Unraveling the Intricacies of Data Analysis: Navigating a Complex Terrain
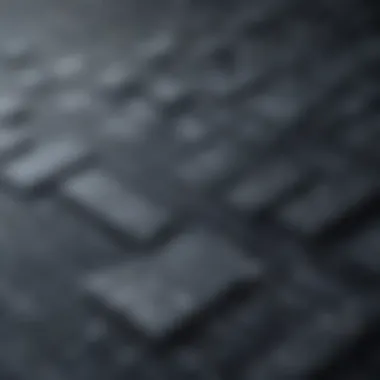
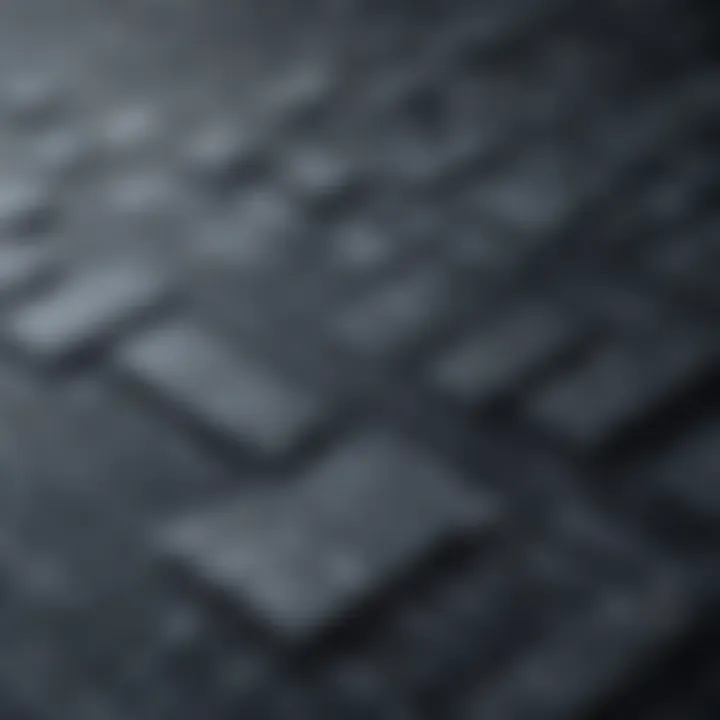
Technological Research Overview
In the realm of data analysis, staying abreast of technological advancements is imperative. Recent technological innovations, ranging from artificial intelligence (AI) to machine learning algorithms, have revolutionized how businesses operate. These innovations have a direct impact on business operations, streamlining processes, enhancing decision-making, and unlocking new avenues for growth. Looking into the future, trends such as blockchain technology and quantum computing are poised to further transform the data analysis landscape.
Data Analytics in Business
Data analytics plays a pivotal role in empowering businesses to make informed decisions. Understanding the importance of data analytics is fundamental to leveraging insights derived from vast datasets. Various tools are available for data analysis, including statistical software, data visualization platforms, and cloud computing services. Real-world case studies showcase organizations that have successfully implemented data-driven strategies to optimize operations, drive revenue, and gain a competitive edge in their respective industries.
Cybersecurity Insights
Navigating the complex landscape of data analysis involves addressing cybersecurity threats. Conducting a thorough threat landscape analysis helps identify vulnerabilities and mitigates potential risks. Best practices in cybersecurity encompass implementing robust encryption protocols, regular security audits, and employee training programs. Adhering to regulatory compliance frameworks ensures data security and fosters trust among consumers and stakeholders.
Artificial Intelligence Applications
Artificial intelligence (AI) is at the forefront of business automation, enhancing productivity and efficiency. AI algorithms power a myriad of applications, from chatbots to predictive analytics, revolutionizing operations across industries. Ethical considerations in AI adoption, such as bias mitigation and data privacy protection, are crucial for fostering responsible AI development and deployment.
Industry-Specific Research
Each industry sector presents unique technological research opportunities. The finance sector explores tech innovations in algorithmic trading and blockchain integration for secure transactions. Healthcare embraces technological advancements like telemedicine and electronic health records to enhance patient care and streamline workflows. The retail industry leverages tech solutions such as augmented reality for immersive customer experiences and data-driven personalization strategies.
Introduction
Data analysis stands as a critical process in today's data-driven world, underpinning decision-making and strategic planning across industries. Understanding the nuances of data analysis is vital for professionals navigating the complex landscape of information interpretation. This section sets the stage for delving into the challenges encountered within data analysis, offering a detailed exploration that sheds light on the intricacies professionals face daily. By unraveling these challenges, we aim to provide a comprehensive guide that equips individuals with the knowledge needed to tackle data analysis hurdles effectively.
