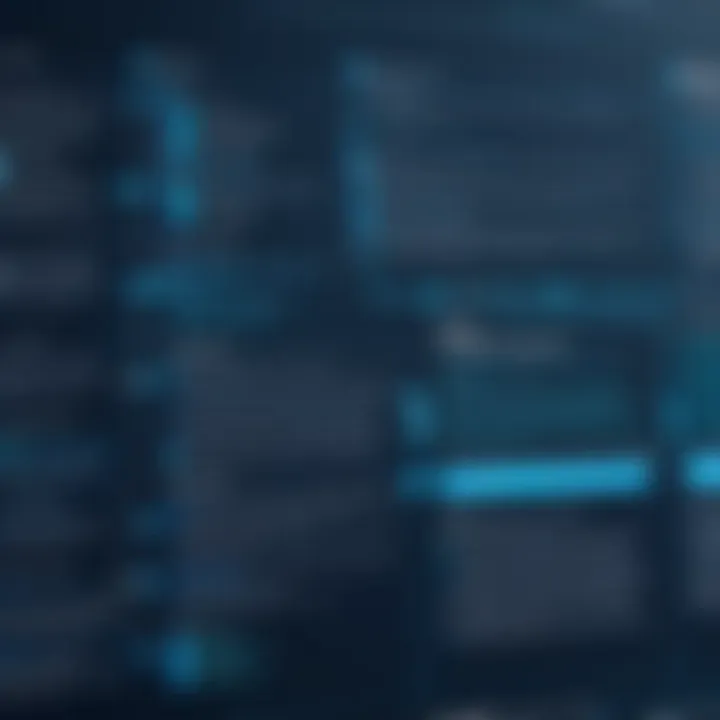
Step-by-Step Implementation Approach
Taking the leap into automation requires a strategic approach. Here’s an outline of a practical step-by-step method:
- Assess Current Processes: Begin by taking stock of current metadata management processes. Engage stakeholders across the organization to pinpoint pain points and areas for improvement. This comprehensive understanding lays the groundwork for effectively tailored solutions.
- Set Clear Objectives: After assessing current processes, define specific goals. Whether it’s reducing manual workload, improving data quality, or increasing compliance, having clear objectives will guide your automation strategy.
- Create a Comprehensive Plan: With goals in hand, map out a detailed plan that includes timelines, resource allocation, and milestones. This roadmap should also address how you will handle potential risks during the transition.
- Conduct a Pilot Test: Before rolling out across the board, consider conducting a pilot test. This approach helps uncover possible hiccups and allows for adjustments before full implementation.
- Measure Success: Post-implementation, it's essential to establish KPIs to measure the success of the automation initiatives. Monitoring outcomes will help refine processes and effectively demonstrate the value added.
Selecting the Right Tools
Choosing the appropriate tools for metadata management automation is pivotal. Not all solutions are created equal, and investing time upfront can save a headache later on. Here are a few considerations:
- User-Friendliness: Tools should not require a PhD to operate. Opt for user-friendly platforms that encourage team-wide adoption and reduce training times.
- Integration Capabilities: Look for solutions that can smoothly integrate with existing systems. A jigsaw puzzle that doesn’t fit will only lead to gaps in efficiency and data quality.
- Scalability: As your organization grows, your tooling needs will too. Ensure that the chosen tools can scale in tandem with your operations, adjusting to the ever-changing landscape of data needs.
- Real-time Monitoring: Effective tools should provide real-time insights into metadata changes and data lineage, enabling immediate action when needed.
Integrating with Existing Systems
Integration is where the rubber meets the road. Seamless integration of new automation tools with legacy systems can be a daunting task. Here are some key points to keep in mind:
- Understand Existing Workflows: Before integration begins, it’s crucial to have a holistic view of how current systems operate. This knowledge aids in understanding where friction points may arise during the integration.
- Ensure Data Compatibility: Different systems might store and use data differently. Check for compatibility to avoid data loss or inconsistencies, which can lead to problems down the line.
- Engage IT and Data Teams: Involvement of IT and data professionals is essential from the outset. Their expertise will guide technical decisions and problem-solving during integration, ensuring smooth sailing.
An effective integration not only brings efficiencies but also fosters a culture of data trust within the organization, where every team member can rely on accurate and timely information for decision-making.
- Training and Support: Every great system needs a support system. Adequate training for the users of the new tools is crucial to foster a smooth transition and adoption across all departments.
Through thoughtful implementation, the journey of automating metadata management can pave the way for better governance and operational efficiency. With the right approach, organizations will find themselves equipped to meet data demands of the future.
Challenges in Automation
In the realm of metadata management, the path to automation is not paved with ease. Understanding the inherent challenges is crucial, as they play a pivotal role in the effective integration and deployment of automated systems. By examining these obstacles, organizations can better prepare for a successful automation journey and enhance their overall data governance framework.
Data Silos
Data silos can act like brick walls in the flow of information across an organization. When teams hoard their data and systems, it becomes nearly impossible to create a unified view of metadata. This disconnection not only hinders the efficiency of automated processes but also contributes to inconsistent data governance practices. For instance, consider a marketing team that relies on its database of customer interactions without sharing its insights with the sales department. If both departments use separate tools and methods, it leads to duplication of effort and wasted resources. To mitigate this issue, organizations should foster a culture of collaboration and invest in integrated tools that encourage sharing data across departments.