Defining Data Analysis
Data analysis in the contemporary landscape
Data analysis in the contemporary landscape represents a fundamental pillar in deciphering vast data sets to extract actionable insights. This analysis approach involves utilizing cutting-edge tools and methodologies to process and interpret data efficiently. The contemporary landscape emphasizes the importance of real-time analysis, enabling timely decision-making based on accurate information. While data analysis in the contemporary landscape streamlines processes and enhances operational efficiency, challenges such as data volume and velocity pose significant hurdles in achieving optimal results. Professionals must grasp the dynamic nature of data analysis in the current landscape to leverage its advantages while mitigating potential drawbacks.
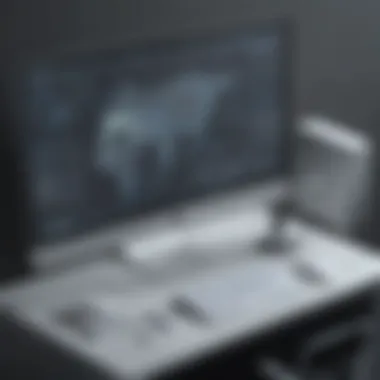
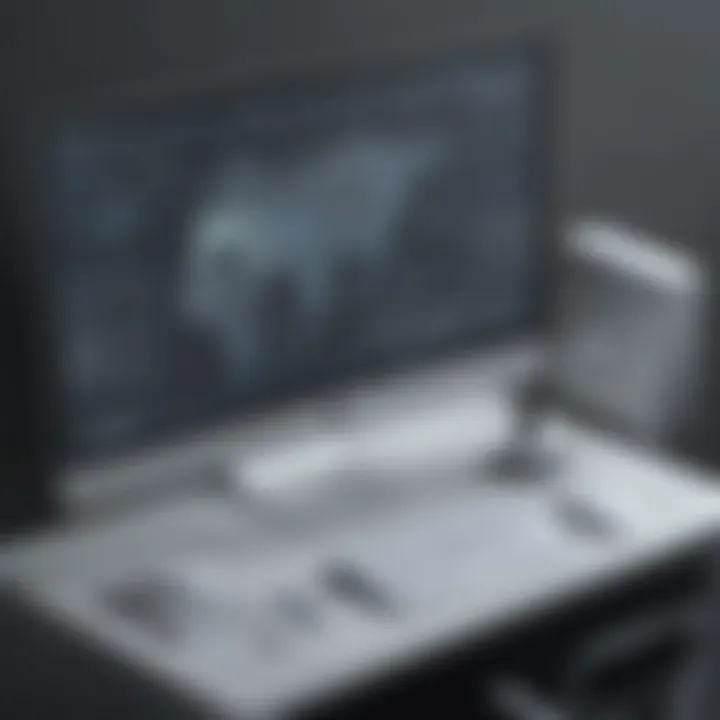
Significance of Data Analysis
Role in decision-making processes
The role of data analysis in decision-making processes cannot be understated, serving as the catalyst for informed choices that drive organizational success. Data analysis empowers leaders to make evidence-based decisions backed by reliable insights derived from thorough data scrutiny. Its pivotal role lies in mitigating risks and enhancing the efficacy of strategic initiatives. Despite its advantages, the complexity of data analysis poses challenges in data interpretation and application, necessitating skilled professionals to navigate the intricacies effectively.
Impact on business strategies
Data analysis plays a crucial role in shaping business strategies, offering a data-driven approach to optimize operations and drive growth. By integrating data analysis into strategic planning, organizations gain a competitive edge through enhanced operational efficiencies and targeted decision-making. The impact of data analysis on business strategies enables companies to adapt to market dynamics swiftly, capitalize on emerging opportunities, and mitigate potential risks effectively. However, harnessing the full potential of data analysis demands adept handling of complexities and aligning analytical insights with strategic goals.
Scope of the Article
Exploring challenges in data analysis
The scope of this article revolves around unraveling the multifaceted challenges encountered in data analysis processes. By examining these challenges in-depth, professionals gain a holistic understanding of the intricacies involved in data interpretation and application. Exploring challenges in data analysis encompasses a diverse range of obstacles, including data quality assurance, privacy concerns, and technological complexities. Insightful analysis of these challenges equips professionals with the knowledge and strategies required to navigate the data analysis landscape successfully, promoting informed decision-making and operational efficiency.
Data Quality
Data quality is a paramount aspect within the realm of data analysis. In the article focusing on challenges in data analysis, the significance of data quality cannot be overstated. Ensuring that datasets are accurate, reliable, and comprehensive is crucial for deriving meaningful insights and making informed decisions. Poor data quality can lead to erroneous conclusions, impacting the reliability and validity of analytical results. Professionals in the data analysis field must pay meticulous attention to data quality to maintain the integrity of their findings and enhance the overall efficacy of their processes.
Incomplete Data Sets
Effects on analysis outcomes
Incomplete data sets pose a significant challenge in data analysis, affecting the reliability and completeness of analytical outcomes. When crucial data points are missing or insufficient, the insights drawn from the analysis may be skewed or inaccurate. This limitation hampers the ability to make well-informed decisions based on incomplete or biased information. Addressing incomplete data sets is essential for improving the accuracy and robustness of data analysis processes, ensuring that insights and conclusions are grounded in comprehensive datasets.
Data Accuracy
Ensuring precision in analysis
Data accuracy is a fundamental pillar of effective data analysis. Ensuring that the data utilized is precise, up-to-date, and free from errors is vital for generating reliable insights and driving informed decision-making. Inaccurate data can lead to flawed analyses, misleading conclusions, and potentially detrimental business actions. By prioritizing data accuracy, professionals can enhance the trustworthiness of their findings and strengthen the overall integrity of their analytical processes.
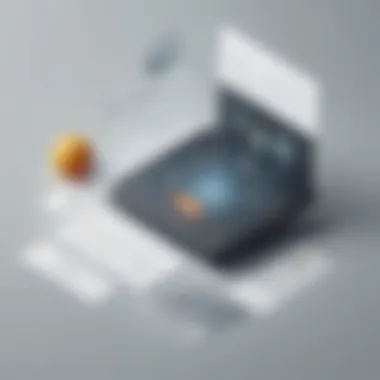
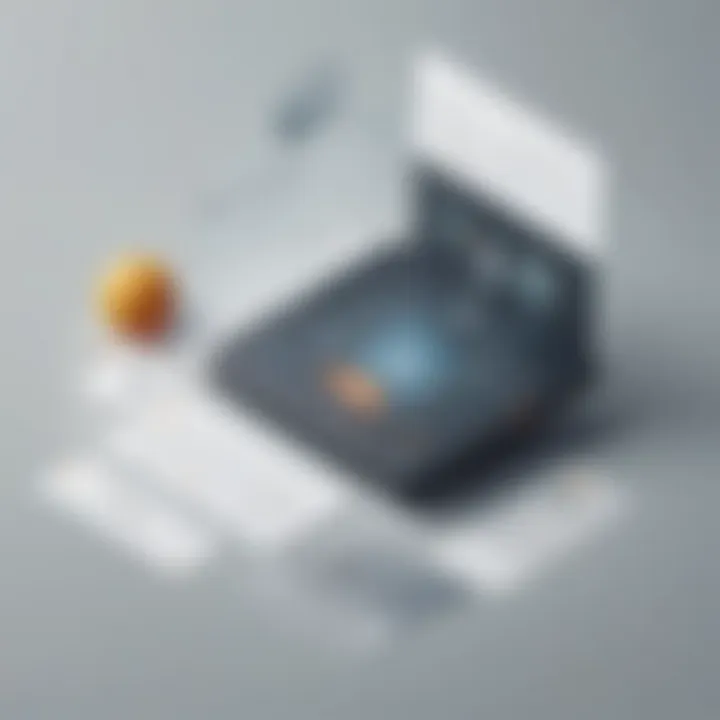
Data Cleaning
Challenges in preprocessing
Data cleaning, though essential, poses several challenges in the preprocessing stage of data analysis. Ensuring data integrity, resolving inconsistencies, handling missing values, and standardizing formats are tasks that demand careful attention and expertise. These challenges can significantly impact the efficiency and accuracy of subsequent analyses. Overcoming obstacles in data cleaning requires robust strategies, advanced tools, and a keen eye for detail to streamline the preprocessing stage and optimize the overall data analysis workflow.
Privacy Concerns
Privacy concerns in the realm of data analysis are of paramount importance. Safeguarding sensitive information from unauthorized access and misuse is a critical aspect that professionals must address diligently. The significance of privacy concerns is underscored by the potential ramifications of data breaches, which can lead to financial losses, damage to reputation, and legal implications. Understanding and implementing robust privacy measures not only uphold ethical standards but also enhance trust and credibility with stakeholders and customers.