Resistance to Change
The reluctance to embrace change can be a formidable barrier to the adoption of automation in metadata management. Many professionals cling to established workflows and systems, fearing the implications of shifting to automated solutions. This often stems from a lack of understanding of how automation could streamline their responsibilities and the overarching fear of job displacement. To combat these fears, organizations must actively engage their teams early in the automation process. Training sessions showcasing the benefits and capabilities of automated systems can ease apprehensions and create advocates for change among staff. In particular, leaders play an important role in demonstrating commitment to technological advancement, which can encourage a more receptive attitude throughout the organization.
Quality Control Standards
When automating metadata management, maintaining quality control standards becomes non-negotiable. Poorly managed automation leads to a cascade of errors, ultimately exacerbating existing issues rather than resolving them. For instance, if an automated system fails to properly extract metadata from various sources, it can result in corrupt or missing data that jeopardizes decision-making processes. Organizations must establish robust protocols and regular checks to ensure the quality of automation outputs. This may involve setting benchmarks, conducting periodic audits, and embracing continuous improvement methodologies. By emphasizing quality control, businesses can safeguard against the pitfalls of hasty automation and sustain the integrity of their metadata frameworks.
"In the rush to automate, one cannot afford to overlook the fundamentals of quality management. It’s one thing to implement technology, another to ensure it delivers value."
Future Trends in Metadata Management Automation
The landscape of metadata management is shifting rapidly, fueled by advancements in technology and the increasing complexities of data governance. As organizations wrestle with massive volumes of data, staying ahead necessitates embracing emerging trends. Here, we elucidate important elements of Future Trends in Metadata Management Automation and their associated benefits.
Increased Adoption of AI-Driven Solutions
Artificial intelligence is no longer just a buzzword; it’s becoming the backbone of innovative metadata management strategies. AI's power lies in its ability to analyze and interpret data at a scale far beyond human capability.
- Efficiency Gains: AI automates repetitive tasks, reducing the manual effort required in metadata tagging and documentation.
- Enhanced Accuracy: With machine learning algorithms, systems can learn from past corrections, improving the quality of metadata over time.
- Predictive Analytics: AI's predictive capabilities enable organizations to forecast trends in data usage, helping businesses to prepare and adapt.
Ultimately, the integration of AI in metadata management leads to streamlined processes and better decision-making, aiding organizations in the quest for a robust data governance framework.
Emergence of Decentralized Approaches
The traditional, centralized model of metadata management is getting a run for its money. The rise of decentralized approaches, largely driven by the need for greater data integrity and resilience, is a game changer.
- Data Ownership: Decentralization encourages a culture of data stewardship, where individual departments take charge of their own metadata.
- Increased Security: Storing metadata across multiple nodes minimizes the risks associated with a single point of failure.
- Collaboration Opportunities: Decentralized systems foster collaboration among teams, allowing for richer metadata development through diverse insights.
As organizations grow, embracing decentralized approaches to metadata management allows them to better manage and utilize data while optimizing resources effectively.
Integration with Blockchain Technology
Blockchain, often recognized for its role in cryptocurrencies, offers a solution for metadata management that extends beyond the financial realm.
- Immutable Records: Blockchain provides an unprecedented level of data integrity. Once information is recorded, it cannot be altered, ensuring that metadata remains accurate and trustworthy.
- Transparency: Blockchain’s open architecture allows stakeholders to access and verify metadata histories, enabling greater confidence in data provenance.
- Smart Contracts: The use of smart contracts can streamline interactions between systems and stakeholders, automating metadata updates when predetermined conditions are met.
In this evolving digital landscape, integrating blockchain technology into metadata management not only secures data but also fosters a more collaborative and transparent environment—key components for effective data governance.
"The future of data management is not just about collecting more data but making it work smarter for us."
In summary, keeping an eye on these trends is crucial for organizations aiming to fortify their metadata management practices and ensure robust governance in an ever-changing environment.
Finale
When we draw the curtain on the critical subject of metadata management automation, it becomes clear just how vital this framework has grown in the realm of data governance. This article has walked through the mere basics to the finer crumbs of how automating these processes holds substantial value for various organizations.
Summary of Key Points
In summary, we traversed the realms of metadata—defining its diverse types, discussing its importance, and laying bare the role it plays within organizations. The need for automation became evident as we unpacked the challenges faced in manual metadata management, from inconsistencies to scalability issues.
While the advantages of automation are apparent, such as improved efficiency and heightened data quality, one cannot ignore the potential challenges that loom. Resistance to change and quality control standards often act as roadblocks, yet the future appears promising. Emerging technologies like AI and blockchain are not just fads; they are tailored to reshape how organizations can harness their data for smarter decision-making.
Final Thoughts on Automation Benefits
As we close this discussion, it is important to acknowledge that adopting an automated approach is not merely a trend; it’s a necessity for businesses seeking sustainability in a rapidly evolving digital era. Businesses today, be it small enterprises or well-established corporations, must recognize that metadata management automation not only streamlines operations but also fortifies data governance.
The benefits prominently showcase operational efficiency, compliance with ever-changing regulations, and the ability to pivot quickly in response to market dynamics. Novices might find the transition daunting, but in the long run, those who embrace automation will undoubtedly stand out as industry leaders. In a world where data drives almost every decision, integrating metadata management automation could very well be the linchpin to thriving in a cutthroat environment.
“In the digital age, it's not just about collecting data, it's about how well you manage it that will set you apart.”