Data Security
Mitigating risks of breaches
Mitigating risks of breaches is a crucial component of data security efforts within organizations. By proactively identifying vulnerabilities and implementing preventive measures, such as encryption protocols and access controls, businesses can reduce the likelihood of unauthorized access to sensitive information. This proactive approach not only minimizes the potential impact of security incidents but also demonstrates a commitment to data protection. Mitigating risks of breaches also involves ongoing monitoring and assessment to stay ahead of emerging threats and vulnerabilities, ensuring a proactive stance in safeguarding valuable data assets.
Compliance Regulations
Adhering to data protection laws
Adhering to data protection laws is a fundamental aspect of regulatory compliance for organizations engaged in data analysis. Compliance with laws such as the GDPR and HIPAA not only mitigates legal risks but also underscores a commitment to respecting individual privacy rights. By implementing appropriate measures to secure data, organizations can navigate complex regulatory landscapes and avoid potential penalties or sanctions. Adhering to data protection laws also instills confidence among customers and partners, fostering a positive reputation and enhancing business opportunities.
Technological Aspects
In the realm of data analysis, technological aspects play a pivotal role in shaping the landscape of information processing and decision-making. In this article, the discussion delves into the intricate web of tools and software that propel the field forward, enabling professionals to extract valuable insights from data with precision and efficiency. By honing in on technological aspects, the narrative aims to underscore the significance of implementing advanced systems and applications to enhance analytical processes and strategic outcomes.
Tools and Software
Optimizing data analysis processes
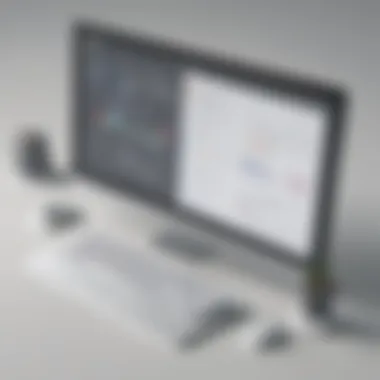
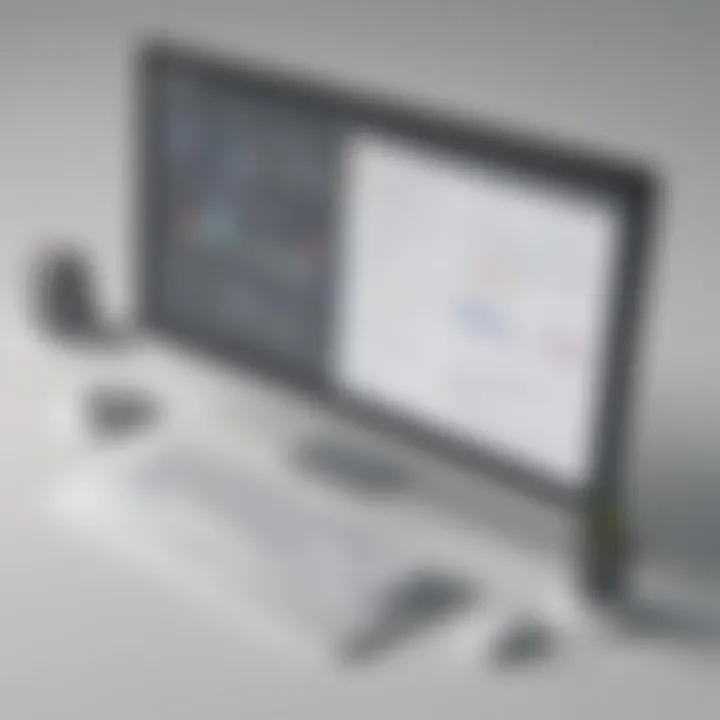
Optimizing data analysis processes stands out as a crucial facet in the successful execution of analytical tasks. This specific aspect focuses on streamlining operations, reducing processing time, and improving the accuracy of analytical results. The key characteristic of optimizing data analysis processes lies in its ability to automate repetitive tasks, integrate diverse data sets seamlessly, and facilitate real-time analysis. This strategic approach proves to be a popular choice in this article due to its proven track record of enhancing workflow efficiency, identifying trends rapidly, and enabling swift decision-making based on data-driven insights. The unique feature of optimizing data analysis processes lies in its capacity to scale operations, adapt to evolving data requirements, and deliver consistent performance over time. While advantageous in expediting analysis, optimizing data analysis processes may pose challenges in terms of initial setup complexity, resource-intensive implementation, and integration issues with existing systems.
Data Integration
Challenges in merging diverse data sources pose a significant consideration in the realm of data analysis, emphasizing the importance of harmonizing disparate information streams for cohesive analysis. This aspect contributes to the overall topic by highlighting the critical need to consolidate data from various sources, ensuring data consistency, and enabling comprehensive analysis of interconnected datasets. The key characteristic of challenges in merging diverse data sources revolves around the complexity of integrating structured and unstructured data, different data formats, and data from multiple platforms effectively. This aspect emerges as a beneficial choice for this article as it addresses the common hurdle faced by professionals in unifying data silos, fostering cross-functional insights, and enhancing analytical completeness. The unique feature of challenges in merging diverse data sources lies in its ability to uncover hidden patterns, reveal correlation insights, and support robust decision-making based on a holistic view of data. While advantageous in promoting data synergy, challenges in merging diverse data sources may present disadvantages such as data inconsistencies, interoperability issues, and data security vulnerabilities within interconnected systems.
Resource Allocation
Resource allocation plays a pivotal role in the context of data analysis challenges. Efficient management and distribution of resources are crucial for the success of projects in this domain. It involves allocating funds, manpower, and technology judiciously to maximize output and minimize bottlenecks. Effective resource allocation ensures that data analysis tasks are completed within budget and on schedule, enhancing overall project efficiency. By focusing on resource allocation, organizations can streamline their operations, optimize performance, and achieve better outcomes in data analysis initiatives.
Budget Constraints
Effect on data analysis projects
Budget constraints have a significant impact on data analysis projects, influencing the scope, quality, and timeliness of deliverables. Limited financial resources can impede the adoption of advanced technologies, hinder data collection efforts, and constrain the hiring of skilled professionals. Such constraints may lead to compromised data integrity, reduced analytical capabilities, and delayed project timelines. Despite its challenges, managing budget constraints encourages resourcefulness, innovation, and prioritization of essential tasks. Organizations must carefully balance cost considerations with quality requirements to maintain project relevance and effectiveness.
Human Capital
Skills gap and training needs
The skills gap and training needs factor prominently in the landscape of data analysis challenges. In a rapidly evolving field driven by technology advancements, professionals often face gaps in expertise and must continually upskill to remain competitive. Bridging these gaps through targeted training programs and professional development initiatives is essential for cultivating a skilled workforce capable of addressing complex data analysis requirements effectively. Identifying specific skill requirements, fostering a learning culture, and investing in employee training empowers individuals to adapt to changing demands and contribute meaningfully to data analysis projects. While addressing skills gaps can be resource-intensive, the long-term benefits of a proficient workforce far outweigh the initial investment, ensuring sustained productivity and innovation.
Conclusion
Challenges in data analysis represent a critical aspect of the modern business landscape, demanding considerable attention and strategic planning. In this article, the Conclusion section serves as a pivotal point in highlighting the significance of addressing these challenges effectively to propel organizations towards data-driven success. By delving into the nuances of data analysis obstacles and exploring viable solutions, professionals can optimize decision-making processes and enhance business strategies, fostering a competitive edge in the market. The Conclusion segment encapsulates the essential elements discussed throughout, emphasizing the imperative need for proactive problem-solving and continuous improvement initiatives to navigate the complex data analysis terrain successfully.
Addressing Data Analysis Challenges
Strategies for Overcoming Obstacles
Strategies for overcoming obstacles in data analysis stand out as a cornerstone for ensuring operational efficiency and maximizing insights from data. This section delves into specific methodologies and frameworks designed to address common hurdles such as data quality issues, privacy concerns, and resource constraints. By implementing robust strategies that focus on data governance, quality assurance, and compliance adherence, organizations can streamline their analytical processes and derive meaningful interpretations from diverse data sets. The strategic deployment of advanced analytics tools and machine learning algorithms also plays a pivotal role in enhancing predictive capabilities and uncovering hidden patterns within complex data structures. However, it is essential to note the nuances of each strategy and adapt them to the unique requirements of the organization to achieve optimal outcomes efficiently.
Looking to the Future
Evolution of Data Analysis Practices
The evolution of data analysis practices signifies a transformative shift towards more agile, scalable, and intelligent analytical frameworks. This section accentuates the dynamic nature of data analytics, underlining the integration of artificial intelligence, big data technologies, and IoT solutions in shaping the future landscape of data-driven decision-making. By embracing emerging trends such as automated analytics, prescriptive modeling, and augmented analytics, organizations can harness the power of data more effectively to drive innovation and competitive advantage. The evolving role of data scientists and analysts in leveraging cutting-edge tools for deep learning and natural language processing further underscores the paradigm shift towards more sophisticated and efficient data analysis methodologies. While this evolution presents exciting opportunities for organizations to unlock new value from their data assets, it also necessitates continuous upskilling and adaptation to stay ahead of the curve in an increasingly data-centric business environment